- Search Menu
- Sign in through your institution
- Advance Articles
- Author Guidelines
- Open Access Policy
- Self-Archiving Policy
- About Significance
- About The Royal Statistical Society
- Editorial Board
- Advertising & Corporate Services
- Journals on Oxford Academic
- Books on Oxford Academic

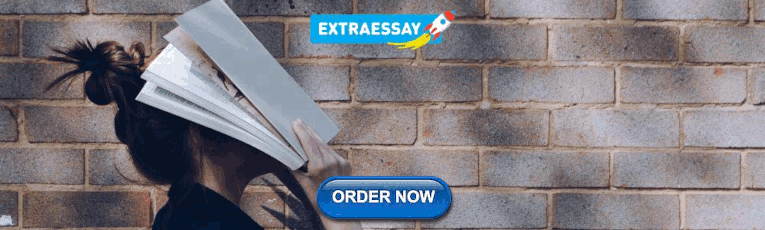
Article Contents
What is randomisation, why do we randomise, choosing a randomisation method, implementing the chosen randomisation method.
- < Previous
Randomisation: What, Why and How?
- Article contents
- Figures & tables
- Supplementary Data
Zoë Hoare, Randomisation: What, Why and How?, Significance , Volume 7, Issue 3, September 2010, Pages 136–138, https://doi.org/10.1111/j.1740-9713.2010.00443.x
- Permissions Icon Permissions
Randomisation is a fundamental aspect of randomised controlled trials, but how many researchers fully understand what randomisation entails or what needs to be taken into consideration to implement it effectively and correctly? Here, for students or for those about to embark on setting up a trial, Zoë Hoare gives a basic introduction to help approach randomisation from a more informed direction.
Most trials of new medical treatments, and most other trials for that matter, now implement some form of randomisation. The idea sounds so simple that defining it becomes almost a joke: randomisation is “putting participants into the treatment groups randomly”. If only it were that simple. Randomisation can be a minefield, and not everyone understands what exactly it is or why they are doing it.
A key feature of a randomised controlled trial is that it is genuinely not known whether the new treatment is better than what is currently offered. The researchers should be in a state of equipoise; although they may hope that the new treatment is better, there is no definitive evidence to back this hypothesis up. This evidence is what the trial is trying to provide.
You will have, at its simplest, two groups: patients who are getting the new treatment, and those getting the control or placebo. You do not hand-select which patient goes into which group, because that would introduce selection bias. Instead you allocate your patients randomly. In its simplest form this can be done by the tossing of a fair coin: heads, the patient gets the trial treatment; tails, he gets the control. Simple randomisation is a fair way of ensuring that any differences that occur between the treatment groups arise completely by chance. But – and this is the first but of many here – simple randomisation can lead to unbalanced groups, that is, groups of unequal size. This is particularly true if the trial is only small. For example, tossing a fair coin 10 times will only result in five heads and five tails about 25% of the time. We would have a 66% chance of getting 6 heads and 4 tails, 5 and 5, or 4 and 6; 33% of the time we would get an even larger imbalance, with 7, 8, 9 or even all 10 patients in one group and the other group correspondingly undersized.
The impact of an imbalance like this is far greater for a small trial than for a larger trial. Tossing a fair coin 100 times will result in imbalance larger than 60–40 less than 1% of the time. One important part of the trial design process is the statement of intention of using randomisation; then we need to establish which method to use, when it will be used, and whether or not it is in fact random.
Randomisation needs to be controlled: You would not want all the males under 30 to be in one trial group and all the women over 70 in the other
It is partly true to say that we do it because we have to. The Consolidated Standards of Reporting Trials (CONSORT) 1 , to which we should all adhere, tells us: “Ideally, participants should be assigned to comparison groups in the trial on the basis of a chance (random) process characterized by unpredictability.” The requirement is there for a reason. Randomisation of the participants is crucial because it allows the principles of statistical theory to stand and as such allows a thorough analysis of the trial data without bias. The exact method of randomisation can have an impact on the trial analyses, and this needs to be taken into account when writing the statistical analysis plan.
Ideally, simple randomisation would always be the preferred option. However, in practice there often needs to be some control of the allocations to avoid severe imbalances within treatments or within categories of patient. You would not want, for example, all the males under 30 to be in one group and all the females over 70 in the other. This is where restricted or stratified randomisation comes in.
Restricted randomisation relates to using any method to control the split of allocations to each of the treatment groups based on certain criteria. This can be as simple as generating a random list, such as AAABBBABABAABB …, and allocating each participant as they arrive to the next treatment on the list. At certain points within the allocations we know that the groups will be balanced in numbers – here at the sixth, eighth, tenth and 14th participants – and we can control the maximum imbalance at any one time.
Stratified randomisation sets out to control the balance in certain baseline characteristics of the participants – such as sex or age. This can be thought of as producing an individual randomisation list for each of the characteristics concerned.

© iStockphoto.com/dra_schwartz
Stratification variables are the baseline characteristics that you think might influence the outcome your trial is trying to measure. For example, if you thought gender was going to have an effect on the efficacy of the treatment then you would use it as one of your stratification variables. A stratified randomisation procedure would aim to ensure a balance of the two gender groups between the two treatment groups.
If you also thought age would be affecting the treatment then you could also stratify by age (young/old) with some sensible limits on what old and young are. Once you start stratifying by age and by gender, you have to start taking care. You will need to use a stratified randomisation process that balances at the stratum level (i.e. at the level of those characteristics) to ensure that all four strata (male/young, male/old, female/young and female/old) have equivalent numbers of each of the treatment groups represented.
“Great”, you might think. “I'll just stratify by all my baseline characteristics!” Better not. Stop and consider what this would mean. As the number of stratification variables increases linearly, the number of strata increases exponentially. This reduces the number of participants that would appear in each stratum. In our example above, with our two stratification variables of age and sex we had four strata; if we added, say “blue-eyed” and “overweight” to our criteria to give four stratification variables each with just two levels we would get 16 represented strata. How likely is it that each of those strata will be represented in the population targeted by the trial? In other words, will we be sure of finding a blue-eyed young male who is also overweight among our patients? And would one such overweight possible Adonis be statistically enough? It becomes evident that implementing pre-generated lists within each stratification level or stratum and maintaining an overall balance of group sizes becomes much more complicated with many stratification variables and the uncertainty of what type of participant will walk through the door next.
Does it matter? There are a wide variety of methods for randomisation, and which one you choose does actually matter. It needs to be able to do everything that is required of it. Ask yourself these questions, and others:
Can the method accommodate enough treatment groups? Some methods are limited to two treatment groups; many trials involve three or more.
What type of randomness, if any, is injected into the method? The level of randomness dictates how predictable a method is.
A deterministic method has no randomness, meaning that with all the previous information you can tell in advance which group the next patient to appear will be allocated to. Allocating alternate participants to the two treatments using ABABABABAB … would be an example.
A static random element means that each allocation is made with a pre-defined probability. The coin-toss method does this.
With a dynamic element the probability of allocation is always changing in relation to the information received, meaning that the probability of allocation can only be worked out with knowledge of the algorithm together with all its settings. A biased coin toss does this where the bias is recalculated for each participant.
Can the method accommodate stratification variables, and if so how many? Not all of them can. And can it cope with continuous stratification variables? Most variables are divided into mutually exclusive categories (e.g. male or female), but sometimes it may be necessary (or preferable) to use a continuous scale of the variable – such as weight, or body mass index.
Can the method use an unequal allocation ratio? Not all trials require equal-sized treatment groups. There are many reasons why it might be wise to have more patients receiving treatment A than treatment B 2 . However, an allocation ratio being something other than 1:1 does impact on the study design and on the calculation of the sample size, so is not something to be changing mid-trial. Not all allocation methods can cope with this inequality.
Is thresholding used in the method? Thresholding handles imbalances in allocation. A threshold is set and if the imbalance becomes greater than the threshold then the allocation becomes deterministic to reduce the imbalance back below the threshold.
Can the method be implemented sequentially? In other words, does it require that the total number of participants be known at the beginning of the allocations? Some methods generate lists requiring exactly N participants to be recruited in order to be effective – and recruiting participants is often one of the more problematic parts of a trial.
Is the method complex? If so, then its practical implementation becomes an issue for the day-to-day running of the trial.
Is the method suitable to apply to a cluster randomisation? Cluster randomisations are used when randomising groups of individuals to a treatment rather than the individuals themselves. This can be due to the nature of the treatment, such as a new teaching method for schools or a dietary intervention for families. Using clusters is a big part of the trial design and the randomisation needs to be handled slightly differently.
Should a response-adaptive method be considered? If there is some evidence that one treatment is better than another, then a response-adaptive method works by taking into account the outcomes of previous allocations and works to minimise the number of participants on the “wrong” treatment.
For multi-centred trials, how to handle the randomisations across the centres should be considered at this point. Do all centres need to be completely balanced? Are all centres the same size? Considering the various centres as stratification variables is one way of dealing with more than one centre.
Once the method of randomisation has been established the next important step is to consider how to implement it. The recommended way is to enlist the services of a central randomisation office that can offer robust, validated techniques with the security and back-up needed to implement many of the methods proposed today. How the method is implemented must be as clearly reported as the method chosen. As part of the implementation it is important to keep the allocations concealed, both those already done and any future ones, from as many people as possible. This helps prevent selection bias: a clinician may withhold a participant if he believes that based on previous allocations the next allocations would not be the “preferred” ones – see the section below on subversion.
Part of the trial design will be to note exactly who should know what about how each participant has been allocated. Researchers and participants may be equally blinded, but that is not always the case.
For example, in a blinded trial there may be researchers who do not know which group the participants have been allocated to. This enables them to conduct the assessments without any bias for the allocation. They may, however, start to guess, on the basis of the results they see. A measure of blinding may be incorporated for the researchers to indicate whether they have remained blind to the treatment allocated. This can be in the form of a simple scale tool for the researcher to indicate how confident they are in knowing which allocated group the participant is in by the end of an assessment. With psychosocial interventions it is often impossible to hide from the participants, let alone the clinicians, which treatment group they have been allocated to.
In a drug trial where a placebo can be prescribed a coded system can ensure that neither patients nor researchers know which group is which until after the analysis stage.
With any level of blinding there may be a requirement to unblind participants or clinicians at any point in the trial, and there should be a documented procedure drawn up on how to unblind a particular participant without risking the unblinding of a trial. For drug trials in particular, the methods for unblinding a participant must be stated in the trial protocol. Wherever possible the data analysts and statisticians should remain blind to the allocation until after the main analysis has taken place.
Blinding should not be confused with allocation concealment. Blinding prevents performance and ascertainment bias within a trial, while allocation concealment prevents selection bias. Bias introduced by poor allocation concealment may be thought of as a predictive bias, trying to influence the results from the outset, while the biases introduced by non-blinding can be thought of as a reactive bias, creating causal links in outcomes because of being in possession of information about the treatment group.
In the literature on randomisation there are numerous tales of how allocation schemes have been subverted by clinicians trying to do the best for the trial or for their patient or both. This includes anecdotal tales of clinicians holding sealed envelopes containing the allocations up to X-ray lights and confessing to breaking into locked filing cabinets to get at the codes 3 . This type of behaviour has many explanations and reasons, but does raise the question whether these clinicians were in a state of equipoise with regard to the trial, and whether therefore they should really have been involved with the trial. Randomisation schemes and their implications must be signed up to by the whole team and are not something that only the participants need to consent to.
Clinicians have been known to X-ray sealed allocation envelopes to try to get their patients into the preferred group in a trial
The 2010 CONSORT statement can be found at http://www.consort-statement.org/consort-statement/ .
Dumville , J. C. , Hahn , S. , Miles , J. N. V. and Torgerson , D. J. ( 2006 ) The use of unequal randomisation ratios in clinical trials: A review . Contemporary Clinical Trials , 27 , 1 – 12 .
Google Scholar
Shulz , K. F. ( 1995 ) Subverting randomisation in controlled trials . Journal of the American Medical Association , 274 , 1456 – 1458 .
Month: | Total Views: |
---|---|
February 2023 | 6 |
March 2023 | 6 |
April 2023 | 7 |
May 2023 | 16 |
June 2023 | 24 |
July 2023 | 19 |
August 2023 | 45 |
September 2023 | 106 |
October 2023 | 139 |
November 2023 | 234 |
December 2023 | 184 |
January 2024 | 239 |
February 2024 | 197 |
March 2024 | 183 |
April 2024 | 140 |
May 2024 | 167 |
June 2024 | 142 |
July 2024 | 208 |
August 2024 | 152 |
Email alerts
Citing articles via.
- Recommend to Your Librarian
- Advertising & Corporate Services
- Journals Career Network
Affiliations
- Online ISSN 1740-9713
- Print ISSN 1740-9705
- Copyright © 2024 Royal Statistical Society
- About Oxford Academic
- Publish journals with us
- University press partners
- What we publish
- New features
- Open access
- Institutional account management
- Rights and permissions
- Get help with access
- Accessibility
- Advertising
- Media enquiries
- Oxford University Press
- Oxford Languages
- University of Oxford
Oxford University Press is a department of the University of Oxford. It furthers the University's objective of excellence in research, scholarship, and education by publishing worldwide
- Copyright © 2024 Oxford University Press
- Cookie settings
- Cookie policy
- Privacy policy
- Legal notice
This Feature Is Available To Subscribers Only
Sign In or Create an Account
This PDF is available to Subscribers Only
For full access to this pdf, sign in to an existing account, or purchase an annual subscription.
What is a Randomized Control Trial (RCT)?
Julia Simkus
Editor at Simply Psychology
BA (Hons) Psychology, Princeton University
Julia Simkus is a graduate of Princeton University with a Bachelor of Arts in Psychology. She is currently studying for a Master's Degree in Counseling for Mental Health and Wellness in September 2023. Julia's research has been published in peer reviewed journals.
Learn about our Editorial Process
Saul McLeod, PhD
Editor-in-Chief for Simply Psychology
BSc (Hons) Psychology, MRes, PhD, University of Manchester
Saul McLeod, PhD., is a qualified psychology teacher with over 18 years of experience in further and higher education. He has been published in peer-reviewed journals, including the Journal of Clinical Psychology.
Olivia Guy-Evans, MSc
Associate Editor for Simply Psychology
BSc (Hons) Psychology, MSc Psychology of Education
Olivia Guy-Evans is a writer and associate editor for Simply Psychology. She has previously worked in healthcare and educational sectors.
On This Page:
A randomized control trial (RCT) is a type of study design that involves randomly assigning participants to either an experimental group or a control group to measure the effectiveness of an intervention or treatment.
Randomized Controlled Trials (RCTs) are considered the “gold standard” in medical and health research due to their rigorous design.
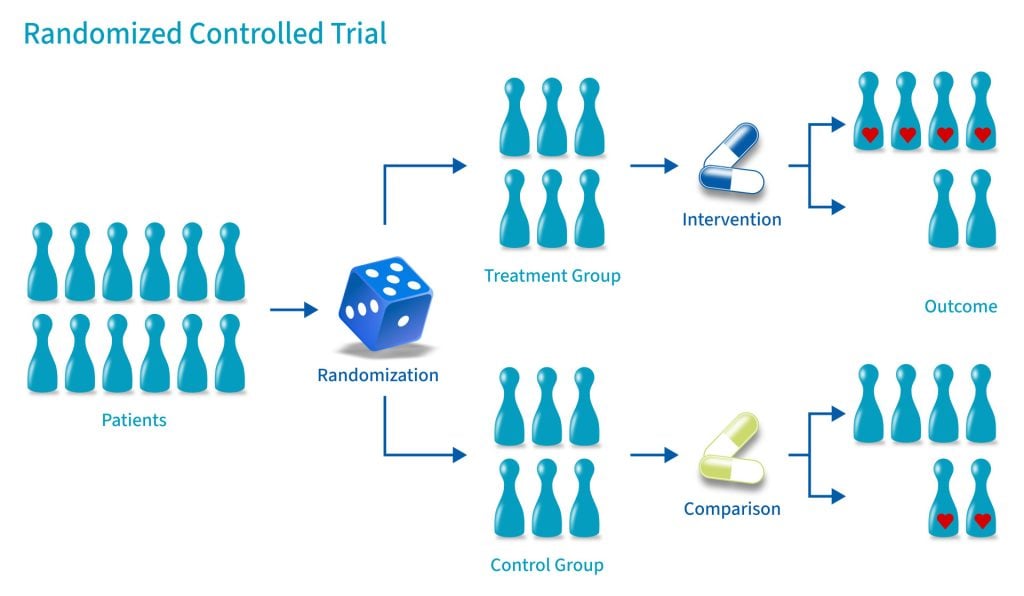
Control Group
A control group consists of participants who do not receive any treatment or intervention but a placebo or reference treatment. The control participants serve as a comparison group.
The control group is matched as closely as possible to the experimental group, including age, gender, social class, ethnicity, etc.
Because the participants are randomly assigned, the characteristics between the two groups should be balanced, enabling researchers to attribute any differences in outcome to the study intervention.
Since researchers can be confident that any differences between the control and treatment groups are due solely to the effects of the treatments, scientists view RCTs as the gold standard for clinical trials.
Random Allocation
Random allocation and random assignment are terms used interchangeably in the context of a randomized controlled trial (RCT).
Both refer to assigning participants to different groups in a study (such as a treatment group or a control group) in a way that is completely determined by chance.
The process of random assignment controls for confounding variables , ensuring differences between groups are due to chance alone.
Without randomization, researchers might consciously or subconsciously assign patients to a particular group for various reasons.
Several methods can be used for randomization in a Randomized Control Trial (RCT). Here are a few examples:
- Simple Randomization: This is the simplest method, like flipping a coin. Each participant has an equal chance of being assigned to any group. This can be achieved using random number tables, computerized random number generators, or drawing lots or envelopes.
- Block Randomization: In this method, participants are randomized within blocks, ensuring that each block has an equal number of participants in each group. This helps to balance the number of participants in each group at any given time during the study.
- Stratified Randomization: This method is used when researchers want to ensure that certain subgroups of participants are equally represented in each group. Participants are divided into strata, or subgroups, based on characteristics like age or disease severity, and then randomized within these strata.
- Cluster Randomization: In this method, groups of participants (like families or entire communities), rather than individuals, are randomized.
- Adaptive Randomization: In this method, the probability of being assigned to each group changes based on the participants already assigned to each group. For example, if more participants have been assigned to the control group, new participants will have a higher probability of being assigned to the experimental group.
Computer software can generate random numbers or sequences that can be used to assign participants to groups in a simple randomization process.
For more complex methods like block, stratified, or adaptive randomization, computer algorithms can be used to consider the additional parameters and ensure that participants are assigned to groups appropriately.
Using a computerized system can also help to maintain the integrity of the randomization process by preventing researchers from knowing in advance which group a participant will be assigned to (a principle known as allocation concealment). This can help to prevent selection bias and ensure the validity of the study results .
Allocation Concealment
Allocation concealment is a technique to ensure the random allocation process is truly random and unbiased.
RCTs use allocation concealment to decide which patients get the real medicine and which get a placebo (a fake medicine)
It involves keeping the sequence of group assignments (i.e., who gets assigned to the treatment group and who gets assigned to the control group next) hidden from the researchers before a participant has enrolled in the study.
This helps to prevent the researchers from consciously or unconsciously selecting certain participants for one group or the other based on their knowledge of which group is next in the sequence.
Allocation concealment ensures that the investigator does not know in advance which treatment the next person will get, thus maintaining the integrity of the randomization process.
Blinding (Masking)
Binding, or masking, refers to withholding information regarding the group assignments (who is in the treatment group and who is in the control group) from the participants, the researchers, or both during the study .
A blinded study prevents the participants from knowing about their treatment to avoid bias in the research. Any information that can influence the subjects is withheld until the completion of the research.
Blinding can be imposed on any participant in an experiment, including researchers, data collectors, evaluators, technicians, and data analysts.
Good blinding can eliminate experimental biases arising from the subjects’ expectations, observer bias, confirmation bias, researcher bias, observer’s effect on the participants, and other biases that may occur in a research test.
In a double-blind study , neither the participants nor the researchers know who is receiving the drug or the placebo. When a participant is enrolled, they are randomly assigned to one of the two groups. The medication they receive looks identical whether it’s the drug or the placebo.
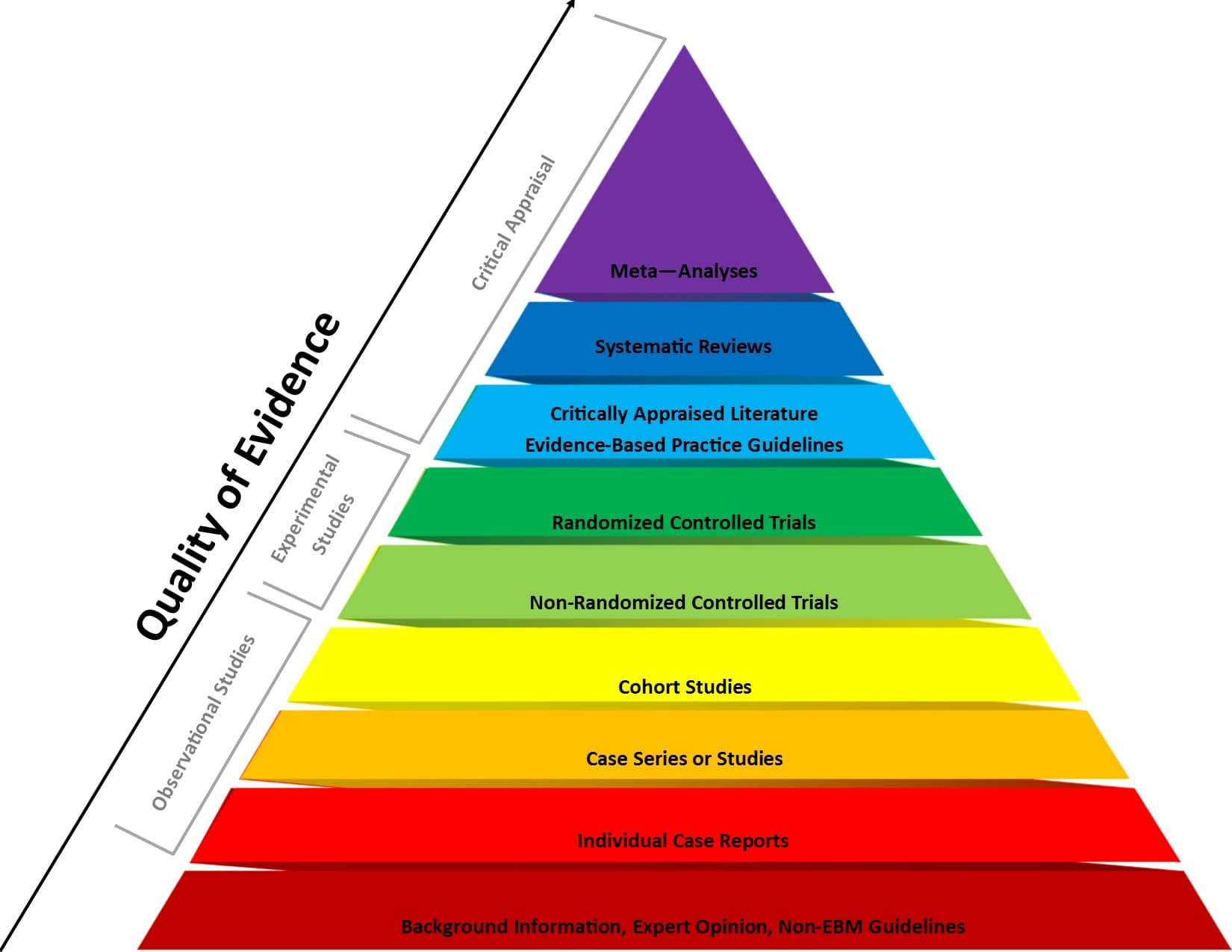
Figure 1 . Evidence-based medicine pyramid. The levels of evidence are appropriately represented by a pyramid as each level, from bottom to top, reflects the quality of research designs (increasing) and quantity (decreasing) of each study design in the body of published literature. For example, randomized control trials are higher quality and more labor intensive to conduct, so there is a lower quantity published.
Resesearch Designs
The choice of design should be guided by the research question, the nature of the treatments or interventions being studied, practical considerations (like sample size and resources), and ethical considerations (such as ensuring all participants have access to potentially beneficial treatments).
The goal is to select a design that provides the most valid and reliable answers to your research questions while minimizing potential biases and confounds.
1. Between-participants randomized designs
Between-participant design involves randomly assigning participants to different treatment conditions. In its simplest form, it has two groups: an experimental group receiving the treatment and a control group.
With more than two levels, multiple treatment conditions are compared. The key feature is that each participant experiences only one condition.
This design allows for clear comparison between groups without worrying about order effects or carryover effects.
It’s particularly useful for treatments that have lasting impacts or when experiencing one condition might influence how participants respond to subsequent conditions.
A study testing a new antidepressant medication might randomly assign 100 participants to either receive the new drug or a placebo.
The researchers would then compare depression scores between the two groups after a specified treatment period to determine if the new medication is more effective than the placebo.
Use this design when:
- You want to compare the effects of different treatments or interventions
- Carryover effects are likely (e.g., learning effects or lasting physiological changes)
- The treatment effect is expected to be permanent
- You have a large enough sample size to ensure groups are equivalent through randomization
2. Factorial designs
Factorial designs investigate the effects of two or more independent variables simultaneously. They allow researchers to study both main effects of each variable and interaction effects between variables.
These can be between-participants (different groups for each combination of conditions), within-participants (all participants experience all conditions), or mixed (combining both approaches).
Factorial designs allow researchers to examine how different factors combine to influence outcomes, providing a more comprehensive understanding of complex phenomena.
They’re more efficient than running separate studies for each variable and can reveal important interactions that might be missed in simpler designs.
A study examining the effects of both exercise intensity (high vs. low) and diet type (high-protein vs. high-carb) on weight loss might use a 2×2 factorial design.
Participants would be randomly assigned to one of four groups: high-intensity exercise with high-protein diet, high-intensity exercise with high-carb diet, low-intensity exercise with high-protein diet, or low-intensity exercise with high-carb diet.
- You want to study the effects of multiple independent variables simultaneously
- You’re interested in potential interactions between variables
- You want to increase the efficiency of your study by testing multiple hypotheses at once
3. Cluster randomized designs
In cluster randomized trials, groups or “clusters” of participants are randomized to treatment conditions, rather than individuals.
This is often used when individual randomization is impractical or when the intervention is naturally applied at a group level.
It’s particularly useful in educational or community-based research where individual randomization might be disruptive or lead to treatment diffusion.
A study testing a new teaching method might randomize entire classrooms to either use the new method or continue with the standard curriculum.
The researchers would then compare student outcomes between the classrooms using the different methods, rather than randomizing individual students.
- You have a smaller sample size available
- Individual differences are likely to be large
- The effects of the treatment are temporary
- You can effectively control for order and carryover effects
4. Within-participants (repeated measures) designs
In these designs, each participant experiences all treatment conditions, serving as their own control.
Within-participants designs are more statistically powerful as they control for individual differences. They require fewer participants, making them more efficient.
However, they’re only appropriate when the treatment effects are temporary and when you can effectively counterbalance to control for order effects.
A study on the effects of caffeine on cognitive performance might have participants complete cognitive tests on three separate occasions: after consuming no caffeine, a low dose of caffeine, and a high dose of caffeine.
The order of these conditions would be counterbalanced across participants to control for order effects.
5. Crossover designs
Crossover designs are a specific type of within-participants design where participants receive different treatments in different time periods.
This allows each participant to serve as their own control and can be more efficient than between-participants designs.
Crossover designs combine the benefits of within-participants designs (increased power, control for individual differences) with the ability to compare different treatments.
They’re particularly useful in clinical trials where you want each participant to experience all treatments, but need to ensure that the effects of one treatment don’t carry over to the next.
A study comparing two different pain medications might have participants use one medication for a month, then switch to the other medication for another month after a washout period.
Pain levels would be measured during both treatment periods, allowing for within-participant comparisons of the two medications’ effectiveness.
- You want to compare the effects of different treatments within the same individuals
- The treatments have temporary effects with a known washout period
- You want to increase statistical power while using a smaller sample size
- You want to control for individual differences in response to treatment
Prevents bias
In randomized control trials, participants must be randomly assigned to either the intervention group or the control group, such that each individual has an equal chance of being placed in either group.
This is meant to prevent selection bias and allocation bias and achieve control over any confounding variables to provide an accurate comparison of the treatment being studied.
Because the distribution of characteristics of patients that could influence the outcome is randomly assigned between groups, any differences in outcome can be explained only by the treatment.
High statistical power
Because the participants are randomized and the characteristics between the two groups are balanced, researchers can assume that if there are significant differences in the primary outcome between the two groups, the differences are likely to be due to the intervention.
This warrants researchers to be confident that randomized control trials will have high statistical power compared to other types of study designs.
Since the focus of conducting a randomized control trial is eliminating bias, blinded RCTs can help minimize any unconscious information bias.
In a blinded RCT, the participants do not know which group they are assigned to or which intervention is received. This blinding procedure should also apply to researchers, health care professionals, assessors, and investigators when possible.
“Single-blind” refers to an RCT where participants do not know the details of the treatment, but the researchers do.
“ Double-blind ” refers to an RCT where both participants and data collectors are masked of the assigned treatment.
Limitations
Costly and timely.
Some interventions require years or even decades to evaluate, rendering them expensive and time-consuming.
It might take an extended period of time before researchers can identify a drug’s effects or discover significant results.
Requires large sample size
There must be enough participants in each group of a randomized control trial so researchers can detect any true differences or effects in outcomes between the groups.
Researchers cannot detect clinically important results if the sample size is too small.
Change in population over time
Because randomized control trials are longitudinal in nature, it is almost inevitable that some participants will not complete the study, whether due to death, migration, non-compliance, or loss of interest in the study.
This tendency is known as selective attrition and can threaten the statistical power of an experiment.
Randomized control trials are not always practical or ethical, and such limitations can prevent researchers from conducting their studies.
For example, a treatment could be too invasive, or administering a placebo instead of an actual drug during a trial for treating a serious illness could deny a participant’s normal course of treatment. Without ethical approval, a randomized control trial cannot proceed.
Fictitious Example
An example of an RCT would be a clinical trial comparing a drug’s effect or a new treatment on a select population.
The researchers would randomly assign participants to either the experimental group or the control group and compare the differences in outcomes between those who receive the drug or treatment and those who do not.
Real-life Examples
- Preventing illicit drug use in adolescents: Long-term follow-up data from a randomized control trial of a school population (Botvin et al., 2000).
- A prospective randomized control trial comparing medical and surgical treatment for early pregnancy failure (Demetroulis et al., 2001).
- A randomized control trial to evaluate a paging system for people with traumatic brain injury (Wilson et al., 2009).
- Prehabilitation versus Rehabilitation: A Randomized Control Trial in Patients Undergoing Colorectal Resection for Cancer (Gillis et al., 2014).
- A Randomized Control Trial of Right-Heart Catheterization in Critically Ill Patients (Guyatt, 1991).
- Berry, R. B., Kryger, M. H., & Massie, C. A. (2011). A novel nasal excitatory positive airway pressure (EPAP) device for the treatment of obstructive sleep apnea: A randomized controlled trial. Sleep , 34, 479–485.
- Gloy, V. L., Briel, M., Bhatt, D. L., Kashyap, S. R., Schauer, P. R., Mingrone, G., . . . Nordmann, A. J. (2013, October 22). Bariatric surgery versus non-surgical treatment for obesity: A systematic review and meta-analysis of randomized controlled trials. BMJ , 347.
- Streeton, C., & Whelan, G. (2001). Naltrexone, a relapse prevention maintenance treatment of alcohol dependence: A meta-analysis of randomized controlled trials. Alcohol and Alcoholism, 36 (6), 544–552.
How Should an RCT be Reported?
Reporting of a Randomized Controlled Trial (RCT) should be done in a clear, transparent, and comprehensive manner to allow readers to understand the design, conduct, analysis, and interpretation of the trial.
The Consolidated Standards of Reporting Trials ( CONSORT ) statement is a widely accepted guideline for reporting RCTs.
Further Information
- Cocks, K., & Torgerson, D. J. (2013). Sample size calculations for pilot randomized trials: a confidence interval approach. Journal of clinical epidemiology, 66(2), 197-201.
- Kendall, J. (2003). Designing a research project: randomised controlled trials and their principles. Emergency medicine journal: EMJ, 20(2), 164.
Akobeng, A.K., Understanding randomized controlled trials. Archives of Disease in Childhood , 2005; 90: 840-844.
Bell, C. C., Gibbons, R., & McKay, M. M. (2008). Building protective factors to offset sexually risky behaviors among black youths: a randomized control trial. Journal of the National Medical Association, 100 (8), 936-944.
Bhide, A., Shah, P. S., & Acharya, G. (2018). A simplified guide to randomized controlled trials. Acta obstetricia et gynecologica Scandinavica, 97 (4), 380-387.
Botvin, G. J., Griffin, K. W., Diaz, T., Scheier, L. M., Williams, C., & Epstein, J. A. (2000). Preventing illicit drug use in adolescents: Long-term follow-up data from a randomized control trial of a school population. Addictive Behaviors, 25 (5), 769-774.
Demetroulis, C., Saridogan, E., Kunde, D., & Naftalin, A. A. (2001). A prospective randomized control trial comparing medical and surgical treatment for early pregnancy failure. Human Reproduction, 16 (2), 365-369.
Gillis, C., Li, C., Lee, L., Awasthi, R., Augustin, B., Gamsa, A., … & Carli, F. (2014). Prehabilitation versus rehabilitation: a randomized control trial in patients undergoing colorectal resection for cancer. Anesthesiology, 121 (5), 937-947.
Globas, C., Becker, C., Cerny, J., Lam, J. M., Lindemann, U., Forrester, L. W., … & Luft, A. R. (2012). Chronic stroke survivors benefit from high-intensity aerobic treadmill exercise: a randomized control trial. Neurorehabilitation and Neural Repair, 26 (1), 85-95.
Guyatt, G. (1991). A randomized control trial of right-heart catheterization in critically ill patients. Journal of Intensive Care Medicine, 6 (2), 91-95.
MediLexicon International. (n.d.). Randomized controlled trials: Overview, benefits, and limitations. Medical News Today. Retrieved from https://www.medicalnewstoday.com/articles/280574#what-is-a-randomized-controlled-trial
Wilson, B. A., Emslie, H., Quirk, K., Evans, J., & Watson, P. (2005). A randomized control trial to evaluate a paging system for people with traumatic brain injury. Brain Injury, 19 (11), 891-894.

- Skip to secondary menu
- Skip to main content
- Skip to primary sidebar
Statistics By Jim
Making statistics intuitive
Randomized Controlled Trial (RCT) Overview
By Jim Frost Leave a Comment
What is a Randomized Controlled Trial (RCT)?
A randomized controlled trial (RCT) is a prospective experimental design that randomly assigns participants to an experimental or control group. RCTs are the gold standard for establishing causal relationships and ruling out confounding variables and selection bias. Researchers must be able to control who receives the treatments and who are the controls to use this design.

Random assignment is crucial for ruling out other potentially explanatory factors that could have caused those outcome differences. This process in RCTs is so effective that it even works with potential confounders that the researchers don’t know about! Think age, lifestyle, or genetics. Learn more about Random Assignment in Experiments .
Scientists use randomized controlled trials most frequently in fields like medicine, psychology, and social sciences to rigorously test interventions and treatments.
In this post, learn how RCTs work, the various types, and their strengths and weaknesses.
Randomized Controlled Trial Example
Imagine testing a new drug against a placebo using a randomized controlled trial. We take a representative sample of 100 patients. 50 get the drug; 50 get the placebo. Who gets what? It’s random! Perhaps we flip a coin. For more complex designs, we’d probably use computers for random assignment.
After a month, we measure health outcomes. Did the drug help more than the placebo? That’s what we find out!
To read about several examples of top-notch RCTs in more detail, read my following posts:
- How Effective Are Flu Shots?
- COVID Vaccination Randomized Controlled Trial
Common Elements for Effective RCT Designs
While randomization springs to mind when discussing RCTs, other equally vital components shape these robust experimental designs. Most well-designed randomized controlled trials contain the following elements.
- Control Group : Almost every RCT features a control group. This group might receive a placebo, no intervention, or standard care. You can estimate the treatment’s effect size by comparing the outcome in a treatment group to the control group. Learn more about Control Groups in an Experiment and controlling for the Placebo Effect .
- Blinding : Blinding hides group assignments from researchers and participants to prevent group assignment knowledge from influencing results. More on this shortly!
- Pre-defined Inclusion and Exclusion Criteria : These criteria set the boundaries for who can participate based on specifics like age or health conditions.
- Baseline Assessment : Before diving in, an initial assessment records participants’ starting conditions.
- Outcome Measures : Clear, pre-defined outcomes, like symptom reduction or survival rates, drive the study’s goals.
- Controlled, Standardized Environments : Ensuring variables are measured and treatments administered consistently minimizes external factors that could affect results.
- Monitoring and Data Collection : Regular checks guarantee participant safety and uniform data gathering.
- Ethical Oversight : Ensures participants’ rights and well-being are prioritized.
- Informed Consent : Participants must know the drill and agree to participate before joining .
- Statistical Plan : Detailing how statisticians will analyze the data before the RCT begins helps keep the evaluation objective and prevents p-hacking. Learn more about P-Hacking Best Practices .
- Protocol Adherence : Consistency is critical. Following the plan ensures reliable results.
- Analysis and Reporting : Once done, researchers share the results—good, bad, or neutral. Transparency builds trust.
These components ensure randomized controlled trials are both rigorous and ethically sound, leading to trustworthy results.
Common Variations of Randomized Controlled Trial Designs
Randomized controlled trial designs aren’t one-size-fits-all. Depending on the research question and context, researchers can apply various configurations.
Let’s explore the most common RCT designs:
- Parallel Group : Participants are randomly put into an intervention or control group.
- Crossover : Participants randomly receive both intervention and control at different times.
- Factorial : Tests multiple interventions at once. Useful for combination therapies.
- Cluster : Groups, not individuals, are randomized. For instance, researchers can randomly assign schools or towns to the experimental groups.
If you can’t randomly assign subjects and you want to draw causal conclusions about an intervention, consider using a quasi-experimental design .
Learn more about Experimental Design: Definition and Types .
Blinding in RCTs
Blinding is a standard protection in randomized controlled trials. The term refers to procedures that hide group assignments from those involved. While randomization ensures initial group balance, it doesn’t prevent uneven treatment or assessment as the RCT progresses, which could skew results.
So, what is the best way to sidestep potential biases?
Keep as many people in the dark about group assignments as possible. In a blinded randomized controlled trial, participants, and sometimes researchers, don’t know who gets the intervention.
There are three types of blinding:
- Single : Participants don’t know if they’re in the intervention or control group.
- Double : Both participants and researchers are in the dark.
- Triple : Participants, researchers, and statisticians all don’t know.
It guards against sneaky biases that might creep into our RCT results. Let’s look at a few:
- Confirmation Bias : Without blinding in a randomized controlled trial, researchers might unconsciously favor results that align with their expectations. For example, they might interpret ambiguous data as positive effects of a new drug if they’re hopeful about its efficacy.
- Placebo Effect : Participants who know they’re getting the ‘real deal’ might report improved outcomes simply because they believe in the treatment’s power. Conversely, those aware they’re in the control group might not notice genuine improvements.
- Observer Bias : If a researcher knows which participant is in which group, they might inadvertently influence outcomes. Imagine a physiotherapist unknowingly encouraging a participant more because they know they’re receiving the new treatment.
Blinding helps keep these biases at bay, making our results more reliable. It boosts confidence in a randomized controlled trial. Let’s close by summarizing the benefits and disadvantages of an RCT.
The Benefits of Randomized Controlled Studies
Randomized controlled trials offer a unique blend of strengths:
- RCTs are best for identifying causal relationships.
- Random assignment reduces both known and unknown biases.
- Many RCT designs exist, tailored for different research questions.
- Well-defined steps and controlled conditions ensure replicability across studies.
- Internal validity tends to be high in a randomized controlled trial. You can be confident that other variables don’t affect or account for the observed relationship.
Learn more about Correlation vs. Causation: Understanding the Differences .
The Drawbacks of RCTs
While powerful, RCTs also come with limitations:
- Randomized controlled trials can be expensive in time, money, and resources.
- Ethical concerns can arise when withholding treatments from a control group.
- Random assignment might not be possible in some circumstances.
- External validity can be low in an RCT. Conditions can be so controlled that the results might not always generalize beyond the study.
For a good comparison, learn about the differences and tradeoffs between using Observational Studies and Randomized Experiments .
Learn more about Internal and External Validity in Experiments and see how they’re a tradeoff.
Share this:

Reader Interactions
Comments and questions cancel reply.
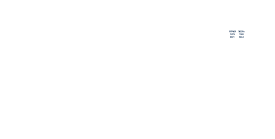
Why randomized controlled trials matter and the procedures that strengthen them
Randomized controlled trials are a key tool to study cause and effect. why do they matter and how do they work.
At Our World in Data, we bring attention to the world's largest problems. We explore what these problems are, why they matter and how large they are. Whenever possible, we try to explain why these problems exist, and how we might solve them.
To make progress, we need to be able to identify real solutions and evaluate them carefully. But doing this well is not simple. It's difficult for scientists to collect good evidence on the costs and benefits of a new idea, policy, or treatment. And it's challenging for decision-makers to scrutinize the evidence that exists, to make better decisions.
What we need are reliable ways to distinguish between ideas that work and those that don't.
In this post, I will explain a crucial tool that helps us do this – randomized controlled trials (RCTs). We will see that RCTs matter for three reasons: when we don't know about the effects of interventions, when we don't know how to study them, and when scientific research is affected by biases.
What are randomized controlled trials?
To begin with, what are RCTs? These are experiments where people are given, at random, either an intervention (such as a drug) or a control and then followed up to see how they fare on various outcomes.
RCTs are conducted by researchers around the world. In the map, you can see how many RCTs have ever been published in high-ranked medical journals, by the country where the first author was based. Over 18,000 of these were from the United States, but most countries have had fewer than a hundred. 1 RCTs have also become more common over time. 2
It's easy to take RCTs for granted, but these trials have transformed our understanding of cause and effect. They are a powerful tool to illuminate what is unknown or uncertain; to discern whether something works and how well it works.
But it's also important to recognize that these trials are not always perfect, and understand why.
The strengths of RCTs are subtle: they are powerful because of the set of procedures that they are expected to follow. This includes the use of controls, placebos, experimentation, randomization, concealment, blinding, intention-to-treat analysis, and pre-registration.
In this post, we will explore why these procedures matter – how each one adds a layer of protection against complications that scientists face when they do research.
The fundamental problem of causal inference
We make decisions based on our understanding of how things work – we try to predict the consequences of our actions.
But understanding cause and effect is not just crucial for personal decisions: our knowledge of what works can have large consequences for people around us.
An example is antiretroviral therapy (ART), which is used to treat HIV/AIDS . The benefits of these drugs were surprising. One of the first ART drugs discovered was azidothymidine, which had previously been abandoned as an ineffective treatment for cancer. 3 The discovery that azidothymidine and other antiretroviral therapies worked, and their use worldwide, has prevented millions of deaths from HIV/AIDS, as the chart shows.
Discovering what works can save lives. But discovering what doesn’t work can do the same. It means we can redirect our time and resources away from things that don't work, towards things that do.
Even when we already know that something works, understanding how well it works can help us make better decisions.
An example for this is the BCG vaccine , which reduces the risk of tuberculosis. The efficacy of this vaccine is different for different people around the world, and the reasons for this are unclear. 4 Still, knowing this is informative, because it tells us that more effort is needed to protect people against the disease in places where the benefit of the vaccine is low.
If there was a reliable way to know about the effects of the measures we took, we could prioritize solutions that are most effective.
So, how would we understand cause and effect without trials?
One way is through observation: we can observe what different people did and track their outcomes afterwards. But it's possible that the events that followed their actions were simply a coincidence, or that they would have happened anyway.
The biggest challenge in trying to understand causes and effects is that we are only able to see one version of history.
When someone makes a particular decision, we are able to see what follows, but we cannot see what would have happened if they had made a different decision. This is known as “the fundamental problem of causal inference." 5
What this means is that it is impossible to predict the effects that an action will have for an individual person, but we can try to predict the effects it would have on average.
Why randomized controlled trials matter
Sometimes we do not need an RCT to identify causes and effects. The effect of smoking on lung cancer is one example, where scientists could be confident as early as the 1960s that the large increase in lung cancer rates could not have been caused by other factors.
This was because there was already knowledge from many different lines of evidence. 6 Experiments, biopsies and population studies all showed that cigarette smoke was associated with specific changes in lung tissue and with the incidence of lung cancer. The association was so large and consistent that it could not be explained by other factors. 7
Even now, smoking is estimated to cause a large proportion of cancer deaths in many countries, as you can see in the chart.
But in other situations, RCTs have made a huge difference to our understanding of the world. Prioritizing the drugs that were shown to be effective in trials saved lives, as we saw with the example of antiretroviral therapy to treat HIV/AIDS.
People sometimes refer to RCTs as the "gold standard of evidence", but it's more useful to recognize that their strengths emerge from a set of procedures that they aim to follow.
Why do RCTs matter?
In my opinion, even though we do have lots of knowledge about various topics, these trials matter for three reasons. They matter because we may not know enough, because we can be wrong, and because we might see what we want to see.
First, they matter when we don't know .
Randomized controlled trials can illuminate our understanding of effects, especially when there is uncertainty around them. They can help resolve disagreements between experts.
In some cases, scientists can use other methods to investigate these topics. But when there is insufficient knowledge, other methods may not be enough.
This is because, in a typical study, scientists need to be able to account for the possibility that other factors are causing the outcome.
They need to have knowledge about why an effect usually occurs and whether it will happen anyway. They need to know about which other risk factors can cause the outcome and what makes it likely that someone will receive the treatment. They also need to know how to measure these factors properly, and how to account for them.
The second reason they matter is when we are wrong .
Even when scientists think they know which risk factors affect the outcome, they might be incorrect. They might not account for the right risk factors or they might account for the wrong ones.
In an RCT where scientists use randomization, blinding and concealment, they can minimize both of these problems. Despite people's risks before the trial, the reason that someone will receive the treatment is the random number they are given. The reason that participants in each group differ at the end of the study is the group they were randomized to.
Even if we don't know about other risk factors, or even if we are wrong about them, these procedures mean that we can still find out whether a treatment has an effect and how large the effect is.
The third reason is when we see what we want to see .
When participants expect to feel different after a treatment, they might report that they feel different, even if they didn't receive the treatment at all.
When scientists want to see people improve after a treatment, they might decide to allocate the treatment to healthier participants. They might measure their data differently or re-analyze their data until they can find an improvement. They might even decide against publishing their findings when they don't find a benefit.
If scientists use concealment, blinding, and pre-registration, they can reduce these biases. They can protect research against their own prejudices and the expectations of participants. They can also defend scientific knowledge from poor incentives, such as the desires of scientists to hype up their findings and the incentives for pharmaceutical companies to claim that their drugs work.
The layers of protection against bias
Previously, I described how the strengths of RCTs emerge from the set of procedures that they aim to follow.
In medicine, this commonly includes the use of controls, placebos, experimentation, randomization, concealment, blinding, intention-to-treat analysis, and pre-registration. 8 Each of these helps to protect the evidence against distortions, which can come from many sources. But they are not always enforced. Below, we will explore them in more detail.
The control group gives us a comparison to see what would have happened otherwise
The most crucial element to understand causes and effects is a control group.
Let's use antidepressants as an example to see why we need them.
If no one in the world received antidepressants, we wouldn’t know what their effects were. But the opposite is also true. If everyone received antidepressants, we wouldn't know how things would be without them.
In order to understand the effect of an antidepressant, we need to see which other outcomes are possible. The most important thing we need to understand effects is a comparison – we need some people who receive the treatment and some who don’t. The people who don’t could be our control group.
An ideal control group should allow us to control the biases and measurement errors in a study completely.
For example, different doctors may diagnose depression differently. If someone had symptoms of depression, then the chances they get diagnosed should be equal in the antidepressant group and the control group. If they weren't equal, we could mistake differences between the groups for an effect of antidepressants, even if they didn't have any effect.
In fact, an ideal control group should allow us to control the total effects of all of the other factors that could affect people's mood, not just control for the biases and errors in studies.
As an example, we know that the symptoms of depression tend to change over time, as I explained in this earlier post .
You can see this in the chart. This shows that the symptoms of depression tend to decline over time, among people who are diagnosed with depression but not treated for it. This is measured by seeing how many patients would still meet the threshold for a diagnosis of depression later on.
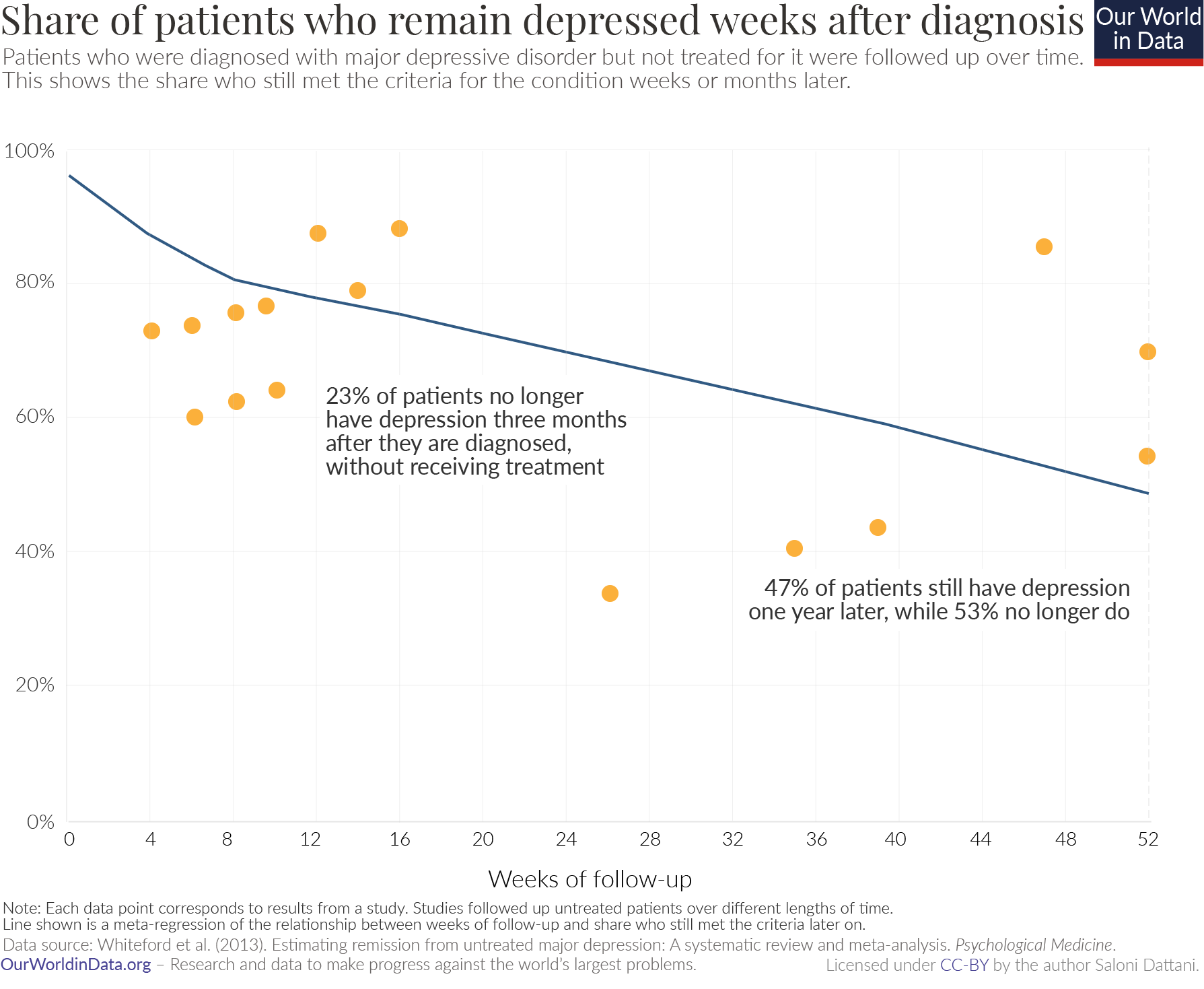
Almost a quarter of patients with depression (23%) would no longer meet the threshold for depression after three months, despite receiving no treatment. Just over half (53%) would no longer meet the threshold after one year. 9 This change is known as "regression to the mean."
If we didn’t have a control group in our study, we might misattribute such an improvement to the antidepressant. We need a control group to know how much their symptoms would improve anyway.
Placebos allow us to account for placebo effects
Some types of controls are special because they resemble the treatment without actually being it – these controls are called placebos. An ideal placebo has all the qualities above and also allows us to account for "placebo effects." In this case, the placebo effect refers to when people's moods improve from the mere procedure of receiving the antidepressant.
For example, taking a pill might improve people's mood because they believe that taking a pill will give them some benefit, even if the pill does not actually contain any active ingredient.
How large is the placebo effect?
Some clinical trials have tried to estimate this by comparing patients who received a placebo to patients who received no treatment at all. Overall, these studies have found that placebo effects are small in clinical trials for many conditions, but are larger for physical treatments, such as acupuncture to treat pain. 10
Placebo effects tend to be larger when scientists are recording symptoms reported by patients, rather than when they are measuring something that can be verified by others, such as results from a blood test or death.
For depression, the placebo effect is non-significant. Studies do not detect a difference in the moods of patients who receive a placebo and those who do not receive any treatment at all. 11 Instead, the placebo group serves as a typical control group, to show what would happen to these patients even if they did not receive treatment.
Randomization ensures that there are no differences between control and treatment group apart from whether they received the treatment
Before participants are enrolled in a trial, they might already have different levels of risk of developing the outcomes.
Let's look at an example to illustrate this point.
Statins are a type of drug commonly used to prevent stroke. But strokes are more common among people who use statins. Does that mean that statins caused an increase in the rates of stroke?
No – people who are prescribed statins are more likely to have cardiovascular problems to begin with, which increases the chances of having a stroke later on. When this is accounted for, researchers find that people who take statins are actually less likely to develop a stroke. 12
If researchers simply compared the rates of stroke in those who used statins with those who did not, they would miss the fact that there were other differences between the two groups, which could have caused differences in their rates of stroke.
Important differences such as these – which affect people's likelihood of receiving the treatment (using statins) and also affect the outcome (the risk of a stroke) – are called ‘confounders’.
But it can be difficult to know what all of these confounders might be. It can also be difficult to measure and account for them properly. In fact, scientists can actually worsen a study by accounting for the wrong factors. 13
What happens when participants are randomized in a trial?
Randomization is the procedure of allocating them into one of two groups at random.
With randomization, the problems above are minimized: everyone has the possibility of receiving the treatment. Whether people receive the treatment is not determined by the risks they have, but whether they are randomly selected to receive the treatment.
So, the overall risks of developing the outcome in one group become comparable to the risks in the other group.
Randomization means that it is not a problem when there are confounders that are not known or not measured. Researchers don't have to know about why or how the outcome usually occurs. 14
Concealment and blinding limit the biases of researchers and expectations of participants
In a clinical trial, participants or scientists might realize which groups they are assigned to. For example, the drug might smell, taste or look different from the placebo. Or it might have obvious benefits or different side effects compared to the placebo. 15
Concealment is a first step in preventing this: this is the procedure of preventing scientists from knowing which treatment people will be assigned to.
Blinding is a second step: this is the procedure of preventing participants and scientists from finding out which treatment group people have been assigned to. 16
When blinding is incomplete, it can partly reverse the benefits of randomization. For example, in clinical trials for oral health, the benefits of treatments appear larger when patients and the scientists who assess their health are not blinded sufficiently. 17
If randomization was maintained, the only reason that groups would differ on their outcomes was the treatment they received. However, if the treatments were not hidden from scientists and participants, other factors could cause differences between them.
Sometimes, blinding is not possible – there might not be something that is safe and closely resembles the treatment, which could be used as a placebo.
Fortunately, this does not necessarily mean that these trials cannot be useful. Researchers can measure verifiable outcomes (such as changes in blood levels or even deaths) to avoid some placebo effects.
But even when blinding occurs, participants and researchers might still make different decisions, because of the effects of the treatment or placebo.
For example, some participants might decide to withdraw from the trial or not follow the protocols of the trial closely. Similarly, scientists might guess which groups people are in and therefore treat them differently or measure their outcomes in a biased way.
It's difficult to predict how this might affect the results of a trial. It could cause us to overestimate or underestimate the benefit of a treatment. For example, in the clinical trials for Covid-19 vaccines , some participants may have guessed that they received the vaccine because they experienced side effects.
So, they may have believed that they were more protected and took fewer precautions. This means they may have increased their risk of catching Covid-19. This would make it appear as if the vaccines gave less protection than they actually did: it would result in an underestimate of their efficacy.
Preregistration allows us to hold researchers accountable to their original study plans
Some of the procedures we've explored so far are used to safeguard research against errors and biases that scientists can have. Pre-registration is another procedure that contributes to the same goal.
After the data in a study is analyzed, scientists have some choice in which results they present to their colleagues and the wider community. This opens up research to the possibility of cherry-picking.
This problem unfortunately often arises in studies that are sponsored by industry. If a pharmaceutical company is testing their new drugs in trials, disappointing results can lead to financial losses. So, they may decide not to publish them.
But this problem is not limited to trials conducted by pharmaceutical companies.
For many reasons, scientists may decide not to publish some of their studies. Or they might re-analyze their data in a different way to find more positive results. Even if scientists want to publish their findings, journals may decide not to publish them because they may be seen as disappointing, controversial or uninteresting.
To counter these incentives, scientists can follow the practice of "pre-registration." This is when they publicly declare which analyses they plan to do in advance of collecting the data.
In 2000, the United States Food and Drug Administration (FDA) established an online registry for the details of clinical trials. The FDA required that scientists who were studying therapies for serious diseases needed to provide some details of their study designs and the outcomes that they planned to investigate. 18
This requirement reduced the bias towards positive findings in published research. We see evidence of this in the chart, showing clinical trials funded by the National Heart, Lung and Blood Institute (NHLBI), which adopted this requirement.
Before 2000 – when pre-registration of studies was not required – most candidate drugs to treat or prevent cardiovascular disease showed large benefits. But most trials published after 2000 showed no benefit. 19
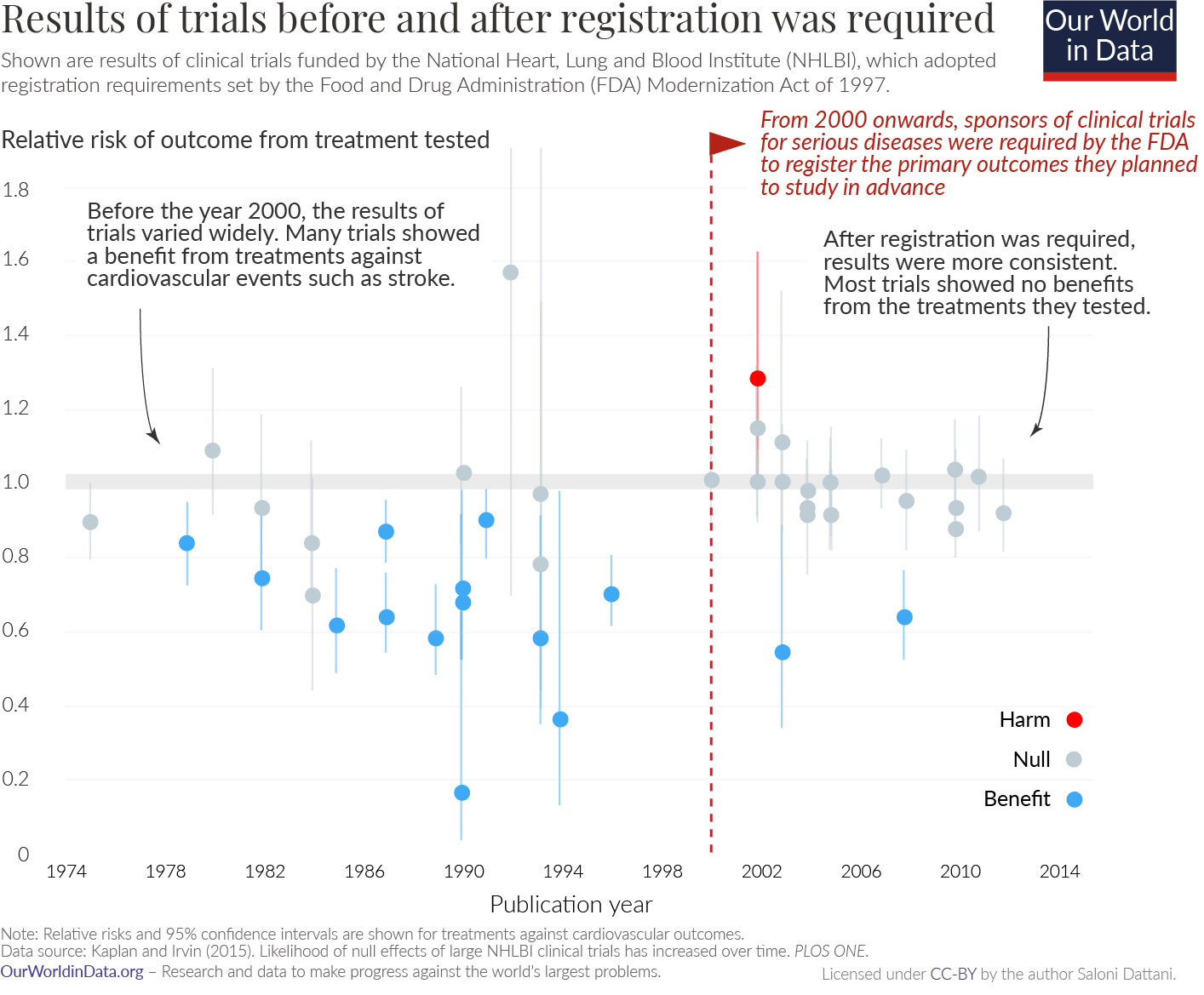
Over the last two decades, this practice was strengthened and expanded. In 2007, the FDA required that most approved trials must be registered when people are enrolled into the study. They introduced notices and civil penalties for not doing so. Now, similar requirements are in place in Europe, Canada and some other parts of the world. 20
Importantly, sponsors of clinical trials are required to share the results online after the trial is completed.
But unfortunately many still violate these requirements. For example, less than half (40%) of trials that were conducted in the US since 2017 actually reported their results on time. 21
Here, you can see patterns in reporting results in Europe. According to EU regulations, all clinical trials in the European Economic Area are required to report their results to the European Clinical Trials Register (EU-CTR) within a year of completing the trial. But reporting rates vary a lot by country. By January 2021, most clinical trials by non-commercial sponsors in the UK (94%) reported their results in time, while nearly none (4%) in France had. 22
Although a large share of clinical trials fail to report their results when they are due, this has improved recently: only half (50%) of clinical trials by non-commercial sponsors reported their results in time in 2018, while more than two-thirds (69%) did in 2021. 23
Pulling the layers together
Together, these procedures give us a far more reliable way to distinguish between what works and what doesn't – even when we don't know enough, when we're wrong and when we see what we want to see.
Unfortunately, we have seen that many clinical trials do not follow them. They tend not to report the details of their methods or test whether their participants were blinded to what they received. These standards cannot just be expected from scientists – they require cooperation from funders and journals, and they need to be actively enforced with penalties and incentives.
We've also seen that trials can still suffer from remaining problems: that participants may drop out of the study and not adhere to the treatment. And clinical trials tend to not share their data or the code that they used to analyze it.
So, despite all the benefits they provide, we shouldn't see these layers as a fixed checklist. Just as some of them were introduced recently, they may still evolve in the future – open data sharing, registered reports and procedures to reduce dropouts may be next on the list. 24
The procedures used in these trials are not a silver bullet. But when they are upheld, they can make these trials a more powerful source of evidence for understanding cause and effect.
To make progress, we need to be able to understand the problems that affect us, their causes and solutions. Randomized controlled trials can give scientists a reliable way to collect evidence on these important questions, and give us the ability to make better decisions. At Our World in Data, this is why they matter most of all.
Acknowledgements
I would like to thank Darren Dahly, Nathaniel Bechhofer, Hannah Ritchie and Max Roser for reading drafts of this post and their very helpful guidance and suggestions to improve it.
Additional information
Trials are useful to test new ideas or treatments, when people do not have enough knowledge to recommend them on a larger scale.
But people also have ethical concerns surrounding trials of treatments that are already used by doctors. For one, they might believe that it isn't justified to give patients a placebo when a drug is available, even if it is unclear how well the drug works.
How can we balance these concerns with the benefits of trials? When are trials important?
A leading view is that they are justified when there is disagreement or uncertainty about the benefits of an intervention. 25
Outside of a trial, some doctors might prescribe a particular treatment, while other doctors would not, because they believe that same treatment is ineffective. In this case, the options in a trial – an intervention or control – would not be so different from what people could already encounter.
When experts disagree on a topic, an RCT is useful because it can resolve these conflicts and inform us about which option is better.
So, to make the most of trials, they should be planned in line with the questions that people have. For example, if we wanted to understand whether vaccines gave less protection to people who were immunocompromised, then we should plan a trial with enough participants with these conditions.
Catalá-López, F., Aleixandre-Benavent, R., Caulley, L., Hutton, B., Tabarés-Seisdedos, R., Moher, D., & Alonso-Arroyo, A. (2020). Global mapping of randomised trials related articles published in high-impact-factor medical journals: a cross-sectional analysis. Trials , 21 (1), 1-24.
Vinkers, C. H., Lamberink, H. J., Tijdink, J. K., Heus, P., Bouter, L., Glasziou, P., Moher, D., Damen, J. A., Hooft, L., & Otte, W. M. (2021). The methodological quality of 176,620 randomized controlled trials published between 1966 and 2018 reveals a positive trend but also an urgent need for improvement. PLOS Biology , 19 (4), e3001162. https://doi.org/10.1371/journal.pbio.3001162
National Research Council (U.S.) (1993). The social impact of AIDS in the United States. 4. Clinical Research and Drug Regulation. National Academy Press.
Dockrell, H. M., & Smith, S. G. (2017). What Have We Learnt about BCG Vaccination in the Last 20 Years? Frontiers in Immunology , 8 , 1134. https://doi.org/10.3389/fimmu.2017.01134
Mangtani, P., Abubakar, I., Ariti, C., Beynon, R., Pimpin, L., Fine, P. E. M., Rodrigues, L. C., Smith, P. G., Lipman, M., Whiting, P. F., & Sterne, J. A. (2014). Protection by BCG Vaccine Against Tuberculosis: A Systematic Review of Randomized Controlled Trials. Clinical Infectious Diseases , 58 (4), 470–480. https://doi.org/10.1093/cid/cit790
Holland, P. W. (1986). Statistics and Causal Inference. Journal of the American Statistical Association , 81 (396), 945–960. https://doi.org/10.1080/01621459.1986.10478354
Imbens, G. W., & Rubin, D. B. (2010). Rubin causal model. In Microeconometrics (pp. 229–241). Springer.
Rubin, D. B. (1977). Assignment to Treatment Group on the Basis of a Covariate. Journal of Educational Statistics , 2 (1), 1–26. https://doi.org/10.3102/10769986002001001
Hill, G., Millar, W., & Connelly, J. (2003). “The Great Debate”: Smoking, Lung Cancer, and Cancer Epidemiology. Canadian Bulletin of Medical History , 20 (2), 367-386.
Cornfield, J., Haenszel, W., Hammond, E. C., Lilienfeld, A. M., Shimkin, M. B., & Wynder, E. L. (1959). Smoking and Lung Cancer: Recent Evidence and a Discussion of Some Questions. JNCI: Journal of the National Cancer Institute . https://doi.org/10.1093/jnci/22.1.173
Although these procedures are also used outside of medicine, it can be difficult to apply them elsewhere. For example, in a trial that tests the effectiveness of talking therapy, it would be known to the participants that they are receiving it; it may not be possible to find a placebo control version to disguise the procedure. Due to constraints in length and focus, I will not detail the advantages of intention-to-treat analysis or experimentation.
Whiteford, H. A., Harris, M. G., McKeon, G., Baxter, A., Pennell, C., Barendregt, J. J., & Wang, J. (2013). Estimating remission from untreated major depression: A systematic review and meta-analysis. Psychological Medicine , 43 (8), 1569–1585. https://doi.org/10.1017/S0033291712001717
Hróbjartsson, A., & Gøtzsche, P. C. (2010). Placebo interventions for all clinical conditions. Cochrane Database of Systematic Reviews . https://doi.org/10.1002/14651858.CD003974.pub3
Hróbjartsson, A., & Gøtzsche, P. C. (2001). Is the Placebo Powerless?: An Analysis of Clinical Trials Comparing Placebo with No Treatment. New England Journal of Medicine , 344 (21), 1594–1602. https://doi.org/10.1056/NEJM200105243442106
Orkaby, A. R., Gaziano, J. M., Djousse, L., & Driver, J. A. (2017). Statins for Primary Prevention of Cardiovascular Events and Mortality in Older Men. Journal of the American Geriatrics Society , 65 (11), 2362–2368. https://doi.org/10.1111/jgs.14993
Makihara, N., Kamouchi, M., Hata, J., Matsuo, R., Ago, T., Kuroda, J., Kuwashiro, T., Sugimori, H., & Kitazono, T. (2013). Statins and the risks of stroke recurrence and death after ischemic stroke: The Fukuoka Stroke Registry. Atherosclerosis , 231 (2), 211–215. https://doi.org/10.1016/j.atherosclerosis.2013.09.017
Ní Chróinín, D., Asplund, K., Åsberg, S., Callaly, E., Cuadrado-Godia, E., Díez-Tejedor, E., Di Napoli, M., Engelter, S. T., Furie, K. L., Giannopoulos, S., Gotto, A. M., Hannon, N., Jonsson, F., Kapral, M. K., Martí-Fàbregas, J., Martínez-Sánchez, P., Milionis, H. J., Montaner, J., Muscari, A., … Kelly, P. J. (2013). Statin Therapy and Outcome After Ischemic Stroke: Systematic Review and Meta-Analysis of Observational Studies and Randomized Trials. Stroke , 44 (2), 448–456. https://doi.org/10.1161/STROKEAHA.112.668277
Cinelli, C., Forney, A., & Pearl, J. (2020). A crash course in good and bad controls. Available at SSRN, 3689437: http://dx.doi.org/10.2139/ssrn.3689437
Aronow, P., Robins, J. M., Saarinen, T., Sävje, F., & Sekhon, J. (2021). Nonparametric identification is not enough, but randomized controlled trials are. ArXiv Preprint ArXiv:2108.11342 .
Higgins, J. P. T., Altman, D. G., Gotzsche, P. C., Juni, P., Moher, D., Oxman, A. D., Savovic, J., Schulz, K. F., Weeks, L., Sterne, J. A. C., Cochrane Bias Methods Group, & Cochrane Statistical Methods Group. (2011). The Cochrane Collaboration’s tool for assessing risk of bias in randomised trials. BMJ , 343 (oct18 2), d5928–d5928. https://doi.org/10.1136/bmj.d5928
Schulz, K. F., Chalmers, I., & Altman, D. G. (2002). The Landscape and Lexicon of Blinding in Randomized Trials. Annals of Internal Medicine , 136 (3), 254. https://doi.org/10.7326/0003-4819-136-3-200202050-00022
Saltaji, H. et al. Influence of blinding on treatment effect size estimate in randomized controlled trials of oral health interventions. BMC Med Res Methodol 18 , 42 (2018).
Dickersin, K., & Rennie, D. (2012). The evolution of trial registries and their use to assess the clinical trial enterprise. Jama , 307 (17), 1861–1864.
Kaplan, R. M., & Irvin, V. L. (2015). Likelihood of Null Effects of Large NHLBI Clinical Trials Has Increased over Time. PLOS ONE , 10 (8), e0132382. https://doi.org/10.1371/journal.pone.0132382
Dickersin, K., & Rennie, D. (2012). The evolution of trial registries and their use to assess the clinical trial enterprise. Jama , 307 (17), 1861–1864. https://doi.org/10.1001/jama.2012.4230
DeVito, N. J., & Goldacre, B. (2021). Evaluation of Compliance With Legal Requirements Under the FDA Amendments Act of 2007 for Timely Registration of Clinical Trials, Data Verification, Delayed Reporting, and Trial Document Submission. JAMA Internal Medicine, 181(8), 1128. https://doi.org/10.1001/jamainternmed.2021.2036
Note: Data is shown until January 2021 for trials. After the UK left the European Union in January 2021, clinical trials in the UK were then no longer required to report their results to the EU-CTR. Only data from trials by non-commercial sponsors is shown. This includes trials sponsored by institutions such as universities, hospitals, research foundations and so on.
Dal-Ré, R., Goldacre, B., Mahillo-Fernández, I., & DeVito, N. J. (2021). European non-commercial sponsors showed substantial variation in results reporting to the EU trial registry. Journal of Clinical Epidemiology , S0895435621003577. https://doi.org/10.1016/j.jclinepi.2021.11.005
Chambers, C., & Tzavella, L. (2020). The past, present, and future of Registered Reports.
Wendler, D. The Ethics of Clinical Research. in The Stanford Encyclopedia of Philosophy (ed. Zalta, E. N.) (Metaphysics Research Lab, Stanford University, 2021).
London, A. J. Equipoise in Research: Integrating Ethics and Science in Human Research. JAMA 317, 525 (2017).
Cite this work
Our articles and data visualizations rely on work from many different people and organizations. When citing this article, please also cite the underlying data sources. This article can be cited as:
BibTeX citation
Reuse this work freely
All visualizations, data, and code produced by Our World in Data are completely open access under the Creative Commons BY license . You have the permission to use, distribute, and reproduce these in any medium, provided the source and authors are credited.
The data produced by third parties and made available by Our World in Data is subject to the license terms from the original third-party authors. We will always indicate the original source of the data in our documentation, so you should always check the license of any such third-party data before use and redistribution.
All of our charts can be embedded in any site.
Our World in Data is free and accessible for everyone.
Help us do this work by making a donation.
- - Google Chrome
Intended for healthcare professionals
- My email alerts
- BMA member login
- Username * Password * Forgot your log in details? Need to activate BMA Member Log In Log in via OpenAthens Log in via your institution

Search form
- Advanced search
- Search responses
- Search blogs
- Understanding...
Understanding controlled trials: Why are randomised controlled trials important?
- Related content
- Peer review
- Bonnie Sibbald , reader in health services research a ,
- Martin Roland , director of research and development a
- a National Primary Care Research and Development Centre, University of Manchester, Manchester M13 9PL
- Correspondence to: Dr Sibbald
Randomised controlled trials are the most rigorous way of determining whether a cause-effect relation exists between treatment and outcome and for assessing the cost effectiveness of a treatment. They have several important features:
Random allocation to intervention groups
Patients and trialists should remain unaware of which treatment was given until the study is completed-although such double blind studies are not always feasible or appropriate
All intervention groups are treated identically except for the experimental treatment
Patients are normally analysed within the group to which they were allocated, irrespective of whether they experienced the intended intervention (intention to treat analysis)
The analysis is focused on estimating the size of the difference in predefined outcomes between intervention groups.
Other study designs, including non-randomised controlled trials, can detect associations between an intervention and an outcome. But they cannot rule out the possibility that the association was caused by a third factor linked to both intervention and outcome. Random allocation ensures no systematic differences between intervention groups in factors, known and unknown, that may affect outcome. Double blinding ensures that the preconceived views of subjects and clinicians cannot systematically bias the assessment of outcomes. Intention to treat analysis maintains the advantages of random allocation, which may be lost if subjects are excluded from analysis through, for example, withdrawal or failure to comply. Meta-analysis of controlled trials shows that failure to conceal random allocation and the absence of double blinding yield exaggerated estimates of treatment effects. 1
Although randomised controlled trials are powerful tools, their use is limited by ethical and practical concerns. Exposing patients to an intervention believed to be inferior to current treatment is often thought unethical. For example, a non-random study suggested that multivitamin supplementation during pregnancy could prevent neural tube defects in children. 2 Although the study was seriously flawed, ethics committees were unwilling to deprive patients of this potentially useful treatment, making it difficult to carry out the trial which later showed that folic acid was the effective part of the multivitamin cocktail. 3 On the other hand, failure to perform trials may result in harmful treatments being used. For example, neonates were widely treated with high concentrations of oxygen until randomised trials identified oxygen as a risk factor for retinopathy of prematurity. 4
In other circumstances a randomised controlled trial may be ethical but infeasible-for example, because of difficulties with randomisation or recruitment. Indeed, once an intervention becomes widespread, it can prove impossible to recruit clinicians who are willing to “experiment” with alternatives. A recent attempt to conduct a trial of counselling in general practice failed when practitioners declined to recruit patients to be allocated at random. 5 Strong patient preferences may also limit recruitment and bias outcomes if not accommodated within the study design. 6
A third limiting factor is that randomised controlled trials are generally more costly and time consuming than other studies. Careful consideration therefore needs to be given to their use and timing.
Is the intervention well enough developed to permit evaluation? This can be especially difficult to decide when new interventions are heavily dependent on clinicians’ skills (surgical procedures 7 or “talk” therapies).
Is there preliminary evidence that the intervention is likely to be beneficial (from observational studies), including some appreciation of the size of the likely treatment effect? Such information is needed to estimate sample sizes and justify the expense of a trial.
Given these constraints, it remains an ideal that all new healthcare interventions should be evaluated through randomised controlled trials. Given that poor design may lead to biased outcomes, 1 trialists should strive for methodological rigour and report their work in enough detail for others to assess its quality. 8
- Schulz KF ,
- Chalmers I ,
- Haynes RJ ,
- Smithells RW ,
- Shepherd S ,
- Schorah CJ ,
- Seller MJ ,
- 3. ↵ MRC Vitamin Study Research Group . Prevention of neural tube defects. Results of the Medical Research Council Vitamin Study . Lancet 1991 ; 338 : 131 - 7 . OpenUrl CrossRef PubMed Web of Science
- Lanman JT ,
- Fairhurst K ,
- Brewin CR ,
Introduction to Field Experiments and Randomized Controlled Trials

Have you ever been curious about the methods researchers employ to determine causal relationships among various factors, ultimately leading to significant breakthroughs and progress in numerous fields? In this article, we offer an overview of field experimentation and its importance in discerning cause and effect relationships. We outline how randomized experiments represent an unbiased method for determining what works. Furthermore, we discuss key aspects of experiments, such as intervention, excludability, and non-interference. To illustrate these concepts, we present a hypothetical example of a randomized controlled trial evaluating the efficacy of an experimental drug called Covi-Mapp.
Why experiments?
Every day, we find ourselves faced with questions of cause and effect. Understanding the driving forces behind outcomes is crucial, ranging from personal decisions like parenting strategies to organizational challenges such as effective advertising. This blog aims to provide a systematic introduction to experimentation, igniting enthusiasm for primary research and highlighting the myriad of experimental applications and opportunities available.
The challenge for those who seek to answer causal questions convincingly is to develop a research methodology that doesn't require identifying or measuring all potential confounders. Since no planned design can eliminate every possible systematic difference between treatment and control groups, random assignment emerges as a powerful tool for minimizing bias. In the contentious world of causal claims, randomized experiments represent an unbiased method for determining what works. Random assignment means participants are assigned to different groups or conditions in a study purely by chance. Basically, each participant has an equal chance to be assigned to a control group or a treatment group.
Field experiments, or randomized studies conducted in real-world settings, can take many forms. While experiments on college campuses are often considered lab studies, certain experiments on campus – such as those examining club participation – may be regarded as field experiments, depending on the experimental design. Ultimately, whether a study is considered a field experiment hinges on the definition of "the field."
Researchers may employ two main scenarios for randomization. The first involves gathering study participants and randomizing them at the time of the experiment. The second capitalizes on naturally occurring randomizations, such as the Vietnam draft lottery.
Intervention, Excludability, and Non-Interference
Three essential features of any experiment are intervention, excludability, and non-interference. In a general sense, the intervention refers to the treatment or action being tested in an experiment. The excludability principle is satisfied when the only difference between the experimental and control groups is the presence or absence of the intervention. The non-interference principle holds when the outcome of one participant in the study does not influence the outcomes of other participants. Together, these principles ensure that the experiment is designed to provide unbiased and reliable results, isolating the causal effect of the intervention under study.
Omitted Variables and Non-Compliance
To ensure unbiased results, researchers must randomize as much as possible to minimize omitted variable bias. Omitted variables are factors that influence the outcome but are not measured or are difficult to measure. These unmeasured attributes, sometimes called confounding variables or unobserved heterogeneity, must be accounted for to guarantee accurate findings.
Non-compliance can also complicate experiments. One-sided non-compliance occurs when individuals assigned to a treatment group don't receive the treatment (failure to treat), while two-sided non-compliance occurs when some subjects assigned to the treatment group go untreated or individuals assigned to the control group receive the treatment. Addressing these issues at the design level by implementing a blind or double-blind study can help mitigate potential biases.
Achieving Precision through Covariate Balance
To ensure the control and treatment groups are comparatively similar in all relevant aspects, particularly when the sample size (n) is small, it is essential to achieve covariate balance. Covariance measures the association between two variables, while a covariate is a factor that influences the outcome variable. By balancing covariates, we can more accurately isolate the effects of the treatment, leading to improved precision in our findings.
Fictional Example of Randomized Controlled Trial of Covi-Mapp for COVID-19 Management
Let's explore a fictional example to better understand experiments: a one-week randomized controlled trial of the experimental drug Covi-Mapp for managing Covid. In this case, the control group receives the standard care for Covid patients, while the treatment group receives the standard care plus Covi-Mapp. The outcome of interest is whether patients have cough symptoms on day 7, as subsidizing cough symptoms is an encouraging sign in Covid recovery. We'll measure the presence of cough on day 0 and day 7, as well as temperature on day 0 and day 7. Gender is also tracked. The control represents the standard care for COVID-19 patients, while the treatment includes standard care plus the experimental drug.
In this Covi-Mapp example, the intervention is the Covi-Mapp drug, the excludability principle is satisfied if the only difference in patient care between the groups is the drug administration, and the non-interference principle holds if one patient's outcome doesn't affect another's.
First, let's assume we have a dataset containing the relevant information for each patient, including cough status on day 0 and day 7, temperature on day 0 and day 7, treatment assignment, and gender. We'll read the data and explore the dataset:
library(data.table) d <- fread("../data/COVID_rct.csv") names(d) "temperature_day0" "cough_day0" "treat_zmapp" "temperature_day14" "cough_day14" "male" |
Simple treatment effect of the experimental drug
Without any covariates, let's first look at the estimated effect of the treatment on the presence of cough on day 7. The estimated proportion of patients with a cough on day 7 for the control group (not receiving the experimental drug) is 0.847458. In other words, about 84.7% of patients in the control group are expected to have a cough on day 7, all else being equal. The estimated effect of the experimental drug on the presence of cough on day 7 is -0.23. This means that, on average, receiving the experimental drug reduces the proportion of patients with a cough on day 7 by 23.8% compared to the control group.
covid_1 <- d[ , lm(cough_day7 ~ treat_drug)] coeftest(covid_1, vcovHC) Estimate Std. Error t value Pr(>|t|) (Intercept) 0.847458 0.047616 17.798 < 2e-16 *** treat_covid_mapp -0.237702 0.091459 -2.599 0.01079 * Signif. codes: 0 ‘***’ 0.001 ‘**’ 0.01 ‘*’ 0.05 ‘.’ 0.1 ‘ ’ 1 |
We know that a patient's initial condition would affect the final outcome. If the patient has a cough and a fever on day 0, they might not fare well with the treatment. To better understand the treatment's effect, let's add these covariates:
covid_2 <- d[ , lm(cough_day7 ~ treat_drug + cough_day0 + temperature_day0)] coeftest(covid_2, vcovHC) Estimate Std. Error t value Pr(>|t|) (Intercept) -19.469655 7.607812 -2.5592 0.012054 * treat_covid_mapp -0.165537 0.081976 -2.0193 0.046242 * cough_day0 0.064557 0.178032 0.3626 0.717689 temperature_day0 0.205548 0.078060 2.6332 0.009859 ** Signif. codes: 0 ‘***’ 0.001 ‘**’ 0.01 ‘*’ 0.05 ‘.’ 0.1 ‘ ’ 1 |
The output shows the results of a linear regression model, estimating the effect of the experimental drug (treat_covid_mapp) on the presence of cough on day 7, adjusting for cough on day 0 and temperature on day 0. The experimental drug significantly reduces the presence of cough on day 7 by approximately 16.6% compared to the control group (p-value = 0.046242). The presence of cough on day 0 does not significantly predict the presence of cough on day 7 (p-value = 0.717689). A one-unit increase in temperature on day 0 is associated with a 20.6% increase in the presence of cough on day 7, and this effect is statistically significant (p-value = 0.009859).
Should we add day 7 temperature as a covariate? By including it, we might find that the treatment is no longer statistically significant since the temperature on day 7 could be affected by the treatment itself. It is a post-treatment variable, and by including it, the experiment loses value as we used something that was affected by intervention as our covariate.
However, we'd like to investigate if the treatment affects men or women differently. Since we collected gender as part of the study, we could check for Heterogeneous Treatment Effect (HTE) for male vs. female. The experimental drug has a marginally significant effect on the outcome variable for females, reducing it by approximately 23.1% (p-value = 0.05391).
covid_4 <- d[ , lm(cough_day7 ~ treat_drug + treat_drug * male + cough_day0 + temperature_day0)] coeftest(covid_4, vcovHC) t test of coefficients: Estimate Std. Error t value Pr(>|t|) (Intercept) 48.712690 10.194000 4.7786 6.499e-06 *** treat_zmapp -0.230866 0.118272 -1.9520 0.05391 . male 3.085486 0.121773 25.3379 < 2.2e-16 *** dehydrated_day0 0.041131 0.194539 0.2114 0.83301 temperature_day0 0.504797 0.104511 4.8301 5.287e-06 *** treat_zmapp:male -2.076686 0.198386 -10.4679 < 2.2e-16 *** Signif. codes: 0 ‘***’ 0.001 ‘**’ 0.01 ‘*’ 0.05 ‘.’ 0.1 ‘ ’ 1 |
Which group, those coded as male == 0 or male == 1, have better health outcomes (cough) in control? What about in treatment? How does this help to contextualize any heterogeneous treatment effect that might have been estimated?
Stargazer is a popular R package that enables users to create well-formatted tables and reports for statistical analysis results.
covid_males <- d[male == 1, lm(temperature_day14 ~ treat_drug)] covid_females <- d[male == 0, lm(temperature_day14 ~ treat_drug)] stargazer(covid_males, covid_females, title = "", type = 'text', dep.var.caption = 'Outcome Variable:', dep.var.labels = c('Cough on Day 7'), se = list( sqrt(diag(vcov(covid_males))), sqrt(diag(vcovHC(covid_females)))) ) =============================================================== Outcome Variable: Temperature on Day 14 (1) (2) treat_covid_mapp -2.591*** -0.323* (0.220) (0.174) Constant 101.692*** 98.487*** (0.153) (0.102) Observations 37 63 R2 0.798 0.057 Adjusted R2 0.793 0.041 Residual Std. Error 0.669 (df = 35) 0.646 (df = 61) F Statistic 138.636*** (df = 1; 35) 3.660* (df = 1; 61) =============================================================== Note: *p<0.1; **p<0.05; ***p<0.01 |
Looking at this regression report, we see that males in control have a temperature of 102; females in control have a temperature of 98.6 (which is very nearly a normal temperature). So, in control, males are worse off. In treatment, males have a temperature of 102 - 2.59 = 99.41. While this is closer to a normal temperature, this is still elevated. Females in treatment have a temperature of 98.5 - .32 = 98.18, which is slightly lower than a normal temperature, and is better than an elevated temperature. It appears that the treatment is able to have a stronger effect among male participants than females because males are *more sick* at baseline.
In conclusion, experimentation offers a fascinating and valuable avenue for primary research, allowing us to address causal questions and enhance our understanding of the world around us. Covariate control helps to isolate the causal effect of the treatment on the outcome variable, ensuring that the observed effect is not driven by confounding factors. Proper control of covariates enhances the internal validity of the study and ensures that the estimated treatment effect is an accurate representation of the true causal relationship. By exploring and accounting for sub groups in data, researchers can identify whether the treatment has different effects on different groups, such as men and women or younger and older individuals. This information can be critical for making informed policy decisions and developing targeted interventions that maximize the benefits for specific groups. The ongoing investigation of experimental methodologies and their potential applications represents a compelling and significant area of inquiry.
Gerber, A. S., & Green, D. P. (2012). Field Experiments: Design, Analysis, and Interpretation . W. W. Norton.
“DALL·E 2.” OpenAI , https://openai.com/product/dall-e-2
“Data Science 241. Experiments and Causal Inference.” UC Berkeley School of Information , https://www.ischool.berkeley.edu/courses/datasci/241
Study Design 101: Randomized Controlled Trial
- Case Report
- Case Control Study
- Cohort Study
- Randomized Controlled Trial
- Practice Guideline
- Systematic Review
- Meta-Analysis
- Helpful Formulas
- Finding Specific Study Types
A study design that randomly assigns participants into an experimental group or a control group. As the study is conducted, the only expected difference between the control and experimental groups in a randomized controlled trial (RCT) is the outcome variable being studied.
- Good randomization will "wash out" any population bias
- Easier to blind/mask than observational studies
- Results can be analyzed with well known statistical tools
- Populations of participating individuals are clearly identified
Disadvantages
- Expensive in terms of time and money
- Volunteer biases: the population that participates may not be representative of the whole
- Loss to follow-up attributed to treatment
Design pitfalls to look out for
An RCT should be a study of one population only.
Was the randomization actually "random", or are there really two populations being studied?
The variables being studied should be the only variables between the experimental group and the control group.
Are there any confounding variables between the groups?
Fictitious Example
To determine how a new type of short wave UVA-blocking sunscreen affects the general health of skin in comparison to a regular long wave UVA-blocking sunscreen, 40 trial participants were randomly separated into equal groups of 20: an experimental group and a control group. All participants' skin health was then initially evaluated. The experimental group wore the short wave UVA-blocking sunscreen daily, and the control group wore the long wave UVA-blocking sunscreen daily.
After one year, the general health of the skin was measured in both groups and statistically analyzed. In the control group, wearing long wave UVA-blocking sunscreen daily led to improvements in general skin health for 60% of the participants. In the experimental group, wearing short wave UVA-blocking sunscreen daily led to improvements in general skin health for 75% of the participants.
Real-life Examples
van Der Horst, N., Smits, D., Petersen, J., Goedhart, E., & Backx, F. (2015). The preventive effect of the nordic hamstring exercise on hamstring injuries in amateur soccer players: a randomized controlled trial. The American Journal of Sports Medicine, 43 (6), 1316-1323. https://doi.org/10.1177/0363546515574057
This article reports on the research investigating whether the Nordic Hamstring Exercise is effective in preventing both the incidence and severity of hamstring injuries in male amateur soccer players. Over the course of a year, there was a statistically significant reduction in the incidence of hamstring injuries in players performing the NHE, but for those injured, there was no difference in severity of injury. There was also a high level of compliance in performing the NHE in that group of players.
Natour, J., Cazotti, L., Ribeiro, L., Baptista, A., & Jones, A. (2015). Pilates improves pain, function and quality of life in patients with chronic low back pain: a randomized controlled trial. Clinical Rehabilitation, 29 (1), 59-68. https://doi.org/10.1177/0269215514538981
This study assessed the effect of adding pilates to a treatment regimen of NSAID use for individuals with chronic low back pain. Individuals who included the pilates method in their therapy took fewer NSAIDs and experienced statistically significant improvements in pain, function, and quality of life.
Related Formulas
- Relative Risk
Related Terms
Blinding/Masking
When the groups that have been randomly selected from a population do not know whether they are in the control group or the experimental group.
Being able to show that an independent variable directly causes the dependent variable. This is generally very difficult to demonstrate in most study designs.
Confounding Variables
Variables that cause/prevent an outcome from occurring outside of or along with the variable being studied. These variables render it difficult or impossible to distinguish the relationship between the variable and outcome being studied).
Correlation
A relationship between two variables, but not necessarily a causation relationship.
Double Blinding/Masking
When the researchers conducting a blinded study do not know which participants are in the control group of the experimental group.
Null Hypothesis
That the relationship between the independent and dependent variables the researchers believe they will prove through conducting a study does not exist. To "reject the null hypothesis" is to say that there is a relationship between the variables.
Population/Cohort
A group that shares the same characteristics among its members (population).
Population Bias/Volunteer Bias
A sample may be skewed by those who are selected or self-selected into a study. If only certain portions of a population are considered in the selection process, the results of a study may have poor validity.
Randomization
Any of a number of mechanisms used to assign participants into different groups with the expectation that these groups will not differ in any significant way other than treatment and outcome.
Research (alternative) Hypothesis
The relationship between the independent and dependent variables that researchers believe they will prove through conducting a study.
Sensitivity
The relationship between what is considered a symptom of an outcome and the outcome itself; or the percent chance of not getting a false positive (see formulas).
Specificity
The relationship between not having a symptom of an outcome and not having the outcome itself; or the percent chance of not getting a false negative (see formulas).
Type 1 error
Rejecting a null hypothesis when it is in fact true. This is also known as an error of commission.
Type 2 error
The failure to reject a null hypothesis when it is in fact false. This is also known as an error of omission.
Now test yourself!
1. Having a volunteer bias in the population group is a good thing because it means the study participants are eager and make the study even stronger.
a) True b) False
2. Why is randomization important to assignment in an RCT?
a) It enables blinding/masking b) So causation may be extrapolated from results c) It balances out individual characteristics between groups. d) a and c e) b and c
Evidence Pyramid - Navigation
- Meta- Analysis
- Case Reports
- << Previous: Cohort Study
- Next: Practice Guideline >>

- Last Updated: Sep 25, 2023 10:59 AM
- URL: https://guides.himmelfarb.gwu.edu/studydesign101

- Himmelfarb Intranet
- Privacy Notice
- Terms of Use
- GW is committed to digital accessibility. If you experience a barrier that affects your ability to access content on this page, let us know via the Accessibility Feedback Form .
- Himmelfarb Health Sciences Library
- 2300 Eye St., NW, Washington, DC 20037
- Phone: (202) 994-2962
- [email protected]
- https://himmelfarb.gwu.edu

An official website of the United States government
The .gov means it’s official. Federal government websites often end in .gov or .mil. Before sharing sensitive information, make sure you’re on a federal government site.
The site is secure. The https:// ensures that you are connecting to the official website and that any information you provide is encrypted and transmitted securely.
- Publications
- Account settings
- My Bibliography
- Collections
- Citation manager
Save citation to file
Email citation, add to collections.
- Create a new collection
- Add to an existing collection
Add to My Bibliography
Your saved search, create a file for external citation management software, your rss feed.
- Search in PubMed
- Search in NLM Catalog
- Add to Search
A simplified guide to randomized controlled trials
Affiliations.
- 1 Fetal Medicine Unit, St. Georges University Hospital, London, UK.
- 2 Division of Neonatology, Department of Pediatrics, Mount Sinai Hospital, Toronto, ON, Canada.
- 3 Institute of Health Policy, Management and Evaluation, University of Toronto, Toronto, ON, Canada.
- 4 Department of Clinical Science, Intervention and Technology, Karolinska Institute and Center for Fetal Medicine, Karolinska University Hospital, Stockholm, Sweden.
- 5 Women's Health and Perinatology Research Group, Department of Clinical Medicine, UiT-The Arctic University of Norway, Tromsø, Norway.
- 6 Department of Obstetrics and Gynecology, University Hospital of North Norway, Tromsø, Norway.
- PMID: 29377058
- DOI: 10.1111/aogs.13309
A randomized controlled trial is a prospective, comparative, quantitative study/experiment performed under controlled conditions with random allocation of interventions to comparison groups. The randomized controlled trial is the most rigorous and robust research method of determining whether a cause-effect relation exists between an intervention and an outcome. High-quality evidence can be generated by performing an randomized controlled trial when evaluating the effectiveness and safety of an intervention. Furthermore, randomized controlled trials yield themselves well to systematic review and meta-analysis providing a solid base for synthesizing evidence generated by such studies. Evidence-based clinical practice improves patient outcomes and safety, and is generally cost-effective. Therefore, randomized controlled trials are becoming increasingly popular in all areas of clinical medicine including perinatology. However, designing and conducting an randomized controlled trial, analyzing data, interpreting findings and disseminating results can be challenging as there are several practicalities to be considered. In this review, we provide simple descriptive guidance on planning, conducting, analyzing and reporting randomized controlled trials.
Keywords: Clinical trial; good clinical practice; random allocation; randomized controlled trial; research methods; study design.
© 2018 Nordic Federation of Societies of Obstetrics and Gynecology.
PubMed Disclaimer
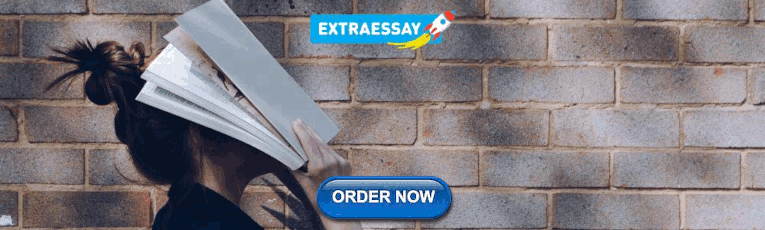
Similar articles
- Methodology citations and the quality of randomized controlled trials in obstetrics and gynecology. Grimes DA, Schulz KF. Grimes DA, et al. Am J Obstet Gynecol. 1996 Apr;174(4):1312-5. doi: 10.1016/s0002-9378(96)70677-4. Am J Obstet Gynecol. 1996. PMID: 8623862
- Clinical research in interventional pain management techniques: the clinician's point of view. Van Zundert J. Van Zundert J. Pain Pract. 2007 Sep;7(3):221-9. doi: 10.1111/j.1533-2500.2007.00139.x. Pain Pract. 2007. PMID: 17714100 Review.
- Assessing the quality of randomization from reports of controlled trials published in obstetrics and gynecology journals. Schulz KF, Chalmers I, Grimes DA, Altman DG. Schulz KF, et al. JAMA. 1994 Jul 13;272(2):125-8. JAMA. 1994. PMID: 8015122
- Evidence-based medicine, systematic reviews, and guidelines in interventional pain management: Part 2: Randomized controlled trials. Manchikanti L, Hirsch JA, Smith HS. Manchikanti L, et al. Pain Physician. 2008 Nov-Dec;11(6):717-73. Pain Physician. 2008. PMID: 19057624 Review.
- Ethical pitfalls in neonatal comparative effectiveness trials. Modi N. Modi N. Neonatology. 2014;105(4):350-1. doi: 10.1159/000360650. Epub 2014 May 30. Neonatology. 2014. PMID: 24931328
- Impact of collegial midwifery assistance during second stage of labour on women's experience: a follow-up from the Swedish Oneplus randomised controlled trial. Häggsgård C, Edqvist M, Teleman P, Tern H, Rubertsson C. Häggsgård C, et al. BMJ Open. 2024 Jul 27;14(7):e077458. doi: 10.1136/bmjopen-2023-077458. BMJ Open. 2024. PMID: 39067883 Free PMC article. Clinical Trial.
- Methodological quality of research on perioperative immunomodulatory supplementation in oncological gastrointestinal tract surgery: a meta-research protocol. Lima LP, Mello AT, Nascimento GM, Trindade EBSM. Lima LP, et al. BMJ Open. 2024 Jul 25;14(7):e082112. doi: 10.1136/bmjopen-2023-082112. BMJ Open. 2024. PMID: 39059807 Free PMC article.
- Influence of Cavity Lining on the 3-Year Clinical Outcome of Posterior Composite Restorations: A Randomized Controlled Clinical Trial. Nguyen AD, Pütz N, Michaelis M, Bitter K, Gernhardt CR. Nguyen AD, et al. Dent J (Basel). 2024 May 7;12(5):128. doi: 10.3390/dj12050128. Dent J (Basel). 2024. PMID: 38786526 Free PMC article.
- Denosumab vs. bisphosphonates in primary osteoporosis: a meta-analysis of comparative safety in randomized controlled trials. Kobayashi T, Morimoto T, Ito K, Mawatari M, Shimazaki T. Kobayashi T, et al. Osteoporos Int. 2024 Aug;35(8):1377-1393. doi: 10.1007/s00198-024-07118-0. Epub 2024 May 11. Osteoporos Int. 2024. PMID: 38733394 Review.
- Portable robots for upper-limb rehabilitation after stroke: a systematic review and meta-analysis. Tseng KC, Wang L, Hsieh C, Wong AM. Tseng KC, et al. Ann Med. 2024 Dec;56(1):2337735. doi: 10.1080/07853890.2024.2337735. Epub 2024 Apr 19. Ann Med. 2024. PMID: 38640459 Free PMC article.
Publication types
- Search in MeSH
Related information
- Cited in Books
LinkOut - more resources
Full text sources.
- Ovid Technologies, Inc.
Other Literature Sources
- scite Smart Citations
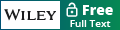
- Citation Manager
NCBI Literature Resources
MeSH PMC Bookshelf Disclaimer
The PubMed wordmark and PubMed logo are registered trademarks of the U.S. Department of Health and Human Services (HHS). Unauthorized use of these marks is strictly prohibited.
Randomization in Statistics and Experimental Design
Design of Experiments > Randomization
What is Randomization?
Randomization in an experiment is where you choose your experimental participants randomly . For example, you might use simple random sampling , where participants names are drawn randomly from a pool where everyone has an even probability of being chosen. You can also assign treatments randomly to participants, by assigning random numbers from a random number table.
If you use randomization in your experiments, you guard against bias . For example, selection bias (where some groups are underrepresented) is eliminated and accidental bias (where chance imbalances happen) is minimized. You can also run a variety of statistical tests on your data (to test your hypotheses) if your sample is random.
Randomization Techniques
The word “random” has a very specific meaning in statistics. Arbitrarily choosing names from a list might seem random, but it actually isn’t. Hidden biases (like a subconscious preference for English names, names that sound like friends, or names that roll off the tongue) means that what you think is a random selection probably isn’t. Because these biases are often hidden, or overlooked, specific randomization techniques have been developed for researchers:
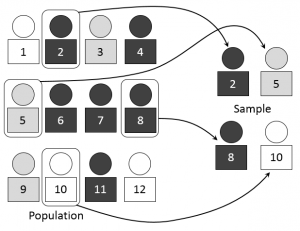
Random Experiments
A random experiment is a type of experiment that has multiple possible outcomes. Such an experiment can be repeated many times. In probability theory, once the random experiment has been performed multiple times then the experimental probabilities of various outcomes can be calculated.
An example of a random experiment is a Bernoulli trial in which there are exactly two possible outcomes. Any outcome of a random experiment cannot be predicted until the experiment has been performed. In this article, we will learn more about a random experiment, its definition, and various associated examples.
1. | |
2. | |
3. | |
4. | |
5. |
What are Random Experiments in Probability?
A random experiment is a very important part of probability theory. This is because probability theory is based on the assumption that an experiment is random and can be repeated several times under the same condition. An experiment in probability will have a sample space, a set of events as well as the probabilities of occurrence of those events.
Random Experiments Definition
Random experiments can be defined as experiments that can be performed many times under the same conditions and their outcome cannot be predicted with complete certainty. In order words, in a random experiment, all the possible outcomes are known, however, its exact outcome cannot be precisely predicted in advance. There are certain terms associated with random experiments that are given as follows:
- Sample space: A sample space can be defined as the list of all possible outcomes of a random experiment.
- Outcome: An outcome is a possible result of the random experiment.
- Event: An event is a possible outcome of an experiment and forms a subset of the sample space.
- Trial: When a random experiment is repeated many times each one is known as a trial.
Random Experiments Example
Suppose a coin is tossed. The two possible outcomes are getting a head or a tail. The outcome of this experiment cannot be predicted before it has been performed. Furthermore, it can be conducted many times under the same conditions. Thus, tossing a coin is an example of a random experiment.
Another random experiment example is that of rolling a dice . There can be 6 possible outcomes {1, 2, 3, 4, 5, 6}. However, none of the outcomes can be exactly predicted.
How to Find Probability of Random Experiments?
Probability can be defined as the likelihood of occurrence of an outcome of a random experiment. The formula for finding the probability is given as the number of favorable outcomes divided by the total number of possible outcomes of that random experiment. Suppose the probability of getting exactly two heads needs to be determined when a fair coin is tossed twice. The steps to find the probability are as follows:
- Step 1: Determine the sample space of the random experiment or the total number of outcomes. The sample space of a coin tossed twice is given as {HH, HT, TH, TT}. Thus, the total number of outcomes are 4.
- Step 2: Find the number of favorable outcomes. As the probability of getting exactly two heads needs to be determined the number of favorable outcomes is 1.
- Step 3: Apply the probability formula. Thus, the probability of getting two heads is 1 / 4 or 0.25.
Related Articles:
- Experimental Probability
- Probability Rules
- Probability and Statistics
Important Notes on Random Experiments
- A random experiment is an experiment whose outcome cannot be predicted.
- A random experiment can be performed several times under the same condition.
- The probability of a random experiment can be given by the number of favorable outcomes / total number of outcomes.
Examples on Random Experiments
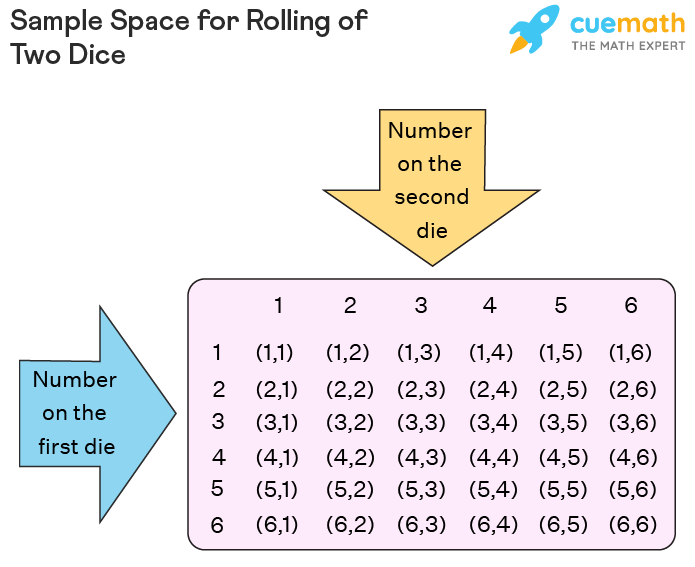
Example 2: Can picking a card from a pack of cards be classified as a random experiment?
Solution: As picking a card can be done multiple times thus, this experiment can be conducted many times.
As any card can be picked up, hence, the outcome of the experiment cannot be predicted
Thus, it is a random experiment,
Answer: Picking a card from a pack of cards is a random experiment
Example 3: If there are 3 green balls, 4 red balls, and 5 pink balls in a bag then what is the probability of drawing a pink ball?
Solution: There are a total of 12 balls in the bag.
As there are 5 pink balls thus, the number of favorable outcomes is 5
P(Pink) = favorable outcomes / total number of outcomes
Answer: The probability of drawing a pink ball is 5 / 12.
go to slide go to slide go to slide
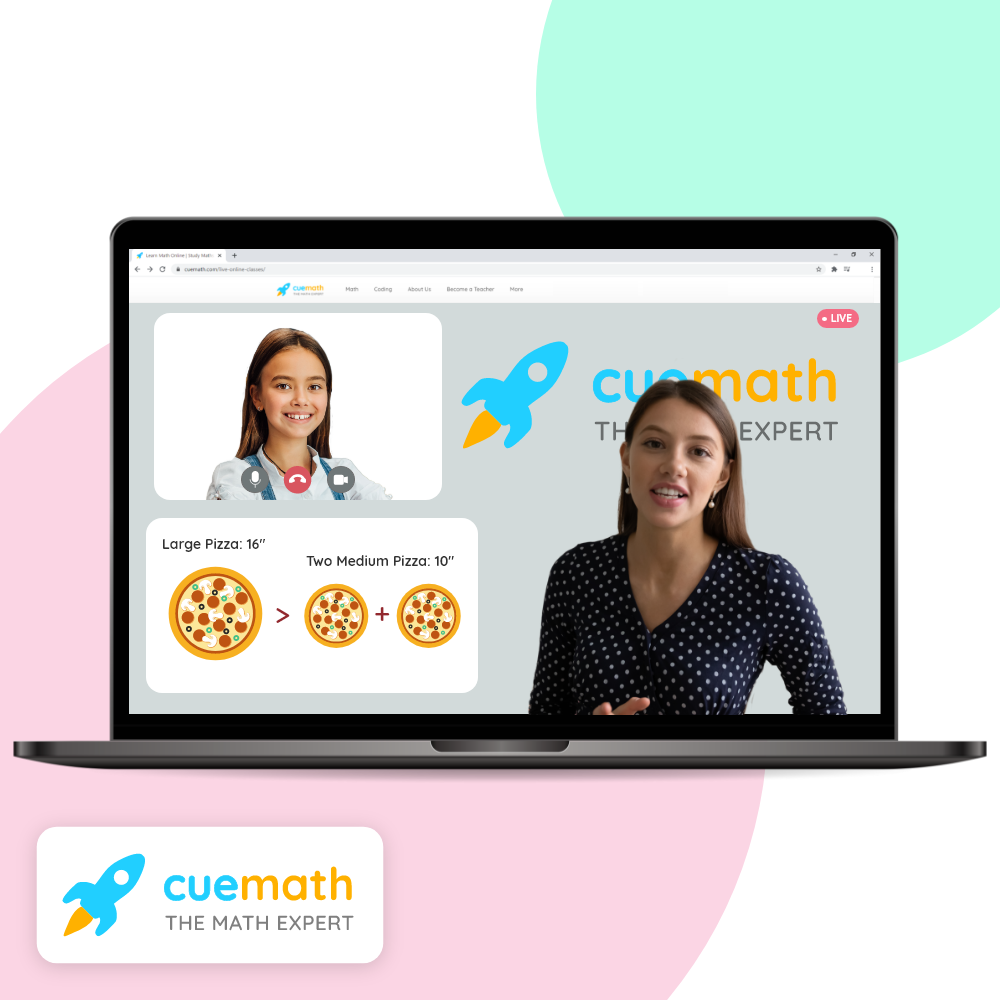
Book a Free Trial Class
FAQs on Random Experiments
What are random experiments.
Random experiments are experiments that can be performed several times and the outcome cannot be predicted beforehand.
What is the Random Experiment Sample Space and Event?
A sample space of a random experiment enlists all the possible outcomes of that experiment. However, an event is a set of possible outcomes of a random experiment that is a subset of the sample space.
What are the Two Conditions that Random Experiments Must Satisfy?
For experiments to be random experiments they must satisfy the following two conditions:
- The experiment can be arbitrarily repeated many times under the same conditions.
- The outcome of each trial of a random experiment cannot be predicted before the experiment has been performed.
What is the Formula to Find the Probability of an Outcome of a Random Experiment?
The likelihood of occurrence of any outcome of a random experiment can be calculated by the formula number of favorable outcomes / total number of outcomes.
What are the Steps to Find the Probability of a Random Experiment?
To find the probability of an outcome the steps are as follows:
- Find the total number of outcomes of the random experiment.
- Find the number of favorable outcomes.
- Divide step 2 by step 3 to determine the probability.
Can Dividing 20 by 5 Be Considered a Random Experiment?
On diving 20 by 5 the outcome will always be 4. Thus, as the outcome is predictable this cannot be classified as a random experiment.
What is a Random Variable and a Random Experiment?
A random variable is a variable that can assume all possible outcomes of a random experiment and its value changes with every trial that is performed.
- Yale Directories
Institution for Social and Policy Studies
Advancing research • shaping policy • developing leaders, why randomize.
About Randomized Field Experiments Randomized field experiments allow researchers to scientifically measure the impact of an intervention on a particular outcome of interest.
What is a randomized field experiment? In a randomized experiment, a study sample is divided into one group that will receive the intervention being studied (the treatment group) and another group that will not receive the intervention (the control group). For instance, a study sample might consist of all registered voters in a particular city. This sample will then be randomly divided into treatment and control groups. Perhaps 40% of the sample will be on a campaign’s Get-Out-the-Vote (GOTV) mailing list and the other 60% of the sample will not receive the GOTV mailings. The outcome measured –voter turnout– can then be compared in the two groups. The difference in turnout will reflect the effectiveness of the intervention.
What does random assignment mean? The key to randomized experimental research design is in the random assignment of study subjects – for example, individual voters, precincts, media markets or some other group – into treatment or control groups. Randomization has a very specific meaning in this context. It does not refer to haphazard or casual choosing of some and not others. Randomization in this context means that care is taken to ensure that no pattern exists between the assignment of subjects into groups and any characteristics of those subjects. Every subject is as likely as any other to be assigned to the treatment (or control) group. Randomization is generally achieved by employing a computer program containing a random number generator. Randomization procedures differ based upon the research design of the experiment. Individuals or groups may be randomly assigned to treatment or control groups. Some research designs stratify subjects by geographic, demographic or other factors prior to random assignment in order to maximize the statistical power of the estimated effect of the treatment (e.g., GOTV intervention). Information about the randomization procedure is included in each experiment summary on the site.
What are the advantages of randomized experimental designs? Randomized experimental design yields the most accurate analysis of the effect of an intervention (e.g., a voter mobilization phone drive or a visit from a GOTV canvasser, on voter behavior). By randomly assigning subjects to be in the group that receives the treatment or to be in the control group, researchers can measure the effect of the mobilization method regardless of other factors that may make some people or groups more likely to participate in the political process. To provide a simple example, say we are testing the effectiveness of a voter education program on high school seniors. If we allow students from the class to volunteer to participate in the program, and we then compare the volunteers’ voting behavior against those who did not participate, our results will reflect something other than the effects of the voter education intervention. This is because there are, no doubt, qualities about those volunteers that make them different from students who do not volunteer. And, most important for our work, those differences may very well correlate with propensity to vote. Instead of letting students self-select, or even letting teachers select students (as teachers may have biases in who they choose), we could randomly assign all students in a given class to be in either a treatment or control group. This would ensure that those in the treatment and control groups differ solely due to chance. The value of randomization may also be seen in the use of walk lists for door-to-door canvassers. If canvassers choose which houses they will go to and which they will skip, they may choose houses that seem more inviting or they may choose houses that are placed closely together rather than those that are more spread out. These differences could conceivably correlate with voter turnout. Or if house numbers are chosen by selecting those on the first half of a ten page list, they may be clustered in neighborhoods that differ in important ways from neighborhoods in the second half of the list. Random assignment controls for both known and unknown variables that can creep in with other selection processes to confound analyses. Randomized experimental design is a powerful tool for drawing valid inferences about cause and effect. The use of randomized experimental design should allow a degree of certainty that the research findings cited in studies that employ this methodology reflect the effects of the interventions being measured and not some other underlying variable or variables.
Random Experiments
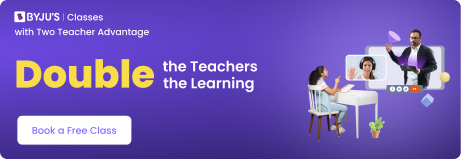
We may perform various activities in our daily existence, sometimes repeating the same actions though we get the same result every time. Suppose, in mathematics, we can directly say that the sum of all interior angles of a given quadrilateral is 360 degrees, even if we don’t know the type of quadrilateral and the measure of each internal angle. Also, we might perform several experimental activities, where the result may or may not be the same even when they are repeated under the same conditions. For example, when we toss a coin, it may turn up a tail or a head, but we are unsure which results will be obtained. These types of experiments are called random experiments.
Random Experiment in Probability
An activity that produces a result or an outcome is called an experiment. It is an element of uncertainty as to which one of these occurs when we perform an activity or experiment. Usually, we may get a different number of outcomes from an experiment. However, when an experiment satisfies the following two conditions, it is called a random experiment.
(i) It has more than one possible outcome.
(ii) It is not possible to predict the outcome in advance.
Let’s have a look at the terms involved in random experiments which we use frequently in probability theory. Also, these terms are used to describe whether an experiment is random or not.
Outcome | A possible result of a random experiment is called its outcome. Example: In an experiment of throwing a die, the outcomes are 1, 2, 3, 4, 5, or 6 |
Sample space | The set of all possible outcomes of a random experiment is called the sample space connected with that experiment and is denoted by the symbol S. Example: In an experiment of throwing a die, sample space is S = {1, 2, 3, 4, 5, 6} |
Sample point | Each element of the sample space is called a sample point. Or Each outcome of the random experiment is also called a sample point. |
Learn more about sample space here.
What is a Random Experiment?
Based on the definition of random experiment we can identify whether the given experiment is random or not. Go through the examples to understand what is a random experiment and what is not a random experiment.
Is picking a card from a well-shuffled deck of cards a random experiment?
We know that a deck contains 52 cards, and each of these cards has an equal chance to be selected.
(i) The experiment can be repeated since we can shuffle the deck of cards every time before picking a card and there are 52 possible outcomes.
(ii) It is possible to pick any of the 52 cards, and hence the outcome is not predictable before.
Thus, the given activity satisfies the two conditions of being a random experiment.
Hence, this is a random experiment.
Consider the experiment of dividing 36 by 4 using a calculator. Check whether it is a random experiment or not.
(i) This activity can be repeated under identical conditions though it has only one possible result.
(ii) The outcome is always 9, which means we can predict the outcome each time we repeat the operation.
Hence, the given activity is not a random experiment.
Examples of Random Experiments
Below are the examples of random experiments and the corresponding sample space.
Number of possible outcomes = 8
Number of possible outcomes = 36
Number of possible outcomes = 100
Similarly, we can write several examples which can be treated as random experiments.
Playing Cards
Probability theory is the systematic consideration of outcomes of a random experiment. As defined above, some of the experiments include rolling a die, tossing coins, and so on. There is another experiment of playing cards. Here, a deck of cards is considered as the sample space. For example, picking a black card from a well-shuffled deck is also considered an event of the experiment, where shuffling cards is treater as the experiment of probability.
A deck contains 52 cards, 26 are black, and 16 are red.
However, these playing cards are classified into 4 suits, namely Spades, Hearts, Diamonds, and Clubs. Each of these four suits contains 13 cards.
We can also classify the playing cards into 3 categories as:
Aces: A deck contains 4 Aces, of which 1 of every suit.
Face cards: Kings, Queens, and Jacks in all four suits, also known as court cards.
Number cards: All cards from 2 to 10 in any suit are called the number cards.
- Spades and Clubs are black cards, whereas Hearts and Diamonds are red.
- 13 cards of each suit = 1 Ace + 3 face cards + 9 number cards
- The probability of drawing any card will always lie between 0 and 1.
- The number of spades, hearts, diamonds, and clubs is the same in every pack of 52 playing cards.
An example problem on picking a card from a deck is given above.
MATHS Related Links | |
Leave a Comment Cancel reply
Your Mobile number and Email id will not be published. Required fields are marked *
Request OTP on Voice Call
Post My Comment

Register with BYJU'S & Download Free PDFs
Register with byju's & watch live videos.

An official website of the United States government
The .gov means it’s official. Federal government websites often end in .gov or .mil. Before sharing sensitive information, make sure you’re on a federal government site.
The site is secure. The https:// ensures that you are connecting to the official website and that any information you provide is encrypted and transmitted securely.
- Publications
- Account settings
Preview improvements coming to the PMC website in October 2024. Learn More or Try it out now .
- Advanced Search
- Journal List
- J Hum Reprod Sci
- v.4(1); Jan-Apr 2011
This article has been retracted.
An overview of randomization techniques: an unbiased assessment of outcome in clinical research.
Department of Biostatics, National Institute of Animal Nutrition & Physiology (NIANP), Adugodi, Bangalore, India
Randomization as a method of experimental control has been extensively used in human clinical trials and other biological experiments. It prevents the selection bias and insures against the accidental bias. It produces the comparable groups and eliminates the source of bias in treatment assignments. Finally, it permits the use of probability theory to express the likelihood of chance as a source for the difference of end outcome. This paper discusses the different methods of randomization and use of online statistical computing web programming ( www.graphpad.com /quickcalcs or www.randomization.com ) to generate the randomization schedule. Issues related to randomization are also discussed in this paper.
INTRODUCTION
A good experiment or trial minimizes the variability of the evaluation and provides unbiased evaluation of the intervention by avoiding confounding from other factors, which are known and unknown. Randomization ensures that each patient has an equal chance of receiving any of the treatments under study, generate comparable intervention groups, which are alike in all the important aspects except for the intervention each groups receives. It also provides a basis for the statistical methods used in analyzing the data. The basic benefits of randomization are as follows: it eliminates the selection bias, balances the groups with respect to many known and unknown confounding or prognostic variables, and forms the basis for statistical tests, a basis for an assumption of free statistical test of the equality of treatments. In general, a randomized experiment is an essential tool for testing the efficacy of the treatment.
In practice, randomization requires generating randomization schedules, which should be reproducible. Generation of a randomization schedule usually includes obtaining the random numbers and assigning random numbers to each subject or treatment conditions. Random numbers can be generated by computers or can come from random number tables found in the most statistical text books. For simple experiments with small number of subjects, randomization can be performed easily by assigning the random numbers from random number tables to the treatment conditions. However, in the large sample size situation or if restricted randomization or stratified randomization to be performed for an experiment or if an unbalanced allocation ratio will be used, it is better to use the computer programming to do the randomization such as SAS, R environment etc.[ 1 – 6 ]
REASON FOR RANDOMIZATION
Researchers in life science research demand randomization for several reasons. First, subjects in various groups should not differ in any systematic way. In a clinical research, if treatment groups are systematically different, research results will be biased. Suppose that subjects are assigned to control and treatment groups in a study examining the efficacy of a surgical intervention. If a greater proportion of older subjects are assigned to the treatment group, then the outcome of the surgical intervention may be influenced by this imbalance. The effects of the treatment would be indistinguishable from the influence of the imbalance of covariates, thereby requiring the researcher to control for the covariates in the analysis to obtain an unbiased result.[ 7 , 8 ]
Second, proper randomization ensures no a priori knowledge of group assignment (i.e., allocation concealment). That is, researchers, subject or patients or participants, and others should not know to which group the subject will be assigned. Knowledge of group assignment creates a layer of potential selection bias that may taint the data.[ 9 ] Schul and Grimes stated that trials with inadequate or unclear randomization tended to overestimate treatment effects up to 40% compared with those that used proper randomization. The outcome of the research can be negatively influenced by this inadequate randomization.
Statistical techniques such as analysis of covariance (ANCOVA), multivariate ANCOVA, or both, are often used to adjust for covariate imbalance in the analysis stage of the clinical research. However, the interpretation of this post adjustment approach is often difficult because imbalance of covariates frequently leads to unanticipated interaction effects, such as unequal slopes among subgroups of covariates.[ 1 ] One of the critical assumptions in ANCOVA is that the slopes of regression lines are the same for each group of covariates. The adjustment needed for each covariate group may vary, which is problematic because ANCOVA uses the average slope across the groups to adjust the outcome variable. Thus, the ideal way of balancing covariates among groups is to apply sound randomization in the design stage of a clinical research (before the adjustment procedure) instead of post data collection. In such instances, random assignment is necessary and guarantees validity for statistical tests of significance that are used to compare treatments.
TYPES OF RANDOMIZATION
Many procedures have been proposed for the random assignment of participants to treatment groups in clinical trials. In this article, common randomization techniques, including simple randomization, block randomization, stratified randomization, and covariate adaptive randomization, are reviewed. Each method is described along with its advantages and disadvantages. It is very important to select a method that will produce interpretable and valid results for your study. Use of online software to generate randomization code using block randomization procedure will be presented.
Simple randomization
Randomization based on a single sequence of random assignments is known as simple randomization.[ 3 ] This technique maintains complete randomness of the assignment of a subject to a particular group. The most common and basic method of simple randomization is flipping a coin. For example, with two treatment groups (control versus treatment), the side of the coin (i.e., heads - control, tails - treatment) determines the assignment of each subject. Other methods include using a shuffled deck of cards (e.g., even - control, odd - treatment) or throwing a dice (e.g., below and equal to 3 - control, over 3 - treatment). A random number table found in a statistics book or computer-generated random numbers can also be used for simple randomization of subjects.
This randomization approach is simple and easy to implement in a clinical research. In large clinical research, simple randomization can be trusted to generate similar numbers of subjects among groups. However, randomization results could be problematic in relatively small sample size clinical research, resulting in an unequal number of participants among groups.
Block randomization
The block randomization method is designed to randomize subjects into groups that result in equal sample sizes. This method is used to ensure a balance in sample size across groups over time. Blocks are small and balanced with predetermined group assignments, which keeps the numbers of subjects in each group similar at all times.[ 1 , 2 ] The block size is determined by the researcher and should be a multiple of the number of groups (i.e., with two treatment groups, block size of either 4, 6, or 8). Blocks are best used in smaller increments as researchers can more easily control balance.[ 10 ]
After block size has been determined, all possible balanced combinations of assignment within the block (i.e., equal number for all groups within the block) must be calculated. Blocks are then randomly chosen to determine the patients’ assignment into the groups.
Although balance in sample size may be achieved with this method, groups may be generated that are rarely comparable in terms of certain covariates. For example, one group may have more participants with secondary diseases (e.g., diabetes, multiple sclerosis, cancer, hypertension, etc.) that could confound the data and may negatively influence the results of the clinical trial.[ 11 ] Pocock and Simon stressed the importance of controlling for these covariates because of serious consequences to the interpretation of the results. Such an imbalance could introduce bias in the statistical analysis and reduce the power of the study. Hence, sample size and covariates must be balanced in clinical research.
Stratified randomization
The stratified randomization method addresses the need to control and balance the influence of covariates. This method can be used to achieve balance among groups in terms of subjects’ baseline characteristics (covariates). Specific covariates must be identified by the researcher who understands the potential influence each covariate has on the dependent variable. Stratified randomization is achieved by generating a separate block for each combination of covariates, and subjects are assigned to the appropriate block of covariates. After all subjects have been identified and assigned into blocks, simple randomization is performed within each block to assign subjects to one of the groups.
The stratified randomization method controls for the possible influence of covariates that would jeopardize the conclusions of the clinical research. For example, a clinical research of different rehabilitation techniques after a surgical procedure will have a number of covariates. It is well known that the age of the subject affects the rate of prognosis. Thus, age could be a confounding variable and influence the outcome of the clinical research. Stratified randomization can balance the control and treatment groups for age or other identified covariates. Although stratified randomization is a relatively simple and useful technique, especially for smaller clinical trials, it becomes complicated to implement if many covariates must be controlled.[ 12 ] Stratified randomization has another limitation; it works only when all subjects have been identified before group assignment. However, this method is rarely applicable because clinical research subjects are often enrolled one at a time on a continuous basis. When baseline characteristics of all subjects are not available before assignment, using stratified randomization is difficult.[ 10 ]
Covariate adaptive randomization
One potential problem with small to moderate size clinical research is that simple randomization (with or without taking stratification of prognostic variables into account) may result in imbalance of important covariates among treatment groups. Imbalance of covariates is important because of its potential to influence the interpretation of a research results. Covariate adaptive randomization has been recommended by many researchers as a valid alternative randomization method for clinical research.[ 8 , 13 ] In covariate adaptive randomization, a new participant is sequentially assigned to a particular treatment group by taking into account the specific covariates and previous assignments of participants.[ 7 ] Covariate adaptive randomization uses the method of minimization by assessing the imbalance of sample size among several covariates.
Using the online randomization http://www.graphpad.com/quickcalcs/index.cfm , researcher can generate randomization plan for treatment assignment to patients. This online software is very simple and easy to implement. Up to 10 treatments can be allocated to patients and the replication of treatment can also be performed up to 9 times. The major limitations of this software is that once the randomization plan is generated, same randomization plan cannot be generated as this uses the seed point of local computer clock and is not displayed for further use. Other limitation of this online software Maximum of only 10 treatments can be assigned to patients. Entering the web address http://www.graphpad.com/quickcalcs/index.cfm on address bar of any browser, the page of graphpad appears with number of options. Select the option of “Random Numbers” and then press continue, Random Number Calculator with three options appears. Select the tab “Randomly assign subjects to groups” and press continue. In the next page, enter the number of subjects in each group in the tab “Assign” and select the number of groups from the tab “Subjects to each group” and keep number 1 in repeat tab if there is no replication in the study. For example, the total number of patients in a three group experimental study is 30 and each group will assigned to 10 patients. Type 10 in the “Assign” tab and select 3 in the tab “Subjects to each group” and then press “do it” button. The results is obtained as shown as below (partial output is presented)
Another randomization online software, which can be used to generate randomization plan is http://www.randomization.com . The seed for the random number generator[ 14 , 15 ] (Wichmann and Hill, 1982, as modified by McLeod, 1985) is obtained from the clock of the local computer and is printed at the bottom of the randomization plan. If a seed is included in the request, it overrides the value obtained from the clock and can be used to reproduce or verify a particular plan. Up to 20 treatments can be specified. The randomization plan is not affected by the order in which the treatments are entered or the particular boxes left blank if not all are needed. The program begins by sorting treatment names internally. The sorting is case sensitive, however, so the same capitalization should be used when recreating an earlier plan. Example of 10 patients allocating to two groups (each with 5 patients), first the enter the treatment labels in the boxes, and enter the total number of patients that is 10 in the tab “Number of subjects per block” and enter the 1 in the tab “Number of blocks” for simple randomization or more than one for Block randomization. The output of this online software is presented as follows.
The benefits of randomization are numerous. It ensures against the accidental bias in the experiment and produces comparable groups in all the respect except the intervention each group received. The purpose of this paper is to introduce the randomization, including concept and significance and to review several randomization techniques to guide the researchers and practitioners to better design their randomized clinical trials. Use of online randomization was effectively demonstrated in this article for benefit of researchers. Simple randomization works well for the large clinical trails ( n >100) and for small to moderate clinical trials ( n <100) without covariates, use of block randomization helps to achieve the balance. For small to moderate size clinical trials with several prognostic factors or covariates, the adaptive randomization method could be more useful in providing a means to achieve treatment balance.
Source of Support: Nil
Conflict of Interest: None declared.

- SUGGESTED TOPICS
- The Magazine
- Newsletters
- Managing Yourself
- Managing Teams
- Work-life Balance
- The Big Idea
- Data & Visuals
- Reading Lists
- Case Selections
- HBR Learning
- Topic Feeds
- Account Settings
- Email Preferences
A Refresher on Randomized Controlled Experiments
How to design the right kind of test.
In order to make smart decisions at work, we need data. Where that data comes from and how we analyze it depends on a lot of factors — for example, what we’re trying to do with the results, how accurate we need the findings to be, and how much of a budget we have. There is a spectrum of experiments that managers can do from quick, informal ones, to pilot studies, to field experiments, and to lab research. One of the more structured experiments is the randomized controlled experiment .

- Amy Gallo is a contributing editor at Harvard Business Review, cohost of the Women at Work podcast , and the author of two books: Getting Along: How to Work with Anyone (Even Difficult People) and the HBR Guide to Dealing with Conflict . She writes and speaks about workplace dynamics. Watch her TEDx talk on conflict and follow her on LinkedIn . amyegallo
Partner Center
- Voxco Online
- Voxco Panel Management
- Voxco Panel Portal
- Voxco Audience
- Voxco Mobile Offline
- Voxco Dialer Cloud
- Voxco Dialer On-premise
- Voxco TCPA Connect
- Voxco Analytics
- Voxco Text & Sentiment Analysis

- 40+ question types
- Drag-and-drop interface
- Skip logic and branching
- Multi-lingual survey
- Text piping
- Question library
- CSS customization
- White-label surveys
- Customizable ‘Thank You’ page
- Customizable survey theme
- Reminder send-outs
- Survey rewards
- Social media
- Website surveys
- Correlation analysis
- Cross-tabulation analysis
- Trend analysis
- Real-time dashboard
- Customizable report
- Email address validation
- Recaptcha validation
- SSL security
Take a peek at our powerful survey features to design surveys that scale discoveries.
Download feature sheet.
- Hospitality
- Academic Research
- Customer Experience
- Employee Experience
- Product Experience
- Market Research
- Social Research
- Data Analysis
Explore Voxco
Need to map Voxco’s features & offerings? We can help!
Watch a Demo
Download Brochures
Get a Quote
- NPS Calculator
- CES Calculator
- A/B Testing Calculator
- Margin of Error Calculator
- Sample Size Calculator
- CX Strategy & Management Hub
- Market Research Hub
- Patient Experience Hub
- Employee Experience Hub
- NPS Knowledge Hub
- Market Research Guide
- Customer Experience Guide
- Survey Research Guides
- Survey Template Library
- Webinars and Events
- Feature Sheets
- Try a sample survey
- Professional Services

Get exclusive insights into research trends and best practices from top experts! Access Voxco’s ‘State of Research Report 2024 edition’ .
We’ve been avid users of the Voxco platform now for over 20 years. It gives us the flexibility to routinely enhance our survey toolkit and provides our clients with a more robust dataset and story to tell their clients.
VP Innovation & Strategic Partnerships, The Logit Group
- Client Stories
- Voxco Reviews
- Why Voxco Research?
- Careers at Voxco
- Vulnerabilities and Ethical Hacking
Explore Regional Offices
- Survey Software The world’s leading omnichannel survey software
- Online Survey Tools Create sophisticated surveys with ease.
- Mobile Offline Conduct efficient field surveys.
- Text Analysis
- Close The Loop
- Automated Translations
- NPS Dashboard
- CATI Manage high volume phone surveys efficiently
- Cloud/On-premise Dialer TCPA compliant Cloud on-premise dialer
- IVR Survey Software Boost productivity with automated call workflows.
- Analytics Analyze survey data with visual dashboards
- Panel Manager Nurture a loyal community of respondents.
- Survey Portal Best-in-class user friendly survey portal.
- Voxco Audience Conduct targeted sample research in hours.
- Predictive Analytics
- Customer 360
- Customer Loyalty
- Fraud & Risk Management
- AI/ML Enablement Services
- Credit Underwriting

Find the best survey software for you! (Along with a checklist to compare platforms)
Get Buyer’s Guide
- 100+ question types
- SMS surveys
- Financial Services
- Banking & Financial Services
- Retail Solution
- Risk Management
- Customer Lifecycle Solutions
- Net Promoter Score
- Customer Behaviour Analytics
- Customer Segmentation
- Data Unification
Explore Voxco
Watch a Demo
Download Brochures
- CX Strategy & Management Hub
- The Voxco Guide to Customer Experience
- Professional services
- Blogs & White papers
- Case Studies
Find the best customer experience platform
Uncover customer pain points, analyze feedback and run successful CX programs with the best CX platform for your team.
Get the Guide Now

VP Innovation & Strategic Partnerships, The Logit Group
- Why Voxco Intelligence?
- Our clients
- Client stories
- Featuresheets
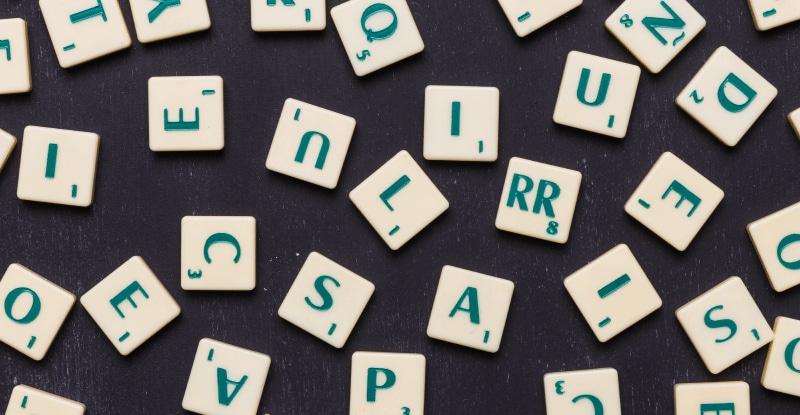
Value and Techniques of Randomization in Experimental Design
- October 5, 2021
SHARE THE ARTICLE ON
What is randomization in experimental design?
Randomization in an experiment refers to a random assignment of participants to the treatment in an experiment. OR, for instance we can say that randomization is assignment of treatment to the participants randomly.
For example : a teacher decides to take a viva in the class and randomly starts asking the students.
Here, all the participants have equal chance of getting into the experiment. Like with our example, every student has equal chance of getting a question asked by the teacher. Randomization helps you stand a chance against biases. It can be a case when you select a group using some category, there can be personal biases or accidental biases. But when the selection is random, you don’t get a chance to look into each participant and hence the groups are fairly divided.
See Voxco survey software in action with a Free demo.
Why is randomization in experimental design important?
As mentioned earlier, randomization minimizes the biases. But apart from that it also provides various benefits when adopted as a selection method in experiments.
- Randomization prevents biases and makes the results fair.
- It makes sure that the groups made for conducting an experiments are as similar as possible to each other so that the results come out as accurate as possible.
- It also helps control the lurking variables which can affect the results to be different from what they are supposed to be.
- The sample that is randomly selected is meant to be representative of the population and since it doesn’t involve researcher’s interference, it is fairly selected.
- Randomizing the experiments helps you get the best cause-effect relationships between the variables.
- It makes sure that the random selection is done from all genders, casts, races and the groups are not too different from each other.
- Researchers control values of the explanatory variable with a randomization procedure. So, if we see a relationship between the explanatory variable and response variables, we can say that it is a causal one.
What are different types of randomization techniques in experimental design?
Randomization can be subject to errors when it comes to “randomly” selecting the participants. As for our example, the teacher surely said she will ask questions to random students, but it is possible that she might subconsciously target mischievous students. This means we think the selection is random, but most of the times it isn’t.
Hence, to avoid these unintended biases, there are three techniques that researchers use commonly:
Simple Random Sampling
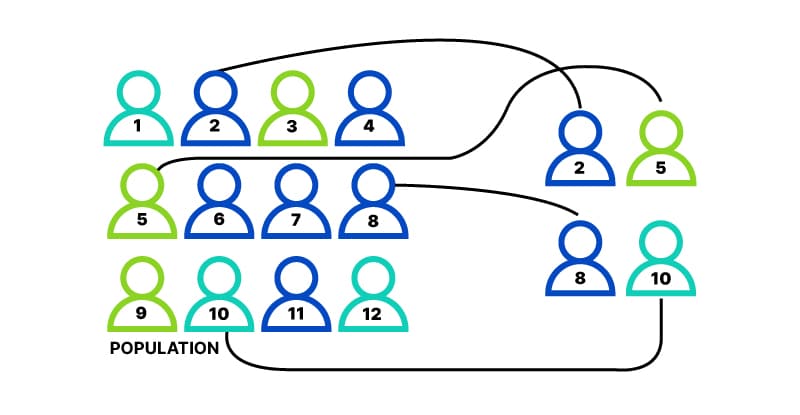
In simple random sampling. The selection of the participants is completely luck and probability based. Every participant has an equal chance of getting into the sample.
This method is theoretically easy to understand and works best against a sample size of 100 or more. The main factor here is that every participants gets an equal chance of being included in a treatment, and this is why it is also called the method of chances.
Methods of simple random sampling:
- Lottery – Like the old ways, the participants are given a number each. The selection is done by randomly drawing a number from the pot.
- Random numbers – Similar to the lottery method, this includes giving numbers to the participants and using random number table.
Example : A teacher wants to know how good her class is in mathematics. So she will give each student a number and will draw numbers from a bunch of chits. This will include a randomly selected sample size and It won’t have any biases depending on teachers interference.

Permuted Block Randomization
It is a method of randomly assigning participants to the treatment groups. A block is a group is randomly ordered treatment group. All the blocks have a fair balance of treatment assignment throughout.
Example : A teacher wants to enroll student in two treatments A and B. and she plans to enroll 6 students per week. The blocks would look like this:
Week 1- AABABA
Week 2- BABAAB
Week 3- BBABAB
Each block has 9 A and 9 B. both treatments have been balanced even though their ordering is random.
There are two types of block assignment in permuted block randomization:
- Random number generator
Generate a random number for each treatment that is assign in the block. In our example, the block “Week 1” would look like- A(4), A(5), B(56), A(33), B(40), A(10)
Then arrange these treatments according to their number is ascending order, the new treatment could be- AAABB
- Permutations
This includes listing the permutations for the block. Simply, writing down all possible variations.
The formula is b! / ((b/2)! (b/2)!)
For our example, the block sixe is 6, so the possible arrangements would be:
6! / ((6/2)! (6/2)!)
6! / (3)! x (3)!
6x5x4x3x2x1 / (3x2x1) x (3x2x1)
20 possible arrangements.
Stratified Random Sampling
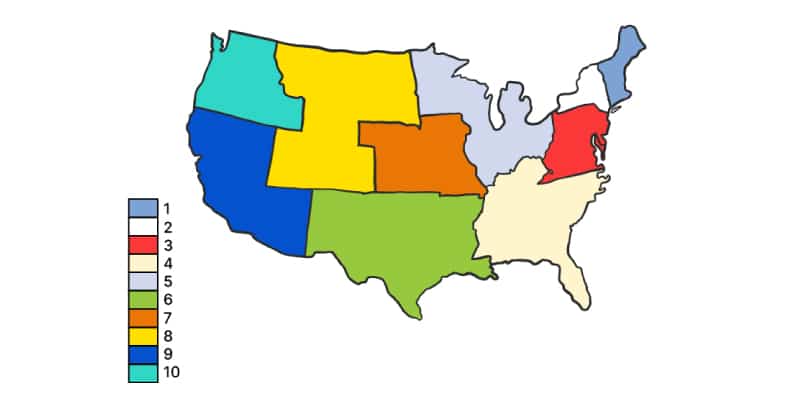
The word “strata” refers to characteristics. Every population has characteristics like gender, cast, age, background etc. Stratified random sampling helps you consider these stratum while sampling the population. The stratum can be pre-defined or you can define them yourself any way you think is best suitable for your study.
Example: you want to categorize population of a state depending on literacy. Your categories would be- (1) Literate (2) Intermediate (3) Illiterate.
Steps to conduct stratified random sampling:
- Define the target audience.
- Identify the stratification variables and decide the number of strata to be used.
- Using a pre-existent sampling frame or by creating a frame that includes all the information of the stratification variable for the elements in the target audience.
- Make changes after evaluating the sampling frame depending on its coverage.
- Each stratum should be unique and should cover each and every member of the population.
- Assign a random, unique number to each element.
- Define the size of each stratum according to your requirement.
- The researcher can then select random elements from each stratum to form the sample.
Explore all the survey question types possible on Voxco
Explore Voxco Survey Software
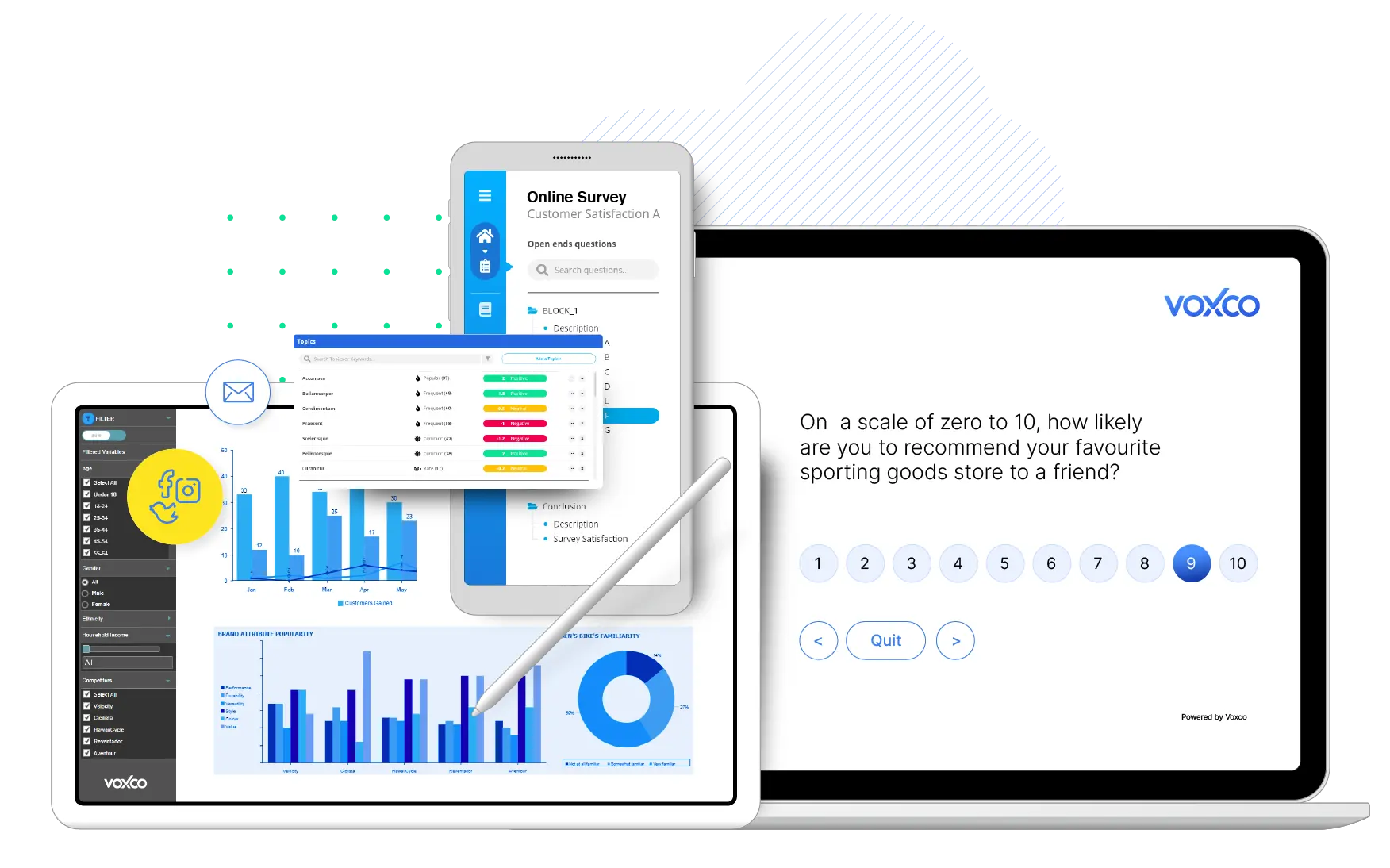
+ Omnichannel Survey Software
+ Online Survey Software
+ CATI Survey Software
+ IVR Survey Software
+ Market Research Tool
+ Customer Experience Tool
+ Product Experience Software
+ Enterprise Survey Software
Dual Axis Chart
Dual Axis Charts: Beyond Simple Visualization SHARE THE ARTICLE ON Table of Contents A dual axis chart also known as multiple axes chart, employs two
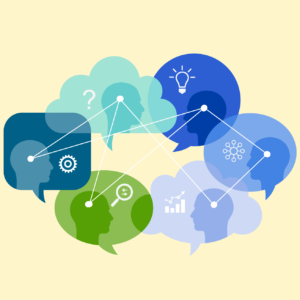
Leverage Marketing Mix Modeling to maximize your ROI
Leverage Marketing Mix Modeling to maximize your Research ROI SHARE THE ARTICLE ON Share on facebook Share on twitter Share on linkedin Table of Contents
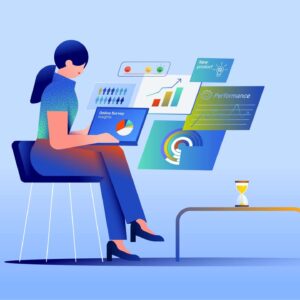
How to make the most of your online surveys?
As channels for gathering feedback from customers and employees, online surveys play a vital role in the organization’s growth. By gaining insights into how people
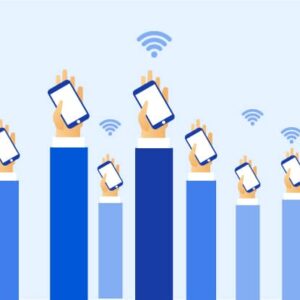
Internet Survey Panel
Internet Survey Panel SHARE THE ARTICLE ON Share on facebook Share on twitter Share on linkedin Table of Contents What is a Panel? In market
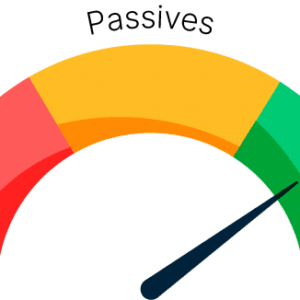
The common threads tying companies with high NPS together
The common threads tying companies with high NPS® together Maximize NPS® Insights Unlock insights to drive growth, improve customer engagement and provide detailed customer feedback
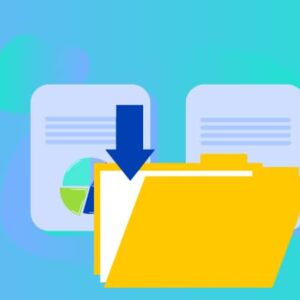
Data Warehouse: Why it is Essential for a Business
Data Warehouse: Why it is Essential for a Business SHARE THE ARTICLE ON Share on facebook Share on twitter Share on linkedin Table of Contents
We use cookies in our website to give you the best browsing experience and to tailor advertising. By continuing to use our website, you give us consent to the use of cookies. Read More
Name | Domain | Purpose | Expiry | Type |
---|---|---|---|---|
hubspotutk | www.voxco.com | HubSpot functional cookie. | 1 year | HTTP |
lhc_dir_locale | amplifyreach.com | --- | 52 years | --- |
lhc_dirclass | amplifyreach.com | --- | 52 years | --- |
Name | Domain | Purpose | Expiry | Type |
---|---|---|---|---|
_fbp | www.voxco.com | Facebook Pixel advertising first-party cookie | 3 months | HTTP |
__hstc | www.voxco.com | Hubspot marketing platform cookie. | 1 year | HTTP |
__hssrc | www.voxco.com | Hubspot marketing platform cookie. | 52 years | HTTP |
__hssc | www.voxco.com | Hubspot marketing platform cookie. | Session | HTTP |
Name | Domain | Purpose | Expiry | Type |
---|---|---|---|---|
_gid | www.voxco.com | Google Universal Analytics short-time unique user tracking identifier. | 1 days | HTTP |
MUID | bing.com | Microsoft User Identifier tracking cookie used by Bing Ads. | 1 year | HTTP |
MR | bat.bing.com | Microsoft User Identifier tracking cookie used by Bing Ads. | 7 days | HTTP |
IDE | doubleclick.net | Google advertising cookie used for user tracking and ad targeting purposes. | 2 years | HTTP |
_vwo_uuid_v2 | www.voxco.com | Generic Visual Website Optimizer (VWO) user tracking cookie. | 1 year | HTTP |
_vis_opt_s | www.voxco.com | Generic Visual Website Optimizer (VWO) user tracking cookie that detects if the user is new or returning to a particular campaign. | 3 months | HTTP |
_vis_opt_test_cookie | www.voxco.com | A session (temporary) cookie used by Generic Visual Website Optimizer (VWO) to detect if the cookies are enabled on the browser of the user or not. | 52 years | HTTP |
_ga | www.voxco.com | Google Universal Analytics long-time unique user tracking identifier. | 2 years | HTTP |
_uetsid | www.voxco.com | Microsoft Bing Ads Universal Event Tracking (UET) tracking cookie. | 1 days | HTTP |
vuid | vimeo.com | Vimeo tracking cookie | 2 years | HTTP |
Name | Domain | Purpose | Expiry | Type |
---|---|---|---|---|
__cf_bm | hubspot.com | Generic CloudFlare functional cookie. | Session | HTTP |
Name | Domain | Purpose | Expiry | Type |
---|---|---|---|---|
_gcl_au | www.voxco.com | --- | 3 months | --- |
_gat_gtag_UA_3262734_1 | www.voxco.com | --- | Session | --- |
_clck | www.voxco.com | --- | 1 year | --- |
_ga_HNFQQ528PZ | www.voxco.com | --- | 2 years | --- |
_clsk | www.voxco.com | --- | 1 days | --- |
visitor_id18452 | pardot.com | --- | 10 years | --- |
visitor_id18452-hash | pardot.com | --- | 10 years | --- |
lpv18452 | pi.pardot.com | --- | Session | --- |
lhc_per | www.voxco.com | --- | 6 months | --- |
_uetvid | www.voxco.com | --- | 1 year | --- |
Thank you for visiting nature.com. You are using a browser version with limited support for CSS. To obtain the best experience, we recommend you use a more up to date browser (or turn off compatibility mode in Internet Explorer). In the meantime, to ensure continued support, we are displaying the site without styles and JavaScript.
- View all journals
- Explore content
- About the journal
- Publish with us
- Sign up for alerts
- Open access
- Published: 21 August 2024
A randomized controlled experiment testing the use of virtual reality to trigger cigarette craving in people who smoke
- Aitor Rovira 1 , 2 ,
- Sinéad Lambe 1 , 2 ,
- Helen Beckwith 1 , 2 ,
- Memoona Ahmed 1 ,
- Felicity Hudson 1 ,
- Phoebe Haynes 1 ,
- Chun-Jou Yu 3 ,
- Kira Williams 1 ,
- Simone Saidel 1 ,
- Ellen Iredale 1 ,
- Sapphira McBride 1 ,
- Felicity Waite 1 , 2 ,
- Xueni Pan 3 &
- Daniel Freeman 1 , 2
Scientific Reports volume 14 , Article number: 19445 ( 2024 ) Cite this article
214 Accesses
5 Altmetric
Metrics details
- Human behaviour
Automated delivery of therapy in virtual reality (VR) has the potential to be used for smoking cessation. Most obviously, it could be used to practise and establish alternative reactions to smoking cues. The first step in treatment development is to show that VR environments can trigger sufficient cravings in smokers. We evaluated a new VR public house outdoor scenario with 100 individuals who smoked daily. Participants were randomly assigned to the VR scenario with smoking cues or a neutral experience in VR. The VR experiences were presented in a standalone VR headset. Before and after VR, we collected self-reported craving scores for cigarettes and alcohol using the Tobacco Craving Questionnaire (TCQ) and visual analogue scales (VAS). Physiological data were also collected. Compared to the neutral condition, exposure to the smoking cues led to a large increase in craving for a cigarette (TCQ β = 11.44, p < 0.0001, Cohen’s d = 1.10) and also a moderate increase in craving for alcohol \((\upbeta =0.7,\text{ p}=0.017,\text{ d}=0.50)\) . There were no significant physiological differences between the two conditions. These results provide good evidence that VR experiences can elicit strong craving for cigarettes. The programming can be part of developing a new VR cognitive therapy to help people reduce smoking.
Similar content being viewed by others
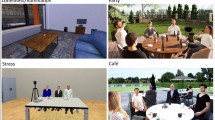
Cigarette craving in virtual reality cue exposure in abstainers and relapsed smokers
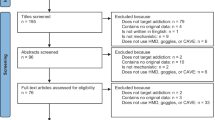
Virtual reality: a powerful technology to provide novel insight into treatment mechanisms of addiction
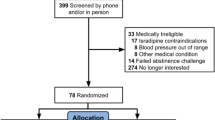
Isradipine augmentation of virtual reality cue exposure therapy for tobacco craving: a triple-blind randomized controlled trial
Introduction.
People often smoke in response to specific cues such as seeing a cigarette, ashtray, or matches 1 , 2 . Hence exposure to smoking cues is an important step in therapies designed to build resilience to craving 3 . Presentation of smoking cues within virtual reality (VR) has been shown to elicit cigarette craving 4 . There are two key advantages of use of VR. First, multiple different smoking cues and scenarios, graduated in difficulty, can be easily presented and there are no actual cigarettes present to smoke and reinforce the established response. Second, it is now possible to automate delivery of therapy within VR 5 . We have successfully piloted with thirteen smokers a VR smoking environment delivered in the new generation of standalone VR headsets 6 . In this paper, we report a definitive test of this environment as the first step in developing a new VR therapy for smoking cessation.
One important reason that prevents people who smoke from successfully quitting is the difficulty of not responding to everyday smoking cues 1 . Smoking cues can be pervasive in daily lives and exposure to these cues is a predictor of smoking 7 . These cues may be specific items related to smoking, such as ashtrays and cigarette butts, and general environments where people usually smoke, such as a bar, and can include time-related events such as a morning coffee routine 8 . Exposure to smoking cues elicits craving, and craving has been identified as the mediator that leads to smoking 9 , 10 , 11 . Drinking alcohol is another well-recognised cue for smoking 12 and people who drink alcohol are more likely to smoke too 13 .
Exposure to smoking cues triggering craving for a cigarette is a well-replicated phenomenon 1 , 14 . The results are consistent across different means of presentation, including pictures 15 , video 16 , feature films 17 , and VR 18 . VR clearly provides a higher degree of experimental control compared to studying occurrences of smoking in a natural setup and also provides a higher degree of immersion and interaction than 2D technologies, in which a typical setup offers a reduced field of view and participants are simply spectators 19 . Furthermore, VR allows the placement of people in a surrounding virtual environment that may be associated to smoking, thus triggering craving from a broad contextual cue 20 .
In a systematic review of 18 studies that involved 541 smokers, it has been shown that VR presentation of cues can produce a large triggering of craving (Cohen’s d = 1.0) 21 . In the largest study to date we wanted to show that similar effects can be produced from delivery of VR scenes in the new generation of standalone headsets. This could then form the basis of the development of a new cognitive intervention for smoking cessation. There is extensive evidence in the literature that exposure to smoking cues in VR triggers craving 18 , 22 , 23 . However, only a few papers have reported the results of randomised control tests of the use of immersive VR technologies so far. A number of studies used VR to expose smokers to cues 24 or used VR to try to improve the results of an approach bias modification approach 25 . Other studies used more limited technologies such as 360-degree videos 26 and Second Life 27 . These studies have taken different approaches in experimental design or the experimental setup, making it difficult to compare their results. None have tested long-term effects.
Subjective measurements are the most common way to assess cravings. A small number of studies have also supplemented self-report with objective measurements such as physiological data. For example, it has been suggested that craving can be a predictor of physiological arousal 28 , and skin conductance has been shown to increase after exposure to smoking cues 29 . Therefore we also included physiological measures in our test.
In our pilot study, simple pre and post testing with 13 smokers indicated that our new VR environment may increase smoking craving 6 . In this paper, we present the results of a randomised controlled study with 100 participants using the same VR smoking cue scenario. We collected self-reported measurements through questionnaires and physiological data related to heart rate and skin conductance.
Experimental design
The study was a between-subject experiment in which we carried out a between group comparison of smoking craving scores after going through a VR experience, either a neutral environment or a scenario depicting potential smoking cues. Participants were randomly allocated to an experimental group using the online tool Sealed Envelope ( https://www.sealedenvelope.com/ ). Ethics approval was granted by the University of Oxford Central University Research Ethics Committee (reference R81586/RE001). All research was performed in accordance with relevant guidelines/regulations, and written informed consent was provided by all participants.
Participants
Participants were recruited through advertisements on social media and local radio stations. The inclusion criteria were: over 18 years old and smoke a minimum of 10 cigarettes per day. The exclusion criteria were: photosensitive epilepsy; significant visual, auditory, or balance impairment; insufficient comprehension of English to complete study measures; using nicotine replacements; primary diagnosis of another substance dependency; or medication that reduces nicotine cravings (e.g. Bupropion).
The main outcome of the study was the score from the Tobacco Craving Questionnaire 30 . It is a 12-item short version of the long 47-item questionnaire. The long version is validated and reported as being reliable for research 31 and the short version has similar internal consistency to the original version. It assesses craving for a cigarette at the time of filling it out, with answers on a 1 (strongly disagree) to 7 (strongly agree) Likert scale. Participants filled out this questionnaire before and after the VR experience. Scores can range from 12 to 84. Higher scores indicate greater craving for a cigarette.
As additional outcomes, participants provided subjective scores of their current cravings of cigarettes and alcohol using two visual analogue scales (VAS), with answers from 0 (Do not want one at all) to 10 (Extremely want one). Participants provided their current cravings before and after the VR experience. Higher scores indicate greater craving. Although internal consistency cannot be checked due to being just a single item, the use of VAS has been an increasingly popular method to quantify subjective experiences (e.g. pain 32 ), and has been found to be a valid way to measure intensity of cigarette craving 33 .
At baseline participants completed the Heaviness of Smoking Index (HSI) 34 , which is a 2-item questionnaire to assess how much a person smokes, including the questions “How many cigarettes do you typically smoke per day?” and “How soon after you wake up do you have your first cigarette?” Answers were given as categorical numbers. These two measurements have been shown to be fairly reliable when used either separately or together 35 .
At baseline participants completed the AUDIT-C 36 , which is a three-item questionnaire to assess average alcohol use. Multiple studies have validated this questionnaire 37 .
Electrodermal activity and heart rate were recorded during baseline and the VR experience with the use of an Empatica E4 wristband ( https://www.empatica.com/en-gb/research/e4/ ). Data included two pairs of inter beat interval (IBI) and electrodermal activity (EDA) files, one pair for baseline and the other recorded during the VR experience.
VR scenarios
We used the Meta Quest 2 VR headset in standalone mode for all the VR sessions. That means we used the VR headset without using a computer to run the simulation. There were two scenarios, one for the experimental group and one for the control group. Each lasted three minutes. In both scenarios participants sat down for the entire duration of the experience.
In the experimental group, participants were placed in an environment that resembled a British pub outdoor space 6 (see Fig. 1 ). There were several people sitting around as on a typical warm sunny day. There were several items related to smoking cues in the scenario—pint glasses, ashtrays with cigarette butts, one of them half extinguished and still releasing a trail of smoke. On the bench next to the participant, two virtual characters were chatting. Over time their discussion turned towards cigarettes and how hard it is to quit smoking. At the end of the scenario, one of the characters turned their head towards the participant and asked them directly if they wanted a cigarette.
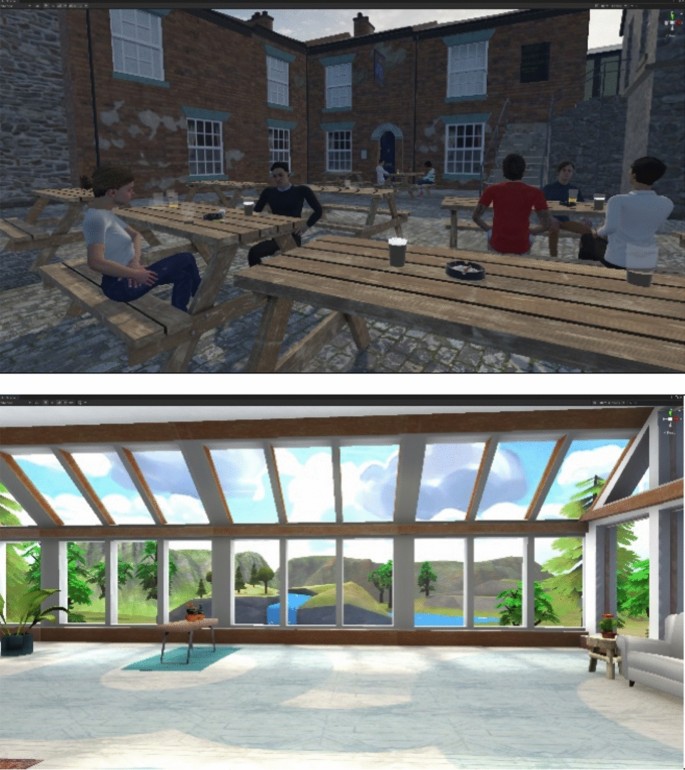
Screenshots of the VR scenarios taken from the initial perspective of the participant (1) the beer garden; (2) the room in the neutral environment. Images created in Unity 2020.3.3f1 ( https://unity.com/releases/editor/whats-new/2022.3.3 ).
In the control group, participants visited a neutral environment in a modern house with wide windows, similar to the welcome room described in 38 (see Fig. 1 ). The landscape outside included different types of vegetation, a water stream, and clear blue sky. The environment included quiet background music. This environment did not contain any smoking cues.
No other hardware was required besides the VR headset, the Empatica E4 wristband, and a smartphone to record the physiological data.
Experimental procedures
Participants were asked to refrain from smoking 30 min before coming to the VR lab. Upon arrival, they were met at the reception area by a researcher who guided them to the VR lab. Once in the lab, they were asked to confirm that they had read the information sheet at least 24 h prior to the VR session and if willing to participate, to sign a consent form. After agreeing to participate, they filled out the AUDIT-C, the Tobacco Craving Questionnaire, and the visual analogue scales to obtain baseline measurements for the initial craving scores.
When they completed the questionnaires, they were randomised and allocated to the experimental condition they were instructed to remain seated for the rest of the session. A researcher helped them put the Empatica E4 wristband on their dominant arm and recorded two minutes of physiological data as a baseline. After that, participants put the VR headset on, the researcher made sure that vision was clear and the headset had been adjusted to the participant’s comfort, the Empatica E4 started recording data once again, and the VR experience started.
After the VR experience ended, the researcher helped them to take the VR headset off and were asked to fill out the Tobacco Craving Questionnaire and the visual analogue scales again. Participants were compensated twenty pounds for their time.
Data analysis
Analyses were conducted in R version 4.3.0 39 . The main outcome was the craving score on the TCQ after the VR experience. We carried out a linear regression analysis with experimental group (StudyCondition) as the independent variable and controlling for initial craving scores.
The scores reported by participants on the two VASs (cigarettes and alcohol) were also analysed using linear regression. Similarly, we used group as the independent variable and controlling for initial craving scores. Linear regression analyses were carried out in R using the lm function. Effect sizes were summarised as Cohen’s d values calculated using the cohen.d function in R.
We tested scores for how much participants smoked (HSI) and drank (AUDIT-C) on average as possible moderators of the main outcome with the following equation:
Electrodermal activity (EDA) data pre-processing and initial visual analysis was carried out using the Matlab-based tool Ledalab 40 . We carried out a visual inspection on each dataset to detect anomalies in the data. We discarded the data from participants if more than 50% of their data were zero on either the baseline or the VR experience dataset. We also discarded the data showing sudden jumps that were too abrupt to be attributed to a change in skin conductance and did not recover to the original level after a few seconds.
Data cleaning 41 included data trimming, smoothing, and correction of artifacts originated from bad readings. We trimmed a few seconds in the beginning and in the end of the dataset as it was common that there were a few faulty readings at both ends. Trimming was done manually keeping the data from the moment the function looked stable. We smoothed out the data to remove high frequency noise using a filter with a Gauss window size 8. We also removed any isolated spike due to bad readings and we reconstructed the signal with either a linear or a Spline interpolation, depending on what was more suitable in each case. The sample rate was kept at the recording rate of 4 Hz.
We split the signal between the tonic and the phasic components using continuous decomposition analysis 40 . The tonic component provides an overall background level and tendency over time of the signal, while the phasic component contains the information about sudden peaks and changes. We then analysed both components separately. We looked at the mean and standard deviation in the tonic component during the VR experience relative to the baseline. For this, we divided both the mean and standard deviation obtained in the VR experience by the values obtained in the baseline. We also looked at the skin conductance level (SCL) as the gradient of the tonic component. In the phasic component, we studied the mean and standard deviation relative to baseline the same way we calculated it in the tonic component. We then compared these extracted features between experimental groups.
Regarding heart rate data, we were interested in the heart rate variability (HRV), calculated from the IBI data. These data are processed only when the two beats are detected, thus the number of samples varied between participants. The mean and standard deviation of the HRV used in the statistical analysis were also relative to the baseline values.
We analysed all the features extracted from both EDA and IBI signals in a linear regression with the experimental group as the sole independent variable.
30 male and 22 female participants were allocated to the experimental group. The average age was 39.12 (SD = 15.12). In the control group there were 27 male and 21 female participants, with an average age of 37.77 (SD = 14.49). No participants selected their gender as either ‘non-binary’ or ‘preferred not to say’. In the experimental group, the average HSI score was 3.33 (SD = 1.20) and the AUDIT-C score was 5 (SD = 3.01), and in the control group, the average HSI was 3.25 (SD = 0.84) and the average AUDIT-C score was 6.25 (SD = 2.97).
Table 1 shows the scores of the three questionnaire outcomes. All three scores obtained after the VR experience were statistically different between experimental groups. Compared to the neutral condition, the experimental group had large effect size increases in cigarette craving and a moderate increase in craving for alcohol. The Heaviness of Smoking Index reported during screening predicted cigarette craving after the VR session across both experimental groups (HSI, p < 0.0001) and alcohol use did not (AUDIT-C, p = 0.85). Looking within groups (i.e. pre to post changes), the experimental group increased in scores on the TCQ (p < 0.01), the VAS for cigarettes (p < 0.0001), and the VAS for alcohol (p < 0.001). The control group decreased in scores on the TCQ (p < 0.0001) and the VAS for cigarettes (p = 0.02) but did not significantly alter in alcohol craving (p = 0.18).
There were missing data from the physiological recordings. We had electrodermal activity (EDA) data from 71 participants (42 in the experimental group and 29 in the control group). Table 2 shows the mean, the standard deviation, and the results from the regression analyses of the different features extracted from the physiological data. The results did not show any statistically significant difference between experimental groups on any of the features extracted.
We conducted the largest experimental test of whether VR simulations can produce craving for cigarettes in people who smoke regularly. Importantly the test used the latest standalone headset without use of an external computer. The VR public house scene produced a large increase in cigarette craving compared to a neutral VR scene. The Cohen’s d for cigarette craving was 1.1, which is similar to the effect size reported in a meta-analysis of studies focused on cue-induced craving in VR 21 . The VR pub experience led to significantly increased levels of craving from before to after immersion (i.e., there was a within group effect), but it should be noted when considering the magnitude of the between groups effect that there was also a significant reduction in cigarette craving in the neutral experience. This reduction may perhaps be explained by the use of VR technology being interesting for the participant and hence distracting from cravings. Furthermore, it is possible that the virtual environment, which had windows with views to a natural landscape, might have been found calming like a relaxation nature scene 42 . The VR pub scene also led to an increase in the smokers in craving for alcohol. The results once again show how VR can induce similar responses to real-world environments. Our VR pub scene could form the basis for the development of a smoking cessation therapy.
We tested whether level of smoking and alcohol consumption affected responses. Neither had a differential affect by the type of VR scene. However, people who reported smoking a greater number of cigarettes had higher levels of cigarette craving in both the VR scene and the neutral scene. In contrast, level of alcohol use did not predict level of cigarette craving in VR. This further validates the use of VR, since it shows that, as would be expected, cravings elicited in VR are affected by a person’s severity of smoking (but not alcohol use).
Regarding the physiological information collected, we explored different features from electrodermal and heart rate data that could be related to craving. The tonic component in the electrodermal data could reveal an overall increase in anxiety. We analysed the mean and standard deviation of this signal relative to the data recorded during baseline. We also looked at skin conductance by calculating the gradient of the linear regression. Electrodermal values naturally change over time, so we predicted that in the experimental group there would be a significantly higher number of participants with a positive slope compared to the control group. We did not find evidence that any feature was statistically different between the randomised groups. Table 2 shows that the values are in the decimals, so we speculate that the signal-to-noise ratio was possibly close to zero dB. Skin conductance values were low. That means that the overall tonic values did not change to any great degree for any participant. Analysing the tonic driver might be more meaningful in longer experiences than three minutes.
The phasic signal is a marker of how participants respond to specific events during the experience. The VR pub scenario contained several smoking cues. We were interested in looking at whether these cues could trigger craving in very specific timestamps thus showing a spike in the data. We analysed the mean and standard deviation of the phasic driver, but the results did not show any difference in the data between the two groups. Data from an accelerometer can provide the information to see if a change in the electrodermal data comes from the movement of limbs. Additionally, electrodermal values can change when people talk, so a voice detection algorithm could be helpful. We finally studied the inter-beat interval to look for statistical differences in the heart rate variability. Again, we looked at the mean and standard deviation relative to baseline for each participant. The results did not show any statistical differences in this case either. Given the strong findings for subjective craving, it is plausible that we did not assess the most useful physiological information to detect it.
The Empatica E4 device has been validated 43 , 44 . However, there were missing data. Researchers need procedures in place for setting it up correctly and to expect that the signal might change if the wristband moves. Recordings were three minutes long. Longer sessions would have provided better estimations of the tonic driver and the overall skin conductance level over time. On the other hand, the phasic signal detecting peaks should not have been too affected by the length of the recording. However, it should be kept in mind that changes in the phasic driver induced by stimuli will be reflected in the signal a few seconds later, between one and five seconds 40 . Our scenario with smoking cues ended with one of the characters looking directly at the participant and offering a cigarette. Recording was stopped right at that moment, whereas it should have carried on longer to capture the response. For the phasic driver, it is important to make an estimation of the signal-to-noise ratio (SNR) to facilitate the task of discriminating the peaks from the noise. We applied a smoothing function when preparing the signal before the decomposition but the phasic signal was not completely noise-free.
There are considerations when using the Empatica E4. If the band is too loose, the readings will vary and data will become unreliable. If the band is too tight, it can create discomfort and interfere with the VR experience. Ideally, the signal acquired needs to be as clean as possible to minimise the amount of post-processing. Another consideration that we noticed is that the wristband has a button that needs to be pressed to start and stop recording data. When the button is pressed, the sensors are pressed against the skin of the person wearing it and that is clearly visible in the data with short spikes and oscillation on the first seconds of the recording, as well as at the end. The data needed to be trimmed but, ideally, the best way to operate the wristband is remotely via the API provided by the manufacturer.
Developing VR therapies based on rigorous experiment is more likely to lead to clinically successful outcomes. This study not only confirms the base potential for VR in helping people smoke less but shows that the scenario can form part of the content of a VR therapy.
Data availability
Deidentified data are available from the corresponding authors on reasonable request and contract with the university.
Droungas, A., Ehrman, R. N., Childress, A. R. & O’Brien, C. P. Effect of smoking cues and cigarette availability on craving and smoking behavior. Addict. Behav. 20 (5), 657–673. https://doi.org/10.1016/0306-4603(95)00029-C (1995).
Article CAS PubMed Google Scholar
Niaura, R. S., Monti, P. M. & Pedraza, M. Relevance of cue reactivity to understanding alcohol and smoking relapse. J. Abnorm. Psychol. 97 (2), 133 (1988).
Martin, T., LaRowe, S. D. & Malcolm, R. Progress in cue exposure therapy for the treatment of addictive disorders: A review update. Open Addict. J. 3 (1), 92–101 (2010).
Article CAS Google Scholar
Bordnick, P. S. et al. Utilizing virtual reality to standardize nicotine craving research: A pilot study. Addict. Behav. 29 (9), 1889–1894. https://doi.org/10.1016/j.addbeh.2004.06.008 (2004).
Article PubMed Google Scholar
Freeman, D. et al. Automated psychological therapy using immersive virtual reality for treatment of fear of heights: A single-blind, parallel-group, randomised controlled trial. Lancet Psychiatry 5 (8), 625–632. https://doi.org/10.1016/S2215-0366(18)30226-8 (2018).
Article PubMed PubMed Central Google Scholar
Yu, C.-J., Rovira, A., Pan, X. & Freeman, D. A validation study to trigger nicotine craving in virtual reality. In 2022 IEEE Conference on Virtual Reality and 3D User Interfaces Abstracts and Workshops (VRW) 868–869. https://doi.org/10.1109/VRW55335.2022.00285 (2022).
Herman, C. P. External and internal cues as determinants of the smoking behavior of light and heavy smokers. J. Pers. Soc. Psychol. 30 (5), 664–672. https://doi.org/10.1037/h0037440 (1974).
Conklin, C. A., Robin, N., Perkins, K. A., Salkeld, R. P. & McClernon, F. J. Proximal versus distal cues to smoke: The effects of environments on smokers’ cue-reactivity’. Exp. Clin. Psychopharmacol. 16 (3), 207–214. https://doi.org/10.1037/1064-1297.16.3.207 (2008).
Allen, S. S., Bade, T., Hatsukami, D. & Center, B. Craving, withdrawal, and smoking urges on days immediately prior to smoking relapse. Nicotine Tob. Res. 10 (1), 35–45. https://doi.org/10.1080/14622200701705076 (2008).
Conklin, C. A. et al. Examining the relationship between cue-induced craving and actual smoking. Exp. Clin. Psychopharmacol. 23 (2), 90–96. https://doi.org/10.1037/a0038826 (2015).
Wray, J. M., Gass, J. C. & Tiffany, S. T. A systematic review of the relationships between craving and smoking cessation. Nicotine Tob. Res. 15 (7), 1167–1182. https://doi.org/10.1093/ntr/nts268 (2013).
Mintz, J., Boyd, G., Rose, J. E., Charuvastra, V. C. & Jarvik, M. E. Alcohol increases cigarette smoking: A laboratory demonstration. Addict. Behav. 10 (3), 203–207. https://doi.org/10.1016/0306-4603(85)90001-2 (1985).
Bien, T. H. & Burge, R. Smoking and drinking: A review of the literature. Int. J. Addict. 25 (12), 1429–1454. https://doi.org/10.3109/10826089009056229 (1990).
Robbins, S. J. & Ehrman, R. N. Designing studies of drug conditioning in humans. Psychopharmacology (Berl.) 106 (2), 143–153. https://doi.org/10.1007/BF02801965 (1992).
Lochbuehler, K., Engels, R. C. M. E. & Scholte, R. H. J. Influence of smoking cues in movies on craving among smokers. Addiction 104 (12), 2102–2109. https://doi.org/10.1111/j.1360-0443.2009.02712.x (2009).
Upadhyaya, H. P., Drobes, D. J. & Thomas, S. E. Reactivity to smoking cues in adolescent cigarette smokers. Addict. Behav. 29 (5), 849–856. https://doi.org/10.1016/j.addbeh.2004.02.040 (2004).
Hines, D., Saris, R. N. & Throckmorton-Belzer, L. Cigarette smoking in popular films: Does it increase viewers’ likelihood to smoke?. J. Appl. Soc. Psychol. 30 (11), 2246–2269. https://doi.org/10.1111/j.1559-1816.2000.tb02435.x (2000).
Article Google Scholar
Bordnick, P. S., Graap, K. M., Copp, H. L., Brooks, J. & Ferrer, M. Virtual reality cue reactivity assessment in cigarette smokers. Cyberpsychol. Behav. 8 (5), 487–492. https://doi.org/10.1089/cpb.2005.8.487 (2005).
Sanchez-Vives, M. V. & Slater, M. From presence to consciousness through virtual reality. Nat. Rev. Neurosci. 6 (4), 332–339. https://doi.org/10.1038/nrn1651 (2005).
Traylor, A. C., Parrish, D. E., Copp, H. L. & Bordnick, P. S. Using virtual reality to investigate complex and contextual cue reactivity in nicotine dependent problem drinkers. Addict. Behav. 36 (11), 1068–1075. https://doi.org/10.1016/j.addbeh.2011.06.014 (2011).
Pericot-Valverde, I., Germeroth, L. J. & Tiffany, S. T. The use of virtual reality in the production of cue-specific craving for cigarettes: A meta-analysis. Nicotine Tob. Res. 18 (5), 538–546. https://doi.org/10.1093/ntr/ntv216 (2016).
Pericot-Valverde, I., Secades-Villa, R., Gutiérrez-Maldonado, J. & García-Rodríguez, O. Effects of systematic cue exposure through virtual reality on cigarette craving. Nicotine Tob. Res. 16 (11), 1470–1477. https://doi.org/10.1093/ntr/ntu104 (2014).
Article CAS PubMed PubMed Central Google Scholar
Zandonai, T. et al. A virtual reality study on postretrieval extinction of smoking memory reconsolidation in smokers. J. Subst. Abuse Treat. 125 , 108317. https://doi.org/10.1016/j.jsat.2021.108317 (2021).
Pericot-Valverde, I., Secades-Villa, R. & Gutiérrez-Maldonado, J. A randomized clinical trial of cue exposure treatment through virtual reality for smoking cessation. J. Subst. Abuse Treat. 96 , 26–32. https://doi.org/10.1016/j.jsat.2018.10.003 (2019).
Machulska, A. et al. Approach bias retraining through virtual reality in smokers willing to quit smoking: A randomized-controlled study. Behav. Res. Ther. 141 , 103858. https://doi.org/10.1016/j.brat.2021.103858 (2021).
Goldenhersch, E. et al. Virtual reality smartphone-based intervention for smoking cessation: Pilot randomized controlled trial on initial clinical efficacy and adherence. J. Med. Internet Res. 22 (7), e17571. https://doi.org/10.2196/17571 (2020).
Culbertson, C. S., Shulenberger, S., De La Garza, R., Newton, T. F. & Brody, A. L. Virtual reality cue exposure therapy for the treatment of tobacco dependence. J. Cyber Ther. Rehabil. 5 (1), 57–64 (2012).
PubMed PubMed Central Google Scholar
Jerome, L. W., Jordan, P. J., Rodericks, R. & Fedenczuk, L. Psychophysiological arousal and craving in smokers, deprived smokers, former smokers, and non-smokers. Stud. Health Technol. Inform. 144 , 179–183 (2009).
PubMed Google Scholar
LaRowe, S. D., Saladin, M. E., Carpenter, M. J. & Upadhyaya, H. P. Reactivity to nicotine cues over repeated cue reactivity sessions. Addict. Behav. 32 (12), 2888–2899. https://doi.org/10.1016/j.addbeh.2007.04.025 (2007).
Heishman, S., Singleton, E. & Pickworth, W. Reliability and validity of a short form of the tobacco craving questionnaire. Nicotine Tob. Res. 10 (4), 643–651. https://doi.org/10.1080/14622200801908174 (2008).
Heishman, S., Singleton, E. & Moolchan, E. Tobacco Craving Questionnaire: Reliability and validity of a new multifactorial instrument. Nicotine Tob. Res. 5 (5), 645–654. https://doi.org/10.1080/1462220031000158681 (2003).
Heller, G. Z., Manuguerra, M. & Chow, R. How to analyze the Visual Analogue Scale: Myths, truths and clinical relevance. Scand. J. Pain 13 (1), 67–75. https://doi.org/10.1016/j.sjpain.2016.06.012 (2016).
Wewers, M. E., Rachfal, C. & Ahijevych, K. A psychometric evaluation of a visual analogue scale of craving for cigarettes. West. J. Nurs. Res. 12 (5), 672–681 (1990).
Heatherton, T. F., Kozlowski, L. T., Frecker, R. C., Rickert, W. & Robinson, J. Measuring the Heaviness of Smoking: Using self-reported time to the first cigarette of the day and number of cigarettes smoked per day. Br. J. Addict. 84 (7), 791–800. https://doi.org/10.1111/j.1360-0443.1989.tb03059.x (1989).
Borland, R., Yong, H.-H., O’Connor, R. J., Hyland, A. & Thompson, M. E. The reliability and predictive validity of the Heaviness of Smoking Index and its two components: Findings from the International Tobacco Control Four Country study. Nicotine Tob. Res. 12 (Supplement 1), S45–S50. https://doi.org/10.1093/ntr/ntq038 (2010).
Bush, K. The AUDIT alcohol consumption questions (AUDIT-C): An effective brief screening test for problem drinking. Arch. Intern. Med. 158 (16), 1789. https://doi.org/10.1001/archinte.158.16.1789 (1998).
Khadjesari, Z. et al. Validation of the AUDIT-C in adults seeking help with their drinking online. Addict. Sci. Clin. Pract. 12 (1), 2. https://doi.org/10.1186/s13722-016-0066-5 (2017).
Freeman, D. et al. Automated VR therapy for improving positive self-beliefs and psychological well-being in young patients with psychosis: A proof of concept evaluation of Phoenix VR self-confidence therapy. Behav. Cogn. Psychother. https://doi.org/10.1017/S1352465823000553 (2023).
R Core Team. R: A Language and Environment for Statistical Computing (R Foundation for Statistical Computing, 2023). https://www.R-project.org/
Benedek, M. & Kaernbach, C. A continuous measure of phasic electrodermal activity. J. Neurosci. Methods 190 (1), 80–91. https://doi.org/10.1016/j.jneumeth.2010.04.028 (2010).
Horvers, A., Tombeng, N., Bosse, T., Lazonder, A. W. & Molenaar, I. Detecting emotions through electrodermal activity in learning contexts: A systematic review. Sensors 21 (23), 7869. https://doi.org/10.3390/s21237869 (2021).
Article ADS PubMed PubMed Central Google Scholar
Anderson, A. P. et al. Relaxation with immersive natural scenes presented using virtual reality. Aerosp. Med. Hum. Perform. 88 (6), 520–526. https://doi.org/10.3357/AMHP.4747.2017 (2017).
McCarthy, C., Pradhan, N., Redpath, C. & Adler, A. Validation of the Empatica E4 wristband. 2016 IEEE EMBS Int. Stud. Conf. Expand. Boundaries Biomed. Eng. Healthc. ISC 2016 – Proc. 4–7. https://doi.org/10.1109/EMBSISC.2016.7508621 (2016).
Schuurmans, A. A. T. et al. Validity of the empatica E4 wristband to measure heart rate variability (HRV) parameters: A comparison to electrocardiography (ECG). J. Med. Syst. 44 (11), 190. https://doi.org/10.1007/s10916-020-01648-w (2020).
Download references
Acknowledgements
This work was funded by a National Institute for Health and Care Research (NIHR) Senior Investigator award to DF (NIHR202385) and the NIHR Oxford Health Biomedical Research Centre (BRC). SL was supported by an NIHR Doctoral Fellowship (NIHR301483). The views expressed are those of the authors and not necessarily those of the NHS, the NIHR, or the Department of Health and Social Care. FW is funded by a Wellcome Trust Clinical Doctoral Fellowship (102176/B/13/Z).
Author information
Authors and affiliations.
Department of Experimental Psychology, University of Oxford, Oxford, UK
Aitor Rovira, Sinéad Lambe, Helen Beckwith, Memoona Ahmed, Felicity Hudson, Phoebe Haynes, Kira Williams, Simone Saidel, Ellen Iredale, Sapphira McBride, Felicity Waite & Daniel Freeman
Oxford Health NHS Foundation Trust, Oxford, UK
Aitor Rovira, Sinéad Lambe, Helen Beckwith, Felicity Waite & Daniel Freeman
Goldsmiths University, London, UK
Chun-Jou Yu & Xueni Pan
You can also search for this author in PubMed Google Scholar
Contributions
DF, SL, and AR conceived the study. DF, SL, AR, KW, SS, EI, SM conducted the experimental design. CYU programmed the VR experience. AR, XP supervised the software development. MA, FH, PH carried out the recruitment and the VR sessions. SL, HB, FW helped supervise the study. AR, DF performed the statistical analysis. AR, DF wrote the manuscript.
Corresponding author
Correspondence to Aitor Rovira .
Ethics declarations
Competing interests.
DF is the scientific founder of Oxford VR, a University of Oxford spin-out company. Oxford VR has not been involved in this study. The rest of the authors declare no competing interests.
Additional information
Publisher's note.
Springer Nature remains neutral with regard to jurisdictional claims in published maps and institutional affiliations.
Rights and permissions
Open Access This article is licensed under a Creative Commons Attribution 4.0 International License, which permits use, sharing, adaptation, distribution and reproduction in any medium or format, as long as you give appropriate credit to the original author(s) and the source, provide a link to the Creative Commons licence, and indicate if changes were made. The images or other third party material in this article are included in the article's Creative Commons licence, unless indicated otherwise in a credit line to the material. If material is not included in the article's Creative Commons licence and your intended use is not permitted by statutory regulation or exceeds the permitted use, you will need to obtain permission directly from the copyright holder. To view a copy of this licence, visit http://creativecommons.org/licenses/by/4.0/ .
Reprints and permissions
About this article
Cite this article.
Rovira, A., Lambe, S., Beckwith, H. et al. A randomized controlled experiment testing the use of virtual reality to trigger cigarette craving in people who smoke. Sci Rep 14 , 19445 (2024). https://doi.org/10.1038/s41598-024-70113-2
Download citation
Received : 19 April 2024
Accepted : 13 August 2024
Published : 21 August 2024
DOI : https://doi.org/10.1038/s41598-024-70113-2
Share this article
Anyone you share the following link with will be able to read this content:
Sorry, a shareable link is not currently available for this article.
Provided by the Springer Nature SharedIt content-sharing initiative
- Cigarette craving
- Virtual reality
By submitting a comment you agree to abide by our Terms and Community Guidelines . If you find something abusive or that does not comply with our terms or guidelines please flag it as inappropriate.
Quick links
- Explore articles by subject
- Guide to authors
- Editorial policies
Sign up for the Nature Briefing newsletter — what matters in science, free to your inbox daily.

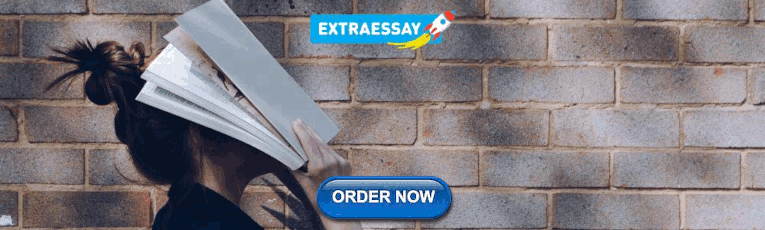
IMAGES
COMMENTS
In the statistical theory of design of experiments, randomization involves randomly allocating the experimental units across the treatment groups. For example, if an experiment compares a new drug against a standard drug, then the patients should be allocated to either the new drug or to the standard drug control using randomization.
A randomized experiment involves randomly splitting a group into smaller groups: one group (the treatment group) receives the intervention, and one does not (the control group ). The researcher selects the assignment mechanism, which is also random. Care is taken to choose a sampling method that results in an equal chance of each participant ...
Randomization is a statistical process in which a random mechanism is employed to select a sample from a population or assign subjects to different groups. [1] [2] [3] The process is crucial in ensuring the random allocation of experimental units or treatment protocols, thereby minimizing selection bias and enhancing the statistical validity. [4]It facilitates the objective comparison of ...
A randomized controlled trial (or randomized control trial; [ 2] RCT) is a form of scientific experiment used to control factors not under direct experimental control. Examples of RCTs are clinical trials that compare the effects of drugs, surgical techniques, medical devices, diagnostic procedures, diets or other medical treatments. [ 3][ 4]
The idea sounds so simple that defining it becomes almost a joke: randomisation is "putting participants into the treatment groups randomly". If only it were that simple. Randomisation can be a minefield, and not everyone understands what exactly it is or why they are doing it. A key feature of a randomised controlled trial is that it is ...
by Zach Bobbitt February 9, 2021. In the field of statistics, randomization refers to the act of randomly assigning subjects in a study to different treatment groups. For example, suppose researchers recruit 100 subjects to participate in a study in which they hope to understand whether or not two different pills have different effects on blood ...
A randomized control trial (RCT) is a type of study design that involves randomly assigning participants to either an experimental group or a control group to measure the effectiveness of an intervention or treatment. Randomized Controlled Trials (RCTs) are considered the "gold standard" in medical and health research due to their rigorous ...
A randomized controlled trial (RCT) is a prospective experimental design that randomly assigns participants to an experimental or control group. RCTs are the gold standard for establishing causal relationships and ruling out confounding variables and selection bias. Researchers must be able to control who receives the treatments and who are the ...
The strengths of RCTs are subtle: they are powerful because of the set of procedures that they are expected to follow. This includes the use of controls, placebos, experimentation, randomization, concealment, blinding, intention-to-treat analysis, and pre-registration.
Simple randomisation is a fair way of ensuring that any differences that occur between the treatment groups arise completely by chance. But - and this is the first but of many here - simple ran-domisation can lead to unbalanced groups, that is, groups of unequal size. This is particularly true if the trial is only small.
Meta-analysis of controlled trials shows that failure to conceal random allocation and the absence of double blinding yield exaggerated estimates of treatment effects. 1. Although randomised controlled trials are powerful tools, their use is limited by ethical and practical concerns. Exposing patients to an intervention believed to be inferior ...
In the contentious world of causal claims, randomized experiments represent an unbiased method for determining what works. Random assignment means participants are assigned to different groups or conditions in a study purely by chance. ... Ultimately, whether a study is considered a field experiment hinges on the definition of "the field ...
Definition. A study design that randomly assigns participants into an experimental group or a control group. As the study is conducted, the only expected difference between the control and experimental groups in a randomized controlled trial (RCT) is the outcome variable being studied. ... Pilates improves pain, function and quality of life in ...
A randomized controlled trial is a prospective, comparative, quantitative study/experiment performed under controlled conditions with random allocation of interventions to comparison groups. The randomized controlled trial is the most rigorous and robust research method of determining whether a cause-effect relation exists between an ...
Randomization in an experiment is where you choose your experimental participants randomly. For example, you might use simple random sampling, where participants names are drawn randomly from a pool where everyone has an even probability of being chosen. You can also assign treatments randomly to participants, by assigning random numbers from a ...
Randomized controlled trials (RCT) are prospective studies that measure the effectiveness of a new intervention or treatment. Although no study is likely on its own to prove causality, randomization reduces bias and provides a rigorous tool to examine cause-effect relationships between an intervention and outcome. This is because the act of ...
Random Experiments Definition. Random experiments can be defined as experiments that can be performed many times under the same conditions and their outcome cannot be predicted with complete certainty. In order words, in a random experiment, all the possible outcomes are known, however, its exact outcome cannot be precisely predicted in advance
About Randomized Field Experiments Randomized field experiments allow researchers to scientifically measure the impact of an intervention on a particular outcome of interest. ... What does random assignment mean? The key to randomized experimental research design is in the random assignment of study subjects - for example, individual voters ...
Examples of Random Experiments. Below are the examples of random experiments and the corresponding sample space. Tossing a coin three times. Number of possible outcomes = 8. Sample space = S = {HHH, HHT, HTH, THH, TTH, THT, HTT, TTT} Three coins are tossed simultaneously. Number of possible outcomes = 8.
In general, a randomized experiment is an essential tool for testing the efficacy of the treatment. In practice, randomization requires generating randomization schedules, which should be reproducible. Generation of a randomization schedule usually includes obtaining the random numbers and assigning random numbers to each subject or treatment ...
A Refresher on Randomized Controlled Experiments. In order to make smart decisions at work, we need data. Where that data comes from and how we analyze it depends on a lot of factors — for ...
Randomized experiments are the gold standard for ascertaining the efficacy of an intervention. In such experiments, the participants are randomly assigned into an experimental group (aka the treated group) or a control group. ... We use the following operational definition of a controlled experiment defined by Sjøberg et al. [47]: Table 1 ...
Randomization in an experiment refers to a random assignment of participants to the treatment in an experiment. OR, for instance we can say that randomization is assignment of treatment to the participants randomly. For example: a teacher decides to take a viva in the class and randomly starts asking the students.
The mean and standard deviation of the HRV used in the statistical analysis were also relative to the baseline values. ... A randomized controlled experiment testing the use of virtual reality to ...