Have a language expert improve your writing
Run a free plagiarism check in 10 minutes, generate accurate citations for free.
- Knowledge Base
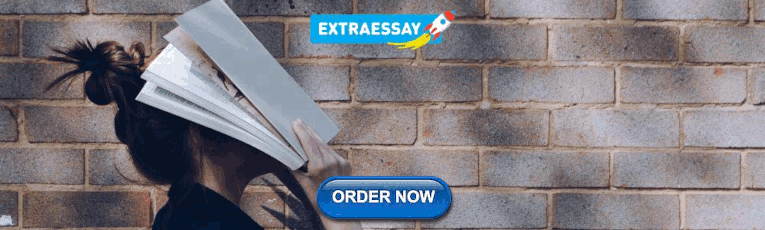
An Introduction to t Tests | Definitions, Formula and Examples
Published on January 31, 2020 by Rebecca Bevans . Revised on June 22, 2023.
A t test is a statistical test that is used to compare the means of two groups. It is often used in hypothesis testing to determine whether a process or treatment actually has an effect on the population of interest, or whether two groups are different from one another.
- The null hypothesis ( H 0 ) is that the true difference between these group means is zero.
- The alternate hypothesis ( H a ) is that the true difference is different from zero.
Table of contents
When to use a t test, what type of t test should i use, performing a t test, interpreting test results, presenting the results of a t test, other interesting articles, frequently asked questions about t tests.
A t test can only be used when comparing the means of two groups (a.k.a. pairwise comparison). If you want to compare more than two groups, or if you want to do multiple pairwise comparisons, use an ANOVA test or a post-hoc test.
The t test is a parametric test of difference, meaning that it makes the same assumptions about your data as other parametric tests. The t test assumes your data:
- are independent
- are (approximately) normally distributed
- have a similar amount of variance within each group being compared (a.k.a. homogeneity of variance)
If your data do not fit these assumptions, you can try a nonparametric alternative to the t test, such as the Wilcoxon Signed-Rank test for data with unequal variances .
Here's why students love Scribbr's proofreading services
Discover proofreading & editing
When choosing a t test, you will need to consider two things: whether the groups being compared come from a single population or two different populations, and whether you want to test the difference in a specific direction.
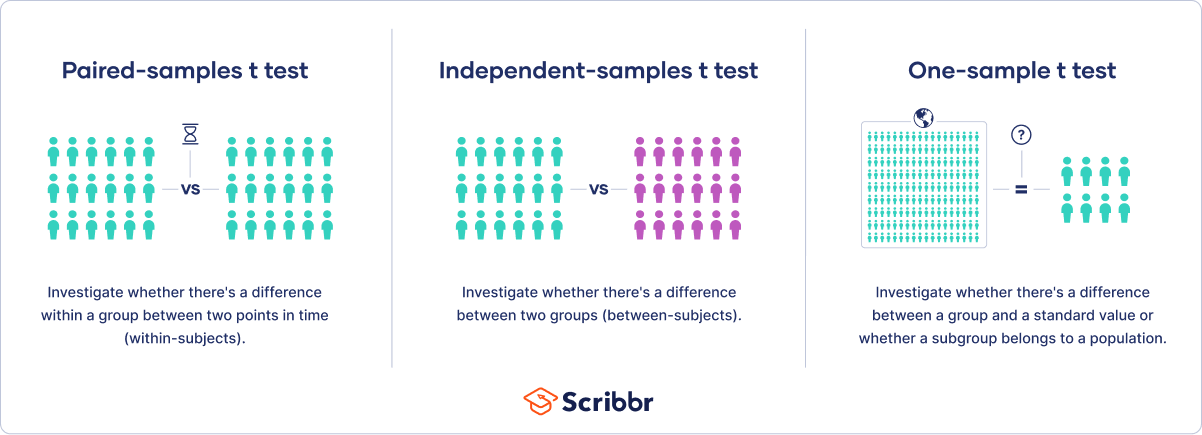
One-sample, two-sample, or paired t test?
- If the groups come from a single population (e.g., measuring before and after an experimental treatment), perform a paired t test . This is a within-subjects design .
- If the groups come from two different populations (e.g., two different species, or people from two separate cities), perform a two-sample t test (a.k.a. independent t test ). This is a between-subjects design .
- If there is one group being compared against a standard value (e.g., comparing the acidity of a liquid to a neutral pH of 7), perform a one-sample t test .
One-tailed or two-tailed t test?
- If you only care whether the two populations are different from one another, perform a two-tailed t test .
- If you want to know whether one population mean is greater than or less than the other, perform a one-tailed t test.
- Your observations come from two separate populations (separate species), so you perform a two-sample t test.
- You don’t care about the direction of the difference, only whether there is a difference, so you choose to use a two-tailed t test.
The t test estimates the true difference between two group means using the ratio of the difference in group means over the pooled standard error of both groups. You can calculate it manually using a formula, or use statistical analysis software.
T test formula
The formula for the two-sample t test (a.k.a. the Student’s t-test) is shown below.
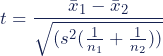
In this formula, t is the t value, x 1 and x 2 are the means of the two groups being compared, s 2 is the pooled standard error of the two groups, and n 1 and n 2 are the number of observations in each of the groups.
A larger t value shows that the difference between group means is greater than the pooled standard error, indicating a more significant difference between the groups.
You can compare your calculated t value against the values in a critical value chart (e.g., Student’s t table) to determine whether your t value is greater than what would be expected by chance. If so, you can reject the null hypothesis and conclude that the two groups are in fact different.
T test function in statistical software
Most statistical software (R, SPSS, etc.) includes a t test function. This built-in function will take your raw data and calculate the t value. It will then compare it to the critical value, and calculate a p -value . This way you can quickly see whether your groups are statistically different.
In your comparison of flower petal lengths, you decide to perform your t test using R. The code looks like this:
Download the data set to practice by yourself.
Sample data set
If you perform the t test for your flower hypothesis in R, you will receive the following output:
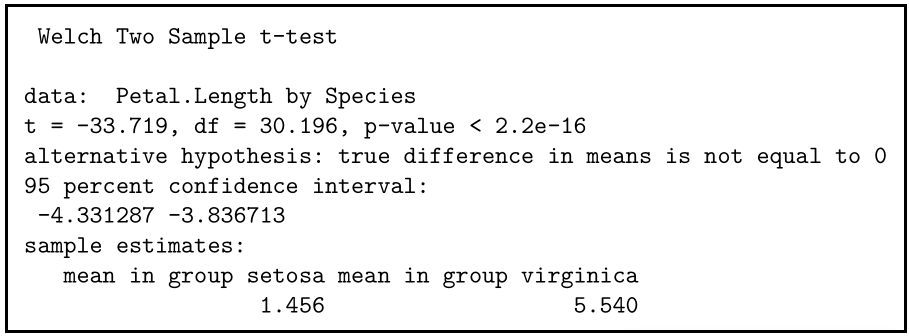
The output provides:
- An explanation of what is being compared, called data in the output table.
- The t value : -33.719. Note that it’s negative; this is fine! In most cases, we only care about the absolute value of the difference, or the distance from 0. It doesn’t matter which direction.
- The degrees of freedom : 30.196. Degrees of freedom is related to your sample size, and shows how many ‘free’ data points are available in your test for making comparisons. The greater the degrees of freedom, the better your statistical test will work.
- The p value : 2.2e-16 (i.e. 2.2 with 15 zeros in front). This describes the probability that you would see a t value as large as this one by chance.
- A statement of the alternative hypothesis ( H a ). In this test, the H a is that the difference is not 0.
- The 95% confidence interval . This is the range of numbers within which the true difference in means will be 95% of the time. This can be changed from 95% if you want a larger or smaller interval, but 95% is very commonly used.
- The mean petal length for each group.
Receive feedback on language, structure, and formatting
Professional editors proofread and edit your paper by focusing on:
- Academic style
- Vague sentences
- Style consistency
See an example
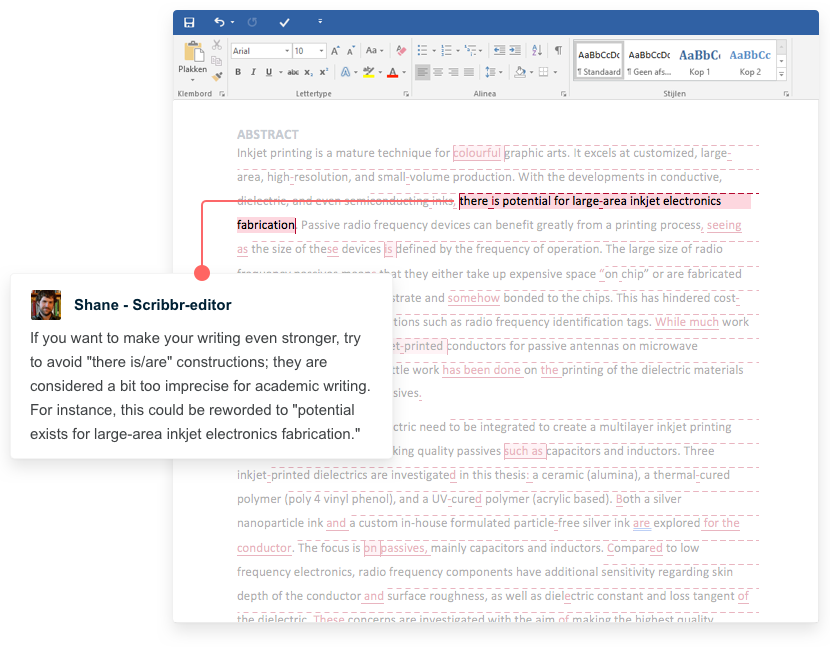
When reporting your t test results, the most important values to include are the t value , the p value , and the degrees of freedom for the test. These will communicate to your audience whether the difference between the two groups is statistically significant (a.k.a. that it is unlikely to have happened by chance).
You can also include the summary statistics for the groups being compared, namely the mean and standard deviation . In R, the code for calculating the mean and the standard deviation from the data looks like this:
flower.data %>% group_by(Species) %>% summarize(mean_length = mean(Petal.Length), sd_length = sd(Petal.Length))
In our example, you would report the results like this:
If you want to know more about statistics , methodology , or research bias , make sure to check out some of our other articles with explanations and examples.
- Chi square test of independence
- Statistical power
- Descriptive statistics
- Degrees of freedom
- Pearson correlation
- Null hypothesis
Methodology
- Double-blind study
- Case-control study
- Research ethics
- Data collection
- Hypothesis testing
- Structured interviews
Research bias
- Hawthorne effect
- Unconscious bias
- Recall bias
- Halo effect
- Self-serving bias
- Information bias
A t-test is a statistical test that compares the means of two samples . It is used in hypothesis testing , with a null hypothesis that the difference in group means is zero and an alternate hypothesis that the difference in group means is different from zero.
A t-test measures the difference in group means divided by the pooled standard error of the two group means.
In this way, it calculates a number (the t-value) illustrating the magnitude of the difference between the two group means being compared, and estimates the likelihood that this difference exists purely by chance (p-value).
Your choice of t-test depends on whether you are studying one group or two groups, and whether you care about the direction of the difference in group means.
If you are studying one group, use a paired t-test to compare the group mean over time or after an intervention, or use a one-sample t-test to compare the group mean to a standard value. If you are studying two groups, use a two-sample t-test .
If you want to know only whether a difference exists, use a two-tailed test . If you want to know if one group mean is greater or less than the other, use a left-tailed or right-tailed one-tailed test .
A one-sample t-test is used to compare a single population to a standard value (for example, to determine whether the average lifespan of a specific town is different from the country average).
A paired t-test is used to compare a single population before and after some experimental intervention or at two different points in time (for example, measuring student performance on a test before and after being taught the material).
A t-test should not be used to measure differences among more than two groups, because the error structure for a t-test will underestimate the actual error when many groups are being compared.
If you want to compare the means of several groups at once, it’s best to use another statistical test such as ANOVA or a post-hoc test.
Cite this Scribbr article
If you want to cite this source, you can copy and paste the citation or click the “Cite this Scribbr article” button to automatically add the citation to our free Citation Generator.
Bevans, R. (2023, June 22). An Introduction to t Tests | Definitions, Formula and Examples. Scribbr. Retrieved August 14, 2024, from https://www.scribbr.com/statistics/t-test/
Is this article helpful?
Rebecca Bevans
Other students also liked, choosing the right statistical test | types & examples, hypothesis testing | a step-by-step guide with easy examples, test statistics | definition, interpretation, and examples, what is your plagiarism score.
Independent Samples T-Test
Cite this chapter.
- Amanda Ross 3 &
- Victor L. Willson 4
2862 Accesses
21 Citations
6 Altmetric
An independent samples t-test compares the means of two groups. The data are interval for the groups. There is not an assumption of normal distribution (if the distribution of one or both groups is really unusual, the t-test will not give good results with unequal sample sizes), but there is an assumption that the two standard deviations are equal. If the sample sizes are equal or very similar in size, even that assumption is not critical.
This is a preview of subscription content, log in via an institution to check access.
Access this chapter
Subscribe and save.
- Get 10 units per month
- Download Article/Chapter or eBook
- 1 Unit = 1 Article or 1 Chapter
- Cancel anytime
- Available as PDF
- Read on any device
- Instant download
- Own it forever
Tax calculation will be finalised at checkout
Purchases are for personal use only
Institutional subscriptions
Unable to display preview. Download preview PDF.
Author information
Authors and affiliations.
A. A. Ross Consulting and Research, USA
Amanda Ross
Texas A&M University, USA
Victor L. Willson
You can also search for this author in PubMed Google Scholar
Rights and permissions
Reprints and permissions
Copyright information
© 2017 Sense Publishers
About this chapter
Ross, A., Willson, V.L. (2017). Independent Samples T-Test. In: Basic and Advanced Statistical Tests. SensePublishers, Rotterdam. https://doi.org/10.1007/978-94-6351-086-8_3
Download citation
DOI : https://doi.org/10.1007/978-94-6351-086-8_3
Publisher Name : SensePublishers, Rotterdam
Online ISBN : 978-94-6351-086-8
eBook Packages : Education Education (R0)
Share this chapter
Anyone you share the following link with will be able to read this content:
Sorry, a shareable link is not currently available for this article.
Provided by the Springer Nature SharedIt content-sharing initiative
- Publish with us
Policies and ethics
- Find a journal
- Track your research
Conduct and Interpret a One-Sample T-Test
What is the One-Sample T-Test?
The one-sample t-test is a member of the t-test family. All the tests in the t-test family compare differences in mean scores of continuous-level (interval or ratio), normally distributed data. Unlike the independent or dependent-sample t-tests, the one-sample t-test works with only one mean score. The one-sample t-test compares the mean of a single sample to a predetermined value to determine if the sample mean is significantly greater or less than that value.
The independent sample t-test compares the mean of one distinct group to the mean of another group. An example research question for an independent sample t-test would be, “ Do boys and girls differ in their SAT scores ?” The dependent sample t-test compares two matched scores or measurements (such as before vs. after). An example research question for a dependent sample t-test would be, “ Do pupils’ grades improve after they receive tutoring ?”
On the other hand, the one-sample t-test compares the mean score found in an observed sample to some predetermined or hypothetical value. Typically, the hypothetical value is the population mean or some other theoretically derived value.
Some possible applications of the one-sample t-test include testing a sample against a predetermined or expected value, testing a sample against a certain benchmark, or testing the results of a replicated experiment against the original study. For example, a researcher may want to determine if the average age of retiring in a certain population is 65. The researcher would draw a representative sample of people entering retirement and ask at what age they retired. A one-sample t-test could then be conducted to compare the mean age obtained in the sample (e.g., 63) to the hypothetical test value of 65. The t-test determines whether the difference we find in our sample is larger than we would expect to see by chance.
The One-Sample T-Test in SPSS
In this example, we will conduct a one-sample t-test to determine if the average age of a population of students is significantly greater or less than 9.5 years.

Need help with your analysis?
Schedule a time to speak with an expert using the calendar below.
User-friendly Software
Transform raw data into written, interpreted, APA formatted t-test results in seconds.
Before we actually conduct the one-sample t-test, our first step is to check the distribution for normality. This may be done with a Q-Q Plot (located under Analyze > Descriptive Statistics in SPSS). Then we simply add the variable we want to test (age) to the box and confirm that the test distribution is set to Normal . This will create the diagram you see below. The output shows that small values and large values somewhat deviate from normality. As an additional check, we can run a Kolmogorov-Smirnov (K-S) test to test the null hypothesis that the variable is normally distributed. We find here that the K-S test is not significant; thus, we cannot reject the null hypothesis and may assume that age is normally distributed.
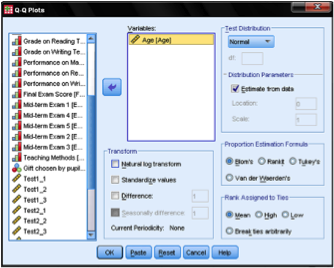
Let’s move on to the one-sample t-test, which can be found in Analyze > Compare Means > One-Sample T Test …
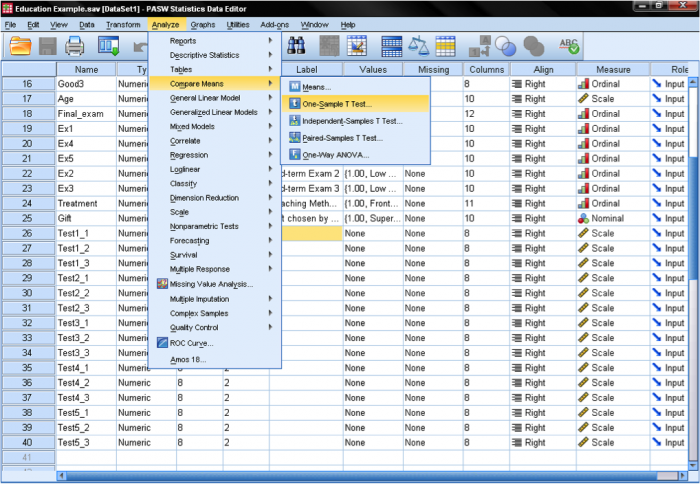
The one-sample t-test dialog box is fairly simple. We add the test variable age to the list of Test Variables and then enter the Test Value . In our case, the hypothetical test value is 9.5. The dialog Options… gives us the setting for how to manage missing values and also the opportunity to specify the width of the confidence interval used for testing.
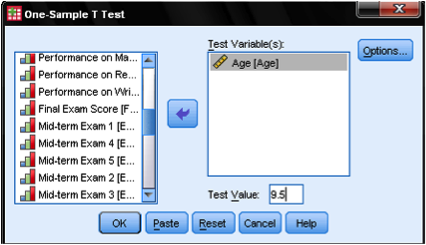
Once all of the appropriate options are set, click OK to run the analysis. The figure below shows the output. The “One-Sample Statistics” section shows descriptive statistics for the sample, including the mean being compared to the test value. The “One-Sample Test” section shows the results of the t-test. In this case, the null hypothesis is that the mean of the sample is equal to 9.5. For the purpose of this example, we will set our significance (alpha) level to .05. The Sig. column displays the p-value for the test. The results show that the p-value (.592) is greater than .05. This suggests that the null hypothesis cannot be rejected, and the age of the sample is not significantly different from 9.5.
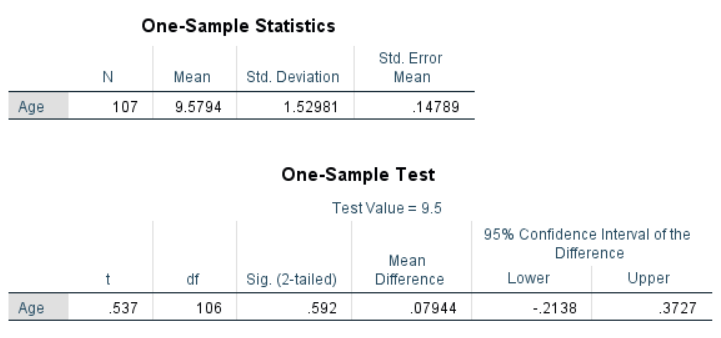
Academia.edu no longer supports Internet Explorer.
To browse Academia.edu and the wider internet faster and more securely, please take a few seconds to upgrade your browser .
Enter the email address you signed up with and we'll email you a reset link.
- We're Hiring!
- Help Center
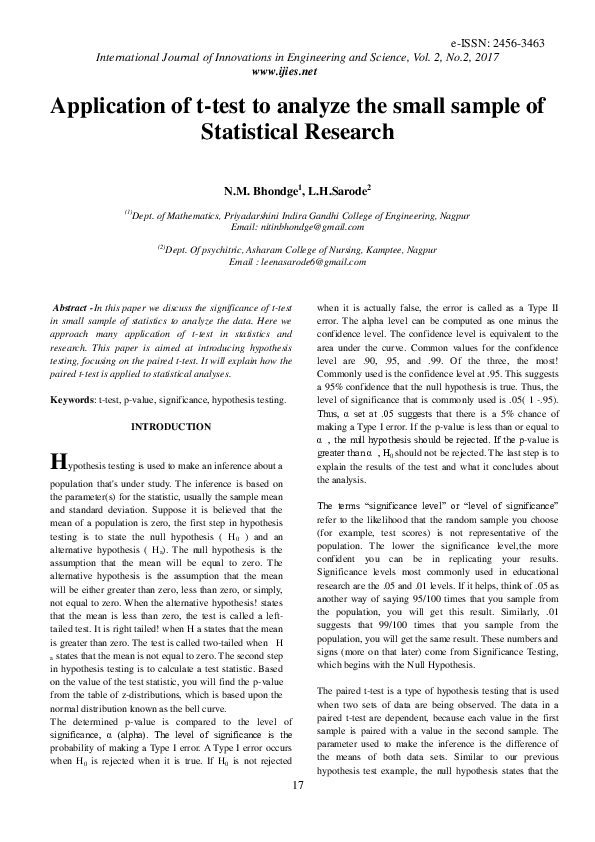
Application of t-test to analyze the small sample of Statistical Research

In this paper we discuss the significance of t-test in small sample of statistics to analyze the data. Here we approach many application of t-test in statistics and research. This paper is aimed at introducing hypothesis testing, focusing on the paired t-test. It will explain how the paired t-test is applied to statistical analyses.
Related Papers
Independent Postgraduate Studies Research Centered on Educational Administration with Research, Training and Development Emphasis.
Shane J Charbonnét, Ph.D.: HCD & UXDE
Preliminary Chapter(s) -- One and Two: T-Test examines the difference(s) between variables with a set (Wrench, Thomas-Maddox, Richmond, and McCroskey, 2013; 2008). The two basic types include the Independent Samples t-Test and the Paired t-Test. This paper interprets derived PSPP results –data set 1 – resulting in an Analysis-Compare Means-Independent Samples t-Test and data set 2 – resulting in an Analysis-Compare Means-Paired Sample t-Test. It’s a 3-page report identifying the significant difference between the means of males/females and time1 / time2 on self-esteem. Independent Sample t-Test examines “one nominal variable with two categories (two independent groups) and their scores on one dependent interval/ratio variable” (Wrench, Thomas-Maddox, Richmond, and Wrench, 2013; 2008, p. 372). Data Set 1 espouses 10 total (gender specified – independent variable; self-esteem score identified – dependent variable), research participants. Each person categorized according to his / her observed self-esteem score-rating post-2-minute inspirational speech. The survey classes include male-gender with a coded numerical value of 1 and female-gender with a coded numerical value of 2. The nominal variable is the independent variable and the interval/ratio variable is the dependent variable. The central objective of the test is to determine if one group does/does not influence the other variable’s placement with the group(s). Applicable to the PSPP-results, group one assesses Group Statistics. The sample (N=5) for Var0001 and Var0002, is 5 (individually) and 10 (collectively). Var0001 mean = 56.80 and Var0002 mean = 52.00. The standard deviation (square root of the variance for Var0001) is 3.12. The standard deviation (√s2 (variance) = 3.16.
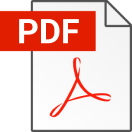
Advances and Applications in Statistics
Mowafaq Al-kassab
JOURNAL OF ADVANCES IN MATHEMATICS
The t-statistic is the ratio of the departure of the estimated value of a parameter from its hypothesized value to its standard error. The term "t-statistic" is abbreviated from "hypothesis test statistic". It was first derived as a posterior distribution in 1876 by Helmertand Lüroth. The purpose of this research is to study the t-test, especially the one sample t-test to determine if the sample data come from the same population. The grade points average (GPA) of the students for the second, third, and fourth grades of the Department of Mathematics Education, Tishk International University are used. The one sample t test is used to predict the GPA of the students for the second, third, and the fourth grades respectively, in addition to the overall average scores for the three grades. The 95% confidence interval for the true population average is also conducted.
K Y PUBLICATION
Ramnath Takiar
The present study was carefully designed to evaluate the performance of ttest as compared to Z-test in testing the significant or non-significant differences between two sample means. The sources of data for the study came by generating four Normal populations (Population A, B, C and D) and then drawing 30 samples each form those populations. Overall, the study covers 14400 comparisons to test for significant differences and 18240 comparisons for non-significant differences between means. It is surprising to note that at = 5%, t-test was able to pick up only 29.3% of the expected significant differences between the sample means of Population C and D, which is quite low. In case of Population A and B, the validity of the test was observed to be relatively better and it was 49.6%. In view of low validity observed in the case of = 5%, the validity was further explored at the higher levels of namely 10%, 15% and 20%. With the rise in levels, the validity was observed to be increasing. For the Population C and D, at = 20% , the validity of t-test rose to 54.3% and for the Population A and B, the validity rose to 76.1%. This suggests that for testing the significant or non-significant differences between the means, especially for small samples, the level can be raised from 5% to 20% so that more valid mean comparisons by t-test can be obtained. In view of Z-test performing better as compared to t-test in picking up the significant differences, correctly, and not lagging behind much in picking up
jorge ponce
(HCM) Võ Thái Thu Hà
Peter Samuels
Paired Samples t-test statstutor worksheet. Available at: http://www.statstutor.ac.uk/resources/uploaded/pairedsamplesttest3.pdf.
Pacing and Clinical Electrophysiology
Marek Malik
Psychological Science
Rand Wilcox
Dr. Mikael Chuaungo
A t-test is a statistic that checks if two means (averages) are reliably different from each other. Looking at the means may tell us there is a difference, but it doesn’t tell us if it’s reliable. Comparing means don’t tell if there is a reliable difference. For eg. If person A and person B flip a coin 100 times. Person A gets heads 52 times and person B gets heads 49 times. This does not tell us that Person A gets reliably more Heads than B, or whether he will get more heads than B if he flipped the coins 100 times again. There is no difference, its just chance.
Loading Preview
Sorry, preview is currently unavailable. You can download the paper by clicking the button above.
RELATED PAPERS
ForsChem Research Reports
Hugo Hernandez
Philips Ido
Edward Volchok
Dr. Edwin A . B . Juma
Akeyede Imam
Critical Care
Jonathan Ball
Engr. Dr. Muhammad Mujtaba Asad
Journal of Modern Applied Statistical Methods
Critical care (London, England)
Journal of Surgical Education
Todd Neideen
Eleazar hernandez vasquez
Joginder Kaur
… bulletin & review
Natalie Obrecht
Journal of emerging technologies and innovative research
Devendra pathak pathak
Sonja Eisenbeiss
Vanderley Borges
IOSR Journals publish within 3 days
British Journal of Mathematical and Statistical Psychology
Larry Toothaker
Handan Ankaralı
Sampark Acharya
BOHR Publishers
BOHR International Journal of Operations Management Research and Practices (BIJOMRP)
Annals of Cardiac Anaesthesia
Prabhaker Mishra
Statistical significance and other complementary measures for the interpretation of the research results
Mildrey Torres
- We're Hiring!
- Help Center
- Find new research papers in:
- Health Sciences
- Earth Sciences
- Cognitive Science
- Mathematics
- Computer Science
- Academia ©2024
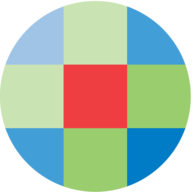
- Get new issue alerts Get alerts
- IARS MEMBER LOGIN
Secondary Logo
Journal logo.
Colleague's E-mail is Invalid
Your message has been successfully sent to your colleague.
Save my selection
Two-Sample Unpaired t Tests in Medical Research
Schober, Patrick MD, PhD, MMedStat * ; Vetter, Thomas R. MD, MPH †
From the * Department of Anesthesiology, Amsterdam UMC, Vrije Universiteit Amsterdam, Amsterdam, the Netherlands
† Department of Surgery and Perioperative Care, Dell Medical School at the University of Texas at Austin, Austin, Texas.
Address correspondence to Patrick Schober, MD, PhD, MMedStat, Department of Anesthesiology, Amsterdam UMC, Vrije Universiteit Amsterdam, De Boelelaan 1117, 1081 HV Amsterdam, the Netherlands. Address e-mail to [email protected] .
A 2-sample t test is commonly used to analyze continuous data, but valid statistical inferences rely on its test assumptions being met.
In this issue of Anesthesia & Analgesia , Wong et al 1 report a randomized trial of the effects of high-flow nasal oxygenation on safe apnea time (oxygen saturation measured by pulse oximetry [Sp o 2 ], >95%) during anesthetic induction of morbidly obese patients. The authors used a 2-sample, unpaired t test and observed a significantly prolonged safe apnea time in the intervention group (Figure).
The 2-sample t test is commonly used to compare 2 independent groups and tests the null hypothesis that the means are equal. 2 Its test statistic can be thought of as a “signal-to-noise” ratio 3 : the ratio of the mean difference between the groups (the “signal”) to a function of the within-group variability (the “noise”). A large mean difference relative to the variability corresponds to a small P value, suggesting that there actually is a difference between the groups.

Valid inferences with a 2-sample t test rely on several assumptions being met, 3 including:
- The dependent variable is continuous.
- Observations are independent of each other.
- The data are approximately normally distributed in each group.
- The variances are approximately equal in both groups.
Assumptions #3 and #4 apply to the population from which the data were sampled, not the sample itself. Hypothesis tests are available to test these assumptions (eg, Shapiro-Wilk test as used by Wong et al 1 for #3 and Levene test for #4) but have low power to detect violations at small sample sizes. These tests should be used in combination with graphical methods (eg, Q-Q plots) and a comparison of the group standard deviations to determine whether the assumptions are met. 4
The t test is relatively robust against nonnormality with larger sample sizes. Conventionally, the sample size needs to be ≥30; however, a much larger sample may be necessary if the data distribution is severely skewed. Importantly, smaller samples definitely do rely on a normal distribution to avoid erroneous conclusions. Nonparametric methods (eg, Mann-Whitney U tests) can be used if this normality assumption is violated.
The t test is also relatively robust against unequal variances if the sample sizes per group are equal and if the sample is large enough (>15 per group). Alternatives like the Welch t test are available if variances are unequal.
Beside inappropriately using t tests when assumptions are (grossly) violated, a common mistake is to use multiple pairwise t tests to compare >2 groups. Other techniques are instead needed, like analysis of variance (ANOVA) with appropriate post hoc tests. 2
While the 2-sample t test is basically a hypothesis test that gives a P value, statistical software packages routinely report the mean difference between the groups including its confidence interval. As appropriately done by Wong et al, 1 we strongly encourage authors to report this estimate because it provides important information about the magnitude of the treatment effect. 5
- Cited Here |
- Google Scholar
- + Favorites
- View in Gallery
Readers Of this Article Also Read
Sample size and power in clinical research, postoperative pain after inguinal herniorrhaphy with different types of..., fatty acid lessens halothane's inhibition of energy metabolism in isolated..., pharmacodynamics and pharmacokinetics of epidural ropivacaine in humans, differences in magnitude and duration of opioid-induced respiratory depression....
Information
- Author Services
Initiatives
You are accessing a machine-readable page. In order to be human-readable, please install an RSS reader.
All articles published by MDPI are made immediately available worldwide under an open access license. No special permission is required to reuse all or part of the article published by MDPI, including figures and tables. For articles published under an open access Creative Common CC BY license, any part of the article may be reused without permission provided that the original article is clearly cited. For more information, please refer to https://www.mdpi.com/openaccess .
Feature papers represent the most advanced research with significant potential for high impact in the field. A Feature Paper should be a substantial original Article that involves several techniques or approaches, provides an outlook for future research directions and describes possible research applications.
Feature papers are submitted upon individual invitation or recommendation by the scientific editors and must receive positive feedback from the reviewers.
Editor’s Choice articles are based on recommendations by the scientific editors of MDPI journals from around the world. Editors select a small number of articles recently published in the journal that they believe will be particularly interesting to readers, or important in the respective research area. The aim is to provide a snapshot of some of the most exciting work published in the various research areas of the journal.
Original Submission Date Received: .
- Active Journals
- Find a Journal
- Proceedings Series
- For Authors
- For Reviewers
- For Editors
- For Librarians
- For Publishers
- For Societies
- For Conference Organizers
- Open Access Policy
- Institutional Open Access Program
- Special Issues Guidelines
- Editorial Process
- Research and Publication Ethics
- Article Processing Charges
- Testimonials
- Preprints.org
- SciProfiles
- Encyclopedia
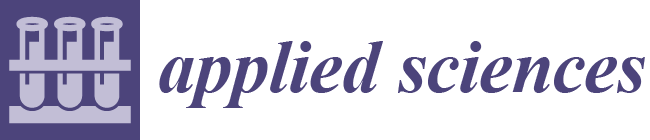
Article Menu
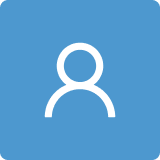
- Subscribe SciFeed
- Recommended Articles
- Author Biographies
- Google Scholar
- on Google Scholar
- Table of Contents
Find support for a specific problem in the support section of our website.
Please let us know what you think of our products and services.
Visit our dedicated information section to learn more about MDPI.
JSmol Viewer
Research on children’s body proportions: determining the canon of head length to total body height on the example of children aged 2 to 15 years.

1. Introduction
1.1. previous studies of the canons and proportions of the human body, 1.2. the aim of the study.
- The canons between 5 HL and 7.5 HL for children of kindergarten and primary school age obtained throughout history are still valid today.
- It is possible to establish a unique canon for children of kindergarten and primary school age (2 to 15 years), which would be used as an aid in the design of various products for children.
- It is possible to determine the grounds for creating an anthropological–biomechanical model, which can be used to determine other anthropometric variables.
2. Materials and Methods
2.1. cities/countries, 2.2. participants, 2.2.1. preschool children, 2.2.2. school children, 2.3. anthropometric variables measured.
- The Altmeter was used for body height measurements, dimensions from 0 to 2000 mm [ 30 ];
- The Calliper was used for the head length measurements [ 31 ] (the shubler mehanická) 16090014//—from 0 to 600 mm, with calibration sheet “č. P540/16”, with an error margin of ±0.02 mm.
2.4. Ethical Approval and Permission for Conducting the Research
2.5. statistical methods, 4. discussion.
- The existing data established in research [ 5 , 6 , 21 , 24 ] on the canon (ratio) of children’s head length (HL) to total body height (BH) coincide with the results of our research, which range from 5.59 and 5.72 (2-year-old girls and boys) to 7.50 and 7.60 (15-year-old boys and girls), depending on age and gender.
- Compared to existing data from the literature ( Table 6 ), the canon has increased with regard to the observed age of children, which confirms the secular trends of the past 100 years.
- Observing the growth and development of a child’s body in relation to an adult, it is obvious that the corresponding canons change faster with age.
- Although the canons for boys and the girls of the same age are similar, they must be observed separately.
- Studies should consider not only the age and gender, but also the origin, nationality, and other sociodemographic parameters of the child in future research.
5. Conclusions
Author contributions, institutional review board statement, informed consent statement, data availability statement, acknowledgments, conflicts of interest.
- Eco, U. The History of Beauty , 2nd ed.; HENA com: Zagreb, Croatia, 2004. (In Croatian) [ Google Scholar ]
- Lomas, J.D.; Xue, H. Harmony in Design: A Synthesis of Literature from Classical Philosophy, the Sciences, Economics, and Design. She Ji J. Des. Econ. Innov. 2022 , 8 , 5–64. [ Google Scholar ] [ CrossRef ]
- Veljović, F. An Introduction to the Study of Harmony , 1st ed.; University of Sarajevo, Faculty of Mechanical Engineering: Sarajevo, Bosnia and Herzegovina, 2007; (In Croatian). Available online: https://olx.ba/artikal/26207901/fikret-veljovic-uvod-u-istrazivanje-harmonije/ (accessed on 18 February 2024).
- Canon. Available online: https://www.dictionary.com/browse/canon (accessed on 24 July 2024).
- Barcsay, J. Anatomy for Artists, 2nd ed ; NIŠRO Forum, Izdavačka delatnost Novi Sad i Jugoslavenska knjiga: Beograd, Yugoslavia, 1989. (In Croatian) [ Google Scholar ]
- Muftić, O.; Veljović, F.; Jurčević Lulić, T.; Miličić, D. Basics of Ergonomics , 1st ed.; University of Sarajevo, Faculty of Mechanical Engineering: Sarajevo, Bosnia and Herzegovina, 2001. (In Croatian) [ Google Scholar ]
- Muftić, O.; Jurčević Lulić, T.; Godan, B. Harmonic distribution of the segmental masses of the human body. Sigurnost 2011 , 53 , 1–10. Available online: https://hrcak.srce.hr/67033 (accessed on 10 February 2024).
- McCormick, E.J.; Sanders, M.S. Human Factors in Engineering and Design, 5th ed ; McGraw-Hill International Students Education: New York, NY, USA, 1982. [ Google Scholar ]
- Grandjean, E. Fitting the Task to the Man: A Textbook of Occupational Ergonomics , 4th ed.; Taylor & Francis: Abingdon, UK, 1988. [ Google Scholar ]
- Kroemer, K.H.E.; Kroemer, H.B.; Kroemer-Elbert, K.E. Ergonomics: How to Design for Ease and Efficiency , 2nd ed.; Prentice Hall, Inc.: Saddle River, NJ, USA, 2003. [ Google Scholar ]
- Domljan, D. The Design of Contemporary School Furniture as a Prerequisite for Maintenance of Pupils’ Health. Ph.D. Thesis, University of Zagreb Faculty of Forestry, Zagreb, Croatia, 18 May 2011. (In Croatian). [ Google Scholar ]
- Iliev, B. Furniture Design in Facilities for Preschool Education as a Basis for Healthy Children’s Growth and Development. Ph.D. Thesis, University of Zagreb, Faculty of Forestry and Wood Technology, Zagreb, Croatia, 8 July 2021. [ Google Scholar ]
- Iliev, B.; Domljan, D.; Vlaović, Z. Importance of Anthropometric Data in Design of Preschool Furniture. In Proceedings of the 7th International Ergonomics Conference ERGONOMICS 2018—Emphasis on Wellbeing, Zadar, Croatia, 13–16 June 2018; Sumpor, D., Salopek Čubrić, I., Jurčević Lulić, T., Čubrić, G., Eds.; Croatian Ergonomics Society: Zagreb, Croatia, 2018; pp. 141–148. [ Google Scholar ]
- Peebes, L. Adultdata: The Handbook of Adult Anthropometric and Strength Measurements: Data for Design Safety ; Government Consumer Safety Research, Department of Trade and Industry: London, UK, 1998. [ Google Scholar ]
- Panero, J.; Zelnik, M. Human Dimension & Interior Space: A Source Book of Design Reference Standards , Revised ed.; Clarkson Potter/Ten Speed: London, UK, 2014. [ Google Scholar ]
- Norris, B.; Wilson, J.R. Childata: The Handbook of Child Measurements and Capabilities ; Data for Design Safety, Department of Trade and Industry: London, UK, 1995. [ Google Scholar ]
- Lueder, R.; Berg Rice, V.J. Ergonomics for Children , 1st ed.; CRC Press: Boca Raton, FL, USA, 2007. [ Google Scholar ] [ CrossRef ]
- Robins, G. Proportion and Style in Ancient Egyptian Art ; Publisher University of Texas Press: Austin, TX, USA, 1994. [ Google Scholar ] [ CrossRef ]
- Waldstein, C. Praxiteles and the Hermes with the Dionysos-Child from the Heraion in Olympia ; Reprinted from the Trans. Roy. Soc. of Literature, Vol xii, Part 2, 1880; Harison and Sons: St. Martins lane, PA, USA; Library of Columbia University: New York, NY, USA, 1879; Available online: https://www.columbia.edu/cu/lweb/digital/collections/cul/texts/ldpd_9097300_000/ldpd_9097300_000.pdf (accessed on 24 July 2024).
- Vitruvius. The Ten Books on Architecture ; Translated by Morgan M.H.; Harvard University Press: Cambridge, MA, USA; Oxford University Press: Cambridge, UK, 1914; Available online: https://www.chenarch.com/images/arch-texts/0000-Vitruvius-50BC-Ten-Books-of-Architecture.pdf (accessed on 5 February 2024).
- Doczi, G. The Power of Limits: Proportional Harmonies in Nature, Art and Architecture ; Shambhala Publications, Inc.: Boston, MA, USA, 1981. [ Google Scholar ]
- Da Vinci, L. Treatise on Painting , 2nd ed.; Nestorović, M., Ed.; Knjižarsko Preduzeće Bata, BIZG.: Beograd, Yugoslavia, 1990. (In Croatian) [ Google Scholar ]
- De Thorne, M. Albrecht Dürer’s Human Proportions ; UT Health Library: San Antonio, TX, USA, 2012; Available online: https://library.uthscsa.edu/2012/03/albrecht-durers-human-proportions (accessed on 16 February 2024).
- Richer, P.M.L.P. Artistic Anatomy: The Great Classic on Artistic Anatomy , Reprint 1st ed.; Hale, R.B., Ed.; Watson-Guptill Publications: New York, NY, USA, 1889. [ Google Scholar ]
- Muftić, O. Biomechanical Ergonomic , 1st ed.; University of Zagreb Faculty of Mechanical Engineering and Naval Architecture: Zagreb, Croatia, 2005. (In Croatian) [ Google Scholar ]
- Muftić, O. Harmonical Anthropometry as the Base for Applied Dynamic Anthropometry. In Proceedings of the Meeting on Construction, Faculty of Mechanical Engineering and Naval Architecture, Zagreb, Croatia, 25 July 1984. [ Google Scholar ]
- Prebeg, Ž.; Prebeg, Ž. Hygiene and School , 5th ed.; Školska knjiga: Zagreb, Croatia, 1985. (In Croatian) [ Google Scholar ]
- Ministry of Labor and Social Policy of the Republic of Macedonia. Law on the Protection of Children ; Official Gazette of the Republic of Macedonia 23/2013: Skopje, North Macedonia, 2013. [ Google Scholar ]
- NIH (National Institutes of Health): 5.4. Anthropometry 2.3. Frankfort Plane. Available online: https://www.ncbi.nlm.nih.gov/projects/gap/cgi-bin/GetPdf.cgi?id=phd001792.2 (accessed on 21 January 2024).
- The Altmeter, Producer SPECIJALNA OPREMA—Lučko d.o.o. Available online: https://specijalna-oprema.hr/visinomjer (accessed on 24 July 2024).
- The Caliper, Producer KIMTEX s.r.o. Available online: https://kmitex.cz/produkt/hloubkomer-bez-nosu-csn-25-1280-din-862-4/?_gl=1%2Adhgizv%2A_up%2AMQ..&gclid=CjwKCAjwzIK1BhAuEiwAHQmU3hVeCoKEaNgWQ2Eea1nu6cFkQZhcopctIj5jsEoMtU6VpZE0jMERHhoCgYQQAvD_BwE (accessed on 24 July 2024).
- UNIZG. Code of Ethics University of Zagreb (UNIZG); Zagreb, Croatia, 2007. Available online: http://www.unizg.hr/fileadmin/rektorat/O_Sveucilistu/Dokumenti_javnost/Propisi/Pravilnici/Eticki_kodeks.pdf (accessed on 21 January 2024).
- CEN TC 122. Technical Report on the Demands and Availability of Anthropometric Data of Children in Europe, EU Project Anthropometric Data on Children in Europe, CEN TC; 122 Ergonomics; DIN Standards Committee Ergonomics CEN/TC 122/WG1/TG1N128, 2020. Available online: https://www.din.de/resource/blob/705730/a798019fbd0c7060fff9e14a76c125be/summary-of-project-phase-1-on-demands-and-availability-of-anthropometric-data.pdf (accessed on 31 August 2023).
- Megersa, B.S.; Zinab, B.; Ali, R.; Kedir, E.; Girma, T.; Berhane, M.; Admassu, B.; Friis, H.; Abera, M.; Olsen, M.F.; et al. Associations of weight and body composition at birth with body composition and cardiometabolic markers in children aged 10 y: The Ethiopian infant anthropometry and body composition birth cohort study. Am. J. Clin. Nutr. 2023 , 118 , 412–421. [ Google Scholar ] [ CrossRef ] [ PubMed ]
- Holland, R.; Bowerman, C.; Stanojevic, S. The Contribution of Anthropometry and Socioeconomic Status to Racial Differences in Measures of Lung Function. CHEST 2022 , 162 , 635–646. [ Google Scholar ] [ CrossRef ] [ PubMed ]
- Nieczuja-Dwojacka, J.; Borowska, B.; Budnik, A.; Marchewka-Długońska, J.; Tabak, I.; Popielarz, K. The Influence of Socioeconomic Factors on the Body Characteristics, Proportion, and Health Behavior of Children Aged 6–12 Years. Int. J. Environ. Res. Public Health 2023 , 20 , 3303. [ Google Scholar ] [ CrossRef ] [ PubMed ]
- Bogin, B.; Varela-Silva, M.I. Leg Length, Body Proportion, and Health: A Review with a Note on Beauty. Int. J. Environ. Res. Public Health 2010 , 7 , 1047–1075. [ Google Scholar ] [ CrossRef ] [ PubMed ]
- Leitch, I. Growth and Health. Br. J. Nutr. 1951 , 5 , 142–151. [ Google Scholar ] [ CrossRef ] [ PubMed ]
- Lu, C.W.; Lu, J.M. Evaluation of the Indonesian National Standard for elementary school furniture based on children’s anthropometry. Appl. Ergon. 2017 , 62 , 168–181. [ Google Scholar ] [ CrossRef ]
- Widyanti, A.; Mahachandra, M.; Soetisna, H.R.; Sutalaksana, I.Z. Anthropometry of Indonesian Sundanese children and the development of clothing size system for Indonesian Sundanese children aged 6–10 year. Int. J. Ind. Ergon. 2017 , 61 , 37–46. [ Google Scholar ] [ CrossRef ]
- Bernstein, S.A.; Lo, M.; Davis, W.S. Proposing Using Waist-to-Height Ratio as the Initial Metric for Body Fat Assessment Standards in the U.S. Army. Mil. Med. 2017 , 182 , 304–309. [ Google Scholar ] [ CrossRef ] [ PubMed ]
- López-Gil, J.F.; García-Hermoso, A.; Sotos-Prieto, M.; Cavero-Redondo, I.; Martínez-Vizcaíno, V.; Kales, S.N. Mediterranean Diet-Based Interventions to Improve Anthropometric and Obesity Indicators in Children and Adolescents: A Systematic Review with Meta-Analysis of Randomized Controlled Trials. Adv. Nutr. 2023 , 14 , 858–869. [ Google Scholar ] [ CrossRef ] [ PubMed ]
- Santoso, M.V.; Kerr, R.N.B.; Kassim, N.; Martin, H.; Mtinda, E.; Njau, P.; Mtei, K.; Hoddinott, J.; Young, S.L. A Nutrition-Sensitive Agroecology Intervention in Rural Tanzania Increases Children’s Dietary Diversity and Household Food Security but does not change Child Anthropometry: Results from a Cluster-Randomized Trial. J. Nutr. 2021 , 151 , 2010–2021. [ Google Scholar ] [ CrossRef ]
- Zhang, Y.-Q.; Li, H. Reference Charts of Sitting Height, Leg Length and Body Proportions for Chinese Children Aged 0–18 Years. Ann. Hum. Biol. 2015 , 42 , 225–232. [ Google Scholar ] [ CrossRef ]
- Gleiss, A.; Lassi, M.; Blümel, P.; Borkenstein, M.; Kapelari, K.; Mayer, M.; Häusler, G. Austrian height and body proportion references for children aged 4 to under 19 years. Ann. Hum. Biol. 2013 , 40 , 324–332. [ Google Scholar ] [ CrossRef ] [ PubMed ]
- Cheng, J.; Leung, S.; Lau, J. Anthropometric Measurements and Body Proportions Among Chinese Children. Clin. Orthop. Relat. Res. 1996 , 323 , 22–30. [ Google Scholar ] [ CrossRef ] [ PubMed ]
- Hattori, K.; Hirohara, T.; Satake, T. Body proportion chart for evaluating changes in stature, sitting height and leg length in children and adolescents. Ann. Hum. Biol. 2011 , 38 , 556–560. [ Google Scholar ] [ CrossRef ] [ PubMed ]
- Loeffler-Wirth, H.; Vogel, M.; Kirsten, T.; Glock, F.; Poulain, T.; Körner, A.; Loeffler, M.; Kiess, W.; Binder, H. Longitudinal anthropometry of children and adolescents using 3D-body scanning. PLoS ONE 2018 , 13 , e0203628. [ Google Scholar ] [ CrossRef ] [ PubMed ]
- Wang, S.A.; Lu, M.H.; Lee, A.T.; Chen, C.Y.; Lee, L.W. Performance of Microsoft Azure Kinect DK as a tool for Estimating Human Body Segment Lengths. Sci. Rep. 2024 , 14 , 15811. [ Google Scholar ] [ CrossRef ]
- Kennedy, S.; Smith, B.; Sobhiyeh, S.; Dechenaud, M.E.; Wong, M.; Kelly, N.; Shepherd, J.; Heymsfield, S.B. Digital Anthropometric Evaluation of Young Children: Comparison to Results Acquired with Conventional Anthropometry. Eur. J. Clin. Nutr. 2022 , 76 , 251–260. [ Google Scholar ] [ CrossRef ] [ PubMed ]
- Nolwenn Regnault, N.; Gillman, M.W.; Kleinman, K.; Rifas-Shiman, S.; Botton, J. Comparative Study of Four Growth Models Applied to Weight and Height Growth Data in a Cohort of US Children from Birth to 9 Years. Ann. Nutr. Metab. 2014 , 65 , 167–174. [ Google Scholar ] [ CrossRef ] [ PubMed ]
Click here to enlarge figure
Group/Class | Children’s Age | Cities/Countries (Number of Children per Location) | Number (Total) (F) | Frequency (%) (Fr) |
---|---|---|---|---|
Preschool children | ||||
I | ≥2–<3 years | Skopje (8); Sofia (2); Zagreb (11) | 21 | 2.8 |
II | ≥3–<4 years | Skopje (30); Sofia (46); Zagreb (58) | 134 | 17.9 |
III | ≥4–<5 years | Skopje (71); Sofia (71); Zagreb (66) | 208 | 27.8 |
IV | ≥5–<6 years | Skopje (86); Sofia (62); Zagreb (62) | 210 | 28.1 |
V | ≥6–<7+ years * | Skopje (25); Sofia (89); Zagreb (60) | 174 | 23.4 |
N | Skopje (220); Sofia (270); Zagreb (257) | 747 | 100.0 | |
School children | ||||
1 | ≥6.5–<7.5 years | Zagreb | 51 | 9.4 |
1–2 | ≥7.5–<8.5 years | Zagreb | 22 | 3.9 |
2–3 | ≥8.5–<9.5 years | Zagreb | 73 | 13.0 |
3–4 | ≥9.5–<10.5 years | Zagreb | 84 | 15.0 |
4–5 | ≥10.5–<11.5 years | Zagreb | 88 | 15.7 |
5–6 | ≥11.5–<12.5 years | Zagreb | 69 | 12.3 |
6–7 | ≥12.5–<13.5 years | Zagreb | 64 | 11.4 |
7–8 | ≥13.5–<14.5 years | Zagreb | 78 | 13.9 |
8 | ≥14.5–<16 years | Zagreb | 30 | 5.3 |
N | Zagreb | 560 | 100.0 | |
Total (all polygons) | 1307 |
Group | Children’s Age | N (Total Number of Children) | Male | Female | Frequency (Fr) (%) | |
---|---|---|---|---|---|---|
i | ≥2–<3 | 21 | 11 | 10 | 1.6 | |
ii | ≥3–<4 | 134 | 68 | 66 | 10.3 | |
iii | ≥4–<5 | 208 | 96 | 112 | 15.9 | |
iv | ≥5–<6 | 210 | 119 | 91 | 16.1 | |
v | ≥6–<7 | 174 | 84 | 90 | 13.3 | |
vi | ≥7–<8 | 57 | 35 | 22 | 4.4 | |
vii | ≥8–<9 | 59 | 33 | 26 | 4.5 | |
viii | ≥9–<10 | 73 | 44 | 29 | 5.6 | |
ix | ≥10–<11 | 87 | 31 | 56 | 6.7 | |
x | ≥11–<12 | 77 | 38 | 39 | 5.9 | |
xi | ≥12–<13 | 66 | 37 | 29 | 5.0 | |
xii | ≥13–<14 | 71 | 35 | 36 | 5.4 | |
xii | ≥14–<15 | 62 | 38 | 24 | 4.7 | |
ivx | ≥15–<16 | 8 | 7 | 1 | 0.6 | |
Total | 1307 | 676 (51.7%) | 631 (48.3%) | 100.0 |
Stature (A) | The vertical distance from the vertex of the head (V) to the substrate on which the feet are placed. The head is positioned in the horizontal Frankfort Plane [ ]. | |
Head length (B) | Vertical distance from the vertex of the head (V) to the top of the chin (gnathion, gn). |
Age Group | N | Mean (SD) | Median | Min–Max | Skewness | Turkey, 1977 Outside | Turkey, 1977 Far-Out | Generalized ESD Test | |
---|---|---|---|---|---|---|---|---|---|
≥2–<3 | 21 | body | 95.2 (3.9) | 95.4 | 86.7–101.4 | −0.506 | none | none | none |
head | 17.2 (0.9) | 17.0 | 15.5–19.0 | 0.399 | none | none | none | ||
ratio | 5.56 (0.35) | 5.61 | 4.73–6.11 | −0.969 | 4.73 | none | 4.73, 4.81 | ||
19 | none | none | none | ||||||
≥3–<4 | 134 | body | 101.8 (3.9) | 101.5 | 89.7–112.3 | −0.183 | one 89.7 | none | none |
head | 17.5 (1.0) | 17.5 | 15.6–22.0 | 0.885 * | 20, 20 | 22 | 22 | ||
133 | body | 101.9 (3.8) | 101.5 | 92.2–112.3 | −0.018 | none | none | none | |
131 | head | 17.5 (0.9) | 17.5 | 15.6–19.6 | 0.281 | none | none | none | |
130 | ratio | 5.83 (0.31) | 5.86 | 4.95–6.57 | −0.395 | 4.95, 5.06, 5.11, 6.57 | none | none | |
126 | none | none | none | ||||||
≥4–<5 | 208 | body | 107.8 (4.7) | 107.5 | 94.2–118.7 | 0.018 | 94.2 | none | none |
head | 18.0 (1.1) | 17.8 | 15.3–21.5 | 0.459 * | 21, 21.2, 21.5 | none | none | ||
207 | body | 107.9 (4.6) | 107.5 | 95.5–118.7 | 0.104 | none | none | none | |
205 | head | 17.9 (1.0) | 17.8 | 15.3–20.5 | 0.196 * | 15.3 | none | none | |
204 | head | 17.9 (0.9) | 17.8 | 15.6–20.5 | 0.270 * | none | none | none | |
203 | ratio | 6.00 (0.33) | 5.99 | 5.25–6.95 | 0.241 | 6.95 | none | none | |
202 | none | none | none | ||||||
≥5–<6 | 210 | body | 114.6 (5.1) | 114.6 | 93.4–132.7 | −0.089 | 129.9, 132.7, | 93.4 | 93.4, 132.7 |
head | 18.7 (1.2) | 18.6 | 16.0–22.5 | 0.494 | 22.1, 22.5, 22.5 | none | none | ||
207 | body | 114.5 (4.6) | 114.5 | 102.5–126.3 | −0.082 | 102.5, 102.6 | none | none | |
205 | body | 114.7 (4.5) | 114.6 | 104.5–126.3 | 0.021 | 126.3 | none | none | |
204 | body | 114.6 (4.4) | 114.6 | 104.5–124.6 | −0.030 | none | none | none | |
207 | head | 18.6 (1.1) | 18.6 | 16.0–21.8 | 0.244 * | none | none | none | |
201 | ratio | 6.17 (0.35) | 6.16 | 5.33–7.21 | 0.329 | 7.21 | none | none | |
200 | none | none | none | ||||||
≥6–<7 | 174 | body | 122.8 (5.2) | 122.6 | 110.3–138.0 | 0.185 | 136.5, 138 | none | none |
head | 19.4 (1.1) | 19.3 | 16.6–25.5 | 1.313 * | 22.5, 23, 23 | 25.5 | 23, 23, 25.5 | ||
172 | body | 122.7 (5.0) | 122.6 | 110.3–134.5 | 0.033 | none | none | none | |
170 | head | 19.3 (0.9) | 19.3 | 16.6–22.0 | 0.117 * | none | none | none | |
168 | ratio | 6.37 (0.32) | 6.35 | 5.58–7.34 | 0.248 | 5.58, 7.29, 7.34 | none | none | |
165 | ratio | 6.37 (0.30) | 6.35 | 5.66–7.12 | 0.105 | 7.11, 7.12 | none | none | |
163 | none | none | none | ||||||
≥7–<8 | 57 | body | 128.2 (6.3) | 129.0 | 111.6–141.0 | −0.278 | none | none | none |
head | 20.5 (2.3) | 20.0 | 17.7–33.0 | 3.107 * | 25 | 33 | 25, 33 | ||
55 | head | 20.2 (1.4) | 20.0 | 17.7–24.0 | 0.481 | none | none | none | |
none | none | none | |||||||
≥8–<9 | 59 | body | 136.0 (5.9) | 136.0 | 124.0–149.0 | 0.048 | none | none | none |
head | 21.3 (1.5) | 21.0 | 18.0–26.0 | 0.283 | 18, 18, 24.5, 26 | none | 26 | ||
55 | head | 21.3 (1.2) | 21.0 | 19.0–24.0 | 0.039 | none | none | none | |
none | none | none | |||||||
≥9–<10 | 73 | body | 139.5 (5.9) | 139.0 | 127.0–158.0 | 0.618 * | 152.5, 155, 158 | none | 158 |
head | 21.1 (1.9) | 21.0 | 17.0–29.0 | 0.915 * | none | 29 | 29 | ||
70 | body | 138.8 (5.0) | 138.8 | 127.0–151.0 | −0.011 | none | none | none | |
72 | head | 20.9 (1.7) | 21.0 | 17.0–24.5 | 0.060 * | none | none | none | |
69 | ratio | 6.68 (0.57) | 6.65 | 5.59–8.41 | 0.698 | 8.32, 8.41 | none | 8.32, 8.41 | |
67 | none | none | none | ||||||
≥10–<11 | 87 | body | 145.3 (6.2) | 145.0 | 129.0–158.5 | 0.057 | 129 | none | none |
head | 21.3 (1.6) | 21.5 | 18.0–25.5 | 0.149 * | 25.5 | none | none | ||
86 | body | 145.5 (6.0) | 145.0 | 133.5–158.5 | 0.208 | none | none | none | |
head | 21.3 (1.5) | 21.3 | 18.0–25.0 | 0.016 * | none | none | none | ||
85 | none | none | none | ||||||
≥11–<12 | 77 | body | 152.3 (8.2) | 152.0 | 126.0–167.0 | −0.279 | 126 | none | 126 |
head | 21.6 (1.8) | 22.0 | 17.5–28.0 | 0.381 * | 28 | none | 28 | ||
76 | body | 152.6 (7.7) | 152.0 | 139.0–167.0 | 0.059 | none | none | none | |
76 | head | 21.5 (1.6) | 22.0 | 17.5–25.5 | −0.146 * | none | none | none | |
75 | ratio | 7.11 (0.49) | 7.13 | 6.00–8.66 | 0.375 | 8.66 | none | 8.66 | |
74 | none | none | none | ||||||
≥12–<13 | 66 | body | 158.5 (7.0) | 157.8 | 139.0–171.5 | −0.192 | none | none | none |
head | 21.4 (1.5) | 21.5 | 18.0–24.0 | −0.198 * | none | none | none | ||
none | none | none | |||||||
≥13–<14 | 71 | body | 163.9 (7.7) | 164.0 | 144.5–183.0 | −0.221 | none | none | none |
head | 22.0 (1.5) | 22.0 | 19.0–26.5 | 0.355 * | 26.5 | none | none | ||
70 | head | 21.9 (1.4) | 22.0 | 19.0–25.5 | 0.101 * | none | none | none | |
none | none | none | |||||||
≥14–<15 | 62 | body | 169.4 (7.8) | 169.0 | 154.2–186.0 | 0.094 | none | none | none |
head | 22.3 (1.7) | 22.5 | 18.0–26.0 | −0.161 | none | none | none | ||
none | none | none | |||||||
≥15–<16 | 8 | body | 170.3 (7.9) | 168.0 | 162.0–183.5 | 0.768 | none | none | none |
head | 23.0 (2.5) | 22.3 | 20.0–28.0 | 1.134 | none | none | 20, 21, 22.5, 24, 24.5, 28 | ||
2 | head | 22.0 (−) | - | 22.0–22.0 | - | - | - | - | |
2 | 7.48 (0.10) † | none | none | none |
Age Group | Gender | N | Mean (SD) | 95% CI for the Mean | Median | 95% CI for the Median | Min–Max | Skewness | p * |
---|---|---|---|---|---|---|---|---|---|
≧2–<3 | Male | 9 | 5.77 (0.21) | 5.60–5.93 | 5.72 | 5.53–5.98 | 5.50–6.11 | 0.311 | 0.05 |
Female | 10 | 5.54 (0.24) | 5.37–5.71 | 5.59 | 5.31–5.78 | 5.16–5.80 | −0.462 | ||
≧3–<4 | Male | 64 | 5.76 (0.25) | 5.70–5.82 | 5.80 | 5.73–5.86 | 5.19–6.31 | −0.412 † | <0.001 |
Female | 62 | 5.93 (0.28) | 5.86–6.00 | 5.98 | 5.89–6.05 | 5.27–6.43 | −0.446 | ||
≧4–<5 | Male | 94 | 5.93 (0.31) | 5.86–5.99 | 5.94 | 5.83–6.02 | 5.25–6.77 | 0.052 | 0.003 |
Female | 108 | 6.06 (0.32) | 6.00–6.12 | 6.02 | 5.96–6.12 | 5.47–6.79 | 0.279 | ||
≧5–<6 | Male | 112 | 6.10 (0.32) | 6.04–6.16 | 6.07 | 5.98–6.16 | 5.45–6.94 | 0.524 | 0.001 |
Female | 88 | 6.26 (0.35) | 6.18–6.33 | 6.27 | 6.18–6.33 | 5.33–7.03 | −0.101 | ||
≧6–<7 | Male | 82 | 6.25 (0.25) | 6.19–6.30 | 6.26 | 6.21–6.33 | 5.66–6.73 | −0.267 | <0.001 |
Female | 81 | 6.47 (0.29) | 6.40–6.53 | 6.46 | 6.37–6.55 | 5.83–6.97 | −0.116 | ||
≧7–<8 | Male | 33 | 6.26 (0.41) | 6.11–6.40 | 6.36 | 6.11–6.46 | 5.17–6.99 | −0.678 | 0.03 |
Female | 22 | 6.51 (0.39) | 6.33–6.68 | 6.47 | 6.35–6.67 | 5.86–7.42 | 0.365 | ||
≧8–<9 | Male | 30 | 6.48 (0.44) | 6.32–6.65 | 6.55 | 6.24–6.70 | 5.67–7.32 | −0.087 | 0.08 |
Female | 25 | 6.30 (0.32) | 6.16–6.43 | 6.27 | 6.14–6.41 | 5.65–7.05 | 0.428 | ||
≧9–<10 | Male | 41 | 6.56 (0.48) | 6.41–6.71 | 6.56 | 6.38–6.69 | 5.59–7.72 | 0.29 | 0.14 |
Female | 26 | 6.74 (0.53) | 6.53–6.95 | 6.78 | 6.42–7.09 | 5.84–7.68 | −0.013 | ||
≧10–<11 | Male | 30 | 6.61 (0.42) | 6.46–6.77 | 6.61 | 6.43–6.70 | 5.92–7.35 | 0.219 | 0.001 |
Female | 55 | 6.99 (0.50) | 6.86–7.13 | 6.95 | 6.75–7.10 | 5.79–8.06 | 0.346 | ||
≧11–<12 | Male | 36 | 6.96 (0.46) | 6.80–7.11 | 6.97 | 6.76–7.18 | 6.00–8.05 | 0.059 | 0.02 |
Female | 38 | 7.22 (0.44) | 7.07–7.36 | 7.19 | 7.04–7.41 | 6.22–8.32 | 0.189 | ||
≧12–<13 | Male | 37 | 7.39 (0.67) | 7.16–7.61 | 7.22 | 7.00–7.56 | 6.32–8.95 | 0.574 | 0.44 |
Female | 29 | 7.50 (0.53) | 7.30–7.70 | 7.46 | 7.18–7.78 | 6.64–8.62 | 0.420 | ||
≧13–<14 | Male | 34 | 7.45 (0.45) | 7.30–7.61 | 7.42 | 7.23–7.69 | 6.60–8.29 | 0.002 | 0.47 |
Female | 36 | 7.53 (0.41) | 7.39–7.67 | 7.46 | 7.30–7.64 | 6.59–8.60 | 0.473 | ||
14–<15 | Male | 38 | 7.58 (0.51) | 7.41–7.75 | 7.50 | 7.40–7.77 | 6.76–8.79 | 0.321 | 0.40 |
Female | 24 | 7.69 (0.53) | 7.47–7.92 | 7.60 | 7.42–7.87 | 6.83–8.90 | 0.748 |
Autor | [ ] Vitruvius (1914) (1st Century BCE) | [ ] Richer (1889) [ ] Barscay (1989) | [ ] Muftić (2001) | Results (2024) |
---|---|---|---|---|
Children’s Age | Canon (HL) | |||
4–5 | No data | No data | 5.5 HL | 5.99 HL |
5–6 | No data | 5.5 HL | No data | 6.18 HL |
10–11 | No data | 6.0 HL | No data | 6.80 HL |
14–15 | No data | 7.0 HL | No data | 7.63 HL |
21–35 | 8 HL | 7.5 HL | 8 HL | No data |
The statements, opinions and data contained in all publications are solely those of the individual author(s) and contributor(s) and not of MDPI and/or the editor(s). MDPI and/or the editor(s) disclaim responsibility for any injury to people or property resulting from any ideas, methods, instructions or products referred to in the content. |
Share and Cite
Domljan, D.; Iliev, B.; Jurčević Lulić, T. Research on Children’s Body Proportions: Determining the Canon of Head Length to Total Body Height on the Example of Children Aged 2 to 15 Years. Appl. Sci. 2024 , 14 , 7185. https://doi.org/10.3390/app14167185
Domljan D, Iliev B, Jurčević Lulić T. Research on Children’s Body Proportions: Determining the Canon of Head Length to Total Body Height on the Example of Children Aged 2 to 15 Years. Applied Sciences . 2024; 14(16):7185. https://doi.org/10.3390/app14167185
Domljan, Danijela, Boris Iliev, and Tanja Jurčević Lulić. 2024. "Research on Children’s Body Proportions: Determining the Canon of Head Length to Total Body Height on the Example of Children Aged 2 to 15 Years" Applied Sciences 14, no. 16: 7185. https://doi.org/10.3390/app14167185
Article Metrics
Article access statistics, further information, mdpi initiatives, follow mdpi.
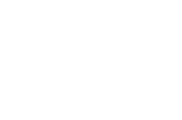
Subscribe to receive issue release notifications and newsletters from MDPI journals

An official website of the United States government
The .gov means it’s official. Federal government websites often end in .gov or .mil. Before sharing sensitive information, make sure you’re on a federal government site.
The site is secure. The https:// ensures that you are connecting to the official website and that any information you provide is encrypted and transmitted securely.
- Publications
- Account settings
Preview improvements coming to the PMC website in October 2024. Learn More or Try it out now .
- Advanced Search
- Journal List
- Pak J Med Sci
- v.31(6); Nov-Dec 2015
The bread and butter of statistical analysis “t-test”: Uses and misuses
Statistical tests are very important in biomedical research. 1 Several factors play a role in selecting the most appropriate statistical test. 2 The misuse or inaccurate use of a statistical test may navigate the research in the wrong direction, and hence incorrect conclusions. Because it is probably the most commonly used statistical test, Student’s t-test is considered “the bread and butter” of statistical analysis. The William Gossett test “Student’s t-test” is easy to use, however, it is also misused. 3 There are three types of the t-test, which are used for comparing either a single mean or two population means ( Table-I ). Each t-test can be used under specific conditions and criteria.
Types of Student’s t-test.
Type of t-test | Test Description |
---|---|
One-sample t-test | To compare a single mean to a fixed number or gold standard |
Two-Sample t-test | To compare two populations means based on independent samples from the two populations or groups |
Paired t-test | To compare two means based on samples that are paired in some way |
1. One- Sample t-Test
It is used for comparing sample results with a known and specified value, sometimes a “gold standard”. The task of this test should be to answer the question “is the mean of the population from which the sample is taken is different from the specified value”? For example, based on a random sample of 200 students, can we conclude that the average IQ score this year is lower than the average from 3 years ago?
In most studies, a sample size of at least 40 can guarantee that the sample mean is approximately normally distributed, and the one-sample t-test can then be safely applied.
It is used to know whether the unknown means of two populations are different from each other based on independent samples from each population. To apply this test, it is very important that the two samples are independent and unrelated to each other. The samples can be obtained from two separate populations, or from a single population that has been randomly divided into two groups, and each group subjected to one of two treatments. The test is only valid for comparing means from a quantitative variable.
It is appropriate for data in which the two samples are paired in some way, such as the following examples.
3-1 Pairs consist of before and after measurements on a single group of subjects.
3-2 Two measurements on the same subject (e.g., right and left arm) are paired.
3-3 Subjects in one group (e.g., those receiving a treatment) are paired or matched on a one-to-one basis with subjects in a second group (e.g., control subjects).
- If the sample size is small (less than 15), the one-sample t-test should not be used if the data are clearly skewed or the outliers are present. Nonparametric test can be performed.
- If the sample size is moderate (at least 15), the one-sample t-test should not be used if there are severe outliers.
- If the outcome measure is categorical (nominal/discrete) variable such as, gender, and even if the data have been numerically coded, the two-sample t-test should not be applied.
- If a group of subjects receives one treatment, and then the same subjects later receive another treatment. This is a paired t-test and not two-sample t-test.
- If subjects receive a treatment, and then the results are compared to a known value (often a “gold standard”). This is a one-sample t-test and not two-sample t-test.
- If the study aims to compare three or more means, then it is better to use an analysis of variance to avoid the loss of control over the experiment-wise significant level.
Thank you for visiting nature.com. You are using a browser version with limited support for CSS. To obtain the best experience, we recommend you use a more up to date browser (or turn off compatibility mode in Internet Explorer). In the meantime, to ensure continued support, we are displaying the site without styles and JavaScript.
- View all journals
- Explore content
- About the journal
- Publish with us
- Sign up for alerts
- Open access
- Published: 07 August 2024
Molecular mimicry in multisystem inflammatory syndrome in children
- Aaron Bodansky ORCID: orcid.org/0000-0001-8943-8233 1 na1 ,
- Robert C. Mettelman ORCID: orcid.org/0000-0002-3527-5616 2 na1 ,
- Joseph J. Sabatino Jr 3 , 4 ,
- Sara E. Vazquez 5 ,
- Janet Chou 6 , 7 ,
- Tanya Novak ORCID: orcid.org/0000-0002-7115-7545 8 , 9 ,
- Kristin L. Moffitt 7 , 10 ,
- Haleigh S. Miller 5 , 11 ,
- Andrew F. Kung 5 , 11 ,
- Elze Rackaityte ORCID: orcid.org/0000-0003-3889-8082 5 ,
- Colin R. Zamecnik ORCID: orcid.org/0000-0002-9477-1388 3 , 4 ,
- Jayant V. Rajan 5 ,
- Hannah Kortbawi 5 , 12 ,
- Caleigh Mandel-Brehm 5 ,
- Anthea Mitchell 13 ,
- Chung-Yu Wang 13 ,
- Aditi Saxena 13 ,
- Kelsey Zorn ORCID: orcid.org/0000-0003-1227-2137 5 ,
- David J. L. Yu 14 ,
- Mikhail V. Pogorelyy 2 ,
- Walid Awad 2 ,
- Allison M. Kirk ORCID: orcid.org/0000-0002-4286-3678 2 ,
- James Asaki 15 ,
- John V. Pluvinage ORCID: orcid.org/0000-0002-9607-2783 4 ,
- Michael R. Wilson ORCID: orcid.org/0000-0002-8705-5084 3 , 4 ,
- Laura D. Zambrano 16 ,
- Angela P. Campbell ORCID: orcid.org/0000-0002-2576-482X 16 ,
- Overcoming COVID-19 Network Investigators ,
- Paul G. Thomas ORCID: orcid.org/0000-0001-7955-0256 2 na2 ,
- Adrienne G. Randolph 7 , 8 , 9 na2 ,
- Mark S. Anderson ORCID: orcid.org/0000-0002-3093-4758 14 , 17 na2 &
- Joseph L. DeRisi ORCID: orcid.org/0000-0002-4611-9205 5 , 13 na2
Nature volume 632 , pages 622–629 ( 2024 ) Cite this article
17k Accesses
801 Altmetric
Metrics details
- Autoimmunity
- Autoinflammatory syndrome
- Immune tolerance
- Inflammation
- Viral infection
Multisystem inflammatory syndrome in children (MIS-C) is a severe, post-infectious sequela of SARS-CoV-2 infection 1 , 2 , yet the pathophysiological mechanism connecting the infection to the broad inflammatory syndrome remains unknown. Here we leveraged a large set of samples from patients with MIS-C to identify a distinct set of host proteins targeted by patient autoantibodies including a particular autoreactive epitope within SNX8, a protein involved in regulating an antiviral pathway associated with MIS-C pathogenesis. In parallel, we also probed antibody responses from patients with MIS-C to the complete SARS-CoV-2 proteome and found enriched reactivity against a distinct domain of the SARS-CoV-2 nucleocapsid protein. The immunogenic regions of the viral nucleocapsid and host SNX8 proteins bear remarkable sequence similarity. Consequently, we found that many children with anti-SNX8 autoantibodies also have cross-reactive T cells engaging both the SNX8 and the SARS-CoV-2 nucleocapsid protein epitopes. Together, these findings suggest that patients with MIS-C develop a characteristic immune response to the SARS-CoV-2 nucleocapsid protein that is associated with cross-reactivity to the self-protein SNX8, demonstrating a mechanistic link between the infection and the inflammatory syndrome, with implications for better understanding a range of post-infectious autoinflammatory diseases.
Similar content being viewed by others
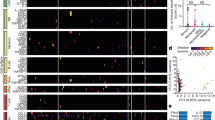
Diverse functional autoantibodies in patients with COVID-19
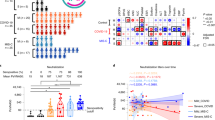
SARS-CoV-2 immune repertoire in MIS-C and pediatric COVID-19
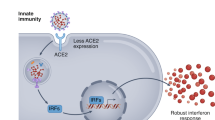
Immunology of SARS-CoV-2 infection in children
Children with severe acute respiratory syndrome coronavirus 2 (SARS-CoV-2) infections typically have mild disease 3 , 4 , but can develop a rare life-threatening post-infectious complication known as MIS-C 1 , 2 . MIS-C presents with a distinctive inflammatory signature indicative of altered innate immune responses 5 , 6 , including dysregulation of the mitochondrial antiviral signalling (MAVS) protein pathway 7 . Aberrant adaptive immunity is also involved, with multiple MIS-C-associated autoantibodies reported 8 , 9 , 10 , 11 , 12 . Furthermore, T cell signatures have also been associated with development of MIS-C 13 , 14 , 15 , 16 , which are accompanied by autoimmune-associated B cell expansions 8 . Some autoimmune diseases have been shown to involve tandem cross-reactive B cell and T cell responses. In multiple sclerosis, for example, cross-reactive B cells and T cells have been shown to respond to Epstein–Barr virus protein (EBNA1) and antigens in the human nervous system 17 , 18 , 19 . Decades of research into paraneoplastic autoimmune encephalitis has also demonstrated that autoreactive B cells and T cells can cause disease through coordinated targeting of a shared intracellular antigen and, in certain cases, a shared epitope 20 , 21 , 22 , 23 , 24 , 25 , 26 . Despite intense interest, a pathophysiological link between SARS-CoV-2 and MIS-C remains enigmatic, and identification of disease-specific autoantigens remains incompletely explored. Here children previously infected with SARS-CoV-2 with ( n = 199) and without ( n = 45) MIS-C were enrolled and comprehensively evaluated for differential autoreactivity to the entire human and SARS-CoV-2 proteome. Patients with MIS-C were found to have both cross-reactive antibodies and T cells targeting an epitope motif shared by the viral nucleocapsid protein and human SNX8, a protein involved in MAVS antiviral function 27 . These findings suggest that many cases of MIS-C may be triggered by molecular mimicry and could provide a framework for identifying potential cross-reactive epitopes in other autoimmune and inflammatory diseases with predicted viral triggers such as Kawasaki disease 28 , type 1 diabetes mellitus (T1DM) 29 and multiple sclerosis.
Patients with MIS-C have a distinct set of autoreactivities
To explore the hypothesis that MIS-C is driven by an autoreactive process, we evaluated the proteome-wide autoantibody profiles of children with MIS-C ( n = 199) and children convalescing following asymptomatic or mild SARS-CoV-2 infection without MIS-C ( n = 45, hereafter referred to as ‘at-risk controls’) using our custom phage immunoprecipitation and sequencing (PhIP-seq) 30 library, which has previously been used to define novel autoimmune syndromes and markers of disease for various conditions 12 , 24 , 25 , 31 , 32 , 33 . Given the inherently heterogeneous nature of antibody repertoires among individuals 34 , the identification of disease-associated autoreactive antigens requires the use of large numbers of cases and controls 12 . To minimize spurious hits, this study includes substantially more patients with MIS-C and controls than similar, previously published studies 8 , 9 , 10 , 12 (Fig. 1a ). Clinical characteristics of this cohort are described in Extended Data Table 1 .
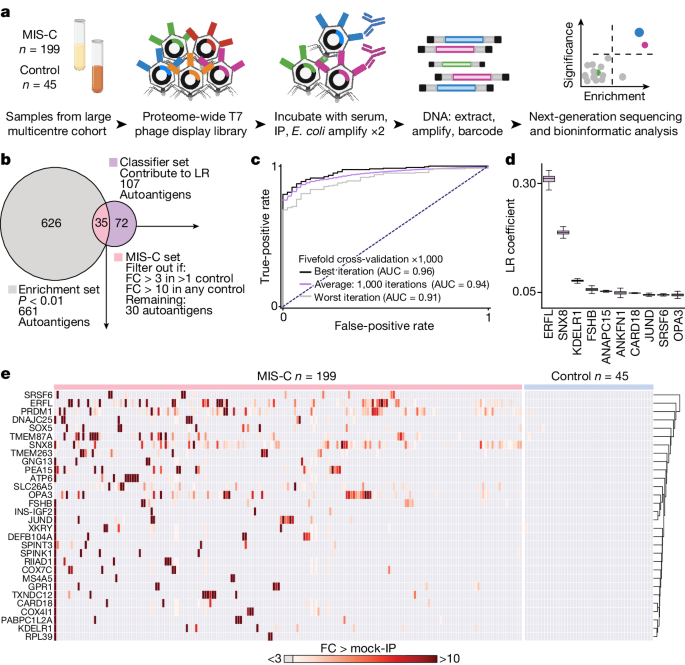
a , Design of the PhIP-seq experiment comparing patients with MIS-C ( n = 199) and at-risk controls ( n = 45; children with SARS-CoV-2 infection at least 5 weeks before sample collection without symptoms of MIS-C). Schematics in panel a were created using BioRender ( https://www.biorender.com ). b , Venn diagram highlighting the number of autoantigens identified with statistically significant PhIP-seq enrichment (‘enrichment set’: grey circle; P < 0.01 on one-sided Kolmogorov–Smirnov test with false discovery rate correction) and autoantigens identified, which contribute to a logistic regression classifier of MIS-C relative to at-risk controls (‘classifier set’: purple circle). There are 35 autoantigens present in both the classifier set and the enrichment set (pink; union of the Venn diagram) of which 30 are exclusive to MIS-C and referred to as the ‘MIS-C set’ (no two controls have low reactivity as defined by the fold-change (FC) signal over the mean of protein A/G beads only (FC > mock-IP) of 3 or greater, and no single control has high reactivity defined as FC > mock-IP greater than 10). LR, logistic regression. c , Receiver operating characteristic curve for the logistic regression classifier showing upper and lower bounds of performance through 1,000 iterations. d , Bar plots with error bars showing logistic regression coefficients for the top 10 autoantigens across 1,000 iterations. The whiskers extend to 1.5 times the interquartile range (IQR) from the quartiles. The boxes represent the IQR, and the centre lines represent the median. e , Hierarchically clustered (Pearson) heatmap showing the PhIP-seq enrichment (FC > mock-IP) for the 30 autoantigens in the MIS-C set in each patient with MIS-C and each at-risk plasma control.
Source Data
For a given set of samples, PhIP-seq can yield dozens to thousands of differential enrichments of phage-displayed peptides. Here logistic regression machine learning was used as an initial unbiased measure of how accurately a set of differentially enriched peptides could classify people with MIS-C and controls—an approach that has been used to classify people with autoimmune polyglandular syndrome type 1 using PhIP-seq data 12 . In all, 107 proteins had logistic regression coefficients greater than zero (‘classifier set’; Fig. 1b ). As this is an unbalanced dataset with a random accuracy less than 50%, we also generated a receiver operating characteristic (ROC) curve. ROC analysis iterated 1,000 times and yielded an average area under the curve (AUC) of 0.94 (Fig. 1c ). Examination of the logistic regression coefficients associated with MIS-C revealed the largest contributions from peptides derived from the ETS repressor factor-like (ERFL), sorting nexin 8 (SNX8) and KDEL endoplasmic reticulum protein retention receptor 1 (KDELR1) coding sequences (Fig. 1d ).
In parallel, a Kolmogorov–Smirnov test was used to define a set of 661 autoreactivities statistically enriched after false discovery rate adjustment for multiple comparisons ( q < 0.01; ‘enrichment set’). To avoid false positives, the intersection of the classifier set and enrichment set were considered further. Of these 35 hits, peptides derived from 30 different proteins satisfied an additional set of conservative criteria, requiring that none was enriched (fold change over mock-immunoprecipitation (IP) of more than 3) in more than a single control, or was enriched more than 10-fold in any control (‘MIS-C set’; Fig. 1e ).
Previously reported MIS-C autoantibodies
To date, at least 34 autoantigen candidates have been reported to associate with MIS-C 8 , 9 , 10 , 12 . However, we found that only UBE3A (a ubiquitously expressed ubiquitin protein ligase) was differentially enriched in our MIS-C dataset, whereas the remaining 33 were present in a similar proportion of cases with MIS-C and at-risk controls (Extended Data Fig. 1a ). Autoreactivity to UBE3A was independently identified in this study as part of both the classifier and the enrichment sets, but was not included in the final MIS-C set due to the low positive signal present in two controls.
In addition, autoantibodies to the receptor antagonist IL-1RA have been previously reported in 13 of 21 (62%) patients with MIS-C 11 . In this cohort, anti-IL-1RA antibodies were detected by PhIP-seq ( z score > 6 over at-risk control) in six patient samples. To further examine immune reactivity to full-length IL-1RA, sera from 196 of the 199 patients in this study were used to immunoprecipitate [35S]-methionine-radiolabelled IL-1RA (radioligand-binding assay (RLBA)). Positive immunoprecipitation of IL-1RA (defined as more than 3 s.d. above mean of controls) was found in 39 of 196 (19.9%) patients with MIS-C. However, many patients with MIS-C were treated with intravenous immunoglobulin (IVIG), a blood product shown to contain autoantibodies 35 . After removing samples from patients treated with IVIG (61 remaining), the difference between samples from patients with MIS-C (5 of 61, 8.2%) and at-risk controls (1 of 45, 2.2%) was not significant ( P = 0.299; Extended Data Fig. 1b ).
MIS-C autoantigens lack tissue-specific associations with clinical phenotypes
Consistent with previous MIS-C reports 1 , 5 , this cohort was clinically heterogeneous (Extended Data Table 2 ). To determine whether specific phenotypes, including myocarditis and the requirement of vasopressors, might be associated with specific autoantigens present in the MIS-C set, tissue expression levels were assigned to each autoantigen 36 (Human Protein Atlas; https://proteinatlas.org ), including the amount of expression in cardiomyocytes and the cardiac endothelium. The PhIP-seq signal for patients with MIS-C with a particular phenotype was compared with those patients with MIS-C without the phenotype. Autoantigens with tissue specificity were not enriched in those patients with MIS-C with phenotypes involving said tissue. Similarly, autoantigens associated with myocarditis or vasopressor requirements did not correlate with increased cardiac expression (Extended Data Fig. 1c ).
Orthogonal validation of PhIP-seq autoantigens
Peptides derived from ERFL, SNX8 and KDELR1 carried the largest logistic regression coefficients in the MIS-C classifier. The PhIP-seq results were orthogonally confirmed by RLBAs using full-length ERFL, SNX8 and KDELR1 proteins. Relative to at-risk controls, samples from patients with MIS-C significantly enriched each of the three target proteins ( P < 1 × 10 −10 for ERFL, SNX8 and KDELR1), consistent with the PhIP-seq assay (Extended Data Fig. 2a ). Using only the RLBA data for these three proteins, MIS-C could be confidently classified (ROC with fivefold cross-validation; 1,000 iterations) from at-risk control sera with an AUC of 0.93, suggesting the potential for molecular diagnostic purposes (Extended Data Fig. 2b ).
As noted, IVIG was administered to 138 of the 199 patients with MIS-C before sample collection and was absent from all 45 at-risk controls. The autoreactivity to the ERFL, SNX8 and KDELR1 proteins from the 61 patients with MIS-C who had not been treated with IVIG before sample collection were compared with the at-risk controls. In contrast to IL-1RA, the differential enrichment of these three proteins remained significant ( P = 6.69 × 10 −10 , P = 6.26 × 10 −5 and P = 0.0001, respectively), suggesting that autoreactivity to ERFL, SNX8 and KDELR1 proteins was not confounded by IVIG treatment (Extended Data Fig. 2c ).
Independent MIS-C cohort validation
To further test the validity of these findings, an independent validation cohort consisting of samples from 24 different patients with MIS-C and 29 children with severe acute COVID-19 was evaluated (acquired via ongoing enrolment of the Overcoming COVID-19 study; Extended Data Table 3 ). Using RLBAs with full-length ERFL, SNX8 and KDELR1 proteins, we found that all three target proteins were significantly enriched compared with both the at-risk controls ( P = 0.00022, P = 3.68 × 10 −5 and P = 2.36 × 10 −5 , respectively) and the patients with severe acute COVID-19 ( P = 0.0066, P = 0.00735 and P = 0.00114, respectively; Extended Data Fig. 2d ). A logistic regression model, trained on the original cohort, classified MIS-C from at-risk controls with an AUC of 0.84, and from severe acute paediatric COVID-19 with an AUC of 0.78 (Extended Data Fig. 2e ). This suggests that autoreactivity to ERFL, SNX8 and KDELR1 is a significant feature of MIS-C that is separable from SARS-CoV-2 exposure and severe acute paediatric COVID-19.
MIS-C autoantibodies target a single epitope within the SNX8 protein
SNX8 is a protein that is 456 amino acids and belongs to a family of sorting nexins involved in endocytosis, endosomal sorting and signalling 37 . Publicly available expression data 36 (Human Protein Atlas) show that SNX8 is widely expressed across various tissues including the brain, heart, gastrointestinal tract, kidneys and skin, with the highest expression in undifferentiated cells and immune cells. Previous work has associated SNX8 with host defence against RNA viruses 27 . ERFL is a poorly characterized 354-amino acid protein. A survey of single-cell RNA sequencing (scRNA-seq) data 36 (Human Protein Atlas) suggests enrichment in plasma cells, B cells and T cells in some tissues. Using a Spearman correlation in principal component analysis (PCA) space based on tissue RNA-seq data 36 (Human Protein Atlas), SNX8 has the second closest expression pattern to ERFL compared with all other coding genes, with a correlation coefficient of 0.81. KDELR1 is a 212-amino acid endoplasmic reticulum–Golgi transport protein essential to lymphocyte development with low tissue expression specificity. All three proteins are predicted to be intracellular, suggesting that putative autoantibodies targeting these proteins are unlikely to be sufficient for disease pathology on their own. However, autoantibodies targeting intracellular antigens are often accompanied by autoreactive T cells specific for the protein from which that antigen was derived, and which targets cell types expressing the protein 22 , 25 , 26 , 38 . We selected SNX8 for further investigation, given its enrichment in immune cells and its putative role in regulating the MAVS pathway in response to RNA virus infection, a pathway implicated in MIS-C pathology 7 .
Full-length SNX8 is represented in this PhIP-seq library by 19 overlapping 49-mer peptides. For all but one patient sample, the peptide fragment spanning amino acid positions 25–73 was the most enriched in the PhIP-seq assay (Fig. 2a ), suggesting a common autoreactive site. A sequential alanine scan was performed to determine the minimal immunoreactive peptide sequence (Fig. 2b ; Methods ). Using samples from six individuals with MIS-C, we determined that the critical region for immunoreactivity was a nonamer spanning positions 51–59 (PSRMQMPQG). Using the wild-type 49-amino acid peptide and the version with the critical region mutated to alanine, 182 of the 199 patients with MIS-C (insufficient sample for the remaining 17) and all 45 controls were assessed for immunoreactivity using a split-luciferase-binding assay (SLBA). We found that samples from 31 of 182 (17.0%) patients with MIS-C immunoprecipitated the wild-type fragment. Of these, 29 (93.5%) failed to immunoprecipitate the mutated peptide, suggesting a common shared autoreactive epitope among nearly all of the patients with MIS-C with anti-SNX8 antibodies (Extended Data Fig. 2f ).
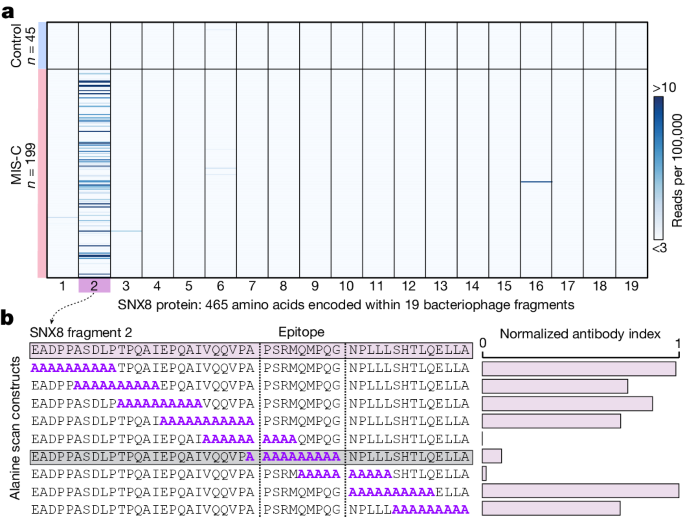
a , PhIP-seq signal (reads per 100,000) for each patient with MIS-C ( n = 199) and each at-risk control ( n = 45) across each of the 19 bacteriophage-encoded peptide fragments, which together tile the full-length SNX8 protein. b , SLBA enrichments (normalized antibody indices) for each sequential alanine mutagenesis construct. Constructs were designed with 10 amino acid alanine windows (highlighted in purple) shifted by 5 amino acids until the entire immunodominant SNX8 region (SNX8 fragment 2) was scanned. Values are averages of six separate patients with MIS-C. The identified autoantibody epitope is bounded by vertical grey dotted lines.
Patients with MIS-C have an altered antibody response to the SARS-CoV-2 nucleocapsid protein
To evaluate whether differences exist in the humoral immune response to SARS-CoV-2 infection in patients with MIS-C relative to at-risk controls, we repeated PhIP-seq with 181 of the original 199 patients with MIS-C and all 45 of the at-risk controls using a previously validated library specific for SARS-CoV-2 (ref. 39 ). To discover whether certain fragments were differentially enriched in either patients with MIS-C or at-risk controls, the enrichment of each phage encoded SARS-CoV-2 peptide (38 amino acids each) across all patients with MIS-C and at-risk controls was normalized to 48 healthy controls pre-COVID-19. Three nearly adjacent peptides derived from the SARS-CoV-2 nucleocapsid protein (fragments 5, 8 and 9) were significantly enriched (Kolmogorov–Smirnov test P < 0.0001 for each). The first peptide (fragment 5), spanning amino acids 77–114, was significantly enriched in the at-risk controls (representing the typical serological response in children), whereas the next two fragments (fragments 8 and 9), spanning amino acids 134–190, were significantly enriched in patients with MIS-C (Fig. 3a,b ). The most differentially reactive region of the SARS-CoV-2 nucleocapsid protein in patients with MIS-C (fragment 8) was termed the MIS-C-associated domain of SARS-CoV-2 (MADS). The PhIP-seq results were orthogonally confirmed using an SLBA measuring the amount of MADS peptide immunoprecipitated with samples from 16 individuals, including 11 patients with MIS-C and 5 at-risk controls (Fig. 3c ). To precisely map the minimal immunoreactive region of MADS in MIS-C samples, peptides featuring a sliding window of ten alanine residues were used as the immunoprecipitation substrate for SLBAs, run in parallel with the SNX8 alanine scanning peptides using sera from three patients with MIS-C (Fig. 3d ). The critical regions identified here in both SNX8 and MADS were highly similar, represented by the (ML)Q(ML)PQG motif (Fig. 3e ).
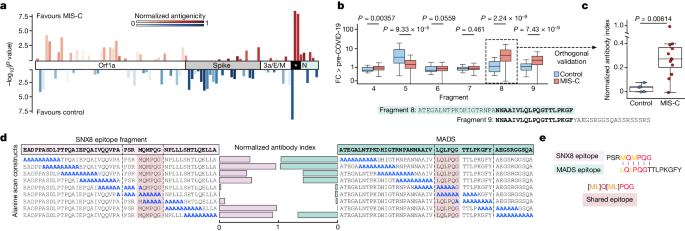
a , Relative PhIP-seq signal (FC over the mean) of 48 controls who are pre-COVID-19 (FC > pre-COVID-19) in patients with MIS-C ( n = 181) and at-risk controls ( n = 45) using a custom phage display library expressing the entire SARS-CoV-2 proteome to different regions of SARS-CoV-2. Only regions with a mean antibody signal of more than 1.5-fold above pre-COVID-19 controls are shown. Antigenicity (sum of the mean FC > pre-COVID-19 in MIS-C and at-risk controls) are represented by darker shades. The length of the bars represents the statistical difference in signal between MIS-C and at-risk controls to a particular region (−log 10 of two-sided Kolmogorov–Smirnov test P values), with upward deflections representing enrichment in MIS-C versus at-risk controls, and downward deflections representing less signal in MIS-C. The asterisk indicates the differentially reactive region of the nucleocapsid (N) protein. b , Bar plots showing the PhIP-seq signal (FC > pre-COVID-19) across the specific region of the SARS-CoV-2 nucleocapsid protein (fragments 4–9) with the most divergent response in MIS-C samples ( n = 181) relative to at-risk controls ( n = 45), compared using a two-sided Kolmogorov–Smirnov test (exact P values are shown in the figure). The amino acid sequence of the region with the highest relative enrichment in MIS-C is highlighted in green and referred to as MADS. c , Strip plots and box plots showing MADS SLBA enrichments (normalized antibody indices) in patients with MIS-C ( n = 11) relative to at-risk controls ( n = 5). d , SLBA signal (normalized antibody indices) for full sequential alanine mutagenesis scans within the same three individuals for SNX8 (left) and MADS (right). Each identified epitope is bounded by black vertical dotted lines. e , Multiple sequence alignment of SNX8 and MADS epitopes with the amino acid sequence for the similarity region shown (for the text in colour, biochemically similar is in orange, and identical is in red). For the box plots ( b , c ), the whiskers extend to 1.5 times the IQR from the quartiles. The boxes represent the IQR, and the centre lines represent the median.
Patients with MIS-C have significantly increased SNX8 autoreactive T cells
In other autoimmune diseases, autoantibodies often arise to intracellular targets, yet the final effectors of cellular destruction are autoreactive T cells 22 , 26 , 40 . Given evidence that certain subsets of MIS-C are associated with HLA 16 , and that SNX8 is an intracellular protein, we hypothesized that patients with MIS-C with anti-SNX8 antibodies may, in addition to possessing SNX8 autoreactive B cells, also possess autoreactive T cells targeting SNX8-expressing cells. To test this hypothesis, T cells from nine patients with MIS-C (eight from SNX8 autoantibody-positive patients and one who was SNX8 autoantibody negative) and ten at-risk controls (chosen randomly) were exposed to a pool of 15-mer peptides with 11-amino acid overlaps tiling the full-length human SNX8 protein. T cell activation was measured by an activation-induced marker assay, which quantifies upregulation of three cell activation markers: OX40, CD69 and CD137 (ref. 41 ). The percent of T cells activated in response to SNX8 protein was significantly higher in patients with MIS-C than in controls ( P = 0.00126). Using a positive cut-off of 3 s.d. above the mean of the controls, 7 of the 9 (78%) patients with MIS-C were positive for SNX8-expressing autoreactive T cells, whereas 0 of 10 (0%) controls met these criteria (Fig. 4a ). With respect to CD4 + and CD8 + subgroups, there was an increased signal in patients with MIS-C compared with controls, which did not meet significance ( P = 0.0711 and P = 0.0581, respectively; Extended Data Fig. 3a ). The patient with MIS-C who was seronegative for the SNX8 autoantibody was also negative for SNX8 autoreactive T cells.
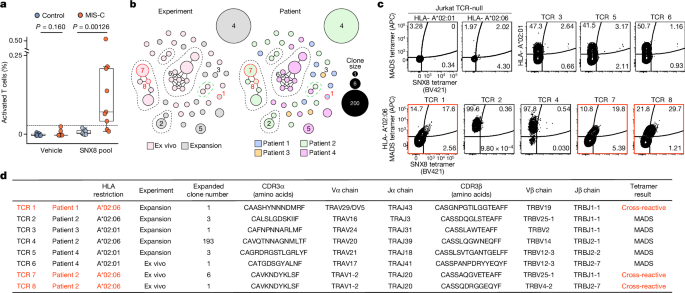
a , Strip plots and box plots showing the distribution of T cells activated in response to either vehicle (culture media + 0.2% DMSO) or the SNX8 peptide pool (SNX8 peptide + culture media + 0.2% DMSO) in patients with MIS-C ( n = 9) and controls ( n = 10). The relative signal was compared using a two-sided Mann–Whitney U -test (exact P values are shown in the figure). The box plot whiskers extend to 1.5 times the IQR from the quartiles, the boxes represent the IQR, and the centre lines represent the median. The dashed line is 3 s.d. above the mean of the controls in the SNX8 pool condition. b , TCRdist similarity network of 48 unique, paired TCRαβ sequences ( n = 259 sequences) obtained from four patients with MIS-C. CD8 + T cells were sorted from PBMCs directly ex vivo or after 10 days of peptide expansion and staining with A*02:01 or A*02:06 HLA class I tetramers loaded with MADS (LQLPQGITL) and SNX8 (MQMPQGNPL) peptides. Each node represents a unique TCR clonotype. Edges connect nodes with a TCRdist score of less than 150. The dashed lines surround TCR similarity clusters. The node size corresponds to the T cell clone size. Nodes are coloured based on the HLA experiment type (left) or patient (right). TCRs selected for further testing are numbered TCR 1–8. The convergent node is circled in green. c , Specificity of putative cross-reactive TCRs expressed in Jurkat-76 cells by HLA-A*02:01 or HLA-A*02:06 tetramers loaded with MADS (LQLPQGITL) and SNX8 (MQMPQGNPL) peptides. Jurkat-76 (TCR-null) cells were used as tetramer background staining controls. The gate values indicate the frequency of MADS–APC + and/or SNX8–BV421 + cells as the percentage of the total PE + cells (combination staining with MADS–PE and SNX8–PE tetramers). TCRs with confirmed cross-reactivity are indicated in red. Outliers are shown. Flow plots are representative of two independent evaluations. d , Summary of TCR sequencing results of the eight TCRs tested.
HLA type A*02 is more likely to present the shared epitope
MIS-C has been associated with HLA alleles A*02, B*35 and C*04 (ref. 16 ). The Immune Epitope Database and Analysis Resource ( https://IEDB.org ) 42 was used to rank the HLA class I (HLA-I) peptide presentation likelihoods for both SNX8 and SARS-CoV-2 nucleocapsid protein with respect to the MIS-C-associated HLA alleles. The distribution of predicted HLA-I-binding scores for nucleocapsid protein and SNX8 fragments matching the (ML)Q(ML)PQG SNX8/MADS motif relative to fragments lacking a match was compared. For HLA-A*02, predicted HLA-I binding was significantly higher ( P = 8.78 × 10 −10 for nucleocapsid protein; P = 0.0112 for SNX8) for fragments containing the putative autoreactive motif. There was no statistical difference for HLA-B*35 and HLA-C*04 predictions (Extended Data Fig. 3b,c ). Of note, of the seven patients with MIS-C with SNX8 autoreactive T cells, at least five were positive for HLA-A*02 (Extended Data Fig. 3a ). To experimentally validate HLA-I-binding predictions to SNX8 and MADS peptides, we measured peptide–HLA (pHLA) monomer stability using a β2 microglobulin (β2m) fold test, which is a proxy for pHLA-binding affinity in which anti-β2m staining reports on the strength of the pHLA complex 43 . SNX8 (MQMPQGNPL) and MADS (LQLPQGITL) peptides were loaded onto unfolded HLA-A*02:01, HLA-A*02:06 or HLA-B*35:01 monomers and stained with an anti-β2m fluorescent antibody. Consistent with the IEDB rankings, both HLA-A*02 alleles bound SNX8 and MADS peptides, with HLA-A*02:06 exhibiting the highest pHLA complex stability (Extended Data Fig. 3d ).
T cells from patients with MIS-C are cross-reactive to the SNX8 and nucleocapsid protein similarity regions
Given the prediction that HLA types associated with MIS-C preferentially display peptides containing the similarity regions for both SNX8 and the SARS-CoV-2 nucleocapsid protein, we sought to determine whether cross-reactive T cells were present and whether they were associated with MIS-C. We stimulated peripheral blood mononuclear cells (PBMCs) from three patients with MIS-C and three at-risk controls with peptides from either the SNX8 similarity region (MQMPQGNPL) or the MADS similarity region (LQLPQGITL) for 7 days to enrich for CD8 + T cells reactive to these epitopes. We then built differently labelled HLA-I tetramers loaded with either the SNX8 or MADS peptides and measured binding to T cells (Extended Data Fig. 4a ). We detected cross-reactive CD8 + T cells, which bound both peptide epitopes, in all three patients with MIS-C, whereas no cross-reactive CD8 + T cells were observed in at-risk controls (Extended Data Fig. 4b ).
As SNX8-responsive T cells were observed in patients with MIS-C, we next asked whether the region of SNX8 similar to the SARS-CoV-2 MADS region was sufficient to activate patient T cells. A pool of 20 10-mer peptides with 9-amino acid overlaps centred on the target motif from SNX8 (collectively spanning amino acids 44–72) was used to stimulate PBMCs from two patients with MIS-C and four at-risk controls. Both patients with MIS-C had activation of T cells, whereas none of the four controls had T cell activation (Extended Data Fig. 4c ).
Identification of ex vivo cross-reactive T cell receptors
Having determined that patients with MIS-C, but not controls, contained putative SNX8/MADS cross-reactive CD8 + T cells, we next sought to identify T cell receptor (TCR) sequences with specificity for both the SARS-CoV-2 MADS and the host SNX8 epitopes. To do this, PBMCs were obtained during the first 72 h of hospital admission from four study participants with HLA-A*02 and confirmed MIS-C (one individual previously identified as having putative cross-reactive T cells, and three new patients). Given that MIS-C PBMCs represent a scarce resource, we chose to expand one aliquot of PBMCs from each of the four participants (distinct from our previous peptide expansion protocol; see Methods ) to maximize the chances of isolating putative cross-reactive TCRs. Although the frequency of ex vivo autoantigen-specific CD8 + T cells are extraordinarily low in peripheral blood, even for bona fide T cell-mediated autoimmune diseases such as T1DM 38 and multiple sclerosis 44 , 45 , we nevertheless utilized the remaining PBMCs from each participant for direct ex vivo analysis without previous expansion. To isolate the antigen-specific TCRs, participant cells (both ex vivo and following peptide expansion) were stained using the same tetramer-labelling strategy, which previously identified the putative cross-reactive TCRs (Extended Data Fig. 4a ); any cell exhibiting binding to at least two peptide-loaded tetramers was individually sorted and full-length paired TCRα and TCRβ sequences were determined. This resulted in 259 complete TCR sequences, comprising 30 and 18 unique T cell clones from the ex vivo and peptide expansion experiments, respectively. A complete list of TCR sequences is provided (Fig. 4 source data).
Next, we sought to validate the specificity of putative SNX8/MADS cross-reactive TCRs identified from the tetramer sorting, and further analyse features of the recovered TCRs. Because clusters of similar TCRs tend to recognize similar peptide antigens, a TCR similarity network was constructed from all 259 full-length TCR sequences using a previously established TCR distance metric (TCRdist) 46 , 47 (Fig. 4b and Extended Data Fig. 4d ). In two of the four patients, we identified unique populations of clonally expanded T cells expressing putative cross-reactive TCRs directly ex vivo, whereas each of the four patients had at least one ex vivo putative cross-reactive TCR (Fig. 4b ). To confirm the specificity of the TCRs identified in our tetramer sorting, we selected eight TCR sequences for additional validation and generated individual cell lines that stably expressed one TCR of interest (Extended Data Fig. 5a ). These Jurkat-TCR + cell lines were tetramer stained, and cross-reactivity was confirmed in three of the Jurkat-TCR + cell lines (TCR 1, 7 and 8; Fig. 4c ). Of these validated cross-reactive TCRs, two were obtained from ex vivo PBMCs from patients with MIS-C including TCR 7, which was clonally expanded. The minimum ex vivo frequency of TCR 7 alone was more than 1 in 25,000 (6 of 140,035) circulating CD8 + T cells. The two cross-reactive TCRs obtained from the ex vivo isolation were derived from the same participant, utilize the same TRAV gene ( TRAV1-2 ) with identical CDR3α sequences and clustered with three additional sequences in the TCRdist space, one of which was also clonally expanded, suggesting that this patient had an active expansion of a large cluster of SNX8/MADS cross-reactive CD8 + T cells (Fig. 4d ). Furthermore, we note a cluster of two similar TCRs obtained from ex vivo sampling of different participants (patients 2 and 4) with different HLA types (‘convergent node’; circled in green in Fig. 4b ). Although these putative cross-reactive TCRs were not evaluated further, the cluster suggests that TCR specificities to these epitopes may converge across individuals.
The remaining five Jurkat-TCR + cell lines (TCR 2–6) exhibited single specificity to the MADS tetramer with four of five coming from the peptide expansion. To evaluate possible interference between tetramers, which can arise when pHLA–TCR-binding affinities differ, Jurkat-TCR + cell lines were stained with individual tetramers. The results confirm that four of these TCRs are indeed reactive only to MADS (Extended Data Fig. 5b ). However, TCR 2, although showing strong binding preference to MADS, also bound the individual SNX8 tetramer, suggesting that the higher affinity for MADS may outcompete binding to the SNX8 tetramer in some cases. This observation is in line with the notion that autoreactive cross-reactive TCRs with lower relative affinities to autoantigens may escape thymic negative selection. Finally, because the original tetramer experiments were based on an early 2020 SARS-CoV-2 minor variant sequence (LQLPQGITL), all eight Jurkat-TCR + cell lines were also stained with HLA tetramers loaded with the SARS-CoV-2 Wuhan MADS sequence (LQLPQGTTL). In all cases, the Jurkat-TCR + cells bound the Wuhan MADS tetramer, consistent with the notion that T cells encoding these and other similar TCRs may be capable of responding to multiple SARS-CoV-2 strains (Extended Data Fig. 5c ).
RNA expression profile of SNX8 during SARS-CoV-2 infection
As previously discussed, SNX8 is expressed across multiple tissues, but is highest in immune cells, consistent with its role in defending against RNA viruses via recruitment of MAVS 27 . To further investigate the potential impact of combined B cell and T cell autoimmunity to SNX8 following SARS-CoV-2 infection, we used scRNA-seq to analyse SNX8 expression in PBMCs from patients with severe, mild or asymptomatic SARS-CoV-2 infection or influenza infection and uninfected healthy controls 48 . Following SARS-CoV-2 infection, SNX8 had the highest mean expression in classical and non-classical monocytes and B cells (Extended Data Fig. 6a,b ) and was elevated in individuals infected with SARS-CoV-2 compared with those who were uninfected (Extended Data Fig. 6c ). Within myeloid lineage cells, SNX8 expression correlated with MAVS expression and OAS1 and OAS2 (which encode two known regulators of the MAVS pathway implicated in MIS-C pathogenesis 7 ) expression (Extended Data Fig. 6d ). Conversely, SNX8 expression is inversely correlated to SARS-CoV-2 infection severity. This follows a similar pattern to OAS1 and OAS2 . However, unlike OAS1 , OAS2 and MAVS , SNX8 is preferentially expressed during SARS-CoV-2 infection compared with influenza virus infection (Extended Data Fig. 6e ).
The SARS-CoV-2 pandemic largely spared children from severe disease. One rare but notable exception is MIS-C, an enigmatic and life-threatening syndrome. Previous studies have surfaced numerous associations, but have failed to identify a direct mechanistic link between SARS-CoV-2 and MIS-C. In this study, 199 samples from patients with MIS-C and 45 paediatric at-risk controls were analysed using customized human and SARS-CoV-2 proteome PhIP-seq libraries. Targeted follow-up experiments from these assays ultimately revealed that patients with MIS-C preferentially had antibodies targeting the epitope motif (ML)Q(ML)PQG shared by both the SARS-CoV-2 nucleocapsid protein and the human protein SNX8. Cross-reactive CD8 + T cells targeting both regions were detected in patients with MIS-C, but not in controls, suggesting that these CD8 + T cells may contribute to immune dysregulation through the inappropriate targeting of immune cells expressing SNX8. We found evidence that the (ML)Q(ML)PQG epitope motif elicits both B cell and T cell reactivity; further study of this epitope convergence is warranted.
These findings help to connect several important known aspects of MIS-C pathophysiology and draw parallels to other diseases in which exposure to a new antigen leads to autoimmunity, such as paraneoplastic autoimmune disease or cross-reactive epitopes between Epstein–Barr virus and host proteins in multiple sclerosis 17 , 18 , 19 , 22 , 26 . An expansion of T cells expressing TCRβ variable gene 11-2 ( TRBV11-2 ) has been shown in MIS-C 8 , 15 , 16 ; however, the underlying driver remains unknown. Although we did not observe an overrepresentation of TRBV11-2 in our putative cross-reactive TCR dataset, we did identify two expanded TRBV11-2 + clones ( n = 6 and n = 2) sequenced directly from ex vivo samples. Although SNX8 is a relatively understudied protein, it has been linked to the function and activity of MAVS 27 . Dysregulation of the MAVS antiviral pathway, by inborn errors of immunity, has been shown to underlie certain cases of MIS-C 7 . The most straightforward connection linking MIS-C to SNX8 may be through an inappropriate autoimmune response against tissues with elevated MAVS pathway expression. These results are the first to directly link the initial SARS-CoV-2 infection and the subsequent development of MIS-C. We propose that MIS-C may be the result of multiple uncommon events converging. The initial insult is probably the formation of a combined B cell and T cell response that preferentially targets a particular motif within the MADS region of the SARS-CoV-2 nucleocapsid protein. In a subset of individuals, these B cell and T cell responses cross-react to the self-protein SNX8. This cross-reactive motif has strong binding characteristics for the MIS-C-associated HLA-A*02 (ref. 16 ), further indicating that this may be an important risk factor in the development of MIS-C.
Using conservative criteria (3 s.d. greater than controls by targeted immunoprecipitation of the epitope-containing peptide), at least 17% of sera from patients with MIS-C are autoreactive for SNX8; however, approximately 37% of sera from patients with MIS-C yielded detectable enrichment compared with controls in the entire dataset. Because we only tested for a single epitope target, we are unable to determine the upper limits of the in vivo frequency of cross-reactive CD8 + T cells in patients with MIS-C. Our results suggest that the frequency of these cross-reactive CD8 + T cells is within the range of 1 in 10,000–100,000 CD8 + T cells. This substantially exceeds the frequency of antigen-specific autoreactive CD8 + T cells found in peripheral circulation in bona fide T cell-mediated autoimmune diseases such as T1DM 38 and multiple sclerosis 44 , 45 . Similar to T1DM, the autoreactive and cross-reactive CD8 + T cells in patients with MIS-C may be found at far greater abundance within peripheral tissues known to be affected by the disease 38 . Even accounting for these limitations, our results describe a subset of MIS-C, indicating that other mechanisms probably exist. Antibodies to ERFL are present in many children with MIS-C who do not have autoreactivity to SNX8, and ERFL has a highly similar tissue RNA expression profile as SNX8 (second-most similar among all known proteins; Human Protein Atlas) 36 . If autoreactive T cells to ERFL indeed exist, they would be predicted to engage a nearly identical set of cells and tissues. It is important to also consider that MIS-C prevalence has rapidly decreased as an increasing number of children have developed immunity through vaccination and natural SARS-CoV-2 infection. We speculate that perhaps this could be related to the strong deviation of the anti-SARS-CoV-2 immune response away from the critical MADS region of the nucleocapsid protein that we have identified, to other major epitopes such as those in the spike protein through vaccination and past infection 49 . Supporting this notion is recent CDC surveillance, which noted that more than 80% (92 of 112) of individuals with MIS-C in 2023 were in unvaccinated children (but vaccine eligible), and that the majority of children who developed MIS-C despite previous vaccination probably had waned immunity 50 .
MIS-C is complex, and more work will be required to fully understand this syndrome. The results of this study, and specifically the development of combined cross-reactive B cells and T cells, build on other notable examples of molecular mimicry; however, the mechanisms by which the presence of a cross-reactive epitope forces a break in tolerance remain unclear. Our results shed light on how one post-infectious disease (MIS-C) develops, yielding insights that may help better explain, diagnose and ultimately treat a range of additional conditions associated with infections.
Patients were recruited through the prospectively enrolling multicentre Overcoming COVID-19 and Taking on COVID-19 Together study in the USA. All patients meeting clinical criteria were included in the study, and therefore no statistical methods were used to predetermine sample size and no blinding or randomization of subjects occurred. The study was approved by the central Boston Children’s Hospital Institutional Review Board (IRB) and reviewed by IRBs of participating sites with CDC IRB reliance. A total of 292 patients consented and were enrolled into one of the following independent cohorts between 1 June 2020 and 9 September 2021: 223 patients hospitalized with MIS-C (199 in the primary discovery cohort and 24 in a separate subsequent validation cohort), 29 patients hospitalized for COVID-19 in either an intensive care or step-down unit (referred to as ‘severe acute COVID-19’ in this study) and 45 outpatients (referred to as ‘at-risk controls’ in this study) post-SARS-CoV-2 infections associated with mild or no symptoms. The demographic and clinical data are summarized in Extended Data Tables 1 – 3 . The 2020 US CDC case definition was used to define MIS-C 51 . All patients with MIS-C had positive SARS-CoV-2 serology results and/or positive SARS-CoV-2 test results by reverse transcriptase quantitative PCR. All patients with severe COVID-19 or outpatient SARS-CoV-2 infections had a positive antigen test or nucleic acid amplification test for SARS-CoV-2. For outpatients, samples were collected from 36 to 190 days after the positive test (median of 70 days after a positive test; interquartile range of 56–81 days). For use as controls in the SARS-CoV-2-specific PhIP-seq, plasma from 48 healthy, pre-COVID-19 controls were obtained as deidentified samples from the New York Blood Center. These samples were part of retention tubes collected at the time of blood donations from volunteer donors who provided informed consent for their samples to be used for research.
DNA oligomers for SLBAs
DNA coding for the desired peptides for use in SLBAs were inserted into split luciferase constructs containing a terminal HiBiT tag and synthesized (Twist Biosciences) as DNA oligomers and verified by Twist Biosciences before shipment. Constructs were amplified by PCR using the 5′- AAGCAGAGCTCGTTTAGTGAACCGTCAGA-3′ and 5′-GGCCGGCCGTTTAAACGCTGATCTT-3′ primer pair.
For SNX8, the oligomers coded for the following sequences:
EADPPASDLPTPQAIEPQAIVQQVPAPSRMQMPQGNPLLLSHTLQELLA
AAAAAAAAAATPQAIEPQAIVQQVPAPSRMQMPQGNPLLLSHTLQELLA
EADPPAAAAAAAAAAEPQAIVQQVPAPSRMQMPQGNPLLLSHTLQELLA
EADPPASDLPAAAAAAAAAAVQQVPAPSRMQMPQGNPLLLSHTLQELLA
EADPPASDLPTPQAIAAAAAAAAAAAPSRMQMPQGNPLLLSHTLQELLA
EADPPASDLPTPQAIEPQAIAAAAAAAAAAQMPQGNPLLLSHTLQELLA
EADPPASDLPTPQAIEPQAIVQQVPAAAAAAAAAANPLLLSHTLQELLA
EADPPASDLPTPQAIEPQAIVQQVPAPSRMAAAAAAAAAASHTLQELLA
EADPPASDLPTPQAIEPQAIVQQVPAPSRMQMPQGAAAAAAAAAAELLA
EADPPASDLPTPQAIEPQAIVQQVPAPSRMQMPQGNPLLLAAAAAAAAA
For SARS-CoV-2 nucleocapsid protein, the oligomers coded for the following sequences:
ATEGALNTPKDHIGTRNPANNAAIVLQLPQGTTLPKGFYAEGSRGGSQA
AAAAAAAAAADHIGTRNPANNAAIVLQLPQGTTLPKGFYAEGSRGGSQA
ATEGAAAAAAAAAAARNPANNAAIVLQLPQGTTLPKGFYAEGSRGGSQA
ATEGALNTPKAAAAAAAAAANAAIVLQLPQGTTLPKGFYAEGSRGGSQA
ATEGALNTPKDHIGTAAAAAAAAAALQLPQGTTLPKGFYAEGSRGGSQA
ATEGALNTPKDHIGTRNPANAAAAAAAAAAGTTLPKGFYAEGSRGGSQA
ATEGALNTPKDHIGTRNPANNAAIVAAAAAAAAAAKGFYAEGSRGGSQA
ATEGALNTPKDHIGTRNPANNAAIVLQLPQAAAAAAAAAAEGSRGGSQA
ATEGALNTPKDHIGTRNPANNAAIVLQLPQGTTLPAAAAAAAAAAGSQA
ATEGALNTPKDHIGTRNPANNAAIVLQLPQGTTLPKGFYAAAAAAAAAA
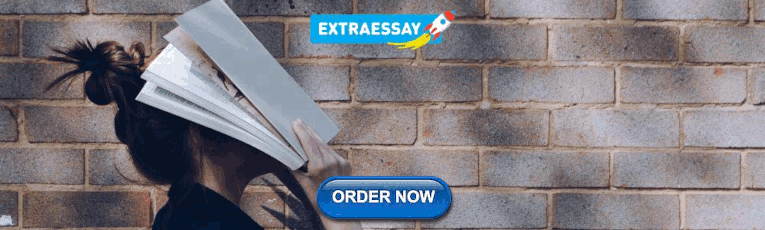
DNA plasmids for RLBAs
For RLBAs, DNA expression plasmids under control of a T7 promoter and with a terminal Myc–DDK tag for the desired protein were utilized. For ERFL, a custom plasmid was ordered from Twist Bioscience in which a Myc–DDK-tagged full-length ERFL sequence under a T7 promoter was inserted into the pTwist Kan High Copy Vector (Twist Bioscience). Twist Bioscience verified a sequence-perfect clone by next-generation sequencing before shipment. Upon receipt, the plasmid was sequence verified by Primordium Labs. For SNX8, a plasmid containing the Myc–DDK-tagged full-length human SNX8 under a T7 promoter was ordered from Origene (RC205847) and was sequence verified by Primordium Labs upon receipt. For KDELR1, a plasmid containing the Myc–DDK-tagged full-length human KDELR1 under a T7 promoter was ordered from Origene (RC205880) and was sequence verified by Primordium Labs upon receipt. For IL1RN, a plasmid containing the Myc–DDK-tagged full-length human IL1RN under a T7 promoter was ordered from Origene (RC218518) and was sequence verified by Primordium Labs upon receipt.
Polypeptide pools for activation-induced marker assays
To obtain polypeptides tiling the full-length SNX8 protein, 15-mer polypeptide fragments with 11-amino acid overlaps were ordered from JPT Peptide Technologies and synthesized. Together, a pool of 130 of these polypeptides (referred to as the ‘SNX8 pool’) spanned all known translated SNX8 (the full-length 465-amino acid SNX8 protein, as well as a unique region of SNX8 isoform 3). A separate pool was designed to cover primarily the region of SNX8 with similarity to the SARS-CoV-2 nucleocapsid protein in high resolution (referred to as the ‘high-resolution epitope pool’). This pool contained 20 10-mers with 9-amino acid overlaps tiling amino acids 44–72 (IVQQVPAPSRMQMPQGNPLLLSHTLQELL) of the full-length SNX8 protein. The sequence of each of these 150 polypeptides was verified by mass spectrometry and purity was calculated by high-performance liquid chromatography (HPLC).
Peptides for tetramer assays
For use in loading tetramers, three peptides were ordered from Genemed Synthesis as 9-mers. LQLPQGTTL and LQLPQGITL correspond to the region of the SARS-CoV-2 nucleocapsid protein with similarity to human SNX8 in the ancestral sequence and a minor variant, respectively. This sequence was verified by mass spectrometry and purity was calculated as 96.61% by HPLC. The other sequence, MQMPQGNPL, corresponds to the region of human SNX8 protein with similarity to the SARS-CoV-2 nucleocapsid protein. This sequence was verified by mass spectrometry and purity was calculated as 95.83% by HPLC.
Human proteome PhIP-seq
Human proteome PhIP-seq was performed following our previously published vacuum-based PhIP-seq protocol 12 ( https://www.protocols.io/view/scaled-high-throughput-vacuum-phip-protocol-ewov1459kvr2/v1 ).
Our human peptidome library consists of a custom-designed phage library of 731,724 unique T7 bacteriophage each presenting a different 49-amino acid peptide on its surface. Collectively, these peptides tile the entire human proteome including all known isoforms (as of 2016) with 25-amino acid overlaps. Of the phage library, 1 ml was incubated with 1 μl of human serum overnight at 4 °C and immunoprecipitated with 25 μl of 1:1 mixed protein A and protein G magnetic beads (10008D and 10009D, Thermo Fisher). These beads were than washed, and the remaining phage–antibody complexes were eluted in 1 ml of Escherichia coli (BLT5403, EMD Millipore) at 0.5–0.7 OD and amplified by growing in a 37 °C incubator. This new phage library was then re-incubated with the serum from the same individual and the previously described protocol was repeated. DNA was then extracted from the final phage library, barcoded, PCR amplified and Illumina adaptors were added. Next-generation sequencing was performed using an Illumina sequencer (Illumina) to a read depth of approximately 1 million per sample.
Human proteome PhIP-seq analysis
All human peptidome analysis (except when specifically stated otherwise) was performed at the gene level, in which all reads for all peptides mapping to the same gene were summed, and 0.5 reads were added to each gene to allow inclusion of genes with zero reads in mathematical analyses. Within each individual sample, reads were normalized by converting to the percentage of total reads. To normalize each sample against background nonspecific binding, a fold change over mock-IP was calculated by dividing the sample read percentage for each gene by the mean read percentage of the same gene for the AG bead-only controls. This fold-change signal was then used for side-by-side comparison between samples and cohorts. Fold-change values were also used to calculate z scores for each patient with MIS-C compared with controls and for each control sample by using all remaining controls. These z scores were used for the logistic-regression feature weighting. In instances of peptide-level analysis, raw reads were normalized by calculating the number of reads per 100,000 reads.
SARS-CoV-2 proteome PhIP-seq
SARS-CoV-2 proteome PhIP-seq was performed as previously described 39 . In brief, 38 amino acid fragments tiling all open reading frames from SARS-CoV-2, SARS-CoV-1 and 7 other CoVs were expressed on T7 bacteriophage with 19-amino acid overlaps. Of the phage library, 1 ml was incubated with 1 μl of human serum overnight at 4 °C and immunoprecipitated with 25 μl of 1:1 mixed protein A and protein G magnetic beads (10008D and 10009D, Thermo Fisher). Beads were washed five times on a magnetic plate using a P1000 multichannel pipette. The remaining phage–antibody complexes were eluted in 1 ml of E. coli (BLT5403, EMD Millipore) at 0.5–0.7 OD and amplified by growing in 37 °C incubator. This new phage library was then re-incubated with the serum of the same individual and the previously described protocol was repeated for a total of three rounds of immunoprecipitations. DNA was then extracted from the final phage library, barcoded, PCR amplified and Illumina adaptors were added. Next-generation sequencing was then performed using an Illumina sequencer (Illumina) to a read depth of approximately 1 million per sample.
Coronavirus proteome PhIP-seq analysis
To account for differing read depths between samples, the total number of reads for each peptide fragment was converted to the number of reads per 100,000 (RPK). To calculate normalized enrichment relative to pre-COVID-19 controls (FC > pre-COVID-19), the RPK for each peptide fragment within each sample was divided by the mean RPK of each peptide fragment among all pre-COVID-19 controls. These FC > pre-COVID-19 values were used for all subsequent analyses as described in the text and figures.
RLBAs were performed as previously described 12 , 32 . In brief, DNA plasmids containing full-length cDNA under the control of a T7 promoter for each of the validated antigens (see ‘DNA plasmids for RLBAs’ above) were verified by Primordium Labs sequencing. The respective DNA templates were used in the T7 TNT in vitro transcription/translation kit (L1170, Promega) using [35S]-methionine (NEG709A, PerkinElmer). Respective protein was column purified on Nap-5 columns (17-0853-01, GE Healthcare), and equal amounts of protein (approximately 35,000 counts per minute) were incubated overnight at 4 °C with 2.5 μl of serum or 1 μl of anti-Myc-positive control antibody (1:10 dilution; 2272S, Cell Signaling Technology). Immunoprecipitation was then performed on 25 μl of Sephadex protein A/G beads (4:1 ratio; GE17-5280-02 and GE17-0618-05, Sigma-Aldrich) in 96-well polyvinylidene difluoride filtration plates (EK-680860, Corning). After thoroughly washing, the counts per minute of immunoprecipitated protein was quantified using a 96-well Microbeta Trilux liquid scintillation plate reader (Perkin Elmer).
SLBA was performed as previously described 52 . A detailed SLBA protocol is available on protocols.io ( https://doi.org/10.17504/protocols.io.4r3l27b9pg1y/v1 ).
In brief, the DNA oligomers listed above (see ‘DNA oligomers for SLBAs’) were amplified by PCR using the primer pairs listed above (see ‘DNA oligomers for SLBAs’). Unpurified PCR product was used as input in the T7 TNT in vitro transcription/translation kit (L1170, Promega) and the Nano-Glo HiBit Lytic Detection System (N3040, Promega) was used to measure relative luciferase units of translated peptides in a luminometre. Equal amounts of protein (in the range of 2 × 10 6 –2 × 10 7 relative luciferase units) were incubated overnight with 2.5 μl patient sera or 1 μl anti-HiBit-positive control antibody (1:10 dilution; CS2006A01, Promega) at 4 °C. Immunoprecipitation was then performed on 25 µl of Sephadex protein A/G beads (1:1 ratio; GE17-5280-02 and GE17-0618-05, Sigma-Aldrich) in 96-well polyvinylidene difluoride filtration plates (EK-680860, Corning). After thoroughly washing, luminescence was measured using the Nano-Glo HiBit Lytic Detection System (N3040, Promega) in a luminometre.
Activation-induced marker assay
PBMCs were obtained from ten patients with MIS-C and ten controls for use in the activation-induced marker assay. PBMCs were thawed, washed, resuspended in serum-free RPMI medium and plated at a concentration of 1 × 10 6 cells per well in a 96-well round-bottom plate. For each individual, PBMCs were stimulated for 24 h with either the SNX8 pool (see above) at a final concentration of 1 mg ml −1 per peptide in 0.2% DMSO or a vehicle control containing 0.2% DMSO only. For four of the controls and two of the patients with MIS-C, there were sufficient PBMCs for an additional stimulation condition using the SNX8 high-resolution epitope pool (see above) also at a concentration of 1 mg ml −1 per peptide in 0.2% DMSO for 24 h. Following the stimulation, cells were washed with FACS buffer (Dulbecco’s PBS without calcium or magnesium, 0.1% sodium azide, 2 mM EDTA and 1% FBS) and stained with the following antibody panel each at 1:100 dilution for 20 min at 4 °C, and then flow cytometry analysis was immediately performed.
For the antibody panel: anti-CD3 Alexa 647 (clone OKT3, 317312, BioLegend), anti-CD4 Alexa 488 (clone OKT4, 317420, BioLegend), anti-CD8 Alexa 700 (clone SK1, 344724, BioLegend), anti-OX-40 (also known as CD134) PE-Dazzle 594 (clone ACT35, 350020, BioLegend), anti-CD69 PE (clone FN-50, 310906, BioLegend), anti-CD137 (also known as 4-1BB) BV421 (clone 4B4-1, 309820, BioLegend), anti-CD14 PerCP-Cy5 (clone HCD14, 325622, BioLegend), anti-CD16 PerCP-Cy5 (clone B73.1, 360712, BioLegend), anti-CD19 PerCP-Cy5 (clone HIB19, 302230, BioLegend) and Live/Dead Dye eFluor 506 (65-0866-14, Invitrogen).
The activation-induced marker analysis was performed using FlowJo software using the gating strategy shown in Extended Data Fig. 7a . All gates were fixed within each condition of each sample. Activated CD4 T cells were defined as those that were co-positive for OX40 and CD137. Activated CD8 T cells were defined as those that were co-positive for CD69 and CD137. Gating thresholds for activation were defined by the outer limits of signal in the vehicle controls allowing for up to two outlier cells. Frequencies were calculated as a percentage of total CD3 + cells (T cells). Two MIS-C samples had insufficient total events captured by flow cytometry (total of 5,099 and 4,919 events, respectively) and were therefore removed from analysis.
Initial tetramer assay
For the initial tetramer assay, see Extended Data Fig. 4a . PBMCs from two patients with MIS-C with HLA-A*02:01 (HLA typed from PAXgene RNAseq, one confirmed by serotyping), one patient with MIS-C with HLA-B*35:01 (HLA typed from PAXgene RNAseq) and three at-risk controls with HLA-A*02.01 (all three identified by serotyping, two of three confirmed by PAXgene RNAseq HLA typing; the other sample did not have genomic DNA available for genotyping) were thawed, washed and put into culture with media containing recombinant human IL-2 at 10 ng ml −1 in 96-well plates. The peptide fragments (details above) LQLPQGITL and MQMPQGNPL were then added to PBMCs to a final concentration of 10 mg ml −1 per peptide and incubated (37 °C at 5% CO 2 ) for 7 days.
Following the 7 days of incubation, a total of eight pHLA class I tetramers were generated from UV-photolabile biotinylated monomers, four each from HLA-A*02:01 and HLA-B*35:01 (NIH Tetramer Core). Peptides were loaded via UV peptide exchange. Tetramerization was carried out using streptavidin conjugated to fluorophores PE and APC or BV421 followed by quenching with 500 µM d -biotin, similar to our previously published methods 44 , 53 . Tetramers were then pooled together as shown below:
For the HLA-A*02:01 pool, the MADS (LQLPQGITL)-loaded PE tetramer, MADS (LQLPQGITL)-loaded APC tetramer, SNX8 (MQMPQGNPL)-loaded PE tetramer and SNX8 (MQMPQGNPL)-loaded BV421 tetramer were used, all with HLA-A*02:01 restriction.
For the HLA-B*35:01 pool, the MADS (LQLPQGITL)-loaded PE tetramer, MADS (LQLPQGITL)-loaded APC tetramer, SNX8 (MQMPQGNPL)-loaded PE tetramer and SNX8 (MQMPQGNPL)-loaded BV421 tetramer were used, all with HLA-B*35:01 restriction.
All PBMCs were then treated with 100 nM dasatinib (StemCell) for 30 min at 37 °C followed by staining (no wash step) with the respective tetramer pool corresponding to their HLA restriction (final concentration of 2–3 µg ml −1 ) for 30 min at 25 °C. Cells were then stained with the following cell-surface markers each at 1:100 dilution for 20 min, followed by immediate analysis on a flow cytometer.
For the surface markers: anti-CD8 Alexa 700 (clone SK1, 357404, BioLegend), anti-CD4 PerCP-Cy5 (clone SK1, 300530, BioLegend), anti-CD14 PerCP-Cy5 (clone HCD14, 325622, BioLegend), anti-CD16 PerCP-Cy5 (clone B73.1, 360712, BioLegend), anti-CD19 PerCP-Cy5 (clone HIB19, 302230, BioLegend) and Live/Dead Dye eFluor 506 (65-0866-14, Invitrogen). Streptavidin was conjugated to PE (S866, Invitrogen), APC (S868, Invitrogen) and BV421 (405225, BioLegend).
The gating strategy is outlined in Extended Data Fig. 7b . A stringent tetramer gating strategy was used to identify cross-reactive T cells, in which CD8 + T cells were required to be triple positive for PE, APC and BV421 labels (that is, a single CD8 T cell bound to PE-conjugated LQLPQGITL and/or PE-conjugated MQMPQGNPL in addition to APC-conjugated LQLPQGITL and BV421-conjugated MQMPQGNPL).
Serotyping was performed using an anti-HLA-A2 antibody (1:100 dilution; FITC anti-human HLA-A2 antibody, clone BB7.2, 343303, BioLegend), and pertinent results are shown in Extended Data Fig. 7c .
Assembly of easYmer monomers and fold testing
For the assembly of HLA class I pHLA easYmer monomers and fold testing, see Fig. 4 . Unfolded, biotinylated easYmer monomers (Immudex) were obtained for HLA-A*02:01 and HLA-A*02:06. SARS-CoV-2 MADS (LQLPQGITL), SARS-CoV-2 Wuhan (LQLPQGTTL) and human SNX8 (MQMPQGNPL) peptides were commercially synthesized (Genscript), diluted to 1 mM in ddH 2 O or DMSO, and loaded onto each easYmer allele according to the manufacturer’s instructions at 18 °C for 48 h. Proper pHLA monomer formation and MADS and SNX8 peptide-binding strength were evaluated for each HLA using a ‘β2m fold test’ relative to negative (no peptide; unloaded monomer) and positive (strong binding peptide; CMV pp65 495–503 (NLVPMVATV)) controls as per the manufacturer’s protocol. In brief, peptide-loaded monomers with a concentration of 500 nM were serially diluted to 9 nM, 3 nM and 1 nM in dilution buffer (1× PBS with 5% glycerol; G5516, Sigma-Aldrich) and incubated with streptavidin beads (6–8 μm; SVP-60-5, Spherotech) at 37 °C for 1 h to allow binding of stable complexes to beads, then washed three times with FACS buffer (1× PBS, 0.5% BSA (A7030, Sigma-Aldrich) and 2 mM EDTA (15575-038, Thermo Fisher Scientific)). Samples were then stained with PE-conjugated anti-human β2m antibody (clone BBM.1, sc-13565, Santa Cruz Biotech) at 1:200 for 30 min at 4 °C, washed three times with FACS buffer and analysed on a 5 Laser 16UV-16V-14B-10YG-8R AURORA spectral cytometer (Cytek). pHLA-binding strength positively correlated with stability and concentration of the pHLA–β2m complex. Therefore, the geometric mean fluorescence intensity of anti-β2m staining in this assay reports on the strength of the pHLA binding compared with the positive and negative controls. We classified binding strength for each HLA and peptide combination based on the fold change in anti-β2m geometric mean fluorescence intensity over the no-peptide negative control at 9 nM. Strong binders were defined at more than 10-fold higher, moderate binders at more than 3-fold, weak binders at more than 1.5-fold and non-binders at less than 1.5-fold change over the negative control. Flow cytometry data were analysed using FlowJo version 10.7.2 software (BD Biosciences).
pHLA tetramer assembly
For the pHLA tetramer assembly, see Fig. 4 . pHLA tetramers were assembled from HLA-A*02:01 and HLA-A*02:06 easYmer monomers (Immudex) with confirmed peptide binding to SARS-CoV-2 MADS (LQLPQGITL), Wuhan (LQLPQGTTL) and SNX8 (MQMPQGNPL) peptides according to the manufacturer’s instructions. In brief, fluorochrome-conjugated streptavidin (0.2 mg ml −1 , PE, 405203, BioLegend; 0.2 mg ml −1 , APC, 405207, BioLegend; and BV421, 405226, BioLegend) was added to loaded monomers at 8 ng per 1 μl pHLA complex (500 nM) in three volumes. After each 1/3 volume addition, samples were mixed and incubated for 15 min at 4 °C in the dark. Assembled tetramers were stored at 4 °C in the dark until use.
Enhanced peptide-specific T cell expansion
For enhanced peptide-specific T cell expansion, see Fig. 4 . PBMCs from MIS-C confirmed participants with HLA-A*02:01 or HLA-A*02:06 were obtained for peptide-specific expansion according to published methods 54 before single-cell sorting of tetramer-positive T cells. On expansion day 0, PBMCs were thawed, counted and seeded onto 96-well round-bottom plates at 100,000 cells per well in 200 μl antigen-presenting cell differentiation media (X-VIVO 15 serum-free haematopoietic cell medium (04-418Q, Lonza) supplemented with human GM-CSF (1,000 IU ml −1 ; 130-095-372, Miltenyi Biotec), human IL-4 (500 IU ml −1 ; 204-IL-010, R&D Systems) and human Flt3-L (50 ng ml −1 ; 308-FKN-025, R&D Systems) final concentrations) and incubated for 24 h at 37 °C and 5% CO 2 . On day 1, 100 μl cell supernatant was replaced with 100 μl Adjuvant Solution (X-VIVO 15 supplemented with R848 (10 μM; tlrl-r848-5, InvivoGen), lipopolysaccharide ( Salmonella minnesota ; 100 ng ml −1 ; tlrl-smlps, InvivoGen) and human IL-1β (10 ng ml −1 ; 201-LB-010, R&D Systems) final concentrations) and pooled MADS (LQLPQGITL) and SNX8 (MQMPQGNPL) peptides at a final concentration of 10 μM each. No-peptide control wells were set up for each sample by adding a 1:2 dilution of DMSO in H 2 O to match the peptide volume and diluent. Cells were incubated for 24 h at 37 °C and 5% CO 2 . On days 2, 4, 7 and 9, 100 μl supernatant was replaced with 100 μl T cell expansion solution: RP-10 (RPMI 1640 (22400-089, Gibco), 10% heat-inactivated human serum AB (100-512, Gemini Bio-Products), 10 mM HEPES, 0.1 mg ml −1 gentamicin (15750-060, Thermo Fisher Scientific) and 1× GlutaMAX (35050-061, Gibco)) supplemented with human IL-2 (10 IU ml −1 ; 202-IL-050, R&D Systems), human IL-7 (10 ng ml −1 ; 207-IL-025, R&D Systems) and human IL-15 (10 ng ml −1 ; 200-15, PeproTech) final concentrations. On day 10, peptide-expanded cells from an individual participant were pooled; cells from no-peptide controls were collected separately.
Single-cell index sorting
Unexpanded PBMCs (direct ex vivo) or peptide-expanded T cells were obtained, washed in 1× PBS and treated with 100 nM dasatinib (CDS023389, Sigma-Aldrich) in 1× PBS for 30 min at 37 °C and 5% CO 2 (ref. 55 ). Cells were then pelleted and resuspended in 50 μl FACS buffer (1× PBS and 0.04% BSA) supplemented with human TruStain FcX blocking buffer (1:10 dilution; 422302, BioLegend), 500 μM d -biotin (B20656, Thermo Fisher Scientific) and a unique tetramer cocktail containing MADS–tetramer–PE (1:10 dilution), MADS–tetramer–APC (1:10 dilution), SNX8–tetramer–PE (1:10 dilution) and SNX8–tetramer–BV421 (1:10 dilution) based on participant HLA type (A*02:01 and A*02:06). Cells were incubated in the dark at 25 °C for 1 h followed by direct addition of 50 μl (100 μl total volume) of FACS supplemented with 500 μM d -biotin and an antibody cocktail containing FITC-conjugated anti-human CD3 (1:20 dilution; clone OKT3, lot B390808, 317306, BioLegend), BV605-conjugated anti-human CD8 (1:20 dilution; clone SK1, lot B371925, 344742, BioLegend), BV510-conjugated anti-human CD4 (1:20 dilution; clone OKT4, lot B375526, 317444, BioLegend), BV510-conjugated anti-human CD14 (1:20 dilution; clone 63D3, lot B390770, 367124, BioLegend), BV510-conjugated anti-human CD16 (1:20 dilution; clone 3G8, lot B372132, 302048, BioLegend), BV510-conjugated anti-human CD19 (1:20 dilution; clone HIB19, lot B390665, 302242, BioLegend) and Ghost Dye Violet 510 Viability Dye (1:400 dilution; lot D0870061322133, 13-0870-T500, Tonbo Biosciences) for 30 min in the dark at 4 °C. Cells were then pelleted, washed twice with 4 ml FACS buffer (containing 500 μM d -biotin), suspended in 500 μl FACS (containing 500 μM d -biotin) and passed through a 45-μM filter before proceeding to single-cell sorting on a Sony SY3200 cell sorter. Individual, live, BV510 dump gate (CD4, CD14, CD16 and CD19)-negative, CD3 + CD8 + T lymphocytes were gated to distinguish tetramer triple-positive cells (PE + APC + BV421 + ) as described in Extended Data Fig. 7d and sorted into individual wells of a 384-well plate loaded with Superscript VILO master mix (11754250, Thermo Fisher Scientific). After sorting, plates were centrifuged at 500 g and stored at −80 °C until processing.
Paired TCRαβ amplification and sequencing
Single-cell paired TCRα and TCRβ chain library preparation and sequencing was performed on T cells sorted into 384-well index plates as previously described 56 . In brief, after reverse transcription of cells sorted in Superscript VILO master mix, cDNA underwent two rounds of nested multiplex PCR amplification using a mix of human V-segment-specific forward primers and human TRAC and TRBC segment-specific reverse primers (see Supplementary Table 1 for primer details). Resulting TCRα and TCRβ amplicons were sequenced on an Illumina MiSeq at 2 × 150-bp read length.
All cultured cell lines were maintained at 37 °C and 5% CO 2 in a humidified incubator. HEK 293T cells (CRL-3216, American Type Culture Collection) were purchased from the American Type Culture Collection and verified commercially. HEK 293T cells were cultured in DMEM (11965-092, Gibco) supplemented with 10% FBS (16140-071, Gibco), 2 mM l -glutamine (25030-081, Gibco) and 100 U ml −1 penicillin–streptomycin (15140-122, Gibco). 2D3 Jurkat J76.7 cells 57 , 58 (TCR-null, CD8 + ) expressing an NFAT–eGFP reporter were kindly provided by F. Fujiki and were cultured in RPMI 1640 (22400-089, Gibco) supplemented with 10% FBS, 2 mM l -glutamine and 100 U ml −1 penicillin–streptomycin. All cell lines were confirmed to be mycoplasma negative during the course of experiments.
TCR repertoire analysis
TCR similarity networks were constructed as previously described 49 , 59 . In brief, to measure the distance between TCRαβ clonotypes, we used the TCRdist algorithm implementation from the CoNGA v0.1.2 Python package 47 . Further analysis was performed using the R language for statistical computing, with merging and subsetting of data performed using the dplyr v1.1.4 package. TCR similarity networks were built using stringdist v0.9.12 and igraph v2.0.3 (ref. 60 ) R packages, and visualized using gephi v0.9.7 (ref. 61 ) software.
TCR reconstruction and cloning
Full-length TCRαβ sequences were reconstructed from V/J gene usage and CDR3 sequences using Stitchr v1.0.0 (ref. 62 ) for each index-sorted T cell. TCRα and TCRβ chain sequences were modified to use murine constant regions and joined by a 2A element from thosea asigna virus (T2A). A sequence encoding mCherry was additionally appended by a 2A element from porcine teschovirus (P2A) as a fluorescent marker of transduction. The full-length gene fragment encoding TCRβ–T2A–TCRα–P2A–mCherry was synthesized and cloned commercially (Genscript) into the lentiviral vector pLVX-EF1α-IRES-Puro (631253, Takara).
Generation of TCR-expressing Jurkat cells
To generate transducing particles packaging individual TCRs of interest (Fig. 4c ), HEK 293T cells were transduced with a pLVX lentiviral vector encoding a unique TCRαβ–mCherry insert, psPAX2 packaging plasmid (plasmid #12260, Addgene) and an pMD2.G envelope plasmid (plasmid #12259, Addgene) at a ratio of 4:3:1. At 24 h and 48 h post-transfection, viral supernatants were harvested, passed through a 0.45-µm SFCA filter (723-9945, Thermo Fisher Scientific), concentrated using Lenti-X Concentrator (631232, Takara) and stored at −80 °C as single-use aliquots. To generate TCR-expressing Jurkat cell lines (Jurkat-TCR + ), 2D3 Jurkat J76.7 cells (TCR-null, CD8 + , NFAT–eGFP reporter) were seeded in a 12-well tissue-culture-treated plate at 1 × 10 6 cells per well in complete RPMI (RPMI 1640, 10% FBS, 2 mM l -glutamine, 100 U ml −1 penicillin–streptomycin) and transduced by adding concentrated lentivirus dropwise to each well. At 48–72 h post-tranduction, puromycin was added at 1 μg ml −1 and cultured for 1 week to select for transduced cells. Jurkat-TCR + cell lines were validated for the presence of correctly folded TCR on the cell surface by flow cytometry using a monoclonal antibody targeting the mouse TCRβ constant region (APC/Fire750-conjugated; clone H57-597, 109246, BioLegend; Extended Data Fig. 5a ). Flow cytometry data were collected on a custom-configured BD Fortessa using FACSDiva software (v8.0.1; Becton Dickinson) and analysed using FlowJo version 10.7.2 software (BD Biosciences).
Specificity validation of putative cross-reactive TCR sequences
The specificity of TCR-expressing Jurkat T cell lines was validated by tetramer staining using the same reagents used for single-cell sorting PBMCs (above). In brief, 1 × 10 6 Jurkat-TCR + cell lines or untransduced Jurkat J76.7 (TCR-null; background control) were washed in 1× PBS and resuspended in 50 μl FACS buffer (1× PBS and 0.04% BSA) and a unique tetramer cocktail containing MADS–tetramer–PE (1:10 dilution), MADS–tetramer–APC (1:10 dilution), SNX8–tetramer–PE (1:10 dilution) and SNX8–tetramer–BV421 (1:10 dilution) based on the restricting HLA type (A*02:01 and A*02:06). Tetramers conjugated to the Wuhan peptide sequence (LQLPQGTTL), including Wuhan–tetramer–PE (1:10 dilution) and Wuhan–tetramer–APC (1:10 dilution), were also tested. A second set of wells were set up in which each individual tetramer was used to stain cells. Cells were incubated in the dark at 25 °C for 30 min after which 50 µl of FACS buffer containing Ghost Dye Violet 510 Viability Dye (1:400 dilution; lot D0870061322133, 13-0870-T500, Tonbo Biosciences) was added for an additional 30-min incubation in the dark at 25 °C. Cells were then washed twice with 1 ml FACS buffer, suspended in 300 μl FACS and analysed by flow cytometry on a custom-configured BD Fortessa using FACSDiva software (v8.0.1; Becton Dickinson). Cell population gating and fluorescence analysis was performed using FlowJo version 10.7.2 software (BD Biosciences) as described in Extended Data Fig. 7e .
scRNA-seq analysis
To assess the cell-type specificity in a relevant disease context, we analysed SNX8 expression from a single-cell sequencing of PBMC samples from patients with severe, mild or asymptomatic COVID-19 infection, influenza virus infection and healthy controls 48 . Gene expression data from 59,572 pre-filtered cells were downloaded from the Gene Expression Omnibus database under accession GSE149689 for analysis and downstream processing with scanpy v1.10.0 (ref. 63 ). Cells with (1) less than 1,000 total counts, (2) less than 800 expressed genes, and (3) more than 3,000 expressed genes were filtered out as further quality control, leaving 42,904 cells for downstream analysis. Gene expression data were normalized to have 10,000 counts per cell and were log1p transformed. Highly variable genes were calculated using the scanpy function highly_variable_genes using Seurat flavor with the default parameters (min_mean = 0.0125, max_mean = 3, and min_disp = 0.5) 64 . Only highly variable genes were used for further analysis. The total number of counts per cell was regressed out, and the gene expression matrix was scaled using the scanpy function scale with max_value = 10. Dimensionality reduction was performed using principal components analysis with 50 principal components. Batch balanced k -nearest neighbours, implemented with scanpy’s function bbknn, was used to compute the top neighbours and normalize batch effects 65 . The batch-corrected cells were clustered using the Leiden algorithm and projected into two dimensions with uniform manifold approximation and projection for visualization. Initial cluster identity was determined by finding marker genes with differential expression analysis performed using a Student’s t -test on log1p-transformed raw counts with the scanpy function rank_genes_groups 66 , 67 .
Statistical methods
All statistical analysis was performed in Python using the Scipy Stats package unless otherwise indicated. For comparisons of distributions of PhIP-seq enrichment between two groups, a non-parametric Kolmogorov–Smirnov test was utilized. For logistic-regression feature weighting, the Scikit-learn package 68 was used, and logistic-regression classifiers were applied to z -scored PhIP-seq values from individuals with MIS-C versus at-risk controls. A liblinear solver was used with L1 regularization, and the model was evaluated using a five-fold cross-validation (four of the five for training, and one of the five for testing). For the RLBAs and SLBAs, first an antibody index was calculated as follows: (sample value – mean blank value)/(positive control antibody values – mean blank values). For the alanine mutagenesis scans, blank values of each construct were combined, and a single mean was calculated. A normalization function was then applied to the experimental samples only (excluding antibody-only controls) to create a normalized antibody index ranging from 0 to 1. Comparisons between two groups of samples were performed using a Mann–Whitney U -test. An antibody was considered to be ‘positive’ when the normalized antibody index in a sample was greater than 3 s.d. above the mean of controls. When comparing two groups of normally distributed data, a Student’s t -test was performed.
Reporting summary
Further information on research design is available in the Nature Portfolio Reporting Summary linked to this article.
Data availability
The published article includes all datasets generated or analysed as a part of this study. Individual source data are provided with associated figures (where appropriate) per the data-sharing agreement stipulated under the Ruth L. Kirschstein National Research Service Award Individual Postdoctoral Fellowship (award no. F32AI157296 to R.C.M.). Raw flow cytometry source files can be made available on reasonable request. All PhIP-seq data are publicly available via a Dryad digital repository ( https://doi.org/10.7272/Q6SJ1HVH ). Raw TCR reads are available through the NCBI Sequence Read Archive (SRA) BioProject PRJNA1110271 , with associated BioSample accession numbers SAMN41334731 , SAMN41334732 , SAMN41334730 and SAMN41334729 . Source data are provided with this paper.
Lu, X. et al. SARS-CoV-2 infection in children. N. Engl. J. Med. 382 , 1663–1665 (2020).
Article PubMed Google Scholar
Viner, R. M. et al. Susceptibility to SARS-CoV-2 infection among children and adolescents compared with adults: a systematic review and meta-analysis. JAMA Pediatr. 175 , 143–156 (2021).
Feldstein, L. R. et al. Characteristics and outcomes of US children and adolescents with multisystem inflammatory syndrome in children (MIS-C) compared with severe acute COVID-19. JAMA 325 , 1074–1087 (2021).
Article CAS PubMed Google Scholar
Whittaker, E. et al. Clinical characteristics of 58 children with a pediatric inflammatory multisystem syndrome temporally associated with SARS-CoV-2. JAMA 324 , 259–269 (2020).
Article CAS PubMed PubMed Central Google Scholar
Diorio, C. et al. Multisystem inflammatory syndrome in children and COVID-19 are distinct presentations of SARS-CoV-2. J. Clin. Invest. 130 , 5967–5975 (2020).
Carter, M. J. et al. Peripheral immunophenotypes in children with multisystem inflammatory syndrome associated with SARS-CoV-2 infection. Nat. Med. 26 , 1701–1707 (2020).
Lee, D. et al. Inborn errors of OAS-RNase L in SARS-CoV-2-related multisystem inflammatory syndrome in children. Science 379 , eabo3627 (2022).
Article Google Scholar
Porritt, R. A. et al. The autoimmune signature of hyperinflammatory multisystem inflammatory syndrome in children. J. Clin. Invest. 131 , e151520 (2021).
Consiglio, C. R. et al. The immunology of multisystem inflammatory syndrome in children with COVID-19. Cell 183 , 968–981.e7 (2020).
Gruber, C. N. et al. Mapping systemic inflammation and antibody responses in multisystem inflammatory syndrome in children (MIS-C). Cell 183 , 982–995.e14 (2020).
Pfeifer, J. et al. Autoantibodies against interleukin-1 receptor antagonist in multisystem inflammatory syndrome in children: a multicentre, retrospective, cohort study. Lancet Rheumatol. 4 , e329–e337 (2022).
Vazquez, S. E. et al. Autoantibody discovery across monogenic, acquired, and COVID-19-associated autoimmunity with scalable PhIP-seq. eLife 11 , e78550 (2022).
Rybkina, K. et al. SARS-CoV-2 infection and recovery in children: distinct T cell responses in MIS-C compared to COVID-19. J. Exp. Med. 220 , e20221518 (2023).
Vella, L. A. et al. Deep immune profiling of MIS-C demonstrates marked but transient immune activation compared to adult and pediatric COVID-19. Sci. Immunol. 6 , eabf7570 (2021).
Article PubMed PubMed Central Google Scholar
Hoste, L. et al. TIM3 + TRBV11-2 T cells and IFNγ signature in patrolling monocytes and CD16 + NK cells delineate MIS-C. J. Exp. Med. 219 , e20211381 (2022).
Porritt, R. A. et al. HLA class I-associated expansion of TRBV11-2 T cells in multisystem inflammatory syndrome in children. J. Clin. Invest. 131 , e146614 (2021).
Tengvall, K. et al. Molecular mimicry between anoctamin 2 and Epstein–Barr virus nuclear antigen 1 associates with multiple sclerosis risk. Proc. Natl Acad. Sci. USA 116 , 16955–16960 (2019).
Article ADS CAS PubMed PubMed Central Google Scholar
Lanz, T. V. et al. Clonally expanded B cells in multiple sclerosis bind EBV EBNA1 and GlialCAM. Nature 603 , 321–327 (2022).
Thomas, O. G. et al. Cross-reactive EBNA1 immunity targets α-crystallin B and is associated with multiple sclerosis. Sci. Adv. 9 , eadg3032 (2023).
Blachère, N. E. et al. T cells targeting a neuronal paraneoplastic antigen mediate tumor rejection and trigger CNS autoimmunity with humoral activation. Eur. J. Immunol. 44 , 3240–3251 (2014).
Roberts, W. K. & Darnell, R. B. Neuroimmunology of the paraneoplastic neurological degenerations. Curr. Opin. Immunol. 16 , 616–622 (2004).
Roberts, W. K. et al. Patients with lung cancer and paraneoplastic Hu syndrome harbor HuD-specific type 2 CD8 + T cells. J. Clin. Invest. 119 , 2042–2051 (2009).
CAS PubMed PubMed Central Google Scholar
Darnell, R. B. & Posner, J. B. Paraneoplastic syndromes involving the nervous system. N. Engl. J. Med. 349 , 1543–1554 (2003).
O’Donovan, B. et al. High-resolution epitope mapping of anti-Hu and anti-Yo autoimmunity by programmable phage display. Brain Commun. 2 , fcaa059 (2020).
Mandel-Brehm, C. et al. Kelch-like protein 11 antibodies in seminoma-associated paraneoplastic encephalitis. N. Engl. J. Med. 381 , 47–54 (2019).
Dubey, D. et al. Expanded clinical phenotype, oncological associations, and immunopathologic insights of paraneoplastic Kelch-like protein-11 encephalitis. JAMA Neurol. 77 , 1420–1429 (2020).
Guo, W. et al. SNX8 modulates the innate immune response to RNA viruses by regulating the aggregation of VISA. Cell. Mol. Immunol. 17 , 1126–1135 (2020).
Sharma, C. et al. Multisystem inflammatory syndrome in children and Kawasaki disease: a critical comparison. Nat. Rev. Rheumatol. 17 , 731–748 (2021).
Oikarinen, M. et al. Type 1 diabetes is associated with enterovirus infection in gut mucosa. Diabetes 61 , 687–691 (2012).
Larman, H. B. et al. Autoantigen discovery with a synthetic human peptidome. Nat. Biotechnol. 29 , 535–541 (2011).
Mandel-Brehm, C. et al. Autoantibodies to perilipin-1 define a subset of acquired generalized lipodystrophy. Diabetes 72 , 59–70 (2023).
Vazquez, S. E. et al. Identification of novel, clinically correlated autoantigens in the monogenic autoimmune syndrome APS1 by proteome-wide PhIP-seq. eLife 9 , e55053 (2020).
Bodansky, A. et al. Autoantigen profiling reveals a shared post-COVID signature in fully recovered and long COVID patients. JCI Insight 8 , e169515 (2023).
Bodansky, A. et al. Unveiling the proteome-wide autoreactome enables enhanced evaluation of emerging CAR-T therapies in autoimmunity. J. Clin. Invest . https://doi.org/10.1172/JCI180012 (2024).
Burbelo, P. D. et al. Autoantibodies against proteins previously associated with autoimmunity in adult and pediatric patients with COVID-19 and children with MIS-C. Front. Immunol. 13 , 841126 (2022).
Uhlén, M. et al. Proteomics. Tissue-based map of the human proteome. Science 347 , 1260419 (2015).
Johannes, L. & Wunder, C. The SNXy flavours of endosomal sorting. Nat. Cell Biol. 13 , 884–886 (2011).
Culina, S. et al. Islet-reactive CD8 + T cell frequencies in the pancreas, but not in blood, distinguish type 1 diabetic patients from healthy donors. Sci. Immunol. 3 , eaao4013 (2018).
Zamecnik, C. R. et al. ReScan, a multiplex diagnostic pipeline, pans human sera for SARS-CoV-2 antigens. Cell Rep. Med. 1 , 100123 (2020).
Pugliese, A. Autoreactive T cells in type 1 diabetes. J. Clin. Invest. 127 , 2881–2891 (2017).
Rydyznski Moderbacher, C. et al. Antigen-specific adaptive immunity to SARS-CoV-2 in acute COVID-19 and associations with age and disease severity. Cell 183 , 996–1012.e19 (2020).
Vita, R. et al. The Immune Epitope Database (IEDB): 2018 update. Nucleic Acids Res. 47 , D339–D343 (2019).
Harndahl, M. et al. Peptide-MHC class I stability is a better predictor than peptide affinity of CTL immunogenicity. Eur. J. Immunol. 42 , 1405–1416 (2012).
Sabatino, J. J. Jr et al. Anti-CD20 therapy depletes activated myelin-specific CD8 + T cells in multiple sclerosis. Proc. Natl Acad. Sci. USA 116 , 25800–25807 (2019).
Elong Ngono, A. et al. Frequency of circulating autoreactive T cells committed to myelin determinants in relapsing-remitting multiple sclerosis patients. Clin. Immunol. 144 , 117–126 (2012).
Dash, P. et al. Quantifiable predictive features define epitope-specific T cell receptor repertoires. Nature 547 , 89–93 (2017).
Schattgen, S. A. et al. Integrating T cell receptor sequences and transcriptional profiles by clonotype neighbor graph analysis (CoNGA). Nat. Biotechnol. 40 , 54–63 (2022).
Lee, J. S. et al. Immunophenotyping of COVID-19 and influenza highlights the role of type I interferons in development of severe COVID-19. Sci. Immunol. 5 , eabd1554 (2020).
Minervina, A. A. et al. SARS-CoV-2 antigen exposure history shapes phenotypes and specificity of memory CD8 + T cells. Nat. Immunol. 23 , 781–790 (2022).
Yousaf, A. R. et al. Notes from the field: surveillance for multisystem inflammatory syndrome in children — United States, 2023. MMWR Morb. Mortal. Wkly Rep. 73 , 225–228 (2024).
CDC. HAN Archive — 00432. https://emergency.cdc.gov/han/2020/han00432.asp (2021).
Rackaityte, E. et al. Validation of a murine proteome-wide phage display library for identification of autoantibody specificities. JCI Insight 8 , e174976 (2023).
Sabatino, J. J. Jr et al. Multiple sclerosis therapies differentially affect SARS-CoV-2 vaccine-induced antibody and T cell immunity and function. JCI Insight 7 , e156978 (2022).
Cimen Bozkus, C., Blazquez, A. B., Enokida, T. & Bhardwaj, N. A T-cell-based immunogenicity protocol for evaluating human antigen-specific responses. STAR Protoc. 2 , 100758 (2021).
Dolton, G. et al. More tricks with tetramers: a practical guide to staining T cells with peptide-MHC multimers. Immunology 146 , 11–22 (2015).
Wang, G. C., Dash, P., McCullers, J. A., Doherty, P. C. & Thomas, P. G. T cell receptor αβ diversity inversely correlates with pathogen-specific antibody levels in human cytomegalovirus infection. Sci. Transl. Med. 4 , 128ra42 (2012).
Versteven, M. et al. A versatile T cell-based assay to assess therapeutic antigen-specific PD-1-targeted approaches. Oncotarget 9 , 27797–27808 (2018).
Campillo-Davo, D. et al. Efficient and non-genotoxic RNA-based engineering of human T cells using tumor-specific T cell receptors with minimal TCR mispairing. Front. Immunol. 9 , 2503 (2018).
Mudd, P. A. et al. SARS-CoV-2 mRNA vaccination elicits a robust and persistent T follicular helper cell response in humans. Cell 185 , 603–613.e15 (2022).
Csardi, G. & Nepusz, T. The Igraph software package for complex network research. Complex Syst. 1695 , 1–9 (2005).
Jacomy, M., Venturini, T., Heymann, S. & Bastian, M. ForceAtlas2, a continuous graph layout algorithm for handy network visualization designed for the Gephi software. PLoS ONE 9 , e98679 (2014).
Article ADS PubMed PubMed Central Google Scholar
Heather, J. M. et al. Stitchr: stitching coding TCR nucleotide sequences from V/J/CDR3 information. Nucleic Acids Res. 50 , e68 (2022).
Wolf, F. A., Angerer, P. & Theis, F. J. SCANPY: large-scale single-cell gene expression data analysis. Genome Biol. 19 , 15 (2018).
Butler, A., Hoffman, P., Smibert, P., Papalexi, E. & Satija, R. Integrating single-cell transcriptomic data across different conditions, technologies, and species. Nat. Biotechnol. 36 , 411–420 (2018).
Polański, K. et al. BBKNN: fast batch alignment of single cell transcriptomes. Bioinformatics 36 , 964–965 (2020).
Traag, V. A., Waltman, L. & van Eck, N. J. From Louvain to Leiden: guaranteeing well-connected communities. Sci Rep. 9 , 5233 (2019).
Becht, E. et al. Dimensionality reduction for visualizing single-cell data using UMAP. Nat. Biotechnol . https://doi.org/10.1038/nbt.4314 (2018).
Pedregosa, F. et al. Scikit-learn: machine learning in Python. J. Mach. Learn. Res. 12 , 2825–2830 (2011).
MathSciNet Google Scholar
Download references
Acknowledgements
The following members of the Overcoming COVID-19 Network Investigators study group were all closely involved with the design, implementation and oversight of the Overcoming COVID-19 study, as well as collecting patient samples and data: M. Kong, H. Kelley, M. Murdock, C. Colston. K. V. Typpo, K. Irby, R. C. Sanders Jr, M. Yates, C. Smith, N. Z. Cvijanovich, M. S. Zinter, A. B. Maddux, E. Port, R. Mansour, S. Shankman, N. Baig, F. Zorensky, P. S. Espinal, B. Chatani, G. McLaughlin, K. M. Tarquinio, K. Jones, B. M. Coates, C. M. Rowan, A. G. Randolph, M. M. Newhams, S. Kucukak, T. Novak, E. R. McNamara, H. Kyung Moon, T. Kobayashi, J. Melo, S. R. Jackson, M. K. Echon Rosales, C. Young, S. R. Chen, J. Chou, R. Da Costa Aguiar, M. Gutierrez-Arcelus and M. Elkins. The Taking On COVID-19 Together team include: D. Williams, L. Williams, L. Cheng, Y. Zhang, D. Crethers, D. Morley, S. Steltz, K. Zakar, M. A. Armant, F. Ciuculescu, H. R. Flori, M. K. Dahmer, E. R. Levy, S. Behl, N. M. Drapeau, C. V. Hobbs, J. E. Schuster, A. Kietzman, S. Hill, M. L. Cullimore, R. J. McCulloh, S. J. Gertz, S. P. Schwartz, T. C. Walker, R. A. Nofziger, M. A. Staat, C. C. Rohlfs, J. C. Fitzgerald, R. Burnett, J. Bush, E. H. Mack, N. Reed, N. B. Halasa, L. L. Loftis, H. Crandall and K. K. Ampofo. Members of the US Centers for Disease Control and Prevention COVID-19 Response Team on the Overcoming COVID-19 study were L. D. Zambrano, M. M. Patel and A. P. Campbell. The authors acknowledge the New York Blood Center for contributing pre-COVID-19 healthy donor blood samples, which were used as controls for the SARS-CoV-2 library PhIP-seq. The authors acknowledge the contributions of W. Browne and S. Pleasure for their work investigating potential central nervous system-specific autoimmunity in MIS-C; T. Kharel for help designing the Python code used in the analysis; D. Blauvelt for ideas regarding the application of advanced statistics to PhIP-seq data analysis; and S. A. Schattgen for thoughtful discussion of TCR sequencing and TCR similarity network analysis and help with deposition of the TCR sequencing into the Sequence Read Archive. BioRender ( https://biorender.com ) was used to build graphics for Fig. 1a and Extended Data Fig. 4a . This work was supported by the Pediatric Scientist Development Program and the Eunice Kennedy Shriver National Institute of Child Health and Human Development (K12-HD000850 to A.B.), and the Chan Zuckerberg Biohub SF (to J.L.D. and M.S.A.). Overcoming COVID-19 Study Network enrolment, patient data and specimen collections were supported by the CDC contracts 75D30120C07725, 75D30121C10297 and 75D30122C13330 from the Centers for Disease Control and Prevention to Boston Children’s Hospital to A.G.R. and the National Institute of Allergy and Infectious Diseases (R01AI154470) to A.G.R. Patient clinical data and specimens also collected at Boston Children’s Hospital for the Taking On COVID-19 Together (TOCT) study were supported in part by the Boston Children’s Hospital Emerging Pathogens and Epidemic Response Cluster of Clinical Research Excellence and the Institutional Centers for Clinical and Translational Research to A.G.R. and K.L.M. P.G.T. is supported by the American Lebanese Syrian Associated Charities at St. Jude Children’s Research Hospital (SJCRH) and funding from the National Institute of Allergy and Infectious Diseases (5R01AI154470-03, 2R01AI136514-06, 3P01AI165077-01S1, 75N93021C00016 and U01 AI144616). R.C.M. is supported by a Ruth L. Kirschstein National Research Service Award Individual Postdoctoral Fellowship award (F32AI157296).
Author information
These authors contributed equally: Aaron Bodansky, Robert C. Mettelman
These authors jointly supervised this work: Paul G. Thomas, Adrienne G. Randolph, Mark S. Anderson, Joseph L. DeRisi
Authors and Affiliations
Department of Pediatrics, Division of Critical Care, University of California San Francisco, San Francisco, CA, USA
Aaron Bodansky & Matt S. Zinter
Department of Host–Microbe Interactions, St. Jude Children’s Research Hospital, Memphis, TN, USA
Robert C. Mettelman, Mikhail V. Pogorelyy, Walid Awad, Allison M. Kirk & Paul G. Thomas
Weill Institute for Neurosciences, University of California San Francisco, San Francisco, CA, USA
Joseph J. Sabatino Jr, Colin R. Zamecnik & Michael R. Wilson
Department of Neurology, University of California San Francisco, San Francisco, CA, USA
Joseph J. Sabatino Jr, Colin R. Zamecnik, John V. Pluvinage & Michael R. Wilson
Department of Biochemistry and Biophysics, University of California San Francisco, San Francisco, CA, USA
Sara E. Vazquez, Haleigh S. Miller, Andrew F. Kung, Elze Rackaityte, Jayant V. Rajan, Hannah Kortbawi, Caleigh Mandel-Brehm, Kelsey Zorn & Joseph L. DeRisi
Division of Immunology, Department of Pediatrics, Boston, MA, USA
Department of Pediatrics, Harvard Medical School, Boston, MA, USA
Janet Chou, Kristin L. Moffitt & Adrienne G. Randolph
Department of Anesthesiology, Critical Care and Pain Medicine, Boston Children’s Hospital, Boston, MA, USA
Tanya Novak & Adrienne G. Randolph
Department of Anesthesia, Harvard Medical School, Boston, MA, USA
Department of Pediatric, Division of Infectious Diseases, Boston Children’s Hospital, Boston, MA, USA
Kristin L. Moffitt
Biological and Medical Informatics Program, University of California San Francisco, San Francisco, CA, USA
Haleigh S. Miller & Andrew F. Kung
Medical Scientist Training Program, University of California San Francisco, San Francisco, CA, USA
Hannah Kortbawi
Chan Zuckerberg Biohub SF, San Francisco, CA, USA
Anthea Mitchell, Chung-Yu Wang, Aditi Saxena & Joseph L. DeRisi
Diabetes Center, School of Medicine, University of California San Francisco, San Francisco, CA, USA
David J. L. Yu & Mark S. Anderson
Biomedical Sciences Program, University of California San Francisco, San Francisco, CA, USA
James Asaki
COVID-19 Response Team and Coronavirus and Other Respiratory Viruses Division, Centers for Disease Control and Prevention, Atlanta, GA, USA
Laura D. Zambrano & Angela P. Campbell
Department of Medicine, Division of Endocrinology and Metabolism, University of California San Francisco, San Francisco, CA, USA
Mark S. Anderson
Department of Pediatrics, Division of Critical Care Medicine, Baylor College of Medicine, Houston, TX, USA
- Laura L. Loftis
Department of Pediatrics, Division of Infectious Diseases, University of Mississippi Medical Center, Jackson, MS, USA
Charlotte V. Hobbs
Department of Pediatrics, Division of Critical Care Medicine, Emory University School of Medicine, Children’s Healthcare of Atlanta, Atlanta, GA, USA
Keiko M. Tarquinio
Department of Pediatrics, Division of Pediatric Critical Care Medicine, University of Alabama at Birmingham, Birmingham, AL, USA
Michele Kong
Department of Anesthesiology and Critical Care, Children’s Hospital of Philadelphia, University of Pennsylvania, Perelman School of Medicine, Philadelphia, PA, USA
Julie C. Fitzgerald
Personalized Medicine and Health Outcomes Research, Nicklaus Children’s Hospital, Miami, FL, USA
Paula S. Espinal
Department of Pediatrics, University of North Carolina at Chapel Hill Children’s Hospital, Chapel Hill, NC, USA
Tracie C. Walker & Stephanie P. Schwartz
Department of Pediatrics, Division of Pediatric Critical Care, University of Utah, Primary Children’s Hospital, Salt Lake City, UT, USA
Hillary Crandall
Section of Pediatric Critical Care, Department of Pediatrics, Arkansas Children’s Hospital, Little Rock, AR, USA
Katherine Irby
Department of Pediatrics, Division of Infectious Diseases, University of Cincinnati and Cincinnati Children’s Hospital Medical Center, Cincinnati, OH, USA
Mary Allen Staat
Department of Pediatrics, Division of Pediatric Critical Care Medicine, Indiana University School of Medicine and Riley Hospital for Children, Indianapolis, IN, USA
Courtney M. Rowan
Department of Pediatrics, Division of Pediatric Infectious Diseases, Children’s Mercy Kansas City, Kansas City, MO, USA
Jennifer E. Schuster
Department of Pediatrics, Division of Pediatric Infectious Diseases, Vanderbilt University Medical Center, Nashville, TN, USA
Natasha B. Halasa
Department of Pediatrics, Division of Pediatric Critical Care, Cooperman Barnabas Medical Center, Livingston, NJ, USA
Shira J. Gertz
Division of Pediatric Critical Care Medicine, Medical University of South Carolina, Charleston, SC, USA
Elizabeth H. Mack
Department of Pediatrics, Section of Critical Care Medicine, University of Colorado School of Medicine and Children’s Hospital Colorado, Aurora, CO, USA
Aline B. Maddux
Division of Critical Care Medicine, UCSF Benioff Children’s Hospital Oakland, Oakland, CA, USA
Natalie Z. Cvijanovich
You can also search for this author in PubMed Google Scholar
Overcoming COVID-19 Network Investigators
- , Charlotte V. Hobbs
- , Keiko M. Tarquinio
- , Michele Kong
- , Julie C. Fitzgerald
- , Paula S. Espinal
- , Tracie C. Walker
- , Stephanie P. Schwartz
- , Hillary Crandall
- , Katherine Irby
- , Mary Allen Staat
- , Courtney M. Rowan
- , Jennifer E. Schuster
- , Natasha B. Halasa
- , Shira J. Gertz
- , Elizabeth H. Mack
- , Aline B. Maddux
- , Natalie Z. Cvijanovich
- & Matt S. Zinter
Contributions
A.B., R.C.M., J.J.S.Jr, S.E.V., J.C., P.G.T., A.G.R., M.S.A. and J.L.D. conceptualized the study. A.B., R.C.M., J.J.S.Jr, S.E.V., E.R., C.R.Z., A.F.K., J.V.R., J.C., P.G.T., A.G.R., M.S.A. and J.L.D. curated the methodology. A.B., R.C.M., J.J.S.Jr, S.E.V., A.M., C.-Y.W., A.S., J.V.P., D.J.L.Y., H.K., W.A., A.M.K. and C.M.-B. performed or contributed to experiments. A.B., R.C.M., J.J.S.Jr, H.S.M., A.F.K., J.A. and M.V.P. performed the formal analysis. K.Z., T.N., L.D.Z., A.P.C., A.G.R., K.L.M. and the Overcoming COVID-19 Network Investigators acquired the patient sample and clinical data. T.N., A.G.R. and the Overcoming COVID-19 Network Investigators curated the clinical data. A.B., H.S.M. and J.L.D. wrote the original draft of the manuscript. A.B., R.C.M., J.C., T.N., H.S.M., L.D.Z., A.P.C., P.G.T., A.G.R., M.R.W., M.S.A. and J.L.D. reviewed and edited the manuscript. J.C., P.G.T., A.G.R., M.S.A. and J.L.D. supervised the study.
Corresponding authors
Correspondence to Mark S. Anderson or Joseph L. DeRisi .
Ethics declarations
Competing interests.
J.L.D. reports being a founder and paid consultant for Delve Bio, Inc., and a paid consultant for the Public Health Company and Allen & Co. M.A.S. receives unrelated research funding from the National Institutes of Health, the Centers for Disease Control and Prevention, Cepheid and Merck and unrelated honoria from UpToDate, Inc. M.R.W. receives unrelated research grant funding from Roche/Genentech and Novartis, and received speaking honoraria from Genentech, Takeda, WebMD and Novartis. J.C. reports consulting fees from GLG group, payments from Elsevier for work as an Associate Editor, a patent pending for methods and compositions for treating and preventing T cell-driven diseases, payments related to participation on a Data Safety Monitoring Board or Advisory Board for Enzyvant, and is a member of the Diagnostic Laboratory Immunology Committee of the Clinical Immunology Society. M.S.Z. receives unrelated funding from the National Heart, Lung, and Blood Institute and consults for Sobi. N.B.H. reports unrelated previous grant support from Sanofi and Quidel, and current grant support from Merck. C.V.H. reports being a speaker for Biofire and a reviewer for UpToDate, Inc. and Dynamed.com. A.G.R. receives royalties as a section editor for Pediatric Critical Care Medicine UpToDate, Inc., and received honoraria for MIS-C-related Grand Round Presentations. A.G.R. is also on the medical advisor board of Families Fighting Flu and is Chair of the International Sepsis Forum, which is supported by industry and has received reagents from Illumina, Inc. P.G.T. is on the Scientific Advisory Board of Immunoscape and Shennon Bio, has received research support and personal fees from Elevate Bio, and consulted for 10X Genomics, Illumina, Pfizer, Cytoagents, Merck and JNJ. The findings and conclusions in this report are those of the authors and do not necessarily represent the views of the Centers for Disease Control and Prevention nor the National Institute of Allergy and Infectious Diseases. All other authors declare no competing interests.
Peer review
Peer review information.
Nature thanks Shiv Pillai and the other, anonymous, reviewer(s) for their contribution to the peer review of this work. Peer reviewer reports are available.
Additional information
Publisher’s note Springer Nature remains neutral with regard to jurisdictional claims in published maps and institutional affiliations.
Extended data figures and tables
Extended data fig. 1 previously reported autoantigens and phenotypic associations of novel autoantigens..
a , Heatmap showing distribution of PhIP-Seq enrichments (FC > Mock-IP) of previously reported MIS-C autoantibodies in MIS-C patients ( n = 199) and at-risk controls (n = 45). (b) Stripplots and boxplots showing distribution of signal (normalized antibody index) for antibodies targeting IL-1 receptor antagonist (IL-1Ra) measured by RLBA in at-risk controls (blue; n = 45), MIS-C patient samples containing IVIG (red; n = 135), and MIS-C patient samples without IVIG (green; n = 61). Dotted line at 3 standard deviations above the mean of controls. Two-sided Mann-Whitney U testing was performed (exact P values shown in figure). c , Heatmap of P values (two-sided Kolmogorov-Smirnov testing) for differences in autoantibody enrichment for MIS-C patients ( n = 199) with versus without each clinical phenotype (numbers vary for each phenotype and are shown in Extended Data Table 2 ). Significant P values in the negative direction (in which there is increased signal in individuals without the phenotype) are masked (colored as P > 0.05). For each autoantigen, tissue RNA-sequencing data from Human Protein Atlas (Proteinatlas.org) is shown. Amount of expression in cardiac tissue in top row (Very high = nTPM >1000, High=nTPM 100-1000, Moderate=nTPM 10-100, Low=nTPM <10), and predominant tissue type in second-from-top row. Explanations of criteria for MIS-C phenotypes, and distribution of each phenotype within the cohort, can be found in Extended Data Table 2 .
Extended Data Fig. 2 Orthogonally validated autoantibodies classify MIS-C and can be epitope specific.
a , Stripplots and boxplots showing radioligand binding assay (RLBA) values (normalized antibody indices) for each of the top 3 autoantibodies identified by PhIP-Seq logistic regression in individuals with MIS-C ( n = 197 for ERFL, n = 196 for SNX8, n = 196 for KDELR1) and each at-risk control ( n = 45 for ERFL, SNX8, and KDELR1). Two-sided Mann-Whitney U testing performed (exact P values shown in figure). b , Logistic regression receiver operating characteristic (ROC) curve using RLBA values as input to distinguish MIS-C patients ( n = 196) from at-risk controls ( n = 45) iterated 1,000 times. c , Stripplots and boxplots showing RLBA enrichments (normalized antibody indices) only in those MIS-C samples without IVIG ( n = 61 for ERFL, n = 60 for SNX8, n = 60 for KDELR1) relative to at-risk controls ( n = 45 for ERFL, SNX8, and KDELR1). Two-sided Mann-Whitney U testing performed (exact P values shown in figure). d , Stripplots abd boxplots showing RLBA enrichments (normalized antibody indices) for ERFL, SNX8, and KDELR1 in an independent cohort of children with MIS-C (red; n = 24 for each RLBA) compared to children severely ill with acute COVID-19 (yellow; n = 29 for each RLBA) and at-risk controls (blue; n = 45 for each RLBA). Two-sided Mann-Whitney U testing performed (exact P values shown in figure). e , Logistic regression ROC curves for classification of the independent MIS-C cohort ( n = 24) versus at-risk controls ( n = 45) (left) and the independent MIS-C ( n = 24) cohort versus children severely ill with acute COVID-19 ( n = 29) (right). f , Paired stripplots and boxplots showing SLBA enrichments (normalized antibody indices) in MIS-C patients ( n = 182) and at-risk controls ( n = 45) for the full 49 amino acid SNX8 wild-type (WT) polypeptide fragment (lavender) relative to the same SNX8 fragment with alanine mutagenesis of the [PSRMQMPQG] epitope (white). SNX8 WT fragment SLBA values are the means of technical replicates, SNX8 epitope mutagenesis values are from a single experiment. Two-sided Mann-Whitney U testing performed (exact P values shown in figure). For all boxplots in the figure, the whiskers extend to 1.5 times the interquartile range (IQR) from the quartiles, the boxes represent the IQR, and centre lines represent the median.
Extended Data Fig. 3 HLA associations of SNX8 activated T cells and HLA binding characteristics of peptides containing SNX8/MADS shared epitope motif.
a , Stripplots and boxplots showing distribution of CD4 + , CD8 + , and total T cells which activate in response to either vehicle (culture media + 0.2% DMSO) or SNX8 peptide pool (SNX8 peptide + culture media + 0.2% DMSO) using AIM assay in MIS-C patients ( n = 9) and controls ( n = 10). Patient HLA type indicated by color of dot. HLA unpredicted means patient contained none of the MIS-C associated HLA types. Dotted line at 3 standard deviations above the mean of the SNX8 stimulated controls. Two-sided Mann-Whitney U testing was performed (exact P values shown in figure). b , Computationally predicted HLA class I presentation scores (Immune Epitope Database; IEDB.org) for each possible peptide fragment of full-length SARS-CoV-2 N protein for each of the three MIS-C associated HLA types (A*02, B*35 and C*04) relative to a reference set of HLA-types encompassing over 99% of humans. Those fragments containing the MADS similarity region “LQLPQG” in orange. Data normally distributed; two-sided t-tests were performed (exact P values shown in figure). Percent of fragments within each specific HLA type with a score greater than 0.1 (likely to be presented) shown on right. c , Identical analysis but using full length SNX8 protein rather than SARS-CoV-2 NP, and the SNX8 similarity region “MGMPQG” rather than the MADS region “LQLPQG”. Data normally distributed; two-sided t-tests were performed (exact P values shown in figure). d , HLA binding results from β2m folding assay for SARS-CoV-2 N and SNX8 peptides representative of two independent evaluations. Each peptide tested for binding in HLA-A*02:01, A*02:06, and B*35:01 class I monomers. Data presented as geometric mean fluorescence intensity (gMFI) of PE-conjugated anti-human β2m antibody staining of peptide-HLA monomers relative to negative (no peptide; unloaded HLA monomer) and positive (strong binding peptide; CMV pp65 495-503 [NLVPMVATV]) controls. For all boxplots in the figure, the whiskers extend to 1.5 times the interquartile range (IQR) from the quartiles, the boxes represent the IQR, and centre lines represent the median.
Extended Data Fig. 4 Identification, activation, and HLA restriction, of cross-reactive CD8+ T cells.
a , Gating strategy used to identify CD8 + T cells which bound to SNX8 epitope and/or MADS N protein epitope (CD8 + T cells positive for PE). Representative MIS-C patient and control showing each CD8 + T cell which bound to any tetramer (PE + ) and the relative binding of that T cell to both the SNX8 epitope (BV421 + ) and the MADS N protein epitope (APC + ) identifying cross-reactive T cells (PE + APC + BV421 + ). Schematics in panel a were created using BioRender ( https://www.biorender.com ). b , Stripplots and boxplots showing percentage of CD8 + T cells which are cross-reactive to both SNX8 and MADS in MIS-C patients ( n = 3) and controls ( n = 3). Insufficient numbers to perform robust statistical testing. c , Stripplots and boxplots showing percentage of total T cells which activate in response to either vehicle (culture media + 0.2% DMSO) or the SNX8 Epitope (SNX8 Epitope (Materials) + culture media + 0.2% DMSO) in MIS-C patients ( n = 2) and at-risk controls ( n = 4) measured by AIM assay. Insufficient numbers to perform robust statistical testing. Dotted line at 3 standard deviations above mean of SNX8 Epitope stimulated controls. d , TCRdist Similarity Network of 48 unique, paired TCRαβ sequences ( n = 259 sequences) obtained from four patients with MIS-C. CD8 + T cells were sorted from PBMCs directly ex vivo or after 10-days of peptide expansion and staining with A*02:01 or A*02:06 HLA class I tetramers loaded with MADS [LQLPQGITL] and SNX8 [MQMPQGNPL] peptides. Each node represents a unique TCR clonotype. Edges connect nodes with a TCRdist score < 150. Dashed lines surround TCR similarity clusters. Node size corresponds to T cell clone size. Nodes are colored based on HLA restriction. TCRs selected for further testing are numbered TCR #1-8. Convergent node circled green. For all boxplots in the figure, the whiskers extend to 1.5 times the interquartile range (IQR) from the quartiles, the boxes represent the IQR, and centre lines represent the median.
Extended Data Fig. 5 Evaluation of Jurkat-TCR lines.
a , Jurkat-76 cells stably expressing putative cross-reactive TCRs (#1-8) stained with anti-murine TCRβ constant region (mTCRβc). Plots depict frequency of transduced (mCherry + ) Jurkat cells with presence of surface TCR (APC/Fire 750 + ) as a percentage of total live cells. b , Jurkat-TCR + cell lines expressing putative cross-reactive TCRs #1-8 stained with individual or combination of HLA-A*02:01 or A*02:06 tetramers loaded with MADS [LQLPQGITL] and SNX8 [MQMPQGNPL] peptides. Blue contour plots indicate staining with MADS-Tetramer (PE) and MADS-Tetramer (APC); purple contour plots indicate staining with SNX8-Tetramer (PE) and SNX8-Tetramer (BV421); red indicates combined staining with a pool of MADS/SNX8-Tetramer (PE), MADS-Tetramer (APC), and SNX8-Tetramer (BV421). Plots shown are gated from total PE + cells. Plots with confirmed cross-reactive TCRs outlined in red. c , Jurkat-TCR+ cell lines expressing putative cross-reactive TCRs #1-8 stained with individual HLA-A*02:01 or A*02:06 tetramers loaded with MADS Wuhan [LQLPQGTTL] peptide. Gate values indicate frequency of MADS-APC + cells as percentage of total MADS-PE + cells. Outliers shown in contour plots. Flow plots representative of two independent evaluations.
Extended Data Fig. 6 SNX8 expression during viral infection.
a , UMAPs showing SNX8 expression in various peripheral blood cell types during SARS-CoV-2 infection. b , Mean expression and percent of cells expressing SNX8 in peripheral blood subsets during SARS-CoV-2 infection. c , Mean expression and percent of cells expressing SNX8 averaged across all peripheral blood mononuclear cells from SARS-CoV-2 infected individuals without symptoms, with mild symptoms, or with severe disease compared to uninfected controls. d , Mean expression and percent of cells expressing SNX8 , OAS1 , OAS2 , and MAVS in peripheral blood subsets during SARS-CoV-2 infection. e , Relative expression of SNX8 , OAS1 , OAS2 , and MAVS during influenza virus infection compared to different severities of SARS-CoV-2 infection.
Extended Data Fig. 7 Representative flow cytometry gating.
a , Flow cytometry gating strategy for identifying CD4 positive and CD8 positive T cells for the AIM analysis with representative activation induced marker (AIM) assay flow cytometry gating strategy measuring percent of CD4 + T cells which activate (CD137 + OX40 + ) and percent of CD8 + T cells which activate (CD137 + CD69 + ) in response to SNX8 protein. b , Flow cytometry gating strategy for the initial SNX8/MADS tetramer cross-reactivity assay (Extended Data Fig. 4a,b ) showing isolation of PE-tetramer positive CD8 positive T cells. c , Flow cytometry plots showing results of serotyping for the PBMCs used in the initial SNX8/MADS tetramer cross-reactivity assay (Extended Data Fig. 4a,b ) which did not have sufficient cells for genotyping. Shown is the 1 MIS-C patient (far left) and 3 controls (middle 3) which are positive for HLA-A*02 and were used and one control negative for HLA-A*02 (far right) which was not used. d , Index sorting strategy for patient PBMCs from ex vivo and peptide expansion experiments for TCR sequencing. Single cells were sorted from live/lineage (CD4, CD14, CD16, CD19)-negative, CD3 + CD8 + T lymphocytes positive for MADS/SNX8-Tetramer (PE) and MADS-Tetramer (APC) and/or SNX8-Tetramer (BV421). e , Flow cytometry gating strategy to evaluate putative cross-reactive Jurkat-TCRs. Gates include single, live, transduced Jurkat lymphocytes triple positive for MADS/SNX8-(PE), MADS-(APC), and SNX8-(BV421) tetramers shown in Fig. 4 .
Supplementary information
Supplementary table 1.
The complete set of primers used for the single-cell T cell receptor (TCR) sequencing by nested multiplex polymerase chain reaction (PCR).
Reporting Summary
Peer review file, source data, source data fig. 1, source data fig. 2, source data fig. 3, source data fig. 4, source data extended data fig. 1, source data extended data fig. 2, source data extended data fig. 3, source data extended data fig. 4, source data extended data fig. 5, rights and permissions.
Open Access This article is licensed under a Creative Commons Attribution 4.0 International License, which permits use, sharing, adaptation, distribution and reproduction in any medium or format, as long as you give appropriate credit to the original author(s) and the source, provide a link to the Creative Commons licence, and indicate if changes were made. The images or other third party material in this article are included in the article’s Creative Commons licence, unless indicated otherwise in a credit line to the material. If material is not included in the article’s Creative Commons licence and your intended use is not permitted by statutory regulation or exceeds the permitted use, you will need to obtain permission directly from the copyright holder. To view a copy of this licence, visit http://creativecommons.org/licenses/by/4.0/ .
Reprints and permissions
About this article
Cite this article.
Bodansky, A., Mettelman, R.C., Sabatino, J.J. et al. Molecular mimicry in multisystem inflammatory syndrome in children. Nature 632 , 622–629 (2024). https://doi.org/10.1038/s41586-024-07722-4
Download citation
Received : 20 June 2023
Accepted : 14 June 2024
Published : 07 August 2024
Issue Date : 15 August 2024
DOI : https://doi.org/10.1038/s41586-024-07722-4
Share this article
Anyone you share the following link with will be able to read this content:
Sorry, a shareable link is not currently available for this article.
Provided by the Springer Nature SharedIt content-sharing initiative
By submitting a comment you agree to abide by our Terms and Community Guidelines . If you find something abusive or that does not comply with our terms or guidelines please flag it as inappropriate.
Quick links
- Explore articles by subject
- Guide to authors
- Editorial policies
Sign up for the Nature Briefing newsletter — what matters in science, free to your inbox daily.

- Skip to main content
- Skip to search
- Skip to footer
Products and Services

Configuration engineer
Ben Harting
"I decided to make a career change when I was twenty-four. I chose to pursue Cisco Certifications because I knew it would put me in the best position to start a career in networking."
How it all began
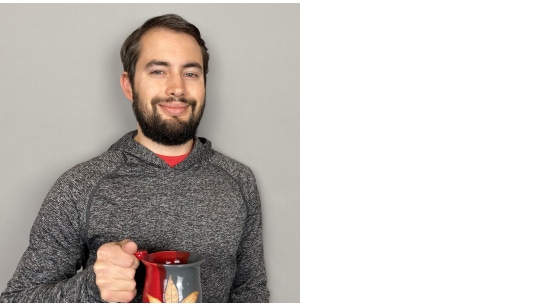
Certifications can improve your ability to do your job
My dad is a systems administrator, so I grew up around computers. Just watching him tinker with them was cool. However, when I went to college, I earned a bachelor’s degree in philosophy. Next, I got a teaching credential, then taught elementary school for a few years. But when I turned 24, I decided I wanted a career change. So, I picked up a book on TCP/IP networking, started studying it, and realized that I really enjoyed it. My dad wasn’t into networking that much, so I didn’t know much about it, and got into it more organically. I considered pursuing Cisco certifications right away since the idea of learning about networking led straight to these credentials. I took a networking class at a local community college, then obtained my Cisco CCNA certification.
I enjoy system administration, I’ve gained communications skills, and I’ve learned tech skills, in Microsoft active directory systems, administration, and networking virtualization. General troubleshooting is a big part of my job, too. Most importantly, I’ve gained the flexibility to work around difficulties and learn new technologies. Just being able to keep up with the constant change in technologies is incredibly valuable.

Certifications

Columbus, OH

Reading Hiking Learning new tech
What does having a Cisco Certification mean to you?
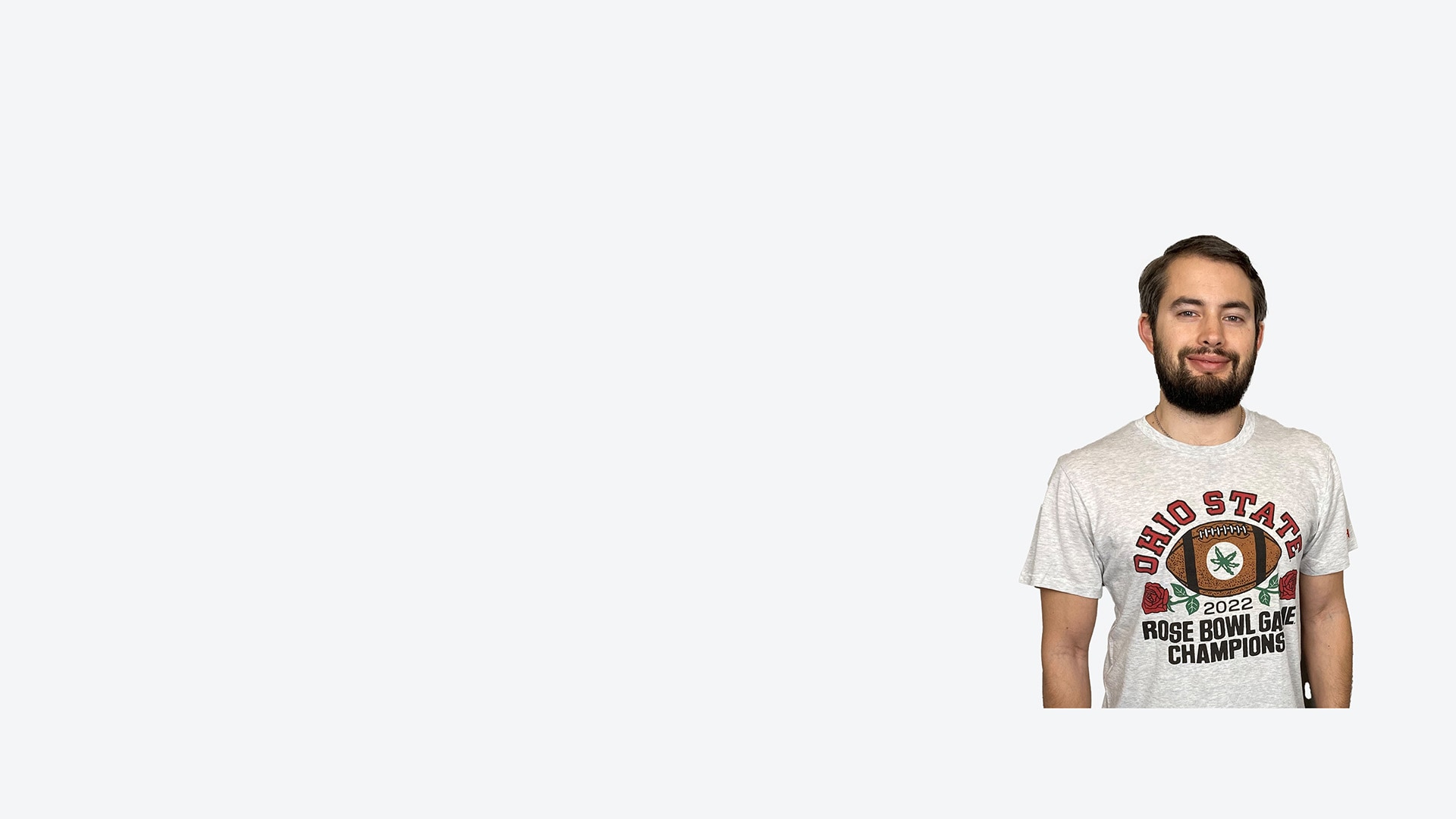
"I’ve gained more knowledge and different skill sets. I’ve opened myself up for more opportunities. And it’s a validation of me and what I’ve learned."
The biggest challenge was where to start, there where a lot of options. A lot of them involved going back to school or spending a lot of money. CCNA was the most cost-effective, and it would put me in the best position to start a career. Cisco is a leader in that. I took a bootcamp course at the local community college that lasted 8 weeks in total.
I was already in my job in IT in tier 1 support, help desk and the CCNA Certification was a catalyst for my first promotion as a second tier support security.

What would you tell your younger self?
Definitely try different things and go all in on whatever interests you the most.
What would you tell a friend?
I’d tell them to go for it. It will open up a lot of opportunities for them. They’ll have all the knowledge they need to go forward in their career.
Ben's journey
Career path.
Current role
Configuration engineers work on systems and network administration.
Previous role
IT Help Desk Support Primary School Educator
Certification path
Most recent certification
Cisco Certified Network Associate (CCNA) certification is the first step toward a career in IT Networking. The CCNA exam covers networking fundamentals, IP services, security fundamentals, automation and programmability.
Read more certification success stories
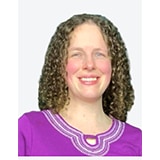
"The guidance I gained from earning the certification helped— in near real time—to determine what was happening on the job, when I became an SOC operator."
Network security analyst CyberOps Associate

Yasser Auda
"You can do anything. You just need to decide to do it, have the will to do it, and never give up. Be confident in yourself and stop the barriers in your mind."
Network security architect CCNA, CCNP Enterprise, CCNP Security, CyberOps Associate, CCIE Enterprise Infrastructure, Cisco Certified DevNet Associate, Cisco Certified DevNet Professional

Olivia Wolf
"The knowledge that I’ve got from studying for those certifications gave me the confidence that I’ll always be able to get a job if I need to."
Systems engineer CCNA, CCNP Enterprise, DevNet Associate
Share your Cisco Certification Success Story
Has earning a Cisco certification positively changed your life or career, or both? Do you think your Cisco certification story would help encourage other people to earn their Cisco certification? If so, we want to talk to you!
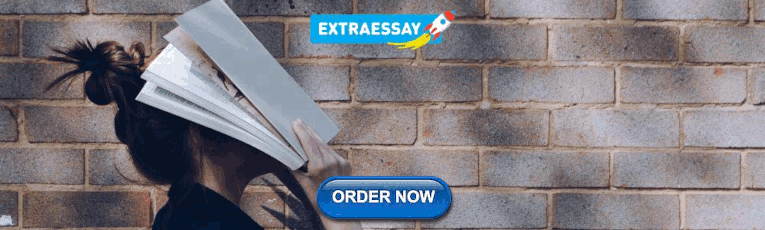
IMAGES
COMMENTS
Learn how to report t-test results in different formats and scenarios, with clear examples and explanations. A useful guide for statistical analysis.
The t -distribution reflects this difference of uncertainty according to sample size. Therefore the shape of t -distribution changes by the degree of freedom (df), which is sample size minus one (n − 1) when one sample mean is tested. The t -distribution appears to be a family of distribution of which shape varies according to its df ( Figure ...
Therefore, there are three forms of Student's t-test about which physicians, particularly physician-scientists, need to be aware: (1) one-sample t-test; (2) two-sample t-test; and (3) two-sample paired t-test. The one-sample t-test evaluates a single list of numbers to test the hypothesis that a statistic of that set is equal to a chosen ...
A t test is a statistical test used to compare the means of two groups. The type of t test you use depends on what you want to find out.
The purpose of this research is to study the t-test, especially the one sample t-test to determine if the sample data come from the same population.
An independent-group t test can be carried out for a comparison of means between two independent groups, with a paired t test for paired data. As the t test is a parametric test, samples should meet certain preconditions, such as normality, equal variances and independence.
The t distribution is a probability distribution similar to the Normal distribution. It is commonly used to test hypotheses involving numerical data. This paper provides an understanding of the t ...
(i.e., independent samples) t test. Unlike the hypothetical examples above, this real example comes from an early draft of a research paper b Shimizu, Pelham, and Sperry (2012). The manipulation in the study was whether participants who tried to take a nap were subliminally primed with sleep-related or neutral words
In medical research, various t -tests and Chi-square tests are the two types of statistical tests most commonly used. In any statistical hypothesis testing situation, if the test statistic follows a Student's t -test distribution under null hypothesis, it is a t -test. Most frequently used t -tests are: For comparison of mean in single sample ...
An independent samples t-test compares the means of two groups. The data are interval for the groups. There is not an assumption of normal distribution (if the distribution of one or both groups is really unusual, the t-test will not give good results with unequal sample sizes), but there is an assumption that the two standard deviations are ...
In this research, using the two-sample t-test, a comparison between the students in the three grades of the Department of Mathematics Education, Tishk International University, is made to see ...
The independent sample t-test compares the mean of one distinct group to the mean of another group. An example research question for an independent sample t-test would be, " Do boys and girls differ in their SAT scores ?" The dependent sample t-test compares two matched scores or measurements (such as before vs. after). An example research question for a dependent sample t-test would be ...
6 Examples of Using T-Tests in Real Life. In statistics, there are three commonly used t-tests: One Sample t-test: Used to compare a population mean to some value. Independent Two Sample t-test: Used to compare two population means. Paired Samples t-test: Used to compare two population means when each observation in one sample can be paired ...
Student's t test ( t test), analysis of variance (ANOVA), and analysis of covariance (ANCOVA) are statistical methods used in the testing of hypothesis for comparison of means between the groups. The Student's t test is used to compare the means between two groups, whereas ANOVA is used to compare the means among three or more groups.
two groups. The independent t- test depends on the means, standard deviation s and sample sizes of each group. Various assumptions also need to be made - see validity section below.
The two basic types include the Independent Samples t-Test and the Paired t-Test. This paper interprets derived PSPP results -data set 1 - resulting in an Analysis-Compare Means-Independent Samples t-Test and data set 2 - resulting in an Analysis-Compare Means-Paired Sample t-Test. It's a 3-page report identifying the significant ...
In this paper we discuss the significance of t-test in small sample of statistics to analyze the data. Here we approach many application of t-test in statistics and research. This paper is aimed at introducing hypothesis testing, focusing on the
A simple explanation of a two sample t-test including a definition, a formula, and a step-by-step example of how to perform it.
The t test is relatively robust against nonnormality with larger sample sizes. Conventionally, the sample size needs to be ≥30; however, a much larger sample may be necessary if the data distribution is severely skewed. Importantly, smaller samples definitely do rely on a normal distribution to avoid erroneous conclusions.
An independent-group t test can be carried out for a. comparison of means between two independen t groups, with a paired t test fo r paired data. As the t test is a parametric. test, samples ...
Feature papers represent the most advanced research with significant potential for high impact in the field. A Feature Paper should be a substantial original Article that involves several techniques or approaches, provides an outlook for future research directions and describes possible research applications.
The bread and butter of statistical analysis "t-test": Uses and misuses. Statistical tests are very important in biomedical research. 1 Several factors play a role in selecting the most appropriate statistical test. 2 The misuse or inaccurate use of a statistical test may navigate the research in the wrong direction, and hence incorrect ...
A cross-reactive antibody and T cell response is identified in a large portion of patients with multisystem inflammatory syndrome in children.
Student's t -test is a method of testing hypotheses about the mean of a small sample drawn from a normally distributed population when the population standard deviation is unknown. In 1908 William ...
I enjoy system administration, I've gained communications skills, and I've learned tech skills, in Microsoft active directory systems, administration, and networking virtualization.
The Paired Sample t-test is a comparative hypothesis test that aims to determine whether there is a difference in the mean of two pairs of paired or related samples (Samuels, 2015).