Have a language expert improve your writing
Run a free plagiarism check in 10 minutes, generate accurate citations for free.
- Knowledge Base
Methodology
- What Is a Case Study? | Definition, Examples & Methods
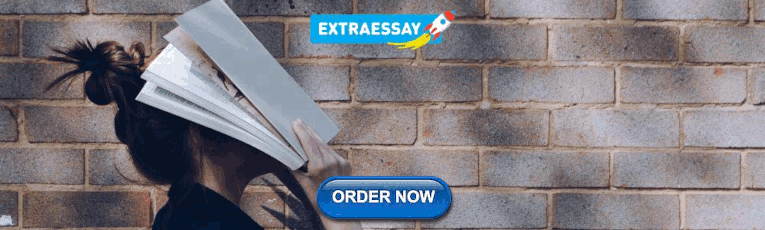
What Is a Case Study? | Definition, Examples & Methods
Published on May 8, 2019 by Shona McCombes . Revised on November 20, 2023.
A case study is a detailed study of a specific subject, such as a person, group, place, event, organization, or phenomenon. Case studies are commonly used in social, educational, clinical, and business research.
A case study research design usually involves qualitative methods , but quantitative methods are sometimes also used. Case studies are good for describing , comparing, evaluating and understanding different aspects of a research problem .
Table of contents
When to do a case study, step 1: select a case, step 2: build a theoretical framework, step 3: collect your data, step 4: describe and analyze the case, other interesting articles.
A case study is an appropriate research design when you want to gain concrete, contextual, in-depth knowledge about a specific real-world subject. It allows you to explore the key characteristics, meanings, and implications of the case.
Case studies are often a good choice in a thesis or dissertation . They keep your project focused and manageable when you don’t have the time or resources to do large-scale research.
You might use just one complex case study where you explore a single subject in depth, or conduct multiple case studies to compare and illuminate different aspects of your research problem.
Research question | Case study |
---|---|
What are the ecological effects of wolf reintroduction? | Case study of wolf reintroduction in Yellowstone National Park |
How do populist politicians use narratives about history to gain support? | Case studies of Hungarian prime minister Viktor Orbán and US president Donald Trump |
How can teachers implement active learning strategies in mixed-level classrooms? | Case study of a local school that promotes active learning |
What are the main advantages and disadvantages of wind farms for rural communities? | Case studies of three rural wind farm development projects in different parts of the country |
How are viral marketing strategies changing the relationship between companies and consumers? | Case study of the iPhone X marketing campaign |
How do experiences of work in the gig economy differ by gender, race and age? | Case studies of Deliveroo and Uber drivers in London |
Receive feedback on language, structure, and formatting
Professional editors proofread and edit your paper by focusing on:
- Academic style
- Vague sentences
- Style consistency
See an example
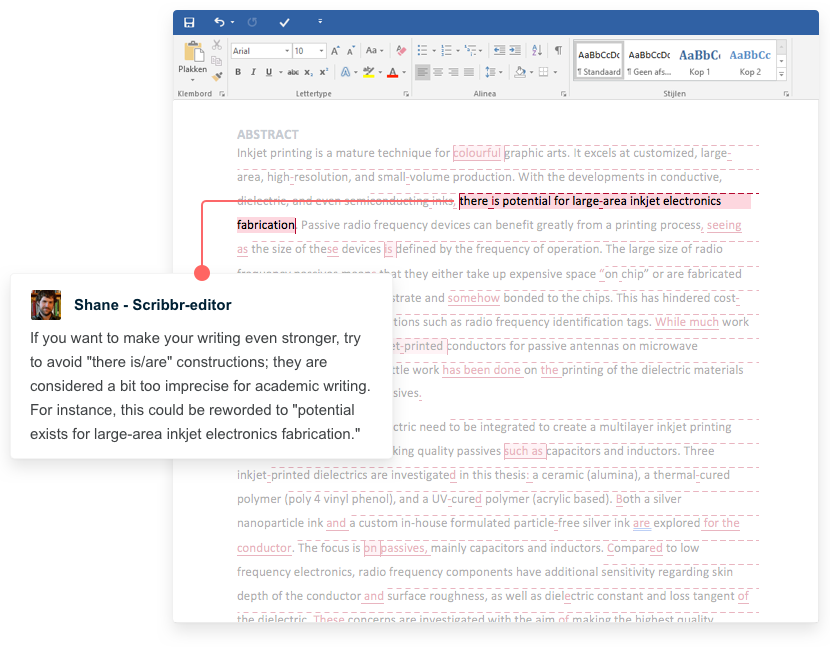
Once you have developed your problem statement and research questions , you should be ready to choose the specific case that you want to focus on. A good case study should have the potential to:
- Provide new or unexpected insights into the subject
- Challenge or complicate existing assumptions and theories
- Propose practical courses of action to resolve a problem
- Open up new directions for future research
TipIf your research is more practical in nature and aims to simultaneously investigate an issue as you solve it, consider conducting action research instead.
Unlike quantitative or experimental research , a strong case study does not require a random or representative sample. In fact, case studies often deliberately focus on unusual, neglected, or outlying cases which may shed new light on the research problem.
Example of an outlying case studyIn the 1960s the town of Roseto, Pennsylvania was discovered to have extremely low rates of heart disease compared to the US average. It became an important case study for understanding previously neglected causes of heart disease.
However, you can also choose a more common or representative case to exemplify a particular category, experience or phenomenon.
Example of a representative case studyIn the 1920s, two sociologists used Muncie, Indiana as a case study of a typical American city that supposedly exemplified the changing culture of the US at the time.
While case studies focus more on concrete details than general theories, they should usually have some connection with theory in the field. This way the case study is not just an isolated description, but is integrated into existing knowledge about the topic. It might aim to:
- Exemplify a theory by showing how it explains the case under investigation
- Expand on a theory by uncovering new concepts and ideas that need to be incorporated
- Challenge a theory by exploring an outlier case that doesn’t fit with established assumptions
To ensure that your analysis of the case has a solid academic grounding, you should conduct a literature review of sources related to the topic and develop a theoretical framework . This means identifying key concepts and theories to guide your analysis and interpretation.
There are many different research methods you can use to collect data on your subject. Case studies tend to focus on qualitative data using methods such as interviews , observations , and analysis of primary and secondary sources (e.g., newspaper articles, photographs, official records). Sometimes a case study will also collect quantitative data.
Example of a mixed methods case studyFor a case study of a wind farm development in a rural area, you could collect quantitative data on employment rates and business revenue, collect qualitative data on local people’s perceptions and experiences, and analyze local and national media coverage of the development.
The aim is to gain as thorough an understanding as possible of the case and its context.
Here's why students love Scribbr's proofreading services
Discover proofreading & editing
In writing up the case study, you need to bring together all the relevant aspects to give as complete a picture as possible of the subject.
How you report your findings depends on the type of research you are doing. Some case studies are structured like a standard scientific paper or thesis , with separate sections or chapters for the methods , results and discussion .
Others are written in a more narrative style, aiming to explore the case from various angles and analyze its meanings and implications (for example, by using textual analysis or discourse analysis ).
In all cases, though, make sure to give contextual details about the case, connect it back to the literature and theory, and discuss how it fits into wider patterns or debates.
If you want to know more about statistics , methodology , or research bias , make sure to check out some of our other articles with explanations and examples.
- Normal distribution
- Degrees of freedom
- Null hypothesis
- Discourse analysis
- Control groups
- Mixed methods research
- Non-probability sampling
- Quantitative research
- Ecological validity
Research bias
- Rosenthal effect
- Implicit bias
- Cognitive bias
- Selection bias
- Negativity bias
- Status quo bias
Cite this Scribbr article
If you want to cite this source, you can copy and paste the citation or click the “Cite this Scribbr article” button to automatically add the citation to our free Citation Generator.
McCombes, S. (2023, November 20). What Is a Case Study? | Definition, Examples & Methods. Scribbr. Retrieved October 14, 2024, from https://www.scribbr.com/methodology/case-study/
Is this article helpful?
Shona McCombes
Other students also liked, primary vs. secondary sources | difference & examples, what is a theoretical framework | guide to organizing, what is action research | definition & examples, "i thought ai proofreading was useless but..".
I've been using Scribbr for years now and I know it's a service that won't disappoint. It does a good job spotting mistakes”
Case Study Research Method in Psychology
Saul McLeod, PhD
Editor-in-Chief for Simply Psychology
BSc (Hons) Psychology, MRes, PhD, University of Manchester
Saul McLeod, PhD., is a qualified psychology teacher with over 18 years of experience in further and higher education. He has been published in peer-reviewed journals, including the Journal of Clinical Psychology.
Learn about our Editorial Process
Olivia Guy-Evans, MSc
Associate Editor for Simply Psychology
BSc (Hons) Psychology, MSc Psychology of Education
Olivia Guy-Evans is a writer and associate editor for Simply Psychology. She has previously worked in healthcare and educational sectors.
On This Page:
Case studies are in-depth investigations of a person, group, event, or community. Typically, data is gathered from various sources using several methods (e.g., observations & interviews).
The case study research method originated in clinical medicine (the case history, i.e., the patient’s personal history). In psychology, case studies are often confined to the study of a particular individual.
The information is mainly biographical and relates to events in the individual’s past (i.e., retrospective), as well as to significant events that are currently occurring in his or her everyday life.
The case study is not a research method, but researchers select methods of data collection and analysis that will generate material suitable for case studies.
Freud (1909a, 1909b) conducted very detailed investigations into the private lives of his patients in an attempt to both understand and help them overcome their illnesses.
This makes it clear that the case study is a method that should only be used by a psychologist, therapist, or psychiatrist, i.e., someone with a professional qualification.
There is an ethical issue of competence. Only someone qualified to diagnose and treat a person can conduct a formal case study relating to atypical (i.e., abnormal) behavior or atypical development.
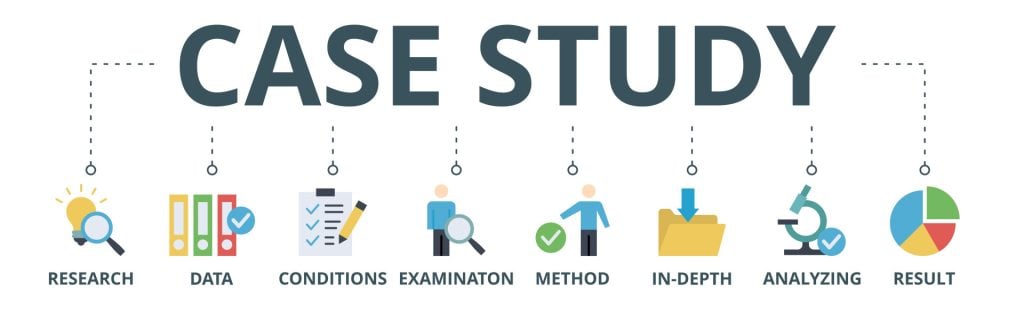
Famous Case Studies
- Anna O – One of the most famous case studies, documenting psychoanalyst Josef Breuer’s treatment of “Anna O” (real name Bertha Pappenheim) for hysteria in the late 1800s using early psychoanalytic theory.
- Little Hans – A child psychoanalysis case study published by Sigmund Freud in 1909 analyzing his five-year-old patient Herbert Graf’s house phobia as related to the Oedipus complex.
- Bruce/Brenda – Gender identity case of the boy (Bruce) whose botched circumcision led psychologist John Money to advise gender reassignment and raise him as a girl (Brenda) in the 1960s.
- Genie Wiley – Linguistics/psychological development case of the victim of extreme isolation abuse who was studied in 1970s California for effects of early language deprivation on acquiring speech later in life.
- Phineas Gage – One of the most famous neuropsychology case studies analyzes personality changes in railroad worker Phineas Gage after an 1848 brain injury involving a tamping iron piercing his skull.
Clinical Case Studies
- Studying the effectiveness of psychotherapy approaches with an individual patient
- Assessing and treating mental illnesses like depression, anxiety disorders, PTSD
- Neuropsychological cases investigating brain injuries or disorders
Child Psychology Case Studies
- Studying psychological development from birth through adolescence
- Cases of learning disabilities, autism spectrum disorders, ADHD
- Effects of trauma, abuse, deprivation on development
Types of Case Studies
- Explanatory case studies : Used to explore causation in order to find underlying principles. Helpful for doing qualitative analysis to explain presumed causal links.
- Exploratory case studies : Used to explore situations where an intervention being evaluated has no clear set of outcomes. It helps define questions and hypotheses for future research.
- Descriptive case studies : Describe an intervention or phenomenon and the real-life context in which it occurred. It is helpful for illustrating certain topics within an evaluation.
- Multiple-case studies : Used to explore differences between cases and replicate findings across cases. Helpful for comparing and contrasting specific cases.
- Intrinsic : Used to gain a better understanding of a particular case. Helpful for capturing the complexity of a single case.
- Collective : Used to explore a general phenomenon using multiple case studies. Helpful for jointly studying a group of cases in order to inquire into the phenomenon.
Where Do You Find Data for a Case Study?
There are several places to find data for a case study. The key is to gather data from multiple sources to get a complete picture of the case and corroborate facts or findings through triangulation of evidence. Most of this information is likely qualitative (i.e., verbal description rather than measurement), but the psychologist might also collect numerical data.
1. Primary sources
- Interviews – Interviewing key people related to the case to get their perspectives and insights. The interview is an extremely effective procedure for obtaining information about an individual, and it may be used to collect comments from the person’s friends, parents, employer, workmates, and others who have a good knowledge of the person, as well as to obtain facts from the person him or herself.
- Observations – Observing behaviors, interactions, processes, etc., related to the case as they unfold in real-time.
- Documents & Records – Reviewing private documents, diaries, public records, correspondence, meeting minutes, etc., relevant to the case.
2. Secondary sources
- News/Media – News coverage of events related to the case study.
- Academic articles – Journal articles, dissertations etc. that discuss the case.
- Government reports – Official data and records related to the case context.
- Books/films – Books, documentaries or films discussing the case.
3. Archival records
Searching historical archives, museum collections and databases to find relevant documents, visual/audio records related to the case history and context.
Public archives like newspapers, organizational records, photographic collections could all include potentially relevant pieces of information to shed light on attitudes, cultural perspectives, common practices and historical contexts related to psychology.
4. Organizational records
Organizational records offer the advantage of often having large datasets collected over time that can reveal or confirm psychological insights.
Of course, privacy and ethical concerns regarding confidential data must be navigated carefully.
However, with proper protocols, organizational records can provide invaluable context and empirical depth to qualitative case studies exploring the intersection of psychology and organizations.
- Organizational/industrial psychology research : Organizational records like employee surveys, turnover/retention data, policies, incident reports etc. may provide insight into topics like job satisfaction, workplace culture and dynamics, leadership issues, employee behaviors etc.
- Clinical psychology : Therapists/hospitals may grant access to anonymized medical records to study aspects like assessments, diagnoses, treatment plans etc. This could shed light on clinical practices.
- School psychology : Studies could utilize anonymized student records like test scores, grades, disciplinary issues, and counseling referrals to study child development, learning barriers, effectiveness of support programs, and more.
How do I Write a Case Study in Psychology?
Follow specified case study guidelines provided by a journal or your psychology tutor. General components of clinical case studies include: background, symptoms, assessments, diagnosis, treatment, and outcomes. Interpreting the information means the researcher decides what to include or leave out. A good case study should always clarify which information is the factual description and which is an inference or the researcher’s opinion.
1. Introduction
- Provide background on the case context and why it is of interest, presenting background information like demographics, relevant history, and presenting problem.
- Compare briefly to similar published cases if applicable. Clearly state the focus/importance of the case.
2. Case Presentation
- Describe the presenting problem in detail, including symptoms, duration,and impact on daily life.
- Include client demographics like age and gender, information about social relationships, and mental health history.
- Describe all physical, emotional, and/or sensory symptoms reported by the client.
- Use patient quotes to describe the initial complaint verbatim. Follow with full-sentence summaries of relevant history details gathered, including key components that led to a working diagnosis.
- Summarize clinical exam results, namely orthopedic/neurological tests, imaging, lab tests, etc. Note actual results rather than subjective conclusions. Provide images if clearly reproducible/anonymized.
- Clearly state the working diagnosis or clinical impression before transitioning to management.
3. Management and Outcome
- Indicate the total duration of care and number of treatments given over what timeframe. Use specific names/descriptions for any therapies/interventions applied.
- Present the results of the intervention,including any quantitative or qualitative data collected.
- For outcomes, utilize visual analog scales for pain, medication usage logs, etc., if possible. Include patient self-reports of improvement/worsening of symptoms. Note the reason for discharge/end of care.
4. Discussion
- Analyze the case, exploring contributing factors, limitations of the study, and connections to existing research.
- Analyze the effectiveness of the intervention,considering factors like participant adherence, limitations of the study, and potential alternative explanations for the results.
- Identify any questions raised in the case analysis and relate insights to established theories and current research if applicable. Avoid definitive claims about physiological explanations.
- Offer clinical implications, and suggest future research directions.
5. Additional Items
- Thank specific assistants for writing support only. No patient acknowledgments.
- References should directly support any key claims or quotes included.
- Use tables/figures/images only if substantially informative. Include permissions and legends/explanatory notes.
- Provides detailed (rich qualitative) information.
- Provides insight for further research.
- Permitting investigation of otherwise impractical (or unethical) situations.
Case studies allow a researcher to investigate a topic in far more detail than might be possible if they were trying to deal with a large number of research participants (nomothetic approach) with the aim of ‘averaging’.
Because of their in-depth, multi-sided approach, case studies often shed light on aspects of human thinking and behavior that would be unethical or impractical to study in other ways.
Research that only looks into the measurable aspects of human behavior is not likely to give us insights into the subjective dimension of experience, which is important to psychoanalytic and humanistic psychologists.
Case studies are often used in exploratory research. They can help us generate new ideas (that might be tested by other methods). They are an important way of illustrating theories and can help show how different aspects of a person’s life are related to each other.
The method is, therefore, important for psychologists who adopt a holistic point of view (i.e., humanistic psychologists ).
Limitations
- Lacking scientific rigor and providing little basis for generalization of results to the wider population.
- Researchers’ own subjective feelings may influence the case study (researcher bias).
- Difficult to replicate.
- Time-consuming and expensive.
- The volume of data, together with the time restrictions in place, impacted the depth of analysis that was possible within the available resources.
Because a case study deals with only one person/event/group, we can never be sure if the case study investigated is representative of the wider body of “similar” instances. This means the conclusions drawn from a particular case may not be transferable to other settings.
Because case studies are based on the analysis of qualitative (i.e., descriptive) data , a lot depends on the psychologist’s interpretation of the information she has acquired.
This means that there is a lot of scope for Anna O , and it could be that the subjective opinions of the psychologist intrude in the assessment of what the data means.
For example, Freud has been criticized for producing case studies in which the information was sometimes distorted to fit particular behavioral theories (e.g., Little Hans ).
This is also true of Money’s interpretation of the Bruce/Brenda case study (Diamond, 1997) when he ignored evidence that went against his theory.
Breuer, J., & Freud, S. (1895). Studies on hysteria . Standard Edition 2: London.
Curtiss, S. (1981). Genie: The case of a modern wild child .
Diamond, M., & Sigmundson, K. (1997). Sex Reassignment at Birth: Long-term Review and Clinical Implications. Archives of Pediatrics & Adolescent Medicine , 151(3), 298-304
Freud, S. (1909a). Analysis of a phobia of a five year old boy. In The Pelican Freud Library (1977), Vol 8, Case Histories 1, pages 169-306
Freud, S. (1909b). Bemerkungen über einen Fall von Zwangsneurose (Der “Rattenmann”). Jb. psychoanal. psychopathol. Forsch ., I, p. 357-421; GW, VII, p. 379-463; Notes upon a case of obsessional neurosis, SE , 10: 151-318.
Harlow J. M. (1848). Passage of an iron rod through the head. Boston Medical and Surgical Journal, 39 , 389–393.
Harlow, J. M. (1868). Recovery from the Passage of an Iron Bar through the Head . Publications of the Massachusetts Medical Society. 2 (3), 327-347.
Money, J., & Ehrhardt, A. A. (1972). Man & Woman, Boy & Girl : The Differentiation and Dimorphism of Gender Identity from Conception to Maturity. Baltimore, Maryland: Johns Hopkins University Press.
Money, J., & Tucker, P. (1975). Sexual signatures: On being a man or a woman.
Further Information
- Case Study Approach
- Case Study Method
- Enhancing the Quality of Case Studies in Health Services Research
- “We do things together” A case study of “couplehood” in dementia
- Using mixed methods for evaluating an integrative approach to cancer care: a case study

Educational resources and simple solutions for your research journey

What is a Case Study in Research? Definition, Methods, and Examples
Case study methodology offers researchers an exciting opportunity to explore intricate phenomena within specific contexts using a wide range of data sources and collection methods. It is highly pertinent in health and social sciences, environmental studies, social work, education, and business studies. Its diverse applications, such as advancing theory, program evaluation, and intervention development, make it an invaluable tool for driving meaningful research and fostering positive change.[ 1]
Table of Contents
What is a Case Study?
A case study method involves a detailed examination of a single subject, such as an individual, group, organization, event, or community, to explore and understand complex issues in real-life contexts. By focusing on one specific case, researchers can gain a deep understanding of the factors and dynamics at play, understanding their complex relationships, which might be missed in broader, more quantitative studies.
When to do a Case Study?
A case study design is useful when you want to explore a phenomenon in-depth and in its natural context. Here are some examples of when to use a case study :[ 2]
- Exploratory Research: When you want to explore a new topic or phenomenon, a case study can help you understand the subject deeply. For example , a researcher studying a newly discovered plant species might use a case study to document its characteristics and behavior.
- Descriptive Research: If you want to describe a complex phenomenon or process, a case study can provide a detailed and comprehensive description. For instance, a case study design could describe the experiences of a group of individuals living with a rare disease.
- Explanatory Research: When you want to understand why a particular phenomenon occurs, a case study can help you identify causal relationships. A case study design could investigate the reasons behind the success or failure of a particular business strategy.
- Theory Building: Case studies can also be used to develop or refine theories. By systematically analyzing a series of cases, researchers can identify patterns and relationships that can contribute to developing new theories or refining existing ones.
- Critical Instance: Sometimes, a single case can be used to study a rare or unusual phenomenon, but it is important for theoretical or practical reasons. For example , the case of Phineas Gage, a man who survived a severe brain injury, has been widely studied to understand the relationship between the brain and behavior.
- Comparative Analysis: Case studies can also compare different cases or contexts. A case study example involves comparing the implementation of a particular policy in different countries to understand its effectiveness and identifying best practices.

How to Create a Case Study – Step by Step
Step 1: select a case .
Careful case selection ensures relevance, insight, and meaningful contribution to existing knowledge in your field. Here’s how you can choose a case study design :[ 3]
- Define Your Objectives: Clarify the purpose of your case study and what you hope to achieve. Do you want to provide new insights, challenge existing theories, propose solutions to a problem, or explore new research directions?
- Consider Unusual or Outlying Cases: Focus on unusual, neglected, or outlying cases that can provide unique insights.
- Choose a Representative Case: Alternatively, select a common or representative case to exemplify a particular category, experience, or phenomenon.
- Avoid Bias: Ensure your selection process is unbiased using random or criteria-based selection.
- Be Clear and Specific: Clearly define the boundaries of your study design , including the scope, timeframe, and key stakeholders.
- Ethical Considerations: Consider ethical issues, such as confidentiality and informed consent.
Step 2: Build a Theoretical Framework
To ensure your case study has a solid academic foundation, it’s important to build a theoretical framework:
- Conduct a Literature Review: Identify key concepts and theories relevant to your case study .
- Establish Connections with Theory: Connect your case study with existing theories in the field.
- Guide Your Analysis and Interpretation: Use your theoretical framework to guide your analysis, ensuring your findings are grounded in established theories and concepts.
Step 3: Collect Your Data
To conduct a comprehensive case study , you can use various research methods. These include interviews, observations, primary and secondary sources analysis, surveys, and a mixed methods approach. The aim is to gather rich and diverse data to enable a detailed analysis of your case study .
Step 4: Describe and Analyze the Case
How you report your findings will depend on the type of research you’re conducting. Here are two approaches:
- Structured Approach: Follows a scientific paper format, making it easier for readers to follow your argument.
- Narrative Approach: A more exploratory style aiming to analyze meanings and implications.
Regardless of the approach you choose, it’s important to include the following elements in your case study :
- Contextual Details: Provide background information about the case, including relevant historical, cultural, and social factors that may have influenced the outcome.
- Literature and Theory: Connect your case study to existing literature and theory in the field. Discuss how your findings contribute to or challenge existing knowledge.
- Wider Patterns or Debates: Consider how your case study fits into wider patterns or debates within the field. Discuss any implications your findings may have for future research or practice.
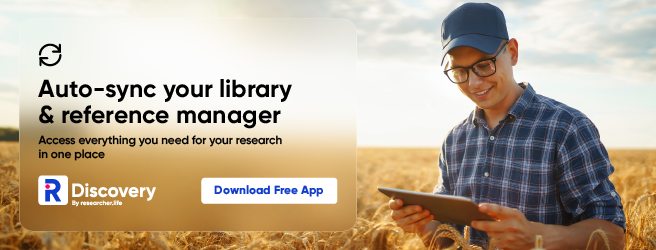
What Are the Benefits of a Case Study
Case studies offer a range of benefits , making them a powerful tool in research.
1. In-Depth Analysis
- Comprehensive Understanding: Case studies allow researchers to thoroughly explore a subject, understanding the complexities and nuances involved.
- Rich Data: They offer rich qualitative and sometimes quantitative data, capturing the intricacies of real-life contexts.
2. Contextual Insight
- Real-World Application: Case studies provide insights into real-world applications, making the findings highly relevant and practical.
- Context-Specific: They highlight how various factors interact within a specific context, offering a detailed picture of the situation.
3. Flexibility
- Methodological Diversity: Case studies can use various data collection methods, including interviews, observations, document analysis, and surveys.
- Adaptability: Researchers can adapt the case study approach to fit the specific needs and circumstances of the research.
4. Practical Solutions
- Actionable Insights: The detailed findings from case studies can inform practical solutions and recommendations for practitioners and policymakers.
- Problem-Solving: They help understand the root causes of problems and devise effective strategies to address them.
5. Unique Cases
- Rare Phenomena: Case studies are particularly valuable for studying rare or unique cases that other research methods may not capture.
- Detailed Documentation: They document and preserve detailed information about specific instances that might otherwise be overlooked.
What Are the Limitations of a Case Study
While case studies offer valuable insights and a detailed understanding of complex issues, they have several limitations .
1. Limited Generalizability
- Specific Context: Case studies often focus on a single case or a small number of cases, which may limit the generalization of findings to broader populations or different contexts.
- Unique Situations: The unique characteristics of the case may not be representative of other situations, reducing the applicability of the results.
2. Subjectivity
- Researcher Bias: The researcher’s perspectives and interpretations can influence the analysis and conclusions, potentially introducing bias.
- Participant Bias: Participants’ responses and behaviors may be influenced by their awareness of being studied, known as the Hawthorne effect.
3. Time-Consuming
- Data Collection and Analysis: Gathering detailed, in-depth data requires significant time and effort, making case studies more time-consuming than other research methods.
- Longitudinal Studies: If the case study observes changes over time, it can become even more prolonged.
4. Resource Intensive
- Financial and Human Resources: Conducting comprehensive case studies may require significant financial investment and human resources, including trained researchers and participant access.
- Access to Data: Accessing relevant and reliable data sources can be challenging, particularly in sensitive or proprietary contexts.
5. Replication Difficulties
- Unique Contexts: A case study’s specific and detailed context makes it difficult to replicate the study exactly, limiting the ability to validate findings through repetition.
- Variability: Differences in contexts, researchers, and methodologies can lead to variations in findings, complicating efforts to achieve consistent results.
By acknowledging and addressing these limitations , researchers can enhance the rigor and reliability of their case study findings.
Key Takeaways
Case studies are valuable in research because they provide an in-depth, contextual analysis of a single subject, event, or organization. They allow researchers to explore complex issues in real-world settings, capturing detailed qualitative and quantitative data. This method is useful for generating insights, developing theories, and offering practical solutions to problems. They are versatile, applicable in diverse fields such as business, education, and health, and can complement other research methods by providing rich, contextual evidence. However, their findings may have limited generalizability due to the focus on a specific case.
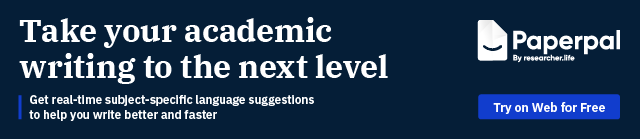
Frequently Asked Questions
Q: What is a case study in research?
A case study in research is an impactful tool for gaining a deep understanding of complex issues within their real-life context. It combines various data collection methods and provides rich, detailed insights that can inform theory development and practical applications.
Q: What are the advantages of using case studies in research?
Case studies are a powerful research method, offering advantages such as in-depth analysis, contextual insights, flexibility, rich data, and the ability to handle complex issues. They are particularly valuable for exploring new areas, generating hypotheses, and providing detailed, illustrative examples that can inform theory and practice.
Q: Can case studies be used in quantitative research?
While case studies are predominantly associated with qualitative research, they can effectively incorporate quantitative methods to provide a more comprehensive analysis. A mixed-methods approach leverages qualitative and quantitative research strengths, offering a powerful tool for exploring complex issues in a real-world context. For example , a new medical treatment case study can incorporate quantitative clinical outcomes (e.g., patient recovery rates and dosage levels) along with qualitative patient interviews.
Q: What are the key components of a case study?
A case study typically includes several key components:
- Introductio n, which provides an overview and sets the context by presenting the problem statement and research objectives;
- Literature review , which connects the study to existing theories and prior research;
- Methodology , which details the case study design , data collection methods, and analysis techniques;
- Findings , which present the data and results, including descriptions, patterns, and themes;
- Discussion and conclusion , which interpret the findings, discuss their implications, and offer conclusions, practical applications, limitations, and suggestions for future research.
Together, these components ensure a comprehensive, systematic, and insightful exploration of the case.
References
- de Vries, K. (2020). Case study methodology. In Critical qualitative health research (pp. 41-52). Routledge.
- Fidel, R. (1984). The case study method: A case study. Library and Information Science Research , 6 (3), 273-288.
- Thomas, G. (2021). How to do your case study. How to do your case study , 1-320.
Editage All Access is a subscription-based platform that unifies the best AI tools and services designed to speed up, simplify, and streamline every step of a researcher’s journey. The Editage All Access Pack is a one-of-a-kind subscription that unlocks full access to an AI writing assistant, literature recommender, journal finder, scientific illustration tool, and exclusive discounts on professional publication services from Editage.
Based on 22+ years of experience in academia, Editage All Access empowers researchers to put their best research forward and move closer to success. Explore our top AI Tools pack, AI Tools + Publication Services pack, or Build Your Own Plan. Find everything a researcher needs to succeed, all in one place – Get All Access now starting at just $14 a month !
Related Posts

Conference Paper vs. Journal Paper: What’s the Difference

What is Availability Heuristic (with Examples)
- Bipolar Disorder
- Therapy Center
- When To See a Therapist
- Types of Therapy
- Best Online Therapy
- Best Couples Therapy
- Managing Stress
- Sleep and Dreaming
- Understanding Emotions
- Self-Improvement
- Healthy Relationships
- Student Resources
- Personality Types
- Guided Meditations
- Verywell Mind Insights
- 2024 Verywell Mind 25
- Mental Health in the Classroom
- Editorial Process
- Meet Our Review Board
- Crisis Support
What Is a Case Study?
Weighing the pros and cons of this method of research
Kendra Cherry, MS, is a psychosocial rehabilitation specialist, psychology educator, and author of the "Everything Psychology Book."
:max_bytes(150000):strip_icc():format(webp)/IMG_9791-89504ab694d54b66bbd72cb84ffb860e.jpg)
Cara Lustik is a fact-checker and copywriter.
:max_bytes(150000):strip_icc():format(webp)/Cara-Lustik-1000-77abe13cf6c14a34a58c2a0ffb7297da.jpg)
Verywell / Colleen Tighe
- Pros and Cons
What Types of Case Studies Are Out There?
Where do you find data for a case study, how do i write a psychology case study.
A case study is an in-depth study of one person, group, or event. In a case study, nearly every aspect of the subject's life and history is analyzed to seek patterns and causes of behavior. Case studies can be used in many different fields, including psychology, medicine, education, anthropology, political science, and social work.
The point of a case study is to learn as much as possible about an individual or group so that the information can be generalized to many others. Unfortunately, case studies tend to be highly subjective, and it is sometimes difficult to generalize results to a larger population.
While case studies focus on a single individual or group, they follow a format similar to other types of psychology writing. If you are writing a case study, we got you—here are some rules of APA format to reference.
At a Glance
A case study, or an in-depth study of a person, group, or event, can be a useful research tool when used wisely. In many cases, case studies are best used in situations where it would be difficult or impossible for you to conduct an experiment. They are helpful for looking at unique situations and allow researchers to gather a lot of˜ information about a specific individual or group of people. However, it's important to be cautious of any bias we draw from them as they are highly subjective.
What Are the Benefits and Limitations of Case Studies?
A case study can have its strengths and weaknesses. Researchers must consider these pros and cons before deciding if this type of study is appropriate for their needs.
One of the greatest advantages of a case study is that it allows researchers to investigate things that are often difficult or impossible to replicate in a lab. Some other benefits of a case study:
- Allows researchers to capture information on the 'how,' 'what,' and 'why,' of something that's implemented
- Gives researchers the chance to collect information on why one strategy might be chosen over another
- Permits researchers to develop hypotheses that can be explored in experimental research
On the other hand, a case study can have some drawbacks:
- It cannot necessarily be generalized to the larger population
- Cannot demonstrate cause and effect
- It may not be scientifically rigorous
- It can lead to bias
Researchers may choose to perform a case study if they want to explore a unique or recently discovered phenomenon. Through their insights, researchers develop additional ideas and study questions that might be explored in future studies.
It's important to remember that the insights from case studies cannot be used to determine cause-and-effect relationships between variables. However, case studies may be used to develop hypotheses that can then be addressed in experimental research.
Case Study Examples
There have been a number of notable case studies in the history of psychology. Much of Freud's work and theories were developed through individual case studies. Some great examples of case studies in psychology include:
- Anna O : Anna O. was a pseudonym of a woman named Bertha Pappenheim, a patient of a physician named Josef Breuer. While she was never a patient of Freud's, Freud and Breuer discussed her case extensively. The woman was experiencing symptoms of a condition that was then known as hysteria and found that talking about her problems helped relieve her symptoms. Her case played an important part in the development of talk therapy as an approach to mental health treatment.
- Phineas Gage : Phineas Gage was a railroad employee who experienced a terrible accident in which an explosion sent a metal rod through his skull, damaging important portions of his brain. Gage recovered from his accident but was left with serious changes in both personality and behavior.
- Genie : Genie was a young girl subjected to horrific abuse and isolation. The case study of Genie allowed researchers to study whether language learning was possible, even after missing critical periods for language development. Her case also served as an example of how scientific research may interfere with treatment and lead to further abuse of vulnerable individuals.
Such cases demonstrate how case research can be used to study things that researchers could not replicate in experimental settings. In Genie's case, her horrific abuse denied her the opportunity to learn a language at critical points in her development.
This is clearly not something researchers could ethically replicate, but conducting a case study on Genie allowed researchers to study phenomena that are otherwise impossible to reproduce.
There are a few different types of case studies that psychologists and other researchers might use:
- Collective case studies : These involve studying a group of individuals. Researchers might study a group of people in a certain setting or look at an entire community. For example, psychologists might explore how access to resources in a community has affected the collective mental well-being of those who live there.
- Descriptive case studies : These involve starting with a descriptive theory. The subjects are then observed, and the information gathered is compared to the pre-existing theory.
- Explanatory case studies : These are often used to do causal investigations. In other words, researchers are interested in looking at factors that may have caused certain things to occur.
- Exploratory case studies : These are sometimes used as a prelude to further, more in-depth research. This allows researchers to gather more information before developing their research questions and hypotheses .
- Instrumental case studies : These occur when the individual or group allows researchers to understand more than what is initially obvious to observers.
- Intrinsic case studies : This type of case study is when the researcher has a personal interest in the case. Jean Piaget's observations of his own children are good examples of how an intrinsic case study can contribute to the development of a psychological theory.
The three main case study types often used are intrinsic, instrumental, and collective. Intrinsic case studies are useful for learning about unique cases. Instrumental case studies help look at an individual to learn more about a broader issue. A collective case study can be useful for looking at several cases simultaneously.
The type of case study that psychology researchers use depends on the unique characteristics of the situation and the case itself.
There are a number of different sources and methods that researchers can use to gather information about an individual or group. Six major sources that have been identified by researchers are:
- Archival records : Census records, survey records, and name lists are examples of archival records.
- Direct observation : This strategy involves observing the subject, often in a natural setting . While an individual observer is sometimes used, it is more common to utilize a group of observers.
- Documents : Letters, newspaper articles, administrative records, etc., are the types of documents often used as sources.
- Interviews : Interviews are one of the most important methods for gathering information in case studies. An interview can involve structured survey questions or more open-ended questions.
- Participant observation : When the researcher serves as a participant in events and observes the actions and outcomes, it is called participant observation.
- Physical artifacts : Tools, objects, instruments, and other artifacts are often observed during a direct observation of the subject.
If you have been directed to write a case study for a psychology course, be sure to check with your instructor for any specific guidelines you need to follow. If you are writing your case study for a professional publication, check with the publisher for their specific guidelines for submitting a case study.
Here is a general outline of what should be included in a case study.
Section 1: A Case History
This section will have the following structure and content:
Background information : The first section of your paper will present your client's background. Include factors such as age, gender, work, health status, family mental health history, family and social relationships, drug and alcohol history, life difficulties, goals, and coping skills and weaknesses.
Description of the presenting problem : In the next section of your case study, you will describe the problem or symptoms that the client presented with.
Describe any physical, emotional, or sensory symptoms reported by the client. Thoughts, feelings, and perceptions related to the symptoms should also be noted. Any screening or diagnostic assessments that are used should also be described in detail and all scores reported.
Your diagnosis : Provide your diagnosis and give the appropriate Diagnostic and Statistical Manual code. Explain how you reached your diagnosis, how the client's symptoms fit the diagnostic criteria for the disorder(s), or any possible difficulties in reaching a diagnosis.
Section 2: Treatment Plan
This portion of the paper will address the chosen treatment for the condition. This might also include the theoretical basis for the chosen treatment or any other evidence that might exist to support why this approach was chosen.
- Cognitive behavioral approach : Explain how a cognitive behavioral therapist would approach treatment. Offer background information on cognitive behavioral therapy and describe the treatment sessions, client response, and outcome of this type of treatment. Make note of any difficulties or successes encountered by your client during treatment.
- Humanistic approach : Describe a humanistic approach that could be used to treat your client, such as client-centered therapy . Provide information on the type of treatment you chose, the client's reaction to the treatment, and the end result of this approach. Explain why the treatment was successful or unsuccessful.
- Psychoanalytic approach : Describe how a psychoanalytic therapist would view the client's problem. Provide some background on the psychoanalytic approach and cite relevant references. Explain how psychoanalytic therapy would be used to treat the client, how the client would respond to therapy, and the effectiveness of this treatment approach.
- Pharmacological approach : If treatment primarily involves the use of medications, explain which medications were used and why. Provide background on the effectiveness of these medications and how monotherapy may compare with an approach that combines medications with therapy or other treatments.
This section of a case study should also include information about the treatment goals, process, and outcomes.
When you are writing a case study, you should also include a section where you discuss the case study itself, including the strengths and limitiations of the study. You should note how the findings of your case study might support previous research.
In your discussion section, you should also describe some of the implications of your case study. What ideas or findings might require further exploration? How might researchers go about exploring some of these questions in additional studies?
Need More Tips?
Here are a few additional pointers to keep in mind when formatting your case study:
- Never refer to the subject of your case study as "the client." Instead, use their name or a pseudonym.
- Read examples of case studies to gain an idea about the style and format.
- Remember to use APA format when citing references .
Crowe S, Cresswell K, Robertson A, Huby G, Avery A, Sheikh A. The case study approach . BMC Med Res Methodol . 2011;11:100.
Crowe S, Cresswell K, Robertson A, Huby G, Avery A, Sheikh A. The case study approach . BMC Med Res Methodol . 2011 Jun 27;11:100. doi:10.1186/1471-2288-11-100
Gagnon, Yves-Chantal. The Case Study as Research Method: A Practical Handbook . Canada, Chicago Review Press Incorporated DBA Independent Pub Group, 2010.
Yin, Robert K. Case Study Research and Applications: Design and Methods . United States, SAGE Publications, 2017.
By Kendra Cherry, MSEd Kendra Cherry, MS, is a psychosocial rehabilitation specialist, psychology educator, and author of the "Everything Psychology Book."

The Ultimate Guide to Qualitative Research - Part 1: The Basics
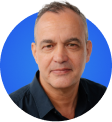
- Introduction and overview
- What is qualitative research?
- What is qualitative data?
- Examples of qualitative data
- Qualitative vs. quantitative research
- Mixed methods
- Qualitative research preparation
- Theoretical perspective
- Theoretical framework
- Literature reviews
Research question
- Conceptual framework
- Conceptual vs. theoretical framework
Data collection
- Qualitative research methods
- Focus groups
- Observational research
What is a case study?
Applications for case study research, what is a good case study, process of case study design, benefits and limitations of case studies.
- Ethnographical research
- Ethical considerations
- Confidentiality and privacy
- Power dynamics
- Reflexivity
Case studies
Case studies are essential to qualitative research , offering a lens through which researchers can investigate complex phenomena within their real-life contexts. This chapter explores the concept, purpose, applications, examples, and types of case studies and provides guidance on how to conduct case study research effectively.
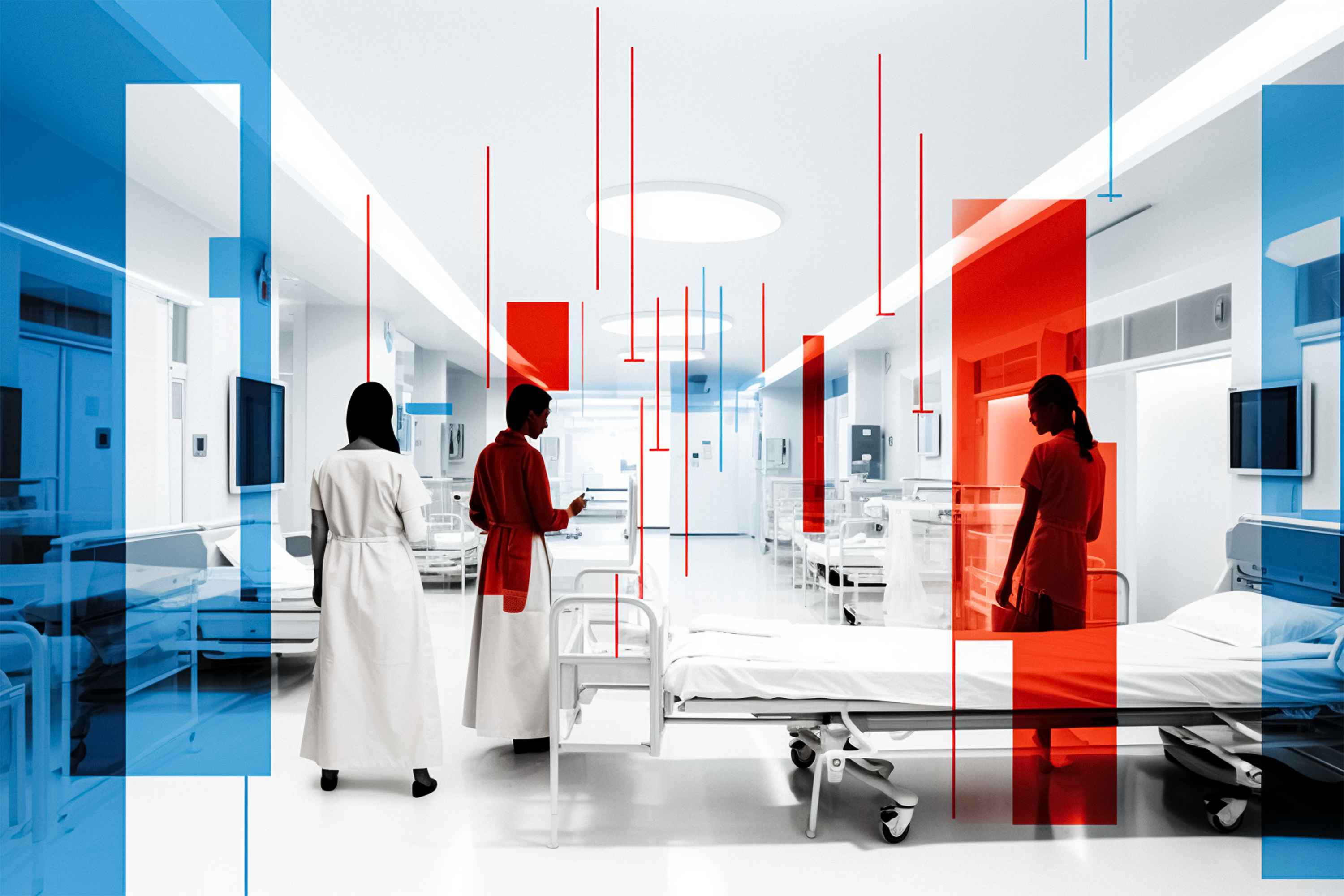
Whereas quantitative methods look at phenomena at scale, case study research looks at a concept or phenomenon in considerable detail. While analyzing a single case can help understand one perspective regarding the object of research inquiry, analyzing multiple cases can help obtain a more holistic sense of the topic or issue. Let's provide a basic definition of a case study, then explore its characteristics and role in the qualitative research process.
Definition of a case study
A case study in qualitative research is a strategy of inquiry that involves an in-depth investigation of a phenomenon within its real-world context. It provides researchers with the opportunity to acquire an in-depth understanding of intricate details that might not be as apparent or accessible through other methods of research. The specific case or cases being studied can be a single person, group, or organization – demarcating what constitutes a relevant case worth studying depends on the researcher and their research question .
Among qualitative research methods , a case study relies on multiple sources of evidence, such as documents, artifacts, interviews , or observations , to present a complete and nuanced understanding of the phenomenon under investigation. The objective is to illuminate the readers' understanding of the phenomenon beyond its abstract statistical or theoretical explanations.
Characteristics of case studies
Case studies typically possess a number of distinct characteristics that set them apart from other research methods. These characteristics include a focus on holistic description and explanation, flexibility in the design and data collection methods, reliance on multiple sources of evidence, and emphasis on the context in which the phenomenon occurs.
Furthermore, case studies can often involve a longitudinal examination of the case, meaning they study the case over a period of time. These characteristics allow case studies to yield comprehensive, in-depth, and richly contextualized insights about the phenomenon of interest.
The role of case studies in research
Case studies hold a unique position in the broader landscape of research methods aimed at theory development. They are instrumental when the primary research interest is to gain an intensive, detailed understanding of a phenomenon in its real-life context.
In addition, case studies can serve different purposes within research - they can be used for exploratory, descriptive, or explanatory purposes, depending on the research question and objectives. This flexibility and depth make case studies a valuable tool in the toolkit of qualitative researchers.
Remember, a well-conducted case study can offer a rich, insightful contribution to both academic and practical knowledge through theory development or theory verification, thus enhancing our understanding of complex phenomena in their real-world contexts.
What is the purpose of a case study?
Case study research aims for a more comprehensive understanding of phenomena, requiring various research methods to gather information for qualitative analysis . Ultimately, a case study can allow the researcher to gain insight into a particular object of inquiry and develop a theoretical framework relevant to the research inquiry.
Why use case studies in qualitative research?
Using case studies as a research strategy depends mainly on the nature of the research question and the researcher's access to the data.
Conducting case study research provides a level of detail and contextual richness that other research methods might not offer. They are beneficial when there's a need to understand complex social phenomena within their natural contexts.
The explanatory, exploratory, and descriptive roles of case studies
Case studies can take on various roles depending on the research objectives. They can be exploratory when the research aims to discover new phenomena or define new research questions; they are descriptive when the objective is to depict a phenomenon within its context in a detailed manner; and they can be explanatory if the goal is to understand specific relationships within the studied context. Thus, the versatility of case studies allows researchers to approach their topic from different angles, offering multiple ways to uncover and interpret the data .
The impact of case studies on knowledge development
Case studies play a significant role in knowledge development across various disciplines. Analysis of cases provides an avenue for researchers to explore phenomena within their context based on the collected data.
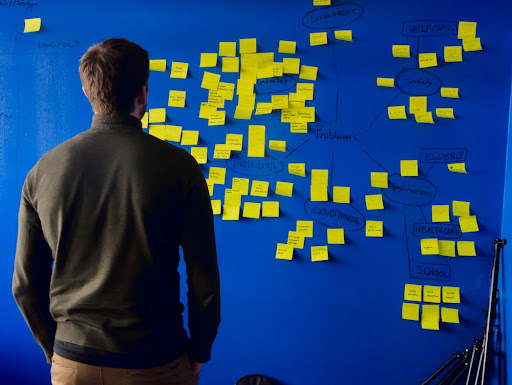
This can result in the production of rich, practical insights that can be instrumental in both theory-building and practice. Case studies allow researchers to delve into the intricacies and complexities of real-life situations, uncovering insights that might otherwise remain hidden.
Types of case studies
In qualitative research , a case study is not a one-size-fits-all approach. Depending on the nature of the research question and the specific objectives of the study, researchers might choose to use different types of case studies. These types differ in their focus, methodology, and the level of detail they provide about the phenomenon under investigation.
Understanding these types is crucial for selecting the most appropriate approach for your research project and effectively achieving your research goals. Let's briefly look at the main types of case studies.
Exploratory case studies
Exploratory case studies are typically conducted to develop a theory or framework around an understudied phenomenon. They can also serve as a precursor to a larger-scale research project. Exploratory case studies are useful when a researcher wants to identify the key issues or questions which can spur more extensive study or be used to develop propositions for further research. These case studies are characterized by flexibility, allowing researchers to explore various aspects of a phenomenon as they emerge, which can also form the foundation for subsequent studies.
Descriptive case studies
Descriptive case studies aim to provide a complete and accurate representation of a phenomenon or event within its context. These case studies are often based on an established theoretical framework, which guides how data is collected and analyzed. The researcher is concerned with describing the phenomenon in detail, as it occurs naturally, without trying to influence or manipulate it.
Explanatory case studies
Explanatory case studies are focused on explanation - they seek to clarify how or why certain phenomena occur. Often used in complex, real-life situations, they can be particularly valuable in clarifying causal relationships among concepts and understanding the interplay between different factors within a specific context.

Intrinsic, instrumental, and collective case studies
These three categories of case studies focus on the nature and purpose of the study. An intrinsic case study is conducted when a researcher has an inherent interest in the case itself. Instrumental case studies are employed when the case is used to provide insight into a particular issue or phenomenon. A collective case study, on the other hand, involves studying multiple cases simultaneously to investigate some general phenomena.
Each type of case study serves a different purpose and has its own strengths and challenges. The selection of the type should be guided by the research question and objectives, as well as the context and constraints of the research.
The flexibility, depth, and contextual richness offered by case studies make this approach an excellent research method for various fields of study. They enable researchers to investigate real-world phenomena within their specific contexts, capturing nuances that other research methods might miss. Across numerous fields, case studies provide valuable insights into complex issues.
Critical information systems research
Case studies provide a detailed understanding of the role and impact of information systems in different contexts. They offer a platform to explore how information systems are designed, implemented, and used and how they interact with various social, economic, and political factors. Case studies in this field often focus on examining the intricate relationship between technology, organizational processes, and user behavior, helping to uncover insights that can inform better system design and implementation.
Health research
Health research is another field where case studies are highly valuable. They offer a way to explore patient experiences, healthcare delivery processes, and the impact of various interventions in a real-world context.
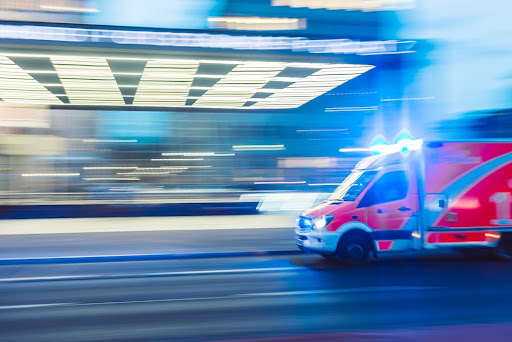
Case studies can provide a deep understanding of a patient's journey, giving insights into the intricacies of disease progression, treatment effects, and the psychosocial aspects of health and illness.
Asthma research studies
Specifically within medical research, studies on asthma often employ case studies to explore the individual and environmental factors that influence asthma development, management, and outcomes. A case study can provide rich, detailed data about individual patients' experiences, from the triggers and symptoms they experience to the effectiveness of various management strategies. This can be crucial for developing patient-centered asthma care approaches.
Other fields
Apart from the fields mentioned, case studies are also extensively used in business and management research, education research, and political sciences, among many others. They provide an opportunity to delve into the intricacies of real-world situations, allowing for a comprehensive understanding of various phenomena.
Case studies, with their depth and contextual focus, offer unique insights across these varied fields. They allow researchers to illuminate the complexities of real-life situations, contributing to both theory and practice.

Whatever field you're in, ATLAS.ti puts your data to work for you
Download a free trial of ATLAS.ti to turn your data into insights.
Understanding the key elements of case study design is crucial for conducting rigorous and impactful case study research. A well-structured design guides the researcher through the process, ensuring that the study is methodologically sound and its findings are reliable and valid. The main elements of case study design include the research question , propositions, units of analysis, and the logic linking the data to the propositions.
The research question is the foundation of any research study. A good research question guides the direction of the study and informs the selection of the case, the methods of collecting data, and the analysis techniques. A well-formulated research question in case study research is typically clear, focused, and complex enough to merit further detailed examination of the relevant case(s).
Propositions
Propositions, though not necessary in every case study, provide a direction by stating what we might expect to find in the data collected. They guide how data is collected and analyzed by helping researchers focus on specific aspects of the case. They are particularly important in explanatory case studies, which seek to understand the relationships among concepts within the studied phenomenon.
Units of analysis
The unit of analysis refers to the case, or the main entity or entities that are being analyzed in the study. In case study research, the unit of analysis can be an individual, a group, an organization, a decision, an event, or even a time period. It's crucial to clearly define the unit of analysis, as it shapes the qualitative data analysis process by allowing the researcher to analyze a particular case and synthesize analysis across multiple case studies to draw conclusions.
Argumentation
This refers to the inferential model that allows researchers to draw conclusions from the data. The researcher needs to ensure that there is a clear link between the data, the propositions (if any), and the conclusions drawn. This argumentation is what enables the researcher to make valid and credible inferences about the phenomenon under study.
Understanding and carefully considering these elements in the design phase of a case study can significantly enhance the quality of the research. It can help ensure that the study is methodologically sound and its findings contribute meaningful insights about the case.
Ready to jumpstart your research with ATLAS.ti?
Conceptualize your research project with our intuitive data analysis interface. Download a free trial today.
Conducting a case study involves several steps, from defining the research question and selecting the case to collecting and analyzing data . This section outlines these key stages, providing a practical guide on how to conduct case study research.
Defining the research question
The first step in case study research is defining a clear, focused research question. This question should guide the entire research process, from case selection to analysis. It's crucial to ensure that the research question is suitable for a case study approach. Typically, such questions are exploratory or descriptive in nature and focus on understanding a phenomenon within its real-life context.
Selecting and defining the case
The selection of the case should be based on the research question and the objectives of the study. It involves choosing a unique example or a set of examples that provide rich, in-depth data about the phenomenon under investigation. After selecting the case, it's crucial to define it clearly, setting the boundaries of the case, including the time period and the specific context.
Previous research can help guide the case study design. When considering a case study, an example of a case could be taken from previous case study research and used to define cases in a new research inquiry. Considering recently published examples can help understand how to select and define cases effectively.
Developing a detailed case study protocol
A case study protocol outlines the procedures and general rules to be followed during the case study. This includes the data collection methods to be used, the sources of data, and the procedures for analysis. Having a detailed case study protocol ensures consistency and reliability in the study.
The protocol should also consider how to work with the people involved in the research context to grant the research team access to collecting data. As mentioned in previous sections of this guide, establishing rapport is an essential component of qualitative research as it shapes the overall potential for collecting and analyzing data.
Collecting data
Gathering data in case study research often involves multiple sources of evidence, including documents, archival records, interviews, observations, and physical artifacts. This allows for a comprehensive understanding of the case. The process for gathering data should be systematic and carefully documented to ensure the reliability and validity of the study.
Analyzing and interpreting data
The next step is analyzing the data. This involves organizing the data , categorizing it into themes or patterns , and interpreting these patterns to answer the research question. The analysis might also involve comparing the findings with prior research or theoretical propositions.
Writing the case study report
The final step is writing the case study report . This should provide a detailed description of the case, the data, the analysis process, and the findings. The report should be clear, organized, and carefully written to ensure that the reader can understand the case and the conclusions drawn from it.
Each of these steps is crucial in ensuring that the case study research is rigorous, reliable, and provides valuable insights about the case.
The type, depth, and quality of data in your study can significantly influence the validity and utility of the study. In case study research, data is usually collected from multiple sources to provide a comprehensive and nuanced understanding of the case. This section will outline the various methods of collecting data used in case study research and discuss considerations for ensuring the quality of the data.
Interviews are a common method of gathering data in case study research. They can provide rich, in-depth data about the perspectives, experiences, and interpretations of the individuals involved in the case. Interviews can be structured , semi-structured , or unstructured , depending on the research question and the degree of flexibility needed.
Observations
Observations involve the researcher observing the case in its natural setting, providing first-hand information about the case and its context. Observations can provide data that might not be revealed in interviews or documents, such as non-verbal cues or contextual information.
Documents and artifacts
Documents and archival records provide a valuable source of data in case study research. They can include reports, letters, memos, meeting minutes, email correspondence, and various public and private documents related to the case.

These records can provide historical context, corroborate evidence from other sources, and offer insights into the case that might not be apparent from interviews or observations.
Physical artifacts refer to any physical evidence related to the case, such as tools, products, or physical environments. These artifacts can provide tangible insights into the case, complementing the data gathered from other sources.
Ensuring the quality of data collection
Determining the quality of data in case study research requires careful planning and execution. It's crucial to ensure that the data is reliable, accurate, and relevant to the research question. This involves selecting appropriate methods of collecting data, properly training interviewers or observers, and systematically recording and storing the data. It also includes considering ethical issues related to collecting and handling data, such as obtaining informed consent and ensuring the privacy and confidentiality of the participants.
Data analysis
Analyzing case study research involves making sense of the rich, detailed data to answer the research question. This process can be challenging due to the volume and complexity of case study data. However, a systematic and rigorous approach to analysis can ensure that the findings are credible and meaningful. This section outlines the main steps and considerations in analyzing data in case study research.
Organizing the data
The first step in the analysis is organizing the data. This involves sorting the data into manageable sections, often according to the data source or the theme. This step can also involve transcribing interviews, digitizing physical artifacts, or organizing observational data.
Categorizing and coding the data
Once the data is organized, the next step is to categorize or code the data. This involves identifying common themes, patterns, or concepts in the data and assigning codes to relevant data segments. Coding can be done manually or with the help of software tools, and in either case, qualitative analysis software can greatly facilitate the entire coding process. Coding helps to reduce the data to a set of themes or categories that can be more easily analyzed.
Identifying patterns and themes
After coding the data, the researcher looks for patterns or themes in the coded data. This involves comparing and contrasting the codes and looking for relationships or patterns among them. The identified patterns and themes should help answer the research question.
Interpreting the data
Once patterns and themes have been identified, the next step is to interpret these findings. This involves explaining what the patterns or themes mean in the context of the research question and the case. This interpretation should be grounded in the data, but it can also involve drawing on theoretical concepts or prior research.
Verification of the data
The last step in the analysis is verification. This involves checking the accuracy and consistency of the analysis process and confirming that the findings are supported by the data. This can involve re-checking the original data, checking the consistency of codes, or seeking feedback from research participants or peers.
Like any research method , case study research has its strengths and limitations. Researchers must be aware of these, as they can influence the design, conduct, and interpretation of the study.
Understanding the strengths and limitations of case study research can also guide researchers in deciding whether this approach is suitable for their research question . This section outlines some of the key strengths and limitations of case study research.
Benefits include the following:
- Rich, detailed data: One of the main strengths of case study research is that it can generate rich, detailed data about the case. This can provide a deep understanding of the case and its context, which can be valuable in exploring complex phenomena.
- Flexibility: Case study research is flexible in terms of design , data collection , and analysis . A sufficient degree of flexibility allows the researcher to adapt the study according to the case and the emerging findings.
- Real-world context: Case study research involves studying the case in its real-world context, which can provide valuable insights into the interplay between the case and its context.
- Multiple sources of evidence: Case study research often involves collecting data from multiple sources , which can enhance the robustness and validity of the findings.
On the other hand, researchers should consider the following limitations:
- Generalizability: A common criticism of case study research is that its findings might not be generalizable to other cases due to the specificity and uniqueness of each case.
- Time and resource intensive: Case study research can be time and resource intensive due to the depth of the investigation and the amount of collected data.
- Complexity of analysis: The rich, detailed data generated in case study research can make analyzing the data challenging.
- Subjectivity: Given the nature of case study research, there may be a higher degree of subjectivity in interpreting the data , so researchers need to reflect on this and transparently convey to audiences how the research was conducted.
Being aware of these strengths and limitations can help researchers design and conduct case study research effectively and interpret and report the findings appropriately.

Ready to analyze your data with ATLAS.ti?
See how our intuitive software can draw key insights from your data with a free trial today.
- User Experience (UX) Testing User Interface (UI) Testing Ecommerce Testing Remote Usability Testing About the company ' data-html="true"> Why Trymata
- Usability testing
Run remote usability tests on any digital product to deep dive into your key user flows
- Product analytics
Learn how users are behaving on your website in real time and uncover points of frustration
- Research repository
A tool for collaborative analysis of qualitative data and for building your research repository and database.
See an example
- Trymata Blog
How-to articles, expert tips, and the latest news in user testing & user experience
- Knowledge Hub
Detailed explainers of Trymata’s features & plans, and UX research terms & topics
Visit Knowledge Hub
- Plans & Pricing
Get paid to test
- User Experience (UX) testing
- User Interface (UI) testing
- Ecommerce testing
- Remote usability testing
- Plans & Pricing
- Customer Stories
How do you want to use Trymata?
Conduct user testing, desktop usability video.
You’re on a business trip in Oakland, CA. You've been working late in downtown and now you're looking for a place nearby to grab a late dinner. You decided to check Zomato to try and find somewhere to eat. (Don't begin searching yet).
- Look around on the home page. Does anything seem interesting to you?
- How would you go about finding a place to eat near you in Downtown Oakland? You want something kind of quick, open late, not too expensive, and with a good rating.
- What do the reviews say about the restaurant you've chosen?
- What was the most important factor for you in choosing this spot?
- You're currently close to the 19th St Bart station, and it's 9PM. How would you get to this restaurant? Do you think you'll be able to make it before closing time?
- Your friend recommended you to check out a place called Belly while you're in Oakland. Try to find where it is, when it's open, and what kind of food options they have.
- Now go to any restaurant's page and try to leave a review (don't actually submit it).
What was the worst thing about your experience?
It was hard to find the bart station. The collections not being able to be sorted was a bit of a bummer
What other aspects of the experience could be improved?
Feedback from the owners would be nice
What did you like about the website?
The flow was good, lots of bright photos
What other comments do you have for the owner of the website?
I like that you can sort by what you are looking for and i like the idea of collections
You're going on a vacation to Italy next month, and you want to learn some basic Italian for getting around while there. You decided to try Duolingo.
- Please begin by downloading the app to your device.
- Choose Italian and get started with the first lesson (stop once you reach the first question).
- Now go all the way through the rest of the first lesson, describing your thoughts as you go.
- Get your profile set up, then view your account page. What information and options are there? Do you feel that these are useful? Why or why not?
- After a week in Italy, you're going to spend a few days in Austria. How would you take German lessons on Duolingo?
- What other languages does the app offer? Do any of them interest you?
I felt like there could have been a little more of an instructional component to the lesson.
It would be cool if there were some feature that could allow two learners studying the same language to take lessons together. I imagine that their screens would be synced and they could go through lessons together and chat along the way.
Overall, the app was very intuitive to use and visually appealing. I also liked the option to connect with others.
Overall, the app seemed very helpful and easy to use. I feel like it makes learning a new language fun and almost like a game. It would be nice, however, if it contained more of an instructional portion.
All accounts, tests, and data have been migrated to our new & improved system!
Use the same email and password to log in:
Legacy login: Our legacy system is still available in view-only mode, login here >
What’s the new system about? Read more about our transition & what it-->
What is a Case Study? Definition, Research Methods, Sampling and Examples
Conduct End-to-End User Testing & Research
What is a Case Study?
A case study is defined as an in-depth analysis of a particular subject, often a real-world situation, individual, group, or organization.
It is a research method that involves the comprehensive examination of a specific instance to gain a better understanding of its complexities, dynamics, and context.
Case studies are commonly used in various fields such as business, psychology, medicine, and education to explore and illustrate phenomena, theories, or practical applications.
In a typical case study, researchers collect and analyze a rich array of qualitative and/or quantitative data, including interviews, observations, documents, and other relevant sources. The goal is to provide a nuanced and holistic perspective on the subject under investigation.
The information gathered here is used to generate insights, draw conclusions, and often to inform broader theories or practices within the respective field.
Case studies offer a valuable method for researchers to explore real-world phenomena in their natural settings, providing an opportunity to delve deeply into the intricacies of a particular case. They are particularly useful when studying complex, multifaceted situations where various factors interact.
Additionally, case studies can be instrumental in generating hypotheses, testing theories, and offering practical insights that can be applied to similar situations. Overall, the comprehensive nature of case studies makes them a powerful tool for gaining a thorough understanding of specific instances within the broader context of academic and professional inquiry.
Key Characteristics of Case Study
Case studies are characterized by several key features that distinguish them from other research methods. Here are some essential characteristics of case studies:
- In-depth Exploration: Case studies involve a thorough and detailed examination of a specific case or instance. Researchers aim to explore the complexities and nuances of the subject under investigation, often using multiple data sources and methods to gather comprehensive information.
- Contextual Analysis: Case studies emphasize the importance of understanding the context in which the case unfolds. Researchers seek to examine the unique circumstances, background, and environmental factors that contribute to the dynamics of the case. Contextual analysis is crucial for drawing meaningful conclusions and generalizing findings to similar situations.
- Holistic Perspective: Rather than focusing on isolated variables, case studies take a holistic approach to studying a phenomenon. Researchers consider a wide range of factors and their interrelationships, aiming to capture the richness and complexity of the case. This holistic perspective helps in providing a more complete understanding of the subject.
- Qualitative and/or Quantitative Data: Case studies can incorporate both qualitative and quantitative data, depending on the research question and objectives. Qualitative data often include interviews, observations, and document analysis, while quantitative data may involve statistical measures or numerical information. The combination of these data types enhances the depth and validity of the study.
- Longitudinal or Retrospective Design: Case studies can be designed as longitudinal studies, where the researcher follows the case over an extended period, or retrospective studies, where the focus is on examining past events. This temporal dimension allows researchers to capture changes and developments within the case.
- Unique and Unpredictable Nature: Each case study is unique, and the findings may not be easily generalized to other situations. The unpredictable nature of real-world cases adds a layer of authenticity to the study, making it an effective method for exploring complex and dynamic phenomena.
- Theory Building or Testing: Case studies can serve different purposes, including theory building or theory testing. In some cases, researchers use case studies to develop new theories or refine existing ones. In others, they may test existing theories by applying them to real-world situations and assessing their explanatory power.
Understanding these key characteristics is essential for researchers and practitioners using case studies as a methodological approach, as it helps guide the design, implementation, and analysis of the study.
Key Components of a Case Study
A well-constructed case study typically consists of several key components that collectively provide a comprehensive understanding of the subject under investigation. Here are the key components of a case study:
- Provide an overview of the context and background information relevant to the case. This may include the history, industry, or setting in which the case is situated.
- Clearly state the purpose and objectives of the case study. Define what the study aims to achieve and the questions it seeks to answer.
- Clearly identify the subject of the case study. This could be an individual, a group, an organization, or a specific event.
- Define the boundaries and scope of the case study. Specify what aspects will be included and excluded from the investigation.
- Provide a brief review of relevant theories or concepts that will guide the analysis. This helps place the case study within the broader theoretical context.
- Summarize existing literature related to the subject, highlighting key findings and gaps in knowledge. This establishes the context for the current case study.
- Describe the research design chosen for the case study (e.g., exploratory, explanatory, descriptive). Justify why this design is appropriate for the research objectives.
- Specify the methods used to gather data, whether through interviews, observations, document analysis, surveys, or a combination of these. Detail the procedures followed to ensure data validity and reliability.
- Explain the criteria for selecting the case and any sampling considerations. Discuss why the chosen case is representative or relevant to the research questions.
- Describe how the collected data will be coded and categorized. Discuss the analytical framework or approach used to identify patterns, themes, or trends.
- If multiple data sources or methods are used, explain how they complement each other to enhance the credibility and validity of the findings.
- Present the key findings in a clear and organized manner. Use tables, charts, or quotes from participants to illustrate the results.
- Interpret the results in the context of the research objectives and theoretical framework. Discuss any unexpected findings and their implications.
- Provide a thorough interpretation of the results, connecting them to the research questions and relevant literature.
- Acknowledge the limitations of the study, such as constraints in data collection, sample size, or generalizability.
- Highlight the contributions of the case study to the existing body of knowledge and identify potential avenues for future research.
- Summarize the key findings and their significance in relation to the research objectives.
- Conclude with a concise summary of the case study, its implications, and potential practical applications.
- Provide a complete list of all the sources cited in the case study, following a consistent citation style.
- Include any additional materials or supplementary information, such as interview transcripts, survey instruments, or supporting documents.
By including these key components, a case study becomes a comprehensive and well-rounded exploration of a specific subject, offering valuable insights and contributing to the body of knowledge in the respective field.
Sampling in a Case Study Research
Sampling in case study research involves selecting a subset of cases or individuals from a larger population to study in depth. Unlike quantitative research where random sampling is often employed, case study sampling is typically purposeful and driven by the specific objectives of the study. Here are some key considerations for sampling in case study research:
- Criterion Sampling: Cases are selected based on specific criteria relevant to the research questions. For example, if studying successful business strategies, cases may be selected based on their demonstrated success.
- Maximum Variation Sampling: Cases are chosen to represent a broad range of variations related to key characteristics. This approach helps capture diversity within the sample.
- Selecting Cases with Rich Information: Researchers aim to choose cases that are information-rich and provide insights into the phenomenon under investigation. These cases should offer a depth of detail and variation relevant to the research objectives.
- Single Case vs. Multiple Cases: Decide whether the study will focus on a single case (single-case study) or multiple cases (multiple-case study). The choice depends on the research objectives, the complexity of the phenomenon, and the depth of understanding required.
- Emergent Nature of Sampling: In some case studies, the sampling strategy may evolve as the study progresses. This is known as theoretical sampling, where new cases are selected based on emerging findings and theoretical insights from earlier analysis.
- Data Saturation: Sampling may continue until data saturation is achieved, meaning that collecting additional cases or data does not yield new insights or information. Saturation indicates that the researcher has adequately explored the phenomenon.
- Defining Case Boundaries: Clearly define the boundaries of the case to ensure consistency and avoid ambiguity. Consider what is included and excluded from the case study, and justify these decisions.
- Practical Considerations: Assess the feasibility of accessing the selected cases. Consider factors such as availability, willingness to participate, and the practicality of data collection methods.
- Informed Consent: Obtain informed consent from participants, ensuring that they understand the purpose of the study and the ways in which their information will be used. Protect the confidentiality and anonymity of participants as needed.
- Pilot Testing the Sampling Strategy: Before conducting the full study, consider pilot testing the sampling strategy to identify potential challenges and refine the approach. This can help ensure the effectiveness of the sampling method.
- Transparent Reporting: Clearly document the sampling process in the research methodology section. Provide a rationale for the chosen sampling strategy and discuss any adjustments made during the study.
Sampling in case study research is a critical step that influences the depth and richness of the study’s findings. By carefully selecting cases based on specific criteria and considering the unique characteristics of the phenomenon under investigation, researchers can enhance the relevance and validity of their case study.
Case Study Research Methods With Examples
- Interviews:
- Interviews involve engaging with participants to gather detailed information, opinions, and insights. In a case study, interviews are often semi-structured, allowing flexibility in questioning.
- Example: A case study on workplace culture might involve conducting interviews with employees at different levels to understand their perceptions, experiences, and attitudes.
- Observations:
- Observations entail direct examination and recording of behavior, activities, or events in their natural setting. This method is valuable for understanding behaviors in context.
- Example: A case study investigating customer interactions at a retail store may involve observing and documenting customer behavior, staff interactions, and overall dynamics.
- Document Analysis:
- Document analysis involves reviewing and interpreting written or recorded materials, such as reports, memos, emails, and other relevant documents.
- Example: In a case study on organizational change, researchers may analyze internal documents, such as communication memos or strategic plans, to trace the evolution of the change process.
- Surveys and Questionnaires:
- Surveys and questionnaires collect structured data from a sample of participants. While less common in case studies, they can be used to supplement other methods.
- Example: A case study on the impact of a health intervention might include a survey to gather quantitative data on participants’ health outcomes.
- Focus Groups:
- Focus groups involve a facilitated discussion among a group of participants to explore their perceptions, attitudes, and experiences.
- Example: In a case study on community development, a focus group might be conducted with residents to discuss their views on recent initiatives and their impact.
- Archival Research:
- Archival research involves examining existing records, historical documents, or artifacts to gain insights into a particular phenomenon.
- Example: A case study on the history of a landmark building may involve archival research, exploring construction records, historical photos, and maintenance logs.
- Longitudinal Studies:
- Longitudinal studies involve the collection of data over an extended period to observe changes and developments.
- Example: A case study tracking the career progression of employees in a company may involve longitudinal interviews and document analysis over several years.
- Cross-Case Analysis:
- Cross-case analysis compares and contrasts multiple cases to identify patterns, similarities, and differences.
- Example: A comparative case study of different educational institutions may involve analyzing common challenges and successful strategies across various cases.
- Ethnography:
- Ethnography involves immersive, in-depth exploration within a cultural or social setting to understand the behaviors and perspectives of participants.
- Example: A case study using ethnographic methods might involve spending an extended period within a community to understand its social dynamics and cultural practices.
- Experimental Designs (Rare):
- While less common, experimental designs involve manipulating variables to observe their effects. In case studies, this might be applied in specific contexts.
- Example: A case study exploring the impact of a new teaching method might involve implementing the method in one classroom while comparing it to a traditional method in another.
These case study research methods offer a versatile toolkit for researchers to investigate and gain insights into complex phenomena across various disciplines. The choice of methods depends on the research questions, the nature of the case, and the desired depth of understanding.
Best Practices for a Case Study in 2024
Creating a high-quality case study involves adhering to best practices that ensure rigor, relevance, and credibility. Here are some key best practices for conducting and presenting a case study:
- Clearly articulate the purpose and objectives of the case study. Define the research questions or problems you aim to address, ensuring a focused and purposeful approach.
- Choose a case that aligns with the research objectives and provides the depth and richness needed for the study. Consider the uniqueness of the case and its relevance to the research questions.
- Develop a robust research design that aligns with the nature of the case study (single-case or multiple-case) and integrates appropriate research methods. Ensure the chosen design is suitable for exploring the complexities of the phenomenon.
- Use a variety of data sources to enhance the validity and reliability of the study. Combine methods such as interviews, observations, document analysis, and surveys to provide a comprehensive understanding of the case.
- Clearly document and describe the procedures for data collection to enhance transparency. Include details on participant selection, sampling strategy, and data collection methods to facilitate replication and evaluation.
- Implement measures to ensure the validity and reliability of the data. Triangulate information from different sources to cross-verify findings and strengthen the credibility of the study.
- Clearly define the boundaries of the case to avoid scope creep and maintain focus. Specify what is included and excluded from the study, providing a clear framework for analysis.
- Include perspectives from various stakeholders within the case to capture a holistic view. This might involve interviewing individuals at different organizational levels, customers, or community members, depending on the context.
- Adhere to ethical principles in research, including obtaining informed consent from participants, ensuring confidentiality, and addressing any potential conflicts of interest.
- Conduct a rigorous analysis of the data, using appropriate analytical techniques. Interpret the findings in the context of the research questions, theoretical framework, and relevant literature.
- Offer detailed and rich descriptions of the case, including the context, key events, and participant perspectives. This helps readers understand the intricacies of the case and supports the generalization of findings.
- Communicate findings in a clear and accessible manner. Avoid jargon and technical language that may hinder understanding. Use visuals, such as charts or graphs, to enhance clarity.
- Seek feedback from colleagues or experts in the field through peer review. This helps ensure the rigor and credibility of the case study and provides valuable insights for improvement.
- Connect the case study findings to existing theories or concepts, contributing to the theoretical understanding of the phenomenon. Discuss practical implications and potential applications in relevant contexts.
- Recognize that case study research is often an iterative process. Be open to revisiting and refining research questions, methods, or analysis as the study progresses. Practice reflexivity by acknowledging and addressing potential biases or preconceptions.
By incorporating these best practices, researchers can enhance the quality and impact of their case studies, making valuable contributions to the academic and practical understanding of complex phenomena.
Interested in learning more about the fields of product, research, and design? Search our articles here for helpful information spanning a wide range of topics!
How Many User Testers Do You Need in Usability Testing?
Optimize user experience with effective lab usability testing, improve omnichannel customer experience for better usability, 9 usability testing checklist to start your first test.
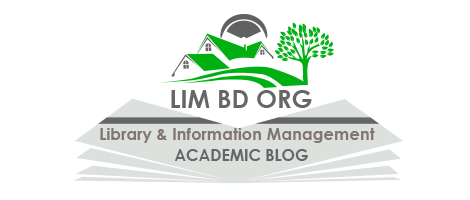
- Classification
- Physical Education
- Travel and Tourism
- BIBLIOMETRICS
- Banking System
- Real Estate
Select Page
Case Study Method | Characteristics, Advantages & Limitations of Case Study Method
Posted by Md. Harun Ar Rashid | Aug 5, 2021 | Research Methodology
Case Study Method
The case study method is a very popular form of qualitative analysis and involves a careful and complete observation of a social unit, be that unit a person, a family, an institution, a cultural group, or even the entire community. It is a method of study in depth rather than breadth. The case study places more emphasis on the full analysis of a limited number of events or conditions and their interrelations. The case study deals with the processes that take place and their interrelationship. Thus, the case study is essentially an intensive investigation of the particular unit under consideration. The object of the case study method is to locate the factors that account for the behavior patterns of the given unit as an integrated totality.
“The case study method is a technique by which individual factor whether it be an institution or just an episode in the life of an individual or a group is analyzed in its relationship to any other in the group.” ( H. Odum )
“A comprehensive study of a social unit be that unit a person, a group, a social institution, a district or a community.” ( Pauline V. Young )
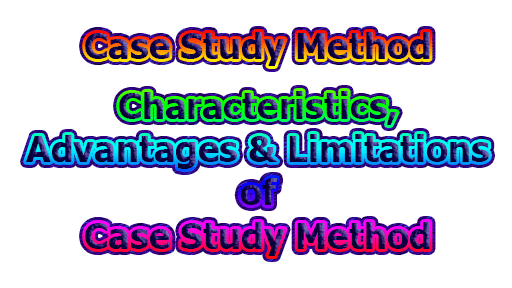
The case study method is a form of qualitative analysis wherein careful and complete observation of an individual or a situation or an institution is done; efforts are made to study each and every aspect of the concerning unit in minute details and then from case data generalizations and inferences are drawn.
Characteristics: The essential characteristics of the case study method are as under:
- The researcher can take one single social unit or more of such units for his study purpose, may even take a situation to study the same comprehensively.
- To obtain enough information for drawing correct inferences.
- To make a complete study of the social unit covering all facets.
- Try to understand the complex factors that are operative within a social unit as an integrated totality.
- The approach happens to be qualitative and not quantitative. Mere quantitative information is not collected. Every possible effort is made to collect information concerning all aspects of life.
- To know the mutual inter-relationship of causal factors.
- The behavior pattern of the concerning unit is studied directly and not by an indirect and abstract approach.
- It results in fruitful hypotheses along with the data which may be helpful in testing them, and thus it enables the generalized knowledge to get richer and richer.
Advantages: There are several advantages of the case study method, some of them are being:
- To understand fully the behavior pattern of the concerned unit.
- Helps to obtain a real and enlightened record of personal experiences.
- This method enables the researcher to trace out the natural history of the social unit and its relationship with the social factors and the forces involved in its surrounding environment.
- It helps in formulating relevant hypotheses along with the data which may be helpful in testing them.
- It facilitates the intensive study of social units that’s why the case study method is being frequently used, particularly in social researches.
- It helps a lot to the researcher in the task of constructing the appropriate questionnaire.
- The researcher can use different methods such as depth interviews, questionnaires, documents, study reports of individuals, and so on.
- It has proved beneficial in determining the nature of units to be studied along with the nature of the universe. So it is known as the “mode of organizing data”.
- It means to well understand the past of a social unit because of its emphasis on historical analysis, also it’s a technique to suggest measures for improvement in the context of the present environment of the concerned social units.
- It represents a real record of personal experiences which very often escape the attention of most of the skilled researchers using other techniques.
- It enhances the experience, analyzing ability, and skills of the researcher.
- It facilitates the drawing of inferences and helps in maintaining the continuity of the research process.
Limitations: Important limitations of the case study method may as well be highlighted.
- Case situations are seldom comparable and as such the information gathered in case studies is often not comparable. Since the subject under the case study tells history in his own words, logical concepts and units of scientific classification have to be read into it or out of it by the investigator.
- Read Bain does not consider the case data as significant scientific data since they do not provide knowledge of the “impersonal, universal, non-ethical, non-practical, repetitive aspects of phenomena.”8 Real information is often not collected because the subjectivity of the researcher does enter in the collection of information in a case study.
- The danger of false generalization is always there in view of the fact that no set rules are followed in the collection of the information and only a few units are studied.
- It consumes more time and requires a lot of expenditure. More time is needed under the case study method since one studies the natural history cycles of social units and that too minutely.
- The case data are often vitiated because the subject, according to reading Bain, may write what he thinks the investigator wants; and the greater the rapport, the more subjective the whole process is.
- The case study method is based on several assumptions which may not be very realistic at times, and as such, the use of case data is always subject to doubt.
- The case study method can be used only in a limited sphere, it is not possible to use it in the case of a big society. Sampling is also not possible under a case study method.
- Response of the investigator is an important limitation of the case study method. He often thinks that he has full knowledge of the unit and can himself answer about it. In case the same is not true, then consequences follow. In fact, this is more the fault of the researcher rather than that of the case method.
Despite the above-stated limitations, we find that case studies are being undertaken in several disciplines, particularly in sociology, as a tool of scientific research in view of the several advantages indicated earlier. Most of the limitations can be removed if researchers are always conscious of these and are well trained in the modern methods of collecting case data and in the scientific techniques of assembling, classifying, and processing the same. Besides, case studies, in modern times, can be conducted in such a manner that the data are amenable to quantification and statistical treatment. Possibly, this is also the reason why case studies are becoming popular day by day.
Reference: Research Methodology written by C.R. Kothari
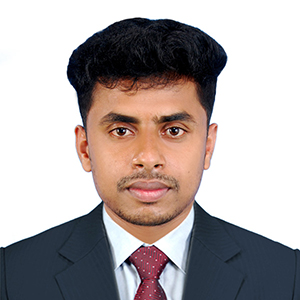
Former Student at Rajshahi University
About The Author
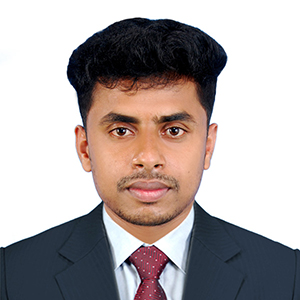
Md. Harun Ar Rashid
Related posts.
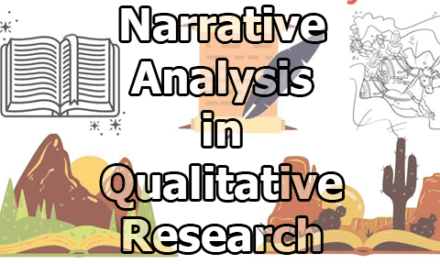
Narrative Analysis in Qualitative Research
March 15, 2023
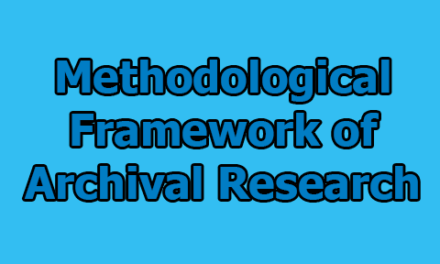
Methodological Framework of Archival Research
November 23, 2023
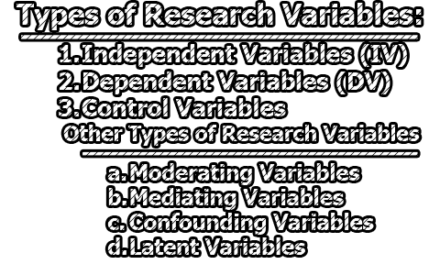
Research Variables | Types of Research Variables
April 5, 2023
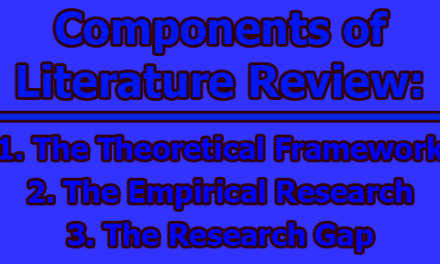
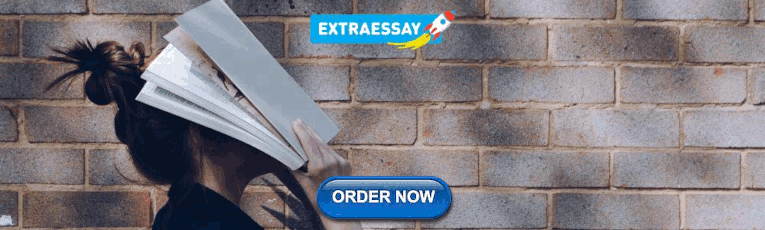
Components of Literature Review
August 9, 2023
Follow us on Facebook
Library & Information Management Community
Recent Posts
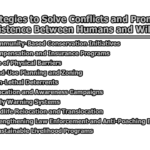
M | T | W | T | F | S | S |
---|---|---|---|---|---|---|
1 | 2 | 3 | 4 | 5 | 6 | |
7 | 8 | 9 | 10 | 11 | 12 | 13 |
14 | 15 | 16 | 17 | 18 | 19 | 20 |
21 | 22 | 23 | 24 | 25 | 26 | 27 |
28 | 29 | 30 | 31 |
Pin It on Pinterest
- LiveJournal
Have a language expert improve your writing
Run a free plagiarism check in 10 minutes, automatically generate references for free.
- Knowledge Base
- Methodology
- Case Study | Definition, Examples & Methods
Case Study | Definition, Examples & Methods
Published on 5 May 2022 by Shona McCombes . Revised on 30 January 2023.
A case study is a detailed study of a specific subject, such as a person, group, place, event, organisation, or phenomenon. Case studies are commonly used in social, educational, clinical, and business research.
A case study research design usually involves qualitative methods , but quantitative methods are sometimes also used. Case studies are good for describing , comparing, evaluating, and understanding different aspects of a research problem .
Table of contents
When to do a case study, step 1: select a case, step 2: build a theoretical framework, step 3: collect your data, step 4: describe and analyse the case.
A case study is an appropriate research design when you want to gain concrete, contextual, in-depth knowledge about a specific real-world subject. It allows you to explore the key characteristics, meanings, and implications of the case.
Case studies are often a good choice in a thesis or dissertation . They keep your project focused and manageable when you don’t have the time or resources to do large-scale research.
You might use just one complex case study where you explore a single subject in depth, or conduct multiple case studies to compare and illuminate different aspects of your research problem.
Research question | Case study |
---|---|
What are the ecological effects of wolf reintroduction? | Case study of wolf reintroduction in Yellowstone National Park in the US |
How do populist politicians use narratives about history to gain support? | Case studies of Hungarian prime minister Viktor Orbán and US president Donald Trump |
How can teachers implement active learning strategies in mixed-level classrooms? | Case study of a local school that promotes active learning |
What are the main advantages and disadvantages of wind farms for rural communities? | Case studies of three rural wind farm development projects in different parts of the country |
How are viral marketing strategies changing the relationship between companies and consumers? | Case study of the iPhone X marketing campaign |
How do experiences of work in the gig economy differ by gender, race, and age? | Case studies of Deliveroo and Uber drivers in London |
Prevent plagiarism, run a free check.
Once you have developed your problem statement and research questions , you should be ready to choose the specific case that you want to focus on. A good case study should have the potential to:
- Provide new or unexpected insights into the subject
- Challenge or complicate existing assumptions and theories
- Propose practical courses of action to resolve a problem
- Open up new directions for future research
Unlike quantitative or experimental research, a strong case study does not require a random or representative sample. In fact, case studies often deliberately focus on unusual, neglected, or outlying cases which may shed new light on the research problem.
If you find yourself aiming to simultaneously investigate and solve an issue, consider conducting action research . As its name suggests, action research conducts research and takes action at the same time, and is highly iterative and flexible.
However, you can also choose a more common or representative case to exemplify a particular category, experience, or phenomenon.
While case studies focus more on concrete details than general theories, they should usually have some connection with theory in the field. This way the case study is not just an isolated description, but is integrated into existing knowledge about the topic. It might aim to:
- Exemplify a theory by showing how it explains the case under investigation
- Expand on a theory by uncovering new concepts and ideas that need to be incorporated
- Challenge a theory by exploring an outlier case that doesn’t fit with established assumptions
To ensure that your analysis of the case has a solid academic grounding, you should conduct a literature review of sources related to the topic and develop a theoretical framework . This means identifying key concepts and theories to guide your analysis and interpretation.
There are many different research methods you can use to collect data on your subject. Case studies tend to focus on qualitative data using methods such as interviews, observations, and analysis of primary and secondary sources (e.g., newspaper articles, photographs, official records). Sometimes a case study will also collect quantitative data .
The aim is to gain as thorough an understanding as possible of the case and its context.
In writing up the case study, you need to bring together all the relevant aspects to give as complete a picture as possible of the subject.
How you report your findings depends on the type of research you are doing. Some case studies are structured like a standard scientific paper or thesis, with separate sections or chapters for the methods , results , and discussion .
Others are written in a more narrative style, aiming to explore the case from various angles and analyse its meanings and implications (for example, by using textual analysis or discourse analysis ).
In all cases, though, make sure to give contextual details about the case, connect it back to the literature and theory, and discuss how it fits into wider patterns or debates.
Cite this Scribbr article
If you want to cite this source, you can copy and paste the citation or click the ‘Cite this Scribbr article’ button to automatically add the citation to our free Reference Generator.
McCombes, S. (2023, January 30). Case Study | Definition, Examples & Methods. Scribbr. Retrieved 14 October 2024, from https://www.scribbr.co.uk/research-methods/case-studies/
Is this article helpful?
Shona McCombes
Other students also liked, correlational research | guide, design & examples, a quick guide to experimental design | 5 steps & examples, descriptive research design | definition, methods & examples.

- SUGGESTED TOPICS
- The Magazine
- Newsletters
- Managing Yourself
- Managing Teams
- Work-life Balance
- The Big Idea
- Data & Visuals
- Case Selections
- HBR Learning
- Topic Feeds
- Account Settings
- Email Preferences
What the Case Study Method Really Teaches
- Nitin Nohria

Seven meta-skills that stick even if the cases fade from memory.
It’s been 100 years since Harvard Business School began using the case study method. Beyond teaching specific subject matter, the case study method excels in instilling meta-skills in students. This article explains the importance of seven such skills: preparation, discernment, bias recognition, judgement, collaboration, curiosity, and self-confidence.
During my decade as dean of Harvard Business School, I spent hundreds of hours talking with our alumni. To enliven these conversations, I relied on a favorite question: “What was the most important thing you learned from your time in our MBA program?”
- Nitin Nohria is the George F. Baker Jr. and Distinguished Service University Professor. He served as the 10th dean of Harvard Business School, from 2010 to 2020.
Partner Center

An official website of the United States government
The .gov means it’s official. Federal government websites often end in .gov or .mil. Before sharing sensitive information, make sure you’re on a federal government site.
The site is secure. The https:// ensures that you are connecting to the official website and that any information you provide is encrypted and transmitted securely.
- Publications
- Account settings
The PMC website is updating on October 15, 2024. Learn More or Try it out now .
- Advanced Search
- Journal List
- Int J Qual Stud Health Well-being
Methodology or method? A critical review of qualitative case study reports
Despite on-going debate about credibility, and reported limitations in comparison to other approaches, case study is an increasingly popular approach among qualitative researchers. We critically analysed the methodological descriptions of published case studies. Three high-impact qualitative methods journals were searched to locate case studies published in the past 5 years; 34 were selected for analysis. Articles were categorized as health and health services ( n= 12), social sciences and anthropology ( n= 7), or methods ( n= 15) case studies. The articles were reviewed using an adapted version of established criteria to determine whether adequate methodological justification was present, and if study aims, methods, and reported findings were consistent with a qualitative case study approach. Findings were grouped into five themes outlining key methodological issues: case study methodology or method, case of something particular and case selection, contextually bound case study, researcher and case interactions and triangulation, and study design inconsistent with methodology reported. Improved reporting of case studies by qualitative researchers will advance the methodology for the benefit of researchers and practitioners.
Case study research is an increasingly popular approach among qualitative researchers (Thomas, 2011 ). Several prominent authors have contributed to methodological developments, which has increased the popularity of case study approaches across disciplines (Creswell, 2013b ; Denzin & Lincoln, 2011b ; Merriam, 2009 ; Ragin & Becker, 1992 ; Stake, 1995 ; Yin, 2009 ). Current qualitative case study approaches are shaped by paradigm, study design, and selection of methods, and, as a result, case studies in the published literature vary. Differences between published case studies can make it difficult for researchers to define and understand case study as a methodology.
Experienced qualitative researchers have identified case study research as a stand-alone qualitative approach (Denzin & Lincoln, 2011b ). Case study research has a level of flexibility that is not readily offered by other qualitative approaches such as grounded theory or phenomenology. Case studies are designed to suit the case and research question and published case studies demonstrate wide diversity in study design. There are two popular case study approaches in qualitative research. The first, proposed by Stake ( 1995 ) and Merriam ( 2009 ), is situated in a social constructivist paradigm, whereas the second, by Yin ( 2012 ), Flyvbjerg ( 2011 ), and Eisenhardt ( 1989 ), approaches case study from a post-positivist viewpoint. Scholarship from both schools of inquiry has contributed to the popularity of case study and development of theoretical frameworks and principles that characterize the methodology.
The diversity of case studies reported in the published literature, and on-going debates about credibility and the use of case study in qualitative research practice, suggests that differences in perspectives on case study methodology may prevent researchers from developing a mutual understanding of practice and rigour. In addition, discussion about case study limitations has led some authors to query whether case study is indeed a methodology (Luck, Jackson, & Usher, 2006 ; Meyer, 2001 ; Thomas, 2010 ; Tight, 2010 ). Methodological discussion of qualitative case study research is timely, and a review is required to analyse and understand how this methodology is applied in the qualitative research literature. The aims of this study were to review methodological descriptions of published qualitative case studies, to review how the case study methodological approach was applied, and to identify issues that need to be addressed by researchers, editors, and reviewers. An outline of the current definitions of case study and an overview of the issues proposed in the qualitative methodological literature are provided to set the scene for the review.
Definitions of qualitative case study research
Case study research is an investigation and analysis of a single or collective case, intended to capture the complexity of the object of study (Stake, 1995 ). Qualitative case study research, as described by Stake ( 1995 ), draws together “naturalistic, holistic, ethnographic, phenomenological, and biographic research methods” in a bricoleur design, or in his words, “a palette of methods” (Stake, 1995 , pp. xi–xii). Case study methodology maintains deep connections to core values and intentions and is “particularistic, descriptive and heuristic” (Merriam, 2009 , p. 46).
As a study design, case study is defined by interest in individual cases rather than the methods of inquiry used. The selection of methods is informed by researcher and case intuition and makes use of naturally occurring sources of knowledge, such as people or observations of interactions that occur in the physical space (Stake, 1998 ). Thomas ( 2011 ) suggested that “analytical eclecticism” is a defining factor (p. 512). Multiple data collection and analysis methods are adopted to further develop and understand the case, shaped by context and emergent data (Stake, 1995 ). This qualitative approach “explores a real-life, contemporary bounded system (a case ) or multiple bounded systems (cases) over time, through detailed, in-depth data collection involving multiple sources of information … and reports a case description and case themes ” (Creswell, 2013b , p. 97). Case study research has been defined by the unit of analysis, the process of study, and the outcome or end product, all essentially the case (Merriam, 2009 ).
The case is an object to be studied for an identified reason that is peculiar or particular. Classification of the case and case selection procedures informs development of the study design and clarifies the research question. Stake ( 1995 ) proposed three types of cases and study design frameworks. These include the intrinsic case, the instrumental case, and the collective instrumental case. The intrinsic case is used to understand the particulars of a single case, rather than what it represents. An instrumental case study provides insight on an issue or is used to refine theory. The case is selected to advance understanding of the object of interest. A collective refers to an instrumental case which is studied as multiple, nested cases, observed in unison, parallel, or sequential order. More than one case can be simultaneously studied; however, each case study is a concentrated, single inquiry, studied holistically in its own entirety (Stake, 1995 , 1998 ).
Researchers who use case study are urged to seek out what is common and what is particular about the case. This involves careful and in-depth consideration of the nature of the case, historical background, physical setting, and other institutional and political contextual factors (Stake, 1998 ). An interpretive or social constructivist approach to qualitative case study research supports a transactional method of inquiry, where the researcher has a personal interaction with the case. The case is developed in a relationship between the researcher and informants, and presented to engage the reader, inviting them to join in this interaction and in case discovery (Stake, 1995 ). A postpositivist approach to case study involves developing a clear case study protocol with careful consideration of validity and potential bias, which might involve an exploratory or pilot phase, and ensures that all elements of the case are measured and adequately described (Yin, 2009 , 2012 ).
Current methodological issues in qualitative case study research
The future of qualitative research will be influenced and constructed by the way research is conducted, and by what is reviewed and published in academic journals (Morse, 2011 ). If case study research is to further develop as a principal qualitative methodological approach, and make a valued contribution to the field of qualitative inquiry, issues related to methodological credibility must be considered. Researchers are required to demonstrate rigour through adequate descriptions of methodological foundations. Case studies published without sufficient detail for the reader to understand the study design, and without rationale for key methodological decisions, may lead to research being interpreted as lacking in quality or credibility (Hallberg, 2013 ; Morse, 2011 ).
There is a level of artistic license that is embraced by qualitative researchers and distinguishes practice, which nurtures creativity, innovation, and reflexivity (Denzin & Lincoln, 2011b ; Morse, 2009 ). Qualitative research is “inherently multimethod” (Denzin & Lincoln, 2011a , p. 5); however, with this creative freedom, it is important for researchers to provide adequate description for methodological justification (Meyer, 2001 ). This includes paradigm and theoretical perspectives that have influenced study design. Without adequate description, study design might not be understood by the reader, and can appear to be dishonest or inaccurate. Reviewers and readers might be confused by the inconsistent or inappropriate terms used to describe case study research approach and methods, and be distracted from important study findings (Sandelowski, 2000 ). This issue extends beyond case study research, and others have noted inconsistencies in reporting of methodology and method by qualitative researchers. Sandelowski ( 2000 , 2010 ) argued for accurate identification of qualitative description as a research approach. She recommended that the selected methodology should be harmonious with the study design, and be reflected in methods and analysis techniques. Similarly, Webb and Kevern ( 2000 ) uncovered inconsistencies in qualitative nursing research with focus group methods, recommending that methodological procedures must cite seminal authors and be applied with respect to the selected theoretical framework. Incorrect labelling using case study might stem from the flexibility in case study design and non-directional character relative to other approaches (Rosenberg & Yates, 2007 ). Methodological integrity is required in design of qualitative studies, including case study, to ensure study rigour and to enhance credibility of the field (Morse, 2011 ).
Case study has been unnecessarily devalued by comparisons with statistical methods (Eisenhardt, 1989 ; Flyvbjerg, 2006 , 2011 ; Jensen & Rodgers, 2001 ; Piekkari, Welch, & Paavilainen, 2009 ; Tight, 2010 ; Yin, 1999 ). It is reputed to be the “the weak sibling” in comparison to other, more rigorous, approaches (Yin, 2009 , p. xiii). Case study is not an inherently comparative approach to research. The objective is not statistical research, and the aim is not to produce outcomes that are generalizable to all populations (Thomas, 2011 ). Comparisons between case study and statistical research do little to advance this qualitative approach, and fail to recognize its inherent value, which can be better understood from the interpretive or social constructionist viewpoint of other authors (Merriam, 2009 ; Stake, 1995 ). Building on discussions relating to “fuzzy” (Bassey, 2001 ), or naturalistic generalizations (Stake, 1978 ), or transference of concepts and theories (Ayres, Kavanaugh, & Knafl, 2003 ; Morse et al., 2011 ) would have more relevance.
Case study research has been used as a catch-all design to justify or add weight to fundamental qualitative descriptive studies that do not fit with other traditional frameworks (Merriam, 2009 ). A case study has been a “convenient label for our research—when we ‘can't think of anything ‘better”—in an attempt to give it [qualitative methodology] some added respectability” (Tight, 2010 , p. 337). Qualitative case study research is a pliable approach (Merriam, 2009 ; Meyer, 2001 ; Stake, 1995 ), and has been likened to a “curious methodological limbo” (Gerring, 2004 , p. 341) or “paradigmatic bridge” (Luck et al., 2006 , p. 104), that is on the borderline between postpositivist and constructionist interpretations. This has resulted in inconsistency in application, which indicates that flexibility comes with limitations (Meyer, 2001 ), and the open nature of case study research might be off-putting to novice researchers (Thomas, 2011 ). The development of a well-(in)formed theoretical framework to guide a case study should improve consistency, rigour, and trust in studies published in qualitative research journals (Meyer, 2001 ).
Assessment of rigour
The purpose of this study was to analyse the methodological descriptions of case studies published in qualitative methods journals. To do this we needed to develop a suitable framework, which used existing, established criteria for appraising qualitative case study research rigour (Creswell, 2013b ; Merriam, 2009 ; Stake, 1995 ). A number of qualitative authors have developed concepts and criteria that are used to determine whether a study is rigorous (Denzin & Lincoln, 2011b ; Lincoln, 1995 ; Sandelowski & Barroso, 2002 ). The criteria proposed by Stake ( 1995 ) provide a framework for readers and reviewers to make judgements regarding case study quality, and identify key characteristics essential for good methodological rigour. Although each of the factors listed in Stake's criteria could enhance the quality of a qualitative research report, in Table I we present an adapted criteria used in this study, which integrates more recent work by Merriam ( 2009 ) and Creswell ( 2013b ). Stake's ( 1995 ) original criteria were separated into two categories. The first list of general criteria is “relevant for all qualitative research.” The second list, “high relevance to qualitative case study research,” was the criteria that we decided had higher relevance to case study research. This second list was the main criteria used to assess the methodological descriptions of the case studies reviewed. The complete table has been preserved so that the reader can determine how the original criteria were adapted.
Framework for assessing quality in qualitative case study research.
Checklist for assessing the quality of a case study report |
---|
Relevant for all qualitative research |
1. Is this report easy to read? |
2. Does it fit together, each sentence contributing to the whole? |
3. Does this report have a conceptual structure (i.e., themes or issues)? |
4. Are its issues developed in a series and scholarly way? |
5. Have quotations been used effectively? |
6. Has the writer made sound assertions, neither over- or under-interpreting? |
7. Are headings, figures, artefacts, appendices, indexes effectively used? |
8. Was it edited well, then again with a last minute polish? |
9. Were sufficient raw data presented? |
10. Is the nature of the intended audience apparent? |
11. Does it appear that individuals were put at risk? |
High relevance to qualitative case study research |
12. Is the case adequately defined? |
13. Is there a sense of story to the presentation? |
14. Is the reader provided some vicarious experience? |
15. Has adequate attention been paid to various contexts? |
16. Were data sources well-chosen and in sufficient number? |
17. Do observations and interpretations appear to have been triangulated? |
18. Is the role and point of view of the researcher nicely apparent? |
19. Is empathy shown for all sides? |
20. Are personal intentions examined? |
Added from Merriam ( ) |
21. Is the case study particular? |
22. Is the case study descriptive? |
23. Is the case study heuristic? |
Added from Creswell ( ) |
24. Was study design appropriate to methodology? |
Adapted from Stake ( 1995 , p. 131).
Study design
The critical review method described by Grant and Booth ( 2009 ) was used, which is appropriate for the assessment of research quality, and is used for literature analysis to inform research and practice. This type of review goes beyond the mapping and description of scoping or rapid reviews, to include “analysis and conceptual innovation” (Grant & Booth, 2009 , p. 93). A critical review is used to develop existing, or produce new, hypotheses or models. This is different to systematic reviews that answer clinical questions. It is used to evaluate existing research and competing ideas, to provide a “launch pad” for conceptual development and “subsequent testing” (Grant & Booth, 2009 , p. 93).
Qualitative methods journals were located by a search of the 2011 ISI Journal Citation Reports in Social Science, via the database Web of Knowledge (see m.webofknowledge.com). No “qualitative research methods” category existed in the citation reports; therefore, a search of all categories was performed using the term “qualitative.” In Table II , we present the qualitative methods journals located, ranked by impact factor. The highest ranked journals were selected for searching. We acknowledge that the impact factor ranking system might not be the best measure of journal quality (Cheek, Garnham, & Quan, 2006 ); however, this was the most appropriate and accessible method available.
International Journal of Qualitative Studies on Health and Well-being.
Journal title | 2011 impact factor | 5-year impact factor |
---|---|---|
2.188 | 2.432 | |
1.426 | N/A | |
0.839 | 1.850 | |
0.780 | N/A | |
0.612 | N/A |
Search strategy
In March 2013, searches of the journals, Qualitative Health Research , Qualitative Research , and Qualitative Inquiry were completed to retrieve studies with “case study” in the abstract field. The search was limited to the past 5 years (1 January 2008 to 1 March 2013). The objective was to locate published qualitative case studies suitable for assessment using the adapted criterion. Viewpoints, commentaries, and other article types were excluded from review. Title and abstracts of the 45 retrieved articles were read by the first author, who identified 34 empirical case studies for review. All authors reviewed the 34 studies to confirm selection and categorization. In Table III , we present the 34 case studies grouped by journal, and categorized by research topic, including health sciences, social sciences and anthropology, and methods research. There was a discrepancy in categorization of one article on pedagogy and a new teaching method published in Qualitative Inquiry (Jorrín-Abellán, Rubia-Avi, Anguita-Martínez, Gómez-Sánchez, & Martínez-Mones, 2008 ). Consensus was to allocate to the methods category.
Outcomes of search of qualitative methods journals.
Journal title | Date of search | Number of studies located | Number of full text studies extracted | Health sciences | Social sciences and anthropology | Methods |
---|---|---|---|---|---|---|
4 Mar 2013 | 18 | 16 | Barone ( ); Bronken et al. ( ); Colón-Emeric et al. ( ); Fourie and Theron ( ); Gallagher et al. ( ); Gillard et al. ( ); Hooghe et al. ( ); Jackson et al. ( ); Ledderer ( ); Mawn et al. ( ); Roscigno et al. ( ); Rytterström et al. ( ) | Nil | Austin, Park, and Goble ( ); Broyles, Rodriguez, Price, Bayliss, and Sevick ( ); De Haene et al. ( ); Fincham et al. ( ) | |
7 Mar 2013 | 11 | 7 | Nil | Adamson and Holloway ( ); Coltart and Henwood ( ) | Buckley and Waring ( ); Cunsolo Willox et al. ( ); Edwards and Weller ( ); Gratton and O'Donnell ( ); Sumsion ( ) | |
4 Mar 2013 | 16 | 11 | Nil | Buzzanell and D’Enbeau ( ); D'Enbeau et al. ( ); Nagar-Ron and Motzafi-Haller ( ); Snyder-Young ( ); Yeh ( ) | Ajodhia-Andrews and Berman ( ); Alexander et al. ( ); Jorrín-Abellán et al. ( ); Nairn and Panelli ( ); Nespor ( ); Wimpenny and Savin-Baden ( ) | |
Total | 45 | 34 | 12 | 7 | 15 |
In Table III , the number of studies located, and final numbers selected for review have been reported. Qualitative Health Research published the most empirical case studies ( n= 16). In the health category, there were 12 case studies of health conditions, health services, and health policy issues, all published in Qualitative Health Research . Seven case studies were categorized as social sciences and anthropology research, which combined case study with biography and ethnography methodologies. All three journals published case studies on methods research to illustrate a data collection or analysis technique, methodological procedure, or related issue.
The methodological descriptions of 34 case studies were critically reviewed using the adapted criteria. All articles reviewed contained a description of study methods; however, the length, amount of detail, and position of the description in the article varied. Few studies provided an accurate description and rationale for using a qualitative case study approach. In the 34 case studies reviewed, three described a theoretical framework informed by Stake ( 1995 ), two by Yin ( 2009 ), and three provided a mixed framework informed by various authors, which might have included both Yin and Stake. Few studies described their case study design, or included a rationale that explained why they excluded or added further procedures, and whether this was to enhance the study design, or to better suit the research question. In 26 of the studies no reference was provided to principal case study authors. From reviewing the description of methods, few authors provided a description or justification of case study methodology that demonstrated how their study was informed by the methodological literature that exists on this approach.
The methodological descriptions of each study were reviewed using the adapted criteria, and the following issues were identified: case study methodology or method; case of something particular and case selection; contextually bound case study; researcher and case interactions and triangulation; and, study design inconsistent with methodology. An outline of how the issues were developed from the critical review is provided, followed by a discussion of how these relate to the current methodological literature.
Case study methodology or method
A third of the case studies reviewed appeared to use a case report method, not case study methodology as described by principal authors (Creswell, 2013b ; Merriam, 2009 ; Stake, 1995 ; Yin, 2009 ). Case studies were identified as a case report because of missing methodological detail and by review of the study aims and purpose. These reports presented data for small samples of no more than three people, places or phenomenon. Four studies, or “case reports” were single cases selected retrospectively from larger studies (Bronken, Kirkevold, Martinsen, & Kvigne, 2012 ; Coltart & Henwood, 2012 ; Hooghe, Neimeyer, & Rober, 2012 ; Roscigno et al., 2012 ). Case reports were not a case of something, instead were a case demonstration or an example presented in a report. These reports presented outcomes, and reported on how the case could be generalized. Descriptions focussed on the phenomena, rather than the case itself, and did not appear to study the case in its entirety.
Case reports had minimal in-text references to case study methodology, and were informed by other qualitative traditions or secondary sources (Adamson & Holloway, 2012 ; Buzzanell & D'Enbeau, 2009 ; Nagar-Ron & Motzafi-Haller, 2011 ). This does not suggest that case study methodology cannot be multimethod, however, methodology should be consistent in design, be clearly described (Meyer, 2001 ; Stake, 1995 ), and maintain focus on the case (Creswell, 2013b ).
To demonstrate how case reports were identified, three examples are provided. The first, Yeh ( 2013 ) described their study as, “the examination of the emergence of vegetarianism in Victorian England serves as a case study to reveal the relationships between boundaries and entities” (p. 306). The findings were a historical case report, which resulted from an ethnographic study of vegetarianism. Cunsolo Willox, Harper, Edge, ‘My Word’: Storytelling and Digital Media Lab, and Rigolet Inuit Community Government (2013) used “a case study that illustrates the usage of digital storytelling within an Inuit community” (p. 130). This case study reported how digital storytelling can be used with indigenous communities as a participatory method to illuminate the benefits of this method for other studies. This “case study was conducted in the Inuit community” but did not include the Inuit community in case analysis (Cunsolo Willox et al., 2013 , p. 130). Bronken et al. ( 2012 ) provided a single case report to demonstrate issues observed in a larger clinical study of aphasia and stroke, without adequate case description or analysis.
Case study of something particular and case selection
Case selection is a precursor to case analysis, which needs to be presented as a convincing argument (Merriam, 2009 ). Descriptions of the case were often not adequate to ascertain why the case was selected, or whether it was a particular exemplar or outlier (Thomas, 2011 ). In a number of case studies in the health and social science categories, it was not explicit whether the case was of something particular, or peculiar to their discipline or field (Adamson & Holloway, 2012 ; Bronken et al., 2012 ; Colón-Emeric et al., 2010 ; Jackson, Botelho, Welch, Joseph, & Tennstedt, 2012 ; Mawn et al., 2010 ; Snyder-Young, 2011 ). There were exceptions in the methods category ( Table III ), where cases were selected by researchers to report on a new or innovative method. The cases emerged through heuristic study, and were reported to be particular, relative to the existing methods literature (Ajodhia-Andrews & Berman, 2009 ; Buckley & Waring, 2013 ; Cunsolo Willox et al., 2013 ; De Haene, Grietens, & Verschueren, 2010 ; Gratton & O'Donnell, 2011 ; Sumsion, 2013 ; Wimpenny & Savin-Baden, 2012 ).
Case selection processes were sometimes insufficient to understand why the case was selected from the global population of cases, or what study of this case would contribute to knowledge as compared with other possible cases (Adamson & Holloway, 2012 ; Bronken et al., 2012 ; Colón-Emeric et al., 2010 ; Jackson et al., 2012 ; Mawn et al., 2010 ). In two studies, local cases were selected (Barone, 2010 ; Fourie & Theron, 2012 ) because the researcher was familiar with and had access to the case. Possible limitations of a convenience sample were not acknowledged. Purposeful sampling was used to recruit participants within the case of one study, but not of the case itself (Gallagher et al., 2013 ). Random sampling was completed for case selection in two studies (Colón-Emeric et al., 2010 ; Jackson et al., 2012 ), which has limited meaning in interpretive qualitative research.
To demonstrate how researchers provided a good justification for the selection of case study approaches, four examples are provided. The first, cases of residential care homes, were selected because of reported occurrences of mistreatment, which included residents being locked in rooms at night (Rytterström, Unosson, & Arman, 2013 ). Roscigno et al. ( 2012 ) selected cases of parents who were admitted for early hospitalization in neonatal intensive care with a threatened preterm delivery before 26 weeks. Hooghe et al. ( 2012 ) used random sampling to select 20 couples that had experienced the death of a child; however, the case study was of one couple and a particular metaphor described only by them. The final example, Coltart and Henwood ( 2012 ), provided a detailed account of how they selected two cases from a sample of 46 fathers based on personal characteristics and beliefs. They described how the analysis of the two cases would contribute to their larger study on first time fathers and parenting.
Contextually bound case study
The limits or boundaries of the case are a defining factor of case study methodology (Merriam, 2009 ; Ragin & Becker, 1992 ; Stake, 1995 ; Yin, 2009 ). Adequate contextual description is required to understand the setting or context in which the case is revealed. In the health category, case studies were used to illustrate a clinical phenomenon or issue such as compliance and health behaviour (Colón-Emeric et al., 2010 ; D'Enbeau, Buzzanell, & Duckworth, 2010 ; Gallagher et al., 2013 ; Hooghe et al., 2012 ; Jackson et al., 2012 ; Roscigno et al., 2012 ). In these case studies, contextual boundaries, such as physical and institutional descriptions, were not sufficient to understand the case as a holistic system, for example, the general practitioner (GP) clinic in Gallagher et al. ( 2013 ), or the nursing home in Colón-Emeric et al. ( 2010 ). Similarly, in the social science and methods categories, attention was paid to some components of the case context, but not others, missing important information required to understand the case as a holistic system (Alexander, Moreira, & Kumar, 2012 ; Buzzanell & D'Enbeau, 2009 ; Nairn & Panelli, 2009 ; Wimpenny & Savin-Baden, 2012 ).
In two studies, vicarious experience or vignettes (Nairn & Panelli, 2009 ) and images (Jorrín-Abellán et al., 2008 ) were effective to support description of context, and might have been a useful addition for other case studies. Missing contextual boundaries suggests that the case might not be adequately defined. Additional information, such as the physical, institutional, political, and community context, would improve understanding of the case (Stake, 1998 ). In Boxes 1 and 2 , we present brief synopses of two studies that were reviewed, which demonstrated a well bounded case. In Box 1 , Ledderer ( 2011 ) used a qualitative case study design informed by Stake's tradition. In Box 2 , Gillard, Witt, and Watts ( 2011 ) were informed by Yin's tradition. By providing a brief outline of the case studies in Boxes 1 and 2 , we demonstrate how effective case boundaries can be constructed and reported, which may be of particular interest to prospective case study researchers.
Article synopsis of case study research using Stake's tradition
Ledderer ( 2011 ) used a qualitative case study research design, informed by modern ethnography. The study is bounded to 10 general practice clinics in Denmark, who had received federal funding to implement preventative care services based on a Motivational Interviewing intervention. The researcher question focussed on “why is it so difficult to create change in medical practice?” (Ledderer, 2011 , p. 27). The study context was adequately described, providing detail on the general practitioner (GP) clinics and relevant political and economic influences. Methodological decisions are described in first person narrative, providing insight on researcher perspectives and interaction with the case. Forty-four interviews were conducted, which focussed on how GPs conducted consultations, and the form, nature and content, rather than asking their opinion or experience (Ledderer, 2011 , p. 30). The duration and intensity of researcher immersion in the case enhanced depth of description and trustworthiness of study findings. Analysis was consistent with Stake's tradition, and the researcher provided examples of inquiry techniques used to challenge assumptions about emerging themes. Several other seminal qualitative works were cited. The themes and typology constructed are rich in narrative data and storytelling by clinic staff, demonstrating individual clinic experiences as well as shared meanings and understandings about changing from a biomedical to psychological approach to preventative health intervention. Conclusions make note of social and cultural meanings and lessons learned, which might not have been uncovered using a different methodology.
Article synopsis of case study research using Yin's tradition
Gillard et al. ( 2011 ) study of camps for adolescents living with HIV/AIDs provided a good example of Yin's interpretive case study approach. The context of the case is bounded by the three summer camps of which the researchers had prior professional involvement. A case study protocol was developed that used multiple methods to gather information at three data collection points coinciding with three youth camps (Teen Forum, Discover Camp, and Camp Strong). Gillard and colleagues followed Yin's ( 2009 ) principles, using a consistent data protocol that enhanced cross-case analysis. Data described the young people, the camp physical environment, camp schedule, objectives and outcomes, and the staff of three youth camps. The findings provided a detailed description of the context, with less detail of individual participants, including insight into researcher's interpretations and methodological decisions throughout the data collection and analysis process. Findings provided the reader with a sense of “being there,” and are discovered through constant comparison of the case with the research issues; the case is the unit of analysis. There is evidence of researcher immersion in the case, and Gillard reports spending significant time in the field in a naturalistic and integrated youth mentor role.
This case study is not intended to have a significant impact on broader health policy, although does have implications for health professionals working with adolescents. Study conclusions will inform future camps for young people with chronic disease, and practitioners are able to compare similarities between this case and their own practice (for knowledge translation). No limitations of this article were reported. Limitations related to publication of this case study were that it was 20 pages long and used three tables to provide sufficient description of the camp and program components, and relationships with the research issue.
Researcher and case interactions and triangulation
Researcher and case interactions and transactions are a defining feature of case study methodology (Stake, 1995 ). Narrative stories, vignettes, and thick description are used to provoke vicarious experience and a sense of being there with the researcher in their interaction with the case. Few of the case studies reviewed provided details of the researcher's relationship with the case, researcher–case interactions, and how these influenced the development of the case study (Buzzanell & D'Enbeau, 2009 ; D'Enbeau et al., 2010 ; Gallagher et al., 2013 ; Gillard et al., 2011 ; Ledderer, 2011 ; Nagar-Ron & Motzafi-Haller, 2011 ). The role and position of the researcher needed to be self-examined and understood by readers, to understand how this influenced interactions with participants, and to determine what triangulation is needed (Merriam, 2009 ; Stake, 1995 ).
Gillard et al. ( 2011 ) provided a good example of triangulation, comparing data sources in a table (p. 1513). Triangulation of sources was used to reveal as much depth as possible in the study by Nagar-Ron and Motzafi-Haller ( 2011 ), while also enhancing confirmation validity. There were several case studies that would have benefited from improved range and use of data sources, and descriptions of researcher–case interactions (Ajodhia-Andrews & Berman, 2009 ; Bronken et al., 2012 ; Fincham, Scourfield, & Langer, 2008 ; Fourie & Theron, 2012 ; Hooghe et al., 2012 ; Snyder-Young, 2011 ; Yeh, 2013 ).
Study design inconsistent with methodology
Good, rigorous case studies require a strong methodological justification (Meyer, 2001 ) and a logical and coherent argument that defines paradigm, methodological position, and selection of study methods (Denzin & Lincoln, 2011b ). Methodological justification was insufficient in several of the studies reviewed (Barone, 2010 ; Bronken et al., 2012 ; Hooghe et al., 2012 ; Mawn et al., 2010 ; Roscigno et al., 2012 ; Yeh, 2013 ). This was judged by the absence, or inadequate or inconsistent reference to case study methodology in-text.
In six studies, the methodological justification provided did not relate to case study. There were common issues identified. Secondary sources were used as primary methodological references indicating that study design might not have been theoretically sound (Colón-Emeric et al., 2010 ; Coltart & Henwood, 2012 ; Roscigno et al., 2012 ; Snyder-Young, 2011 ). Authors and sources cited in methodological descriptions were inconsistent with the actual study design and practices used (Fourie & Theron, 2012 ; Hooghe et al., 2012 ; Jorrín-Abellán et al., 2008 ; Mawn et al., 2010 ; Rytterström et al., 2013 ; Wimpenny & Savin-Baden, 2012 ). This occurred when researchers cited Stake or Yin, or both (Mawn et al., 2010 ; Rytterström et al., 2013 ), although did not follow their paradigmatic or methodological approach. In 26 studies there were no citations for a case study methodological approach.
The findings of this study have highlighted a number of issues for researchers. A considerable number of case studies reviewed were missing key elements that define qualitative case study methodology and the tradition cited. A significant number of studies did not provide a clear methodological description or justification relevant to case study. Case studies in health and social sciences did not provide sufficient information for the reader to understand case selection, and why this case was chosen above others. The context of the cases were not described in adequate detail to understand all relevant elements of the case context, which indicated that cases may have not been contextually bounded. There were inconsistencies between reported methodology, study design, and paradigmatic approach in case studies reviewed, which made it difficult to understand the study methodology and theoretical foundations. These issues have implications for methodological integrity and honesty when reporting study design, which are values of the qualitative research tradition and are ethical requirements (Wager & Kleinert, 2010a ). Poorly described methodological descriptions may lead the reader to misinterpret or discredit study findings, which limits the impact of the study, and, as a collective, hinders advancements in the broader qualitative research field.
The issues highlighted in our review build on current debates in the case study literature, and queries about the value of this methodology. Case study research can be situated within different paradigms or designed with an array of methods. In order to maintain the creativity and flexibility that is valued in this methodology, clearer descriptions of paradigm and theoretical position and methods should be provided so that study findings are not undervalued or discredited. Case study research is an interdisciplinary practice, which means that clear methodological descriptions might be more important for this approach than other methodologies that are predominantly driven by fewer disciplines (Creswell, 2013b ).
Authors frequently omit elements of methodologies and include others to strengthen study design, and we do not propose a rigid or purist ideology in this paper. On the contrary, we encourage new ideas about using case study, together with adequate reporting, which will advance the value and practice of case study. The implications of unclear methodological descriptions in the studies reviewed were that study design appeared to be inconsistent with reported methodology, and key elements required for making judgements of rigour were missing. It was not clear whether the deviations from methodological tradition were made by researchers to strengthen the study design, or because of misinterpretations. Morse ( 2011 ) recommended that innovations and deviations from practice are best made by experienced researchers, and that a novice might be unaware of the issues involved with making these changes. To perpetuate the tradition of case study research, applications in the published literature should have consistencies with traditional methodological constructions, and deviations should be described with a rationale that is inherent in study conduct and findings. Providing methodological descriptions that demonstrate a strong theoretical foundation and coherent study design will add credibility to the study, while ensuring the intrinsic meaning of case study is maintained.
The value of this review is that it contributes to discussion of whether case study is a methodology or method. We propose possible reasons why researchers might make this misinterpretation. Researchers may interchange the terms methods and methodology, and conduct research without adequate attention to epistemology and historical tradition (Carter & Little, 2007 ; Sandelowski, 2010 ). If the rich meaning that naming a qualitative methodology brings to the study is not recognized, a case study might appear to be inconsistent with the traditional approaches described by principal authors (Creswell, 2013a ; Merriam, 2009 ; Stake, 1995 ; Yin, 2009 ). If case studies are not methodologically and theoretically situated, then they might appear to be a case report.
Case reports are promoted by university and medical journals as a method of reporting on medical or scientific cases; guidelines for case reports are publicly available on websites ( http://www.hopkinsmedicine.org/institutional_review_board/guidelines_policies/guidelines/case_report.html ). The various case report guidelines provide a general criteria for case reports, which describes that this form of report does not meet the criteria of research, is used for retrospective analysis of up to three clinical cases, and is primarily illustrative and for educational purposes. Case reports can be published in academic journals, but do not require approval from a human research ethics committee. Traditionally, case reports describe a single case, to explain how and what occurred in a selected setting, for example, to illustrate a new phenomenon that has emerged from a larger study. A case report is not necessarily particular or the study of a case in its entirety, and the larger study would usually be guided by a different research methodology.
This description of a case report is similar to what was provided in some studies reviewed. This form of report lacks methodological grounding and qualities of research rigour. The case report has publication value in demonstrating an example and for dissemination of knowledge (Flanagan, 1999 ). However, case reports have different meaning and purpose to case study, which needs to be distinguished. Findings of our review suggest that the medical understanding of a case report has been confused with qualitative case study approaches.
In this review, a number of case studies did not have methodological descriptions that included key characteristics of case study listed in the adapted criteria, and several issues have been discussed. There have been calls for improvements in publication quality of qualitative research (Morse, 2011 ), and for improvements in peer review of submitted manuscripts (Carter & Little, 2007 ; Jasper, Vaismoradi, Bondas, & Turunen, 2013 ). The challenging nature of editor and reviewers responsibilities are acknowledged in the literature (Hames, 2013 ; Wager & Kleinert, 2010b ); however, review of case study methodology should be prioritized because of disputes on methodological value.
Authors using case study approaches are recommended to describe their theoretical framework and methods clearly, and to seek and follow specialist methodological advice when needed (Wager & Kleinert, 2010a ). Adequate page space for case study description would contribute to better publications (Gillard et al., 2011 ). Capitalizing on the ability to publish complementary resources should be considered.
Limitations of the review
There is a level of subjectivity involved in this type of review and this should be considered when interpreting study findings. Qualitative methods journals were selected because the aims and scope of these journals are to publish studies that contribute to methodological discussion and development of qualitative research. Generalist health and social science journals were excluded that might have contained good quality case studies. Journals in business or education were also excluded, although a review of case studies in international business journals has been published elsewhere (Piekkari et al., 2009 ).
The criteria used to assess the quality of the case studies were a set of qualitative indicators. A numerical or ranking system might have resulted in different results. Stake's ( 1995 ) criteria have been referenced elsewhere, and was deemed the best available (Creswell, 2013b ; Crowe et al., 2011 ). Not all qualitative studies are reported in a consistent way and some authors choose to report findings in a narrative form in comparison to a typical biomedical report style (Sandelowski & Barroso, 2002 ), if misinterpretations were made this may have affected the review.
Case study research is an increasingly popular approach among qualitative researchers, which provides methodological flexibility through the incorporation of different paradigmatic positions, study designs, and methods. However, whereas flexibility can be an advantage, a myriad of different interpretations has resulted in critics questioning the use of case study as a methodology. Using an adaptation of established criteria, we aimed to identify and assess the methodological descriptions of case studies in high impact, qualitative methods journals. Few articles were identified that applied qualitative case study approaches as described by experts in case study design. There were inconsistencies in methodology and study design, which indicated that researchers were confused whether case study was a methodology or a method. Commonly, there appeared to be confusion between case studies and case reports. Without clear understanding and application of the principles and key elements of case study methodology, there is a risk that the flexibility of the approach will result in haphazard reporting, and will limit its global application as a valuable, theoretically supported methodology that can be rigorously applied across disciplines and fields.
Conflict of interest and funding
The authors have not received any funding or benefits from industry or elsewhere to conduct this study.
- Adamson S, Holloway M. Negotiating sensitivities and grappling with intangibles: Experiences from a study of spirituality and funerals. Qualitative Research. 2012; 12 (6):735–752. doi: 10.1177/1468794112439008. [ CrossRef ] [ Google Scholar ]
- Ajodhia-Andrews A, Berman R. Exploring school life from the lens of a child who does not use speech to communicate. Qualitative Inquiry. 2009; 15 (5):931–951. doi: 10.1177/1077800408322789. [ CrossRef ] [ Google Scholar ]
- Alexander B. K, Moreira C, Kumar H. S. Resisting (resistance) stories: A tri-autoethnographic exploration of father narratives across shades of difference. Qualitative Inquiry. 2012; 18 (2):121–133. doi: 10.1177/1077800411429087. [ CrossRef ] [ Google Scholar ]
- Austin W, Park C, Goble E. From interdisciplinary to transdisciplinary research: A case study. Qualitative Health Research. 2008; 18 (4):557–564. doi: 10.1177/1049732307308514. [ PubMed ] [ CrossRef ] [ Google Scholar ]
- Ayres L, Kavanaugh K, Knafl K. A. Within-case and across-case approaches to qualitative data analysis. Qualitative Health Research. 2003; 13 (6):871–883. doi: 10.1177/1049732303013006008. [ PubMed ] [ CrossRef ] [ Google Scholar ]
- Barone T. L. Culturally sensitive care 1969–2000: The Indian Chicano Health Center. Qualitative Health Research. 2010; 20 (4):453–464. doi: 10.1177/1049732310361893. [ PubMed ] [ CrossRef ] [ Google Scholar ]
- Bassey M. A solution to the problem of generalisation in educational research: Fuzzy prediction. Oxford Review of Education. 2001; 27 (1):5–22. doi: 10.1080/03054980123773. [ CrossRef ] [ Google Scholar ]
- Bronken B. A, Kirkevold M, Martinsen R, Kvigne K. The aphasic storyteller: Coconstructing stories to promote psychosocial well-being after stroke. Qualitative Health Research. 2012; 22 (10):1303–1316. doi: 10.1177/1049732312450366. [ PubMed ] [ CrossRef ] [ Google Scholar ]
- Broyles L. M, Rodriguez K. L, Price P. A, Bayliss N. K, Sevick M. A. Overcoming barriers to the recruitment of nurses as participants in health care research. Qualitative Health Research. 2011; 21 (12):1705–1718. doi: 10.1177/1049732311417727. [ PubMed ] [ CrossRef ] [ Google Scholar ]
- Buckley C. A, Waring M. J. Using diagrams to support the research process: Examples from grounded theory. Qualitative Research. 2013; 13 (2):148–172. doi: 10.1177/1468794112472280. [ CrossRef ] [ Google Scholar ]
- Buzzanell P. M, D'Enbeau S. Stories of caregiving: Intersections of academic research and women's everyday experiences. Qualitative Inquiry. 2009; 15 (7):1199–1224. doi: 10.1177/1077800409338025. [ CrossRef ] [ Google Scholar ]
- Carter S. M, Little M. Justifying knowledge, justifying method, taking action: Epistemologies, methodologies, and methods in qualitative research. Qualitative Health Research. 2007; 17 (10):1316–1328. doi: 10.1177/1049732307306927. [ PubMed ] [ CrossRef ] [ Google Scholar ]
- Cheek J, Garnham B, Quan J. What's in a number? Issues in providing evidence of impact and quality of research(ers) Qualitative Health Research. 2006; 16 (3):423–435. doi: 10.1177/1049732305285701. [ PubMed ] [ CrossRef ] [ Google Scholar ]
- Colón-Emeric C. S, Plowman D, Bailey D, Corazzini K, Utley-Smith Q, Ammarell N, et al. Regulation and mindful resident care in nursing homes. Qualitative Health Research. 2010; 20 (9):1283–1294. doi: 10.1177/1049732310369337. [ PMC free article ] [ PubMed ] [ CrossRef ] [ Google Scholar ]
- Coltart C, Henwood K. On paternal subjectivity: A qualitative longitudinal and psychosocial case analysis of men's classed positions and transitions to first-time fatherhood. Qualitative Research. 2012; 12 (1):35–52. doi: 10.1177/1468794111426224. [ CrossRef ] [ Google Scholar ]
- Creswell J. W. Five qualitative approaches to inquiry. In: Creswell J. W, editor. Qualitative inquiry and research design: Choosing among five approaches. 3rd ed. Thousand Oaks, CA: Sage; 2013a. pp. 53–84. [ Google Scholar ]
- Creswell J. W. Qualitative inquiry and research design: Choosing among five approaches. 3rd ed. Thousand Oaks, CA: Sage; 2013b. [ Google Scholar ]
- Crowe S, Cresswell K, Robertson A, Huby G, Avery A, Sheikh A. The case study approach. BMC Medical Research Methodology. 2011; 11 (1):1–9. doi: 10.1186/1471-2288-11-100. [ PMC free article ] [ PubMed ] [ CrossRef ] [ Google Scholar ]
- Cunsolo Willox A, Harper S. L, Edge V. L, ‘My Word’: Storytelling and Digital Media Lab, & Rigolet Inuit Community Government Storytelling in a digital age: Digital storytelling as an emerging narrative method for preserving and promoting indigenous oral wisdom. Qualitative Research. 2013; 13 (2):127–147. doi: 10.1177/1468794112446105. [ CrossRef ] [ Google Scholar ]
- De Haene L, Grietens H, Verschueren K. Holding harm: Narrative methods in mental health research on refugee trauma. Qualitative Health Research. 2010; 20 (12):1664–1676. doi: 10.1177/1049732310376521. [ PubMed ] [ CrossRef ] [ Google Scholar ]
- D'Enbeau S, Buzzanell P. M, Duckworth J. Problematizing classed identities in fatherhood: Development of integrative case studies for analysis and praxis. Qualitative Inquiry. 2010; 16 (9):709–720. doi: 10.1177/1077800410374183. [ CrossRef ] [ Google Scholar ]
- Denzin N. K, Lincoln Y. S. Introduction: Disciplining the practice of qualitative research. In: Denzin N. K, Lincoln Y. S, editors. The SAGE handbook of qualitative research. 4th ed. Thousand Oaks, CA: Sage; 2011a. pp. 1–6. [ Google Scholar ]
- Denzin N. K, Lincoln Y. S, editors. The SAGE handbook of qualitative research. 4th ed. Thousand Oaks, CA: Sage; 2011b. [ Google Scholar ]
- Edwards R, Weller S. Shifting analytic ontology: Using I-poems in qualitative longitudinal research. Qualitative Research. 2012; 12 (2):202–217. doi: 10.1177/1468794111422040. [ CrossRef ] [ Google Scholar ]
- Eisenhardt K. M. Building theories from case study research. The Academy of Management Review. 1989; 14 (4):532–550. doi: 10.2307/258557. [ CrossRef ] [ Google Scholar ]
- Fincham B, Scourfield J, Langer S. The impact of working with disturbing secondary data: Reading suicide files in a coroner's office. Qualitative Health Research. 2008; 18 (6):853–862. doi: 10.1177/1049732307308945. [ PubMed ] [ CrossRef ] [ Google Scholar ]
- Flanagan J. Public participation in the design of educational programmes for cancer nurses: A case report. European Journal of Cancer Care. 1999; 8 (2):107–112. doi: 10.1046/j.1365-2354.1999.00141.x. [ PubMed ] [ CrossRef ] [ Google Scholar ]
- Flyvbjerg B. Five misunderstandings about case-study research. Qualitative Inquiry. 2006; 12 (2):219–245. doi: 10.1177/1077800405284.363. [ CrossRef ] [ Google Scholar ]
- Flyvbjerg B. Case study. In: Denzin N. K, Lincoln Y. S, editors. The SAGE handbook of qualitative research. 4th ed. Thousand Oaks, CA: Sage; 2011. pp. 301–316. [ Google Scholar ]
- Fourie C. L, Theron L. C. Resilience in the face of fragile X syndrome. Qualitative Health Research. 2012; 22 (10):1355–1368. doi: 10.1177/1049732312451871. [ PubMed ] [ CrossRef ] [ Google Scholar ]
- Gallagher N, MacFarlane A, Murphy A. W, Freeman G. K, Glynn L. G, Bradley C. P. Service users’ and caregivers’ perspectives on continuity of care in out-of-hours primary care. Qualitative Health Research. 2013; 23 (3):407–421. doi: 10.1177/1049732312470521. [ PubMed ] [ CrossRef ] [ Google Scholar ]
- Gerring J. What is a case study and what is it good for? American Political Science Review. 2004; 98 (2):341–354. doi: 10.1017/S0003055404001182. [ CrossRef ] [ Google Scholar ]
- Gillard A, Witt P. A, Watts C. E. Outcomes and processes at a camp for youth with HIV/AIDS. Qualitative Health Research. 2011; 21 (11):1508–1526. doi: 10.1177/1049732311413907. [ PubMed ] [ CrossRef ] [ Google Scholar ]
- Grant M, Booth A. A typology of reviews: An analysis of 14 review types and associated methodologies. Health Information and Libraries Journal. 2009; 26 :91–108. doi: 10.1111/j.1471-1842.2009.00848.x. [ PubMed ] [ CrossRef ] [ Google Scholar ]
- Gratton M.-F, O'Donnell S. Communication technologies for focus groups with remote communities: A case study of research with First Nations in Canada. Qualitative Research. 2011; 11 (2):159–175. doi: 10.1177/1468794110394068. [ CrossRef ] [ Google Scholar ]
- Hallberg L. Quality criteria and generalization of results from qualitative studies. International Journal of Qualitative Studies on Health and Wellbeing. 2013; 8 :1. doi: 10.3402/qhw.v8i0.20647. [ PMC free article ] [ PubMed ] [ CrossRef ] [ Google Scholar ]
- Hames I. Committee on Publication Ethics, 1. 2013, March. COPE Ethical guidelines for peer reviewers. Retrieved April 7, 2013, from http://publicationethics.org/resources/guidelines . [ Google Scholar ]
- Hooghe A, Neimeyer R. A, Rober P. “Cycling around an emotional core of sadness”: Emotion regulation in a couple after the loss of a child. Qualitative Health Research. 2012; 22 (9):1220–1231. doi: 10.1177/1049732312449209. [ PubMed ] [ CrossRef ] [ Google Scholar ]
- Jackson C. B, Botelho E. M, Welch L. C, Joseph J, Tennstedt S. L. Talking with others about stigmatized health conditions: Implications for managing symptoms. Qualitative Health Research. 2012; 22 (11):1468–1475. doi: 10.1177/1049732312450323. [ PMC free article ] [ PubMed ] [ CrossRef ] [ Google Scholar ]
- Jasper M, Vaismoradi M, Bondas T, Turunen H. Validity and reliability of the scientific review process in nursing journals—time for a rethink? Nursing Inquiry. 2013 doi: 10.1111/nin.12030. [ PubMed ] [ CrossRef ] [ Google Scholar ]
- Jensen J. L, Rodgers R. Cumulating the intellectual gold of case study research. Public Administration Review. 2001; 61 (2):235–246. doi: 10.1111/0033-3352.00025. [ CrossRef ] [ Google Scholar ]
- Jorrín-Abellán I. M, Rubia-Avi B, Anguita-Martínez R, Gómez-Sánchez E, Martínez-Mones A. Bouncing between the dark and bright sides: Can technology help qualitative research? Qualitative Inquiry. 2008; 14 (7):1187–1204. doi: 10.1177/1077800408318435. [ CrossRef ] [ Google Scholar ]
- Ledderer L. Understanding change in medical practice: The role of shared meaning in preventive treatment. Qualitative Health Research. 2011; 21 (1):27–40. doi: 10.1177/1049732310377451. [ PubMed ] [ CrossRef ] [ Google Scholar ]
- Lincoln Y. S. Emerging criteria for quality in qualitative and interpretive research. Qualitative Inquiry. 1995; 1 (3):275–289. doi: 10.1177/107780049500100301. [ CrossRef ] [ Google Scholar ]
- Luck L, Jackson D, Usher K. Case study: A bridge across the paradigms. Nursing Inquiry. 2006; 13 (2):103–109. doi: 10.1111/j.1440-1800.2006.00309.x. [ PubMed ] [ CrossRef ] [ Google Scholar ]
- Mawn B, Siqueira E, Koren A, Slatin C, Devereaux Melillo K, Pearce C, et al. Health disparities among health care workers. Qualitative Health Research. 2010; 20 (1):68–80. doi: 10.1177/1049732309355590. [ PMC free article ] [ PubMed ] [ CrossRef ] [ Google Scholar ]
- Merriam S. B. Qualitative research: A guide to design and implementation. 3rd ed. San Francisco, CA: Jossey-Bass; 2009. [ Google Scholar ]
- Meyer C. B. A case in case study methodology. Field Methods. 2001; 13 (4):329–352. doi: 10.1177/1525822x0101300402. [ CrossRef ] [ Google Scholar ]
- Morse J. M. Mixing qualitative methods. Qualitative Health Research. 2009; 19 (11):1523–1524. doi: 10.1177/1049732309349360. [ PubMed ] [ CrossRef ] [ Google Scholar ]
- Morse J. M. Molding qualitative health research. Qualitative Health Research. 2011; 21 (8):1019–1021. doi: 10.1177/1049732311404706. [ PubMed ] [ CrossRef ] [ Google Scholar ]
- Morse J. M, Dimitroff L. J, Harper R, Koontz A, Kumra S, Matthew-Maich N, et al. Considering the qualitative–quantitative language divide. Qualitative Health Research. 2011; 21 (9):1302–1303. doi: 10.1177/1049732310392386. [ PubMed ] [ CrossRef ] [ Google Scholar ]
- Nagar-Ron S, Motzafi-Haller P. “My life? There is not much to tell”: On voice, silence and agency in interviews with first-generation Mizrahi Jewish women immigrants to Israel. Qualitative Inquiry. 2011; 17 (7):653–663. doi: 10.1177/1077800411414007. [ CrossRef ] [ Google Scholar ]
- Nairn K, Panelli R. Using fiction to make meaning in research with young people in rural New Zealand. Qualitative Inquiry. 2009; 15 (1):96–112. doi: 10.1177/1077800408318314. [ CrossRef ] [ Google Scholar ]
- Nespor J. The afterlife of “teachers’ beliefs”: Qualitative methodology and the textline. Qualitative Inquiry. 2012; 18 (5):449–460. doi: 10.1177/1077800412439530. [ CrossRef ] [ Google Scholar ]
- Piekkari R, Welch C, Paavilainen E. The case study as disciplinary convention: Evidence from international business journals. Organizational Research Methods. 2009; 12 (3):567–589. doi: 10.1177/1094428108319905. [ CrossRef ] [ Google Scholar ]
- Ragin C. C, Becker H. S. What is a case?: Exploring the foundations of social inquiry. Cambridge: Cambridge University Press; 1992. [ Google Scholar ]
- Roscigno C. I, Savage T. A, Kavanaugh K, Moro T. T, Kilpatrick S. J, Strassner H. T, et al. Divergent views of hope influencing communications between parents and hospital providers. Qualitative Health Research. 2012; 22 (9):1232–1246. doi: 10.1177/1049732312449210. [ PMC free article ] [ PubMed ] [ CrossRef ] [ Google Scholar ]
- Rosenberg J. P, Yates P. M. Schematic representation of case study research designs. Journal of Advanced Nursing. 2007; 60 (4):447–452. doi: 10.1111/j.1365-2648.2007.04385.x. [ PubMed ] [ CrossRef ] [ Google Scholar ]
- Rytterström P, Unosson M, Arman M. Care culture as a meaning- making process: A study of a mistreatment investigation. Qualitative Health Research. 2013; 23 :1179–1187. doi: 10.1177/1049732312470760. [ PubMed ] [ CrossRef ] [ Google Scholar ]
- Sandelowski M. Whatever happened to qualitative description? Research in Nursing & Health. 2000; 23 (4):334–340. doi: 10.1002/1098-240X. [ PubMed ] [ CrossRef ] [ Google Scholar ]
- Sandelowski M. What's in a name? Qualitative description revisited. Research in Nursing & Health. 2010; 33 (1):77–84. doi: 10.1002/nur.20362. [ PubMed ] [ CrossRef ] [ Google Scholar ]
- Sandelowski M, Barroso J. Reading qualitative studies. International Journal of Qualitative Methods. 2002; 1 (1):74–108. [ Google Scholar ]
- Snyder-Young D. “Here to tell her story”: Analyzing the autoethnographic performances of others. Qualitative Inquiry. 2011; 17 (10):943–951. doi: 10.1177/1077800411425149. [ CrossRef ] [ Google Scholar ]
- Stake R. E. The case study method in social inquiry. Educational Researcher. 1978; 7 (2):5–8. [ Google Scholar ]
- Stake R. E. The art of case study research. Thousand Oaks, CA: Sage; 1995. [ Google Scholar ]
- Stake R. E. Case studies. In: Denzin N. K, Lincoln Y. S, editors. Strategies of qualitative inquiry. Thousand Oaks, CA: Sage; 1998. pp. 86–109. [ Google Scholar ]
- Sumsion J. Opening up possibilities through team research: Investigating infants’ experiences of early childhood education and care. Qualitative Research. 2013; 14 (2):149–165. doi: 10.1177/1468794112468471.. [ CrossRef ] [ Google Scholar ]
- Thomas G. Doing case study: Abduction not induction, phronesis not theory. Qualitative Inquiry. 2010; 16 (7):575–582. doi: 10.1177/1077800410372601. [ CrossRef ] [ Google Scholar ]
- Thomas G. A typology for the case study in social science following a review of definition, discourse, and structure. Qualitative Inquiry. 2011; 17 (6):511–521. doi: 10.1177/1077800411409884. [ CrossRef ] [ Google Scholar ]
- Tight M. The curious case of case study: A viewpoint. International Journal of Social Research Methodology. 2010; 13 (4):329–339. doi: 10.1080/13645570903187181. [ CrossRef ] [ Google Scholar ]
- Wager E, Kleinert S. Responsible research publication: International standards for authors. A position statement developed at the 2nd World Conference on Research Integrity, Singapore, July 22–24, 2010. In: Mayer T, Steneck N, editors. Promoting research integrity in a global environment. Singapore: Imperial College Press/World Scientific; 2010a. pp. 309–316. [ Google Scholar ]
- Wager E, Kleinert S. Responsible research publication: International standards for editors. A position statement developed at the 2nd World Conference on Research Integrity, Singapore, July 22–24, 2010. In: Mayer T, Steneck N, editors. Promoting research integrity in a global environment. Singapore: Imperial College Press/World Scientific; 2010b. pp. 317–328. [ Google Scholar ]
- Webb C, Kevern J. Focus groups as a research method: A critique of some aspects of their use in nursing research. Journal of Advanced Nursing. 2000; 33 (6):798–805. doi: 10.1046/j.1365-2648.2001.01720.x. [ PubMed ] [ CrossRef ] [ Google Scholar ]
- Wimpenny K, Savin-Baden M. Exploring and implementing participatory action synthesis. Qualitative Inquiry. 2012; 18 (8):689–698. doi: 10.1177/1077800412452854. [ CrossRef ] [ Google Scholar ]
- Yeh H.-Y. Boundaries, entities, and modern vegetarianism: Examining the emergence of the first vegetarian organization. Qualitative Inquiry. 2013; 19 (4):298–309. doi: 10.1177/1077800412471516. [ CrossRef ] [ Google Scholar ]
- Yin R. K. Enhancing the quality of case studies in health services research. Health Services Research. 1999; 34 (5 Pt 2):1209–1224. [ PMC free article ] [ PubMed ] [ Google Scholar ]
- Yin R. K. Case study research: Design and methods. 4th ed. Thousand Oaks, CA: Sage; 2009. [ Google Scholar ]
- Yin R. K. Applications of case study research. 3rd ed. Thousand Oaks, CA: Sage; 2012. [ Google Scholar ]
- Open access
- Published: 14 October 2024
Private hospitals in low- and middle-income countries: a typology using the cluster method, the case of Morocco
- Saad Zbiri ORCID: orcid.org/0000-0001-7059-0577 1 , 2 , 3 , 4 ,
- Abdelali Belghiti Alaoui 1 , 3 ,
- Imad El Badisy 1 , 2 , 5 ,
- Najib Diouri 6 ,
- Sanaa Belabbes 1 , 2 , 3 ,
- Radouane Belouali 1 , 2 , 3 &
- Zakaria Belrhiti ORCID: orcid.org/0000-0002-0115-682X 1 , 2 , 3
BMC Health Services Research volume 24 , Article number: 1231 ( 2024 ) Cite this article
Metrics details
The private healthcare sector has become an essential component of healthcare systems globally. This interest has increased with the universal health coverage agenda. However, in most low- and middle-income countries, few classificatory studies of the private hospital sector were carried out.
This study describes the private hospital sector in a developing country setup and propose a typology that could facilitate the identification of its categories and the understanding of its organizational and strategic characteristics.
All private hospitals in Morocco as of December 31, 2021 including 397 facilities are included. Most hospitals are for-profit, poly-disciplinary, independent, commercial societies, have fewer than 30 beds or between 30 and 99 beds and are located in urban areas. Private hospitals have a median turnover of 9.8 million MAD and a median capital value of 2 million MAD. The clustering method identifies three main categories of private hospitals: for-profit hospitals with medium size and turnover, spread across the country but with a high concentration in large regions; not-for-profit hospitals, with medium to large size, high turnover, located in large regions and including university hospitals; and small for-profit hospitals with low turnover, independent ownership and wide distribution over the country. Three criteria have the most significant discriminatory power: ownership, size (beds, turnover) and mode of governance.
Conclusions
Private hospitals in Morocco are organized into three types according to three similarity criteria including ownership, size and governance. These criteria might be used as the basis for a common typology of private hospitals in Morocco and possibly in other low- and middle-income countries with similar contexts.
Peer Review reports
Academic interest in the private healthcare sector dates back to the 1960s with the emergence of private for-profit hospital companies in the United States heralding the birth of the hospital industry. This birth occurred during a period of rapid growth in health expenditure and allowed the creation of a new competitive environment allowing hospitals to develop independently from health policies [ 1 ]. To segment this new market, a first categorization of hospitals was imposed through the identification of three types of establishments in the private hospital sector depending on the ownership of the hospitals: not-for-profit hospitals, independent hospitals and investor-owned hospitals. This development also enabled hospital activity to establish itself as an industrial category in the United Nations’ international classification of economic activities (ISIC rev.3) from 1989 and subsequently in other industrial classifications.
In developing countries, the dynamics of healthcare demand and supply have led to a growing need for private healthcare facilities. The area where private sector involvement held the greatest potential was in hospital care [ 2 ]. More recently, healthcare reforms in various countries have sought to increase the role of the private healthcare sector to complete public sector activities. The general argument was that these reforms could maintain equity in healthcare financing while promoting efficiency by introducing and encouraging competition. Today, the private sector is increasingly serving as a partner to public health systems, particularly in the provision of clinical care [ 3 ].
Yet, mixed evidence still exists on the relationship between hospital ownership (private not- for-profit, for-profit and public) and the variable effects on hospital performance and quality of care, as well as on out-of-pocket payments. These variations are largely explained by differences in institutional context, including differences between markets, regions, data sources and over time [ 4 , 5 , 6 ].
An increasing interest has been paid by international organizations in redefining the role of the private sector in health reforms [ 1 ] and in progressing towards achieving public health objectives [ 7 ]. In the same line, the WHO proposed a definition and an initial global typology of the private healthcare sector in 2005 [ 7 ]. Recently, this growing interest increased with the advent of universal health coverage (UHC) which led to considering the private sector as lever for health systems strengthening in all low and middle-income countries (LMICs) [ 8 , 9 ].
Currently, the private sector provides almost 40% of all health care in the Latin American, African and Western Pacific regions, 57% in the South-East Asian region and 62% in the Eastern Mediterranean region. Weighted regional results indicate that 53% of hospital care is provided in the private for-profit sector [ 10 ]. In OECD countries, private hospitals, both for-profit and not-for-profit, have gained significant shares of the healthcare market. They now represent 77% of hospitals in the United States, 75% in Germany, 56% in Spain, 55% in France and 48% in Australia [ 11 ].
However, there are very few descriptions of the profile of private hospitals in LMICs. In the Philippines, Lavado et al. were able to cover nearly all aspects of hospital organization. But their study only focused on four regions [ 12 ]. To our knowledge, this is the only study identified in LMICs and that covers so many variables. This can be explained by the fact that in the Philippines the private hospital sector is highly developed. In addition, very few studies have been carried out using clustering methods applied to private hospitals in LMICs. Most of these studies do not use national databases and do not offer a typology [ 13 ]. Some studies have used hospital clustering methods based on the characteristics of health professionals and not on the characteristics of hospitals [ 14 ]. Others studies have attempted a typology focusing on the entire private sector and not just on private hospitals [ 15 ].
Thus, through its evolution, the private hospital sector become an important component of the healthcare system and of the reform dynamics. Despite this importance, the classification and typology of private hospitals have received little attention from policy makers and researchers. This study argues that it is not possible to adequately approach the analysis and development of the private sector without an empirical understanding of its overall organization and characteristics. In this context, and in order to advance the understanding and documentation of the private hospital sector, we propose the development of a typology to better describe and understand the private hospital market. This typology could thus facilitate the identification of categories of private hospitals sharing common organizational and strategic characteristics, which could be used to analyse the dynamics of change in this sector. The relevance of this typology is tested through its application for the documentation of the private hospital sector in Morocco.
Study context
The Moroccan health system is organized into several interconnected healthcare networks, combining a dense public healthcare network in 12 decentralized health regions, and an expanding private healthcare sector, located mainly in major urban areas, particularly on the Casablanca-Rabat axis. In 2021, the public sector comprises 2985 primary healthcare centers providing supportive, preventive and curative care [ 16 ]. Secondary and tertiary care are provided by a pyramid-shaped public hospital network including 165 local, regional and inter-regional hospitals (as well as 157 support facilities) accounting for a total of 26 771 beds and 13 682 medical practitioners. The public sector has a ratio of 1375 inhabitants per hospital bed. The private sector has over 12 534 beds and 14 199 medical practitioners [ 17 ]. Most private physicians are self-employed but some physicians are public-sector employees and are practicing in private hospitals (part-time practice or dual practice). The capital of private hospitals is funded by independent actors (mostly physicians) (86%), national and international financial holdings (8%), health insurance organizations (3,3%) and non-profit foundations (2,6%) [ 18 ].
Delimitation of the study object
The overall definition of what constitutes a “hospital” is similar in all countries, with specific characteristics inherent to each country’s history. The word “hospital” currently covers a wide range of institutions, from small rural facilities to large university clinics, from small community centers to giant complexes with multiple head offices and large numbers of employees [ 19 ]. In the United Nations classification of all economic activities (ISIC rev.4), hospitals are understood as “ human health institutions which have accommodation facilities and which engage in providing diagnostic and medical treatment to inpatients with any of a wide variety of medical conditions ” [ 20 ]. In the OECD classification of systems of health accounts, hospitals are defined as “ licensed establishments that are primarily engaged in providing medical , diagnostic and treatment services that include physician , nursing and other health services , to inpatients and the specialized accommodation services required by inpatients ” [ 21 ]. In some countries, health facilities must additionally have a minimum size, such as a number of beds and medical staff to ensure 24-hour access, in order to be registered as a hospital. Currently, the private hospital sector comes in many shapes and sizes, ranging from small physician-owned facilities to large, publicly traded, for-profit hospital chains [ 22 ].
In a similar vein, private hospitals are, according to the global health observatory of the WHO, “ hospitals not owned by government or to parastatal organizations ”. They include both private not-for-profit hospitals and private for-profit hospitals [ 10 ]. Thus, a private hospital is a non-state-owned health facility authorized to provide specialized inpatient and/or outpatient healthcare. It is sometimes called a private clinic (Morocco, Tunisia) or a private health establishment (France). In this work, these names will be used interchangeably.
Framework development
The rationale behind developing a typology of healthcare facilities is to allow for continuous refinement of actors, understanding and analysis of the healthcare system and systematic organizational analysis. It also facilitates comparison by referring to the same categorization criteria, and can be used as a regulatory framework. Typologies are thus a useful and widely used tool in understanding and describing health organizations. Thereby, the use of typologies makes it possible to reduce the complexity of “messy” empirical realities, allowing it to systematically analyse similarities and variations and to detect and interpret patterns [ 23 ].
To develop a framework to categorize private hospitals, we adopted an empirically-driven approach rather than a theoretically-driven approach. The empirical approach is not based on a theoretical pre-selection of relevant indicators to deduce ideal types but rather on empirical data [ 24 ]. We built our framework primarily on international classifications governing the hospital market as a health industry (United Nations, OECD, WHO, Research and Markets) (see Additional file 1 for more details), discussed and supplemented by empirical policy documents based on country experiences (Morocco, Tunisia, France, United States) (see Additional file 2 for more details). Therefore, the resulting typology corresponds to a real categorization (clusters) and not to a theoretical modelling. We adopted a single two-step approach, in which the cluster analysis followed the descriptive analysis and literature review. The steps of the typology building process are described in Fig. 1 .
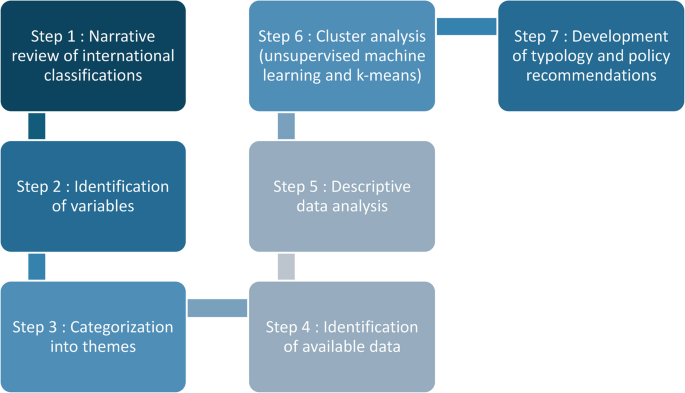
Flow diagram of the typology building process
In this context, our narrative review of international classifications enabled us to identify nine criteria with 15 variables for the categorization of private hospitals (Table 1 ).
Collection of data
We collected data in Morocco during the year 2021 using two main databases:
The database of the Ministry of Health relating to the supply of care [ 25 ].
The private database of Inforisk SA. specialized in legal and financial information on companies. It is the richest and most up-to-date database on Moroccan companies. Its platform Charika.ma provides access to legal and financial information on more than 760 000 Moroccan companies and more than 500 million companies in 230 countries around the world.
These two databases have been cross-referenced, cleaned up and completed by collecting updated information through direct contact with private hospital key stakeholders. These collected data were the subject of a two-stage analysis. First, a descriptive statistical analysis to define the profiles of private hospitals in Morocco was based on seven criteria and ten variables, as shown in Table 1 , including hospital ownership, specialisation, number of beds, type of governance, declared turnover, capital, legal form, university status, population covered and region. Second, a cluster analysis was performed to identify a private hospital typology.
Cluster analysis
Factor, cluster and discriminant analyses are used to analyse data collected from private hospitals for the year 2021. Private hospital data were analysed using an unsupervised clustering approach, where nine variables with seven criteria were used to construct the clusters. These variables cover the main characteristics of private hospitals. The other two criteria including membership and performance, as well as six variables were not analysed, as the corresponding information is not available in the majority of private hospitals.
The method used to construct the clusters is a mixed method based on the combination of the k-means algorithm [ 26 ] and Gower’s distance measure [ 27 , 28 ].
In practice, a dissimilarity matrix between observations is first calculated. Then, this matrix is used as input to the k-means algorithm.
The k-means method aims to separate data points with different characteristics into different clusters and group data points with similar characteristics together. It is an iterative algorithm that, regardless of its starting point, converges to a solution. For each starting point, a different solution can be found. The calculations are repeated several times to select the best solution for the chosen criterion.
Iteratively, a starting point is chosen for the first iteration, which consists of matching observations with the centroids of the k clusters. After determining the distance of the observations from the k centroids, they are assigned to the closest one. The observations are then redistributed according to their distance from the new centroids, and so on, until convergence is achieved.
The optimal number of clusters can be determined using a variety of techniques. In our study, we used the elbow method [ 29 ]. It uses sum-of-square to evaluate the goodness of split. Then, an elbow plot of sum-of-square for k values ranging from two to N is created. As a rule, when k increases, the corresponding sum-of-square will decrease. A trade-off between k (i.e. number of clusters) and sum-of-square can be observed. Typically, the optimal value of k starts to flatten out and forms an elbow shape.
All the analysis was carried out using the R software [ 30 ].
Ethical and regulatory considerations
As the study is retrospective, based on anonymized data and purely observational, it was exempt from institutional review board approval according to the Moroccan legislation (law number 28 − 13) [ 31 ].
A total of 397 private hospitals are considered in this study and are thus included in the clustering algorithm. They represent all private hospitals in Morocco, called private clinics, as of December 31, 2021. “Establishments assimilated” to private clinics, such as dialysis centers and medical radiology centers, are not taken into consideration in this study because they do not correspond to our definition of a private hospital.
Private hospital characteristics
The distribution of the characteristics of the study hospitals are presented in Table 2 . Regarding hospital ownership, the majority (93.2%) are for-profit hospitals and 6.8% are not-for-profit hospitals. However, the latter represent 17.3% of the total bed capacity of private hospitals due to the importance of their size. 18.6% of the study hospitals are mono-disciplinary while 81.4% are poly-disciplinary. 50.4% of private hospitals have less than 30 beds and barely 5.8% have 100 beds or more. In terms of geographical distribution, among the 12 regions in Morocco, 5 regions include 79.3% of private hospitals (Casablanca-Settat, Rabat-Sale-Kenitra, Marrakech-Safi, Fes-Meknes and Tanger-Tetouan-Al Hoceima). The Casablanca-Settat region alone includes 30% of private hospitals and 40% of their beds, at a time when two regions have none. Also, the majority of private hospitals are located in urban areas (89.4% versus 10.6%). In terms of governance, the majority (86.6%) are independent hospitals while 7.3%, 2.8% and 3.3% are corporate group, health network and under tutelage private hospitals, respectively. The turnover of the study hospitals ranges from 6.5 thousand to 178 million Moroccan dirhams (MAD), with a median of 9.8 million MAD and a mean of 16 million MAD. Regarding the value of capital, nearly 55% of private hospitals report it among their data. It varies from 10 thousand to 775 million MAD with an average of 8.3 million and a median of 2 million MAD. The range of variation in turnover and capital is too wide between private hospitals. This shows that the declaration of these values is most likely not well regulated. Finally, regarding their legal form, 92.2% of hospitals are commercial societies (SA, SARL, SARLAU or SNC), 1% are civil societies (SCP), 3.5% are non-governmental organizations (NGOs) or foundations and 3.3% have other legal forms.
Number of clusters
One of the most difficult problems in any applied cluster analysis is determining the number of clusters in which to group the data. Our clustering separation metrics recommend three as the number of clusters that convey most information. This is consistent with the aspect of the elbow plot, which shows that the additional contribution to the variance explained is smaller after three clusters (Fig. 2 ). Following the elbow plot and the majority rule, the number of clusters was set to three.
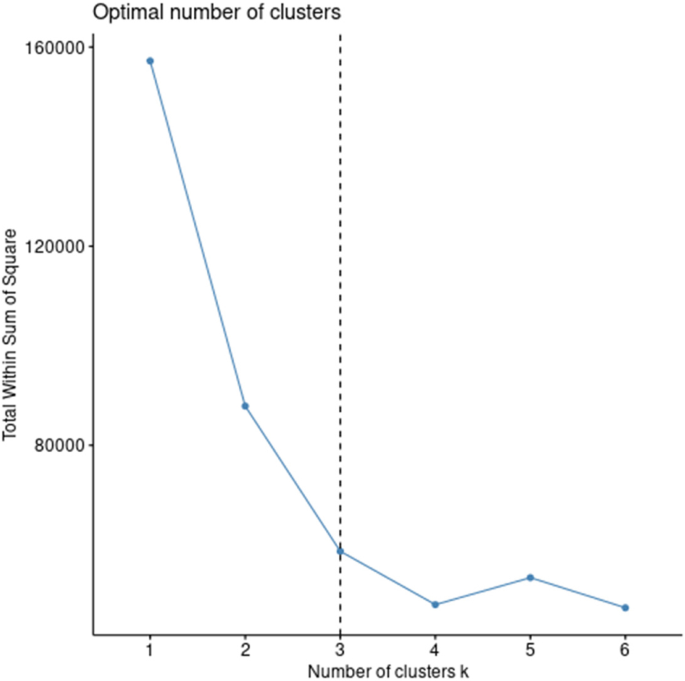
Cluster separation according to the number of groups in the clustering algorithm
Private hospital categories
The hospital profiles identified by the k-means algorithm are presented in Table 3 . The hospitals included in each cluster have different patterns.
Cluster 1 consists of 169 private hospitals. All hospitals are for-profit and most of them are poly-disciplinary (91.7%). The number of beds is average with 93.5% hospitals having between 30 and 99 beds, and only 6.5% having 100 beds or more. In terms of governance, hospitals are mainly independent (88.8%) and 11.2% are part of corporate groups. The turnover of the hospitals ranges from 492 thousand to 87.7 million MAD, with a mean of 19.2 million MAD and a median of 14.5 million MAD. The most common legal form is commercial society (99.4%) while only one hospital is a civil society owned hospital. All hospitals are non-teaching centers and the majority of them are located in the urban area (94.7%). Hospitals are located in the region of Casablanca-Settat (37.3%), the region of Rabat-Sale-Kenitra (16%), the region of Fes-Meknes (11.2%), the region of Oriental (9.5%), the region of Marrakech-Safi (8.9%), the region of Tanger-Tetouan-Al Hoceima (6.5%), the region of Souss-Massa (6.5%), the region of Beni Mellal-Khenifra (3%) and the region of Draa-Tafilalet (1.2%).
Cluster 2 is the smallest cluster including 32 private hospitals. The majority of hospitals are not-for-profit (84.4%) and poly-disciplinary (87.5%). The number of beds is medium to high with 37.3% of hospitals having 100 beds or more, 50% having between 30 and 99 beds and only 12.5% having less than 30 beds. In terms of governance, most hospitals are under tutelage (40.6%) or in network (34.4%) and only few hospitals are independent (12.5%) or part of corporate groups (12.5%). Hospital turnover is high and ranges from 2.9 to 178 million MAD, with a mean of 54.5 million MAD and a median of 37.6 million MAD. In terms of hospital legal form, most hospitals are NGOs or foundations (43.8%) or had other forms (40.6%), few hospitals are commercial societies (15.6%) and none is a civil society. 12.5% of hospitals are teaching centers. Most hospitals are in the urban area (81.3%) and mainly concentrated in the region of Casablanca-Settat (43.8%), followed by the region of Tanger-Tetouan-Al Hoceima (21.9%), the region of Marrakech-Safi (12.5%) and the region of Rabat-Sale-Kenitra (9.4%).
Cluster 3 is the largest cluster with 196 private hospitals. All hospitals are for-profit and mono-disciplinary (28.6%) or poly-disciplinary (71.4%). The number of beds is low with all hospitals having less than 30 beds. Regarding governance, most hospitals are independent (96.9%) and only 3.1% are part of corporate groups. The turnover of the hospitals is low and ranges from 6.5 thousand to 55.9 million MAD, with a mean of 7 million MAD and a median of 7.5 million MAD. The most common legal form is commercial society (98.5%) and only few hospitals are civil societies (1.5%). All hospitals are non-teaching centers. 86.2% of hospitals serve a predominantly urban population and 13.8% serve a predominantly rural population. Hospitals are well distributed over the national territory with 21.4% in the region of Casablanca-Settat, 19.4% in the region of Rabat-Sale-Kenitra, 12.8% in the region of Marrakech-Safi, 11.2% in the region of Fes-Meknes, 11.2% in the region of Tanger-Tetouan-Al Hoceima, 10.2% in the region of Beni Mellal-Khenifra, 9.2% in the region of Souss-Massa, 3.1% in the region of Oriental, 1% in the region of Draa-Tafilalet and 0.5% in the region of Guelmim-Oued Noun.
Therefore, three categories of private hospitals stand out. A first type which groups together for-profit hospitals, rather poly-disciplinary and independent, mainly commercial companies, with medium size and turnover. They are spread over the whole territory, but with a high concentration in large regions. A second type of private hospitals, which is mainly made up of non-profit hospitals, rather poly-disciplinary, in network or under tutelage, with medium to large size and high turnover. They are concentrated in large regions and may be university hospitals. A third type of hospitals is a group of private for-profit hospitals, rather independent, with small size and low turnover and well distributed over the territory.
Thus, the Table 3 shows that the variables which have the most significant discriminatory power in this segmentation are: the property, the number of beds, the turnover and the governance. The characteristics relating to the other variables studied are practically found in the three clusters. These four discriminating variables refer to three criteria (Table 1 ): the ownership, size of hospitals (beds and turnover) and governance. These criteria can be used for the classification of private hospitals in LMICs which often do not have large databases of private health facilities.
Sensitivity analysis
Figure 3 displays the distribution of hospitals in the three clusters. Observations (i.e. hospitals) are represented by points and variables have been transformed into two dimensions using principal component analysis. This representation shows the distribution of observations in the three clusters, represented on a Euclidean space. This sensitivity check confirms that the three private hospital clusters are distinct. We can thus consider that our clustering algorithm used is robust.
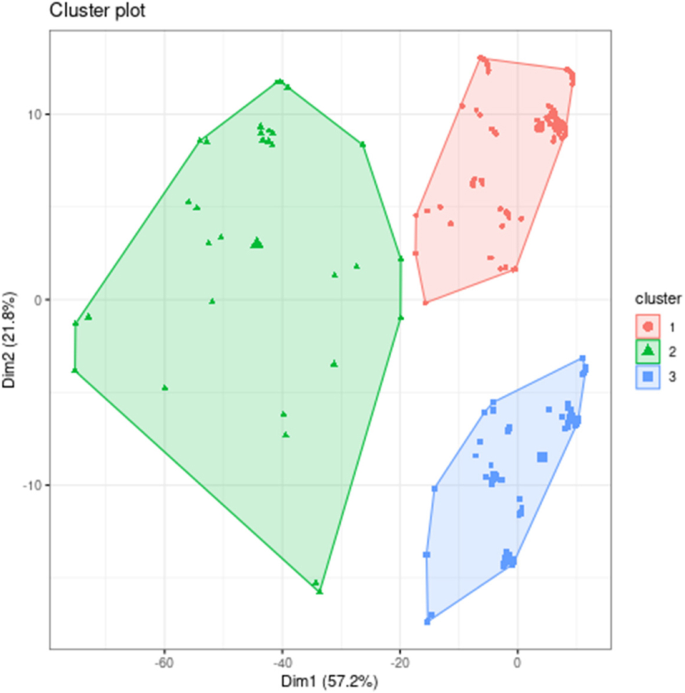
Distribution of observations in the three clusters
The private health sector plays an important and growing role in the healthcare systems of the LMICs. But significant gaps remain about its characteristics and development. Due to various demographic and epidemiological changes, the public sector has been overwhelmed with the demand for healthcare services, especially services provided by hospitals. This forced changes in health markets, which led to significant increases in private sector participation in healthcare delivery [ 3 ]. The international community, including the WHO, lacks a common framework for analysing the private health sector. It also lacks an evidence base from which to develop guidance on the types of services and activities where it might have a role or comparative advantage in strengthening health systems [ 9 ]. Whatever approach is taken to overcoming this obstacle, it must begin by understanding the rules that govern the organization and functioning of the private care sector.
Our study focuses on private hospitals, which are health facilities that contain a lot of equipment and consume a lot of resources, and whose use is costly for users and for the UHC. In Morocco, there are 397 private hospitals with 14 502 beds in 2021. They thus represent 70.6% of all hospitals (public and private) and 35.2% of the national capacity in hospital beds (excluding military hospitals). In addition, the private hospital sector is the preferred recourse for beneficiaries of compulsory health insurance [ 32 ]. Since 2022, this health insurance has been generalized to the entire Moroccan population. This makes knowledge of the private hospital sector useful for achieving universal access to healthcare and for regulating health insurance.
Almost all private hospital classifications have a legal rather than an empirical basis. To understand the organization and real characteristics of the private hospital sector in Morocco, we adopted a two-step approach in our study: (i) a descriptive approach based on a statistical analysis including ten variables identified through a review of the literature on hospital classification; and (ii) an unsupervised learning approach based on clustering algorithm (k-means) to identify hidden patterns or groupings in our empirical data.
The first stage of analysis is the most common in the literature. It allowed us to better define the profile of private hospitals since it was based on ten variables, while the private hospital data collected by the Ministry of Health is based on only three variables: the ownership, the number of beds and the geographical site. Compared to Morocco, Tunisia has the same basis for describing the private hospital sector, with an additional criterion relating to specialization [ 33 ]. Due to lack of data, our descriptive analysis of private hospitals did not cover variables relating to human resources, medical technology and use of services, as is the case in the OECD database (OECD.stat) considered among the most important health databases [ 11 ]. Despite its importance, OECD.stat remains a general health database. Indeed, compared to our typology, the OECD database does not include key variables such as turnover, capital, governance and ownership, which are essential to describe the profile of private hospitals. This means that our analysis is more specific to the private hospital sector than that of the OECD. As for the WHO, its global health observatory has no data on private hospitals and its description of the hospital sector is limited to the overall density of beds [ 10 ]. Thus, the results of our descriptive analysis provide a better understanding of the profile of private hospitals and their differential characteristics compared to public hospitals. They help identify gaps in healthcare coverage and guide healthcare planning. This better description of hospital profiles also makes it possible to draw cross-national comparisons. However, despite its importance, the private hospital sector is not considered in the analysis of healthcare systems at the global level.
According to the second stage of analysis, it was a question of building a typology by using a grouping algorithm based on our empirical data covering the entire national territory. The clustering method showed that it is possible to build a solid segmentation with only three criteria of similarity: ownership, hospital size (beds and turnover) and governance. Hospital size related criterion can be enriched by the use of data on staff, technology and on patients when they exist. In our study, we identified three main types of private hospitals. The first type is made up of for-profit, independent or group hospitals, which are medium in size and turnover. The second type is made up of large private, not-for-profit, under tutelage or networked hospitals with high turnover. The third type is made up of small, for-profit, independent, low-turnover hospitals.
In high-income countries, hospital clustering aimed to analyse trends in hospital organization (vertical and horizontal integration) [ 34 ]. Some countries have even applied it to the public hospital sector [ 35 ]. Some high-income countries categorize private hospitals according to their function (general or specialist care; acute or long-term) or according to their legal status or ownership, such as in the United States, France or Germany. In the United States, due to the development of hospital groups, hospital segmentation is organized into systems and networks to inform health policy and practice, as well as research on the performance of different health groups and clusters [ 36 ]. In Morocco, the creation of hospital groups is a relatively recent phenomenon. Although it concerns 41 private hospitals, it did not impose itself as a cluster whatever the number k of groups explored with k-means algorithm.
Thus, this single two-step approach to analyse the private hospital sector, adopted by our study, appear to be comprehensive. The descriptive approach makes it possible to define the private hospital general profile based on specific variables identified and tested. As for the unsupervised learning approach, it makes it possible to build clusters of hospitals on the basis of attributes of similarities identified in a more objective way by clustering algorithm. This enables to systematically analyse similarities and variation as well as detect and interpret patterns for a better understanding of the dynamics of this sector which is still poorly understood in LMICs.
Our findings may have several implications for public authorities. This study provides valuable insights into the private hospital market in Morocco, which could be used to inform policy and decision-making in the healthcare sector. Understanding the characteristics and emerging typology of private hospitals can help to better regulate the private sector and to better plan its complementarity with the public sector. In addition, both in Morocco and in similar LMICs, private hospitals play an increasing role in the healthcare system, providing a substantial portion of healthcare services to the population, and playing a crucial role in achieving UHC. Clarke et al. highlight the importance of the private sector in UHC and consider that it is necessary to have political commitment and effective regulation to optimize its potential [ 9 ]. Fallah and Bazrafshan conducted a systematic scoping review of evidence from developing countries, examining the participation of private hospitals in delivering services towards UHC [ 37 ]. Siddiqi et al. draw lessons from country experiences to understand the role of the private sector in delivering health benefit packages of UHC [ 38 ]. These studies collectively underscore the significance of the private sector in the context of UHC, while also highlighting challenges and lessons learned from country experiences. Similarly, Jeurissen et al. suggested the role of public support and reimbursement strategies within UHC reforms in promoting the growth of the private sector in the United States, the United Kingdom, Germany, and the Netherlands [ 22 ]. These lessons could inspire private sector growth in Morocco, from small physician-owned private hospitals to private hospital networks.
Overall, these studies highlight the heterogeneity of the private hospital sector in LMICs and the need to consider its diversity when designing health policies and regulations. In addition, the majority of these studies were descriptive and did not include a clustering algorithm of the data. Our study is the first in Morocco and among the few in LMICs to identify the typology of private hospitals using an unsupervised clustering approach. Another strength of our study is the use of a database that includes all private hospitals in the country. Our study also used a robust methodology and two-stage approach to categorize a new typology of the private sector hospitals that presents high contextual relevance for the ongoing implementation of UHC policy and health system reform in Morocco. In addition, the ten variables used considered a wide range of hospital characteristics that, to our knowledge, have never been analysed before in Morocco and in the LMICs. However, our study may have some limitations. A limitation is that our analysis did not include some important hospital characteristics, such as staffing, technology and utilization, due to the unavailability of these data. This shows the importance of feeding the routine information system with data from the private sector. Another limitation of our study is that our literature review was not comprehensive, but was based on a narrative review of typologies adopted by international organizations.
In LMICs, the private hospital sector is not sufficiently known. The data available to define its profile are insufficient to provide an understanding of its organization and the dynamics of its development. LMIC governments will find it difficult to define the role of this sector in improving access to healthcare and in moving towards UHC. The national information systems of these countries must therefore be strengthened and enriched with data on the private healthcare sector. In the meantime, studies similar to our can be considered to position and regulate the private hospital sector. We consider that the results of our study offer a starting point for adapting the regulation and governance of this sector in Morocco and in LMICs. However, our single case study is not sufficient to test the transferability of our typology. Further research needs to be carried out in other contexts to judge its generalizability to other similar LMICs contexts.
In Morocco, the routine information system has few variables to describe the profile of the private hospital sector while it represents more than a third of all hospitals. We explored the characteristics and typology of the private hospital sector in Morocco using ten variables. Our results show that the private hospital sector is organized into three types of hospitals according to three similarity criteria: ownership, size (beds and turnover) and governance. This typology contributes to the understanding of the development dynamics of this sector and can guide efforts to plan and regulate the supply and demand of care in the current context of the generalization of compulsory health insurance which makes the private sector open to beneficiaries of all medical coverage schemes. Our study has several policy implications. It offers policymakers with a typology of private facilities that may guide the formulation of policies to enhance the growth of the private sector in order to improve regulation, supervision and the design of appropriate policies and incentives to increase quality of care, performance and accessibility of care within the context of UHC extension and decentralization reform.
Availability of data and materials
The data that support the results of this study cannot be publicly available because the source of data did not provide permission to do so.
Abbreviations
International Standard Industrial Classification of All Economic Activities
Low and Middle-Income Countries
Moroccan Dirhams
Organisation for Economic Co-operation and Development
Universal Health Coverage
World Health Organization
Institute of Medicine. The New Health Care for profit: doctors and hospitals in a competitive environment. Washington, DC: National Academies; 1983.
Google Scholar
Griffin CC. Strengthening health services in developing countries through the private sector. Discussion Paper 4. Washington, DC: International Finance Corporation. 1989.
Nah S-H, Osifo-Dawodu E. Establishing Private Health Care Facilities in developing countries: a guide for medical entrepreneurs. World Bank; 2007.
Eggleston K, Shen YC, Lau J, Schmid CH, Chan J. Hospital ownership and quality of care: what explains the different results in the literature? Health Econ. 2008;17(12):1345–62.
Article PubMed Google Scholar
Herrera CA, Rada G, Kuhn-Barrientos L, Barrios X. Does ownership matter? An overview of systematic reviews of the performance of private for-profit, private not-for-profit and public healthcare providers. PLoS ONE. 2014;9(12):e93456.
Article PubMed PubMed Central Google Scholar
Weinberger M, Bellows N, Stover J. Estimating private sector out-of-pocket expenditures on family planning commodities in low-and-middle-income countries. BMJ Glob Health. 2021;6(4):e004635.
Word Health Organization - WHO. Working with the non-state sector to achieve public health goals. 2005. https://apps.who.int/iris/handle/10665/69202 (Accessed: 4 Jun 2023).
Morgan R, Ensor T, Waters H. Performance of private sector health care: implications for universal health coverage. Lancet. 2016;388(10044):606–12.
Clarke D, Doerr S, Hunter M, Schmets G, Soucat A, Paviza A. The private sector and universal health coverage. Bull World Health Organ. 2019;97(6):434–5.
Word Health Organization - WHO. Global Health Observatory. 2020. https://www.who.int/data/gho (Accessed: 4 Jun 2023).
Organisation for Economic Co-operation and Development - OECD. OECD.Stat. 2021. https://stats.oecd.org/ (Accessed: 4 Jun 2023).
Lavado RF, Ulep VGT, Pantig IMT, Ortiz DAP, Jimenez JF, Datu MBG, Banzon EP. Profile of Private Hospitals in the Philippines. Discussion Papers DP 2011-05. Philippine Institute for Development Studies. 2011.
Romadon YW, Fitrianah D. The comparative study on Clustering Method Using Hospital Facility Data in Jakarta District and surrounding areas. Int J Mach Learn Comput. 2019;9(6):749–55.
Article Google Scholar
Setiawan KE, Kurniawan A, Chowanda A, Suhartono D. Clustering models for hospitals in Jakarta using fuzzy c-means and k-means. Procedia Comput Sci. 2023;216:356–63.
Mackintosh M, Channon A, Karan A, Selvaraj S, Cavagnero E, Zhao H. What is the private sector? Understanding private provision in the health systems of low-income and middle-income countries. Lancet. 2016;388(10044):596–605.
Belrhiti Z, Van Belle S, Criel B. How medical dominance and interprofessional conflicts undermine patient-centred care in hospitals: historical analysis and multiple embedded case study in Morocco. BMJ Glob Health. 2021;6(7):e006140.
Ministry of Health of Morocco. Health in figures 2021. https://www.sante.gov.ma/Documents/2024/04/Sante%20en%20chiffre%202021%20VF%20(2).pdf
Competition Council of the Kingdom Of Morocco. Notice of the Competition Council concerning the competitive operation of the market for medical care provided by private clinics and similar establishments in Morocco. Rabat. 2022. https://conseil-concurrence.ma/wp-content/uploads/2023/10/Avis-du-Conseil-de-la-Concurrence-Avis-A.4.22-FR-.pdf
Durán A, Wright S. Understanding hospitals in changing Health systems. Ed. Palgrave Macmillan; 2020.
United Nations. International Standard Industrial Classification of all Economic Activities (ISIC) Rev.4. New York: United Nations; 2008. p. 252.
Organisation for Economic Co-operation and Development - OECD. A system of Health accounts 2011: revised edition. Paris: OECD Publishing; 2017.
Jeurissen PPT, Kruse FM, Busse R, Himmelstein DU, Mossialos E, Woolhandler S. For-profit hospitals have Thrived because of generous public reimbursement schemes, not Greater Efficiency: a Multi-country Case Study. Int J Health Serv. 2021;51(1):67–89.
de Carvalho G, Schmid A, Fischer J. Classifications of health care systems: do existing typologies reflect the particularities of the Global South? Global Social Policy. 2021;21(2):278–300.
Reibling N, Ariaans M, Wendt C. Worlds of Healthcare: a Healthcare System Typology of OECD Countries. Health Policy. 2019;123(7):611–20.
Ministry of Health of Morocco. Health care services in Morocco. 2021. http://cartesanitaire.sante.gov.ma/dashboard/pages2/index_2021.html (Accessed: 29 Mar 2023).
Hartigan JA, Wong MA, Algorithm. AS 136: a K-Means Clustering Algorithm. J Royal Stat Soc Ser C (Applied Statistics). 1979;28(1):100–8.
Gower JC. A general coefficient of similarity and some of its properties. Biometrics. 1971;27(4):857–71.
Foss AH, Markatou M, Ray BK. Distance metrics and clustering methods for mixed-type data. Int Stat Rev. 2019;87:80–109.
Kodinariya TM, Makwana PR. Review on determining number of Cluster in K-Means clustering. Int J. 2013;1:90–5.
R Core Team. The R Project for Statistical Computing. 2022. https://www.r-project.org/ (Accessed: 4 Jun 2023).
Ministry of Health of Morocco. Law no. 28 – 13 on the protection of persons participating in biomedical research. 2015. https://www.sante.gov.ma/Reglementation/REGLEMENTATIONDESPRATIQUESMEDICALES/28-13.pdf (Accessed: 1 Jun 2023).
Ministry of Health of Morocco. National health accounts. 2018. p. 113.
Ministry of Health of Tunisia. Health care services in Tunisia. 2022. http://www.santetunisie.rns.tn/fr/carte-sanitaire/carte-sanitaire-2011 (Accessed: 4 Jun 2023).
Thomas JW, Griffith JR, Durance P. Defining hospital clusters and associated service communities in metropolitan areas. Socioecon Plann Sci. 1981;15(2):45–51.
Chrusciel J, Le Guillou A, Daoud E, Laplanche D, Steunou S, Clément MC, Sanchez S. Making sense of the French public hospital system: a network-based approach to hospital clustering using unsupervised learning methods. BMC Health Serv Res. 2021;21(1):1244.
Nolte E, Pitchforth E, Miani C, Mc Hugh S. The changing Hospital Landscape: An Exploration of International experiences. Rand Health Q. 2014;4(3):1.
PubMed PubMed Central Google Scholar
Fallah R, Bazrafshan A. Participation of delivering private hospital services in universal health coverage: a systematic scoping review of the developing countries’ evidence. J Educ Health Promot. 2021;10:113.
Siddiqi S, Aftab W, Venkat Raman A, Soucat A, Alwan A. The role of the private sector in delivering essential packages of health services: lessons from country experiences. BMJ Glob Health. 2023;8(Suppl 1):e010742.
Download references
Acknowledgements
None declared.
No funding received.
Author information
Authors and affiliations.
Mohammed VI International School of Public Health, Mohammed VI University of Sciences and Health (UM6SS), Boulevard Mohammed Taïeb Naciri, Commune Hay Hassani, 82 403, Casablanca, Morocco
Saad Zbiri, Abdelali Belghiti Alaoui, Imad El Badisy, Sanaa Belabbes, Radouane Belouali & Zakaria Belrhiti
Laboratory of Public Health, Health Economics and Health Management, Mohammed VI Center for Research and Innovation (CM6RI), Rabat, Morocco
Saad Zbiri, Imad El Badisy, Sanaa Belabbes, Radouane Belouali & Zakaria Belrhiti
Knowledge for Health Policies Center, Casablanca, Morocco
Saad Zbiri, Abdelali Belghiti Alaoui, Sanaa Belabbes, Radouane Belouali & Zakaria Belrhiti
Institut d’Analyse des Systèmes de Santé (IA2S), Paris, France
Platform for bioinformatics and data analysis, Mohammed VI Center for Research and Innovation (CM6RI), Rabat, Morocco
Imad El Badisy
Alium Santé Maroc, Casablanca, Morocco
Najib Diouri
You can also search for this author in PubMed Google Scholar
Contributions
ABA and ND provided the data. IEB and SZ analysed the data. SZ and ABA wrote the manuscript. ZB, RB and SB edited the manuscript. All authors approved the final manuscript.
Corresponding author
Correspondence to Saad Zbiri .
Ethics declarations
Ethics approval and consent to participate.
The data used for this study is retrospective, based on anonymized data, purely observational and do not involve humans. Therefore, the study does not require specific institutional review board approval and consent to participate, according to the Moroccan legislation (law number 28 − 13) [ 31 ].
Consent for publication
Not applicable.
Competing interests
The authors declare no competing interests.
Additional information
Publisher’s note.
Springer Nature remains neutral with regard to jurisdictional claims in published maps and institutional affiliations.
Supplementary Information
Supplementary material 1, supplementary material 2, rights and permissions.
Open Access This article is licensed under a Creative Commons Attribution-NonCommercial-NoDerivatives 4.0 International License, which permits any non-commercial use, sharing, distribution and reproduction in any medium or format, as long as you give appropriate credit to the original author(s) and the source, provide a link to the Creative Commons licence, and indicate if you modified the licensed material. You do not have permission under this licence to share adapted material derived from this article or parts of it. The images or other third party material in this article are included in the article’s Creative Commons licence, unless indicated otherwise in a credit line to the material. If material is not included in the article’s Creative Commons licence and your intended use is not permitted by statutory regulation or exceeds the permitted use, you will need to obtain permission directly from the copyright holder. To view a copy of this licence, visit http://creativecommons.org/licenses/by-nc-nd/4.0/ .
Reprints and permissions
About this article
Cite this article.
Zbiri, S., Belghiti Alaoui, A., El Badisy, I. et al. Private hospitals in low- and middle-income countries: a typology using the cluster method, the case of Morocco. BMC Health Serv Res 24 , 1231 (2024). https://doi.org/10.1186/s12913-024-11660-2
Download citation
Received : 29 September 2023
Accepted : 26 September 2024
Published : 14 October 2024
DOI : https://doi.org/10.1186/s12913-024-11660-2
Share this article
Anyone you share the following link with will be able to read this content:
Sorry, a shareable link is not currently available for this article.
Provided by the Springer Nature SharedIt content-sharing initiative
- Private sector
- Characteristics
BMC Health Services Research
ISSN: 1472-6963
- General enquiries: [email protected]
Thank you for visiting nature.com. You are using a browser version with limited support for CSS. To obtain the best experience, we recommend you use a more up to date browser (or turn off compatibility mode in Internet Explorer). In the meantime, to ensure continued support, we are displaying the site without styles and JavaScript.
- View all journals
- Explore content
- About the journal
- Publish with us
- Sign up for alerts
- Open access
- Published: 13 October 2024
Machine learning-aided hybrid technique for dynamics of rail transit stations classification: a case study
- Ahad Amini Pishro 1 , 3 ,
- Shiquan Zhang 2 ,
- Alain L’Hostis 3 ,
- Yuetong Liu 2 ,
- Qixiao Hu 2 ,
- Farzad Hejazi 4 ,
- Maryam Shahpasand 5 ,
- Ali Rahman 6 ,
- Abdelbacet Oueslati 7 &
- Zhengrui Zhang 1
Scientific Reports volume 14 , Article number: 23929 ( 2024 ) Cite this article
Metrics details
- Applied mathematics
- Civil engineering
- Computational science
Accurate classification of rail transit stations is crucial for successful Transit-Oriented Development (TOD) and sustainable urban growth. This paper introduces a novel classification model integrating traditional methodologies with advanced machine learning algorithms. By employing mathematical models, clustering methods, and neural network techniques, the model enhances the precision of station classification, allowing for a refined evaluation of station attributes. A comprehensive case study on the Chengdu rail transit network validates the model’s efficacy, highlighting its value in optimizing TOD strategies and guiding decision-making processes for urban planners and policymakers. The study employs several regression models trained on existing data to generate accurate ridership forecasts, and data clustering using mathematical algorithms reveals distinct categories of stations. Evaluation metrics confirm the rationality and accuracy of the results. Additionally, a neural network achieving high accuracy on labeled data enhances the model’s predictive capabilities for unlabeled instances. The research demonstrates high accuracy, with the Mean Squared Error (MSE) for regression models (Multiple Linear Regression (MLR), Deep-Learning Neural Network (DNN), and K-Nearest Neighbor (KNN)) remaining below 0.012, while the neural networks used for station classification achieve 100% accuracy across seven time intervals and 98.15% accuracy for the eighth, ensuring reliable ridership forecasts and classification outcomes. Accuracy in rail transit station classification is critical, as it not only strengthens the model’s predictive capabilities but also ensures more reliable data-driven decisions for transit planning and development, allowing for more precise ridership forecasts and evidence-based strategies for optimizing TOD. This classification model provides stakeholders with valuable insights into the dynamics and features of rail transit stations, supporting sustainable urban development planning.
Similar content being viewed by others
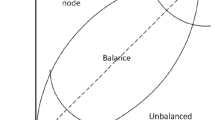
Node, place, ridership, and time model for rail-transit stations: a case study
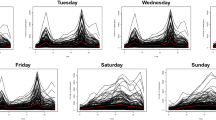
Machine learning approach for study on subway passenger flow
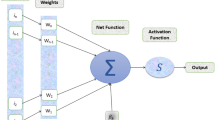
Deep learning solutions for smart city challenges in urban development
Introduction.
Transit-Oriented Development (TOD) is a strategic urban planning and design framework that establishes mixed-use, high-density developments close to public transportation infrastructure. The core tenets of TOD entail optimizing land utilization, fostering pedestrian-friendly environments, and facilitating seamless access to efficient public transit systems 1 , 2 . By concentrating development activities around transit nodes, TOD aims to minimize reliance on private automobiles, curb traffic congestion, and mitigate environmental impacts 3 . In addition to these primary objectives, TOD enhances urban livability by creating vibrant, walkable communities that foster social interaction and a strong sense of place. By reducing the need for long commutes, TOD contributes to better work-life balance and improved public health. Furthermore, the emphasis on mixed-use development supports local economies by attracting diverse businesses and services, providing job opportunities, and stimulating local investment.
TOD’s multifaceted significance lies in its capacity to enhance transportation efficiency, bolster sustainability, stimulate economic growth, elevate the quality of life, and promote social equity. By strategically integrating residential, commercial, and recreational spaces within walking distance of transit hubs, TOD optimizes travel distances, cultivates vibrant neighborhoods, reduces energy consumption, mitigates greenhouse gas emissions, attracts business investment, generates fiscal revenues, and fosters inclusive communities that cater to diverse income groups. As a genuine urbanistic concept and hence multiscale by design, TOD conveys a transit line and network dimension that connects to urban and urban region planning perspectives.
Classifying rail transit stations using mathematical methods involves algorithms and models, including machine learning and clustering techniques, to examine and categorize stations based on passenger flow, connectivity, and infrastructure features. K-Means is a renowned method for grouping data into K-distinct sections by reducing the total variance within the clusters 4 . K-Means is suitable for the first attempt at analyzing rail transit station data because its iterative and straightforward nature makes it an easy option. For instance, in a TOD scenario, K-Means can quickly and effectively identify similar stations regarding ridership volume, connectivity, and accessibility. This kind of clustering is of great help for urban planners in listing and counting transport hubs and identifying unfulfilled stations and urban zones that might need some infrastructural improvements 5 , 6 , 7 . It is one of the most effective ways to concentrate resources and pave the path for urban development through smart infrastructural design.
AGNES clustering, a type of hierarchical clustering, creates interior clusters by combining or dividing them based on particular conditions. Unlike K-Means, K-Means does not require knowing the number of clusters earlier; instead, it makes the procedure more flexible and adjustable 8 . In terms of rail transit stations, AGNES is a significant way to identify the network hierarchical structure, finding both macro and micro-clusters. This tool is very efficient in recognizing the direct and indirect connections between stations and in the station’s hierarchy, whether it is a main, a branch, or a leaf, and it also gives an overall impact of the network topology on strategic planning for TOD, among other things 9 .
DBSCAN, a density-based clustering algorithm, determines the clusters of various shapes and sizes other than the density of data points. The method of dealing with noise and outliers is one of its most prominent advantages 10 . DBSCAN can reveal sets of spherical stations in transportation stations, which is not the case for natural K-Means. This is the primary TOD application because it can find city blocks that are lastly joined or other problematic places that may result from stations being isolated or performing at lower levels. DBSCAN is an all-around tool that improves transit networking for better passenger service through decisions on the density of station usage and connectivity 11 .
GMM is one of the basic paradigmatic methods of cluster analysis, which is grounded on the a priori assumption of the mixture of several pairs of Gaussian distributions. This action provides an all-around perspective necessary for the group’s different shapes, sizes, and orientations, making it highly versatile and robust 12 . By applying GMM technology to rail transit stations, we can also analyze the complex station characteristics and usage patterns and thus gain a deep insight into the transit network. The stochastic feature of the process is also an economic assignment that allows the stations to be put into clusters, with weights exerted by the GMM but part of the weight belonging to the so-called ``soft’’ group that sometimes assigns the stations if they are used for public services or are dwelling in or nearby communities. This ability is vitally important in the field of TOD, as it is critical for developing multifunctional strategies that consider each platform’s different roles and necessities.
Applying innovative clustering methods to rail transit station classification is a new approach in TOD studies. Each contributes differently to the analysis, providing fresh insights and suggestions for city planners and politicians 4 , 5 , 6 , 7 , 8 , 9 , 10 , 11 , 12 , 13 , 14 , 15 . By utilizing the four approaches, namely K-Means, AGNES, DBSCAN, and GMM, the TOD projects will get a much clearer picture of transit networks, better resource allocation, eco-friendly urban development, and data utilization in decision-making. These modes of operations can fully delineate the transit system, whereby the mind can be steered to the primary nodes, connections, and areas that need to be developed. Technical approaches such as modeling the travel time of linear vibrating PETs (public electric transportation) and the angle of environmental noise sources at the same place coordinates can be utilized for safety and shared streets. The concept of “equal access” can be best understood by inspectors of social safety in real-time in mixed and shared traffic and pedestrians through digital twins of ADA/VDAs. Nevertheless, the ultimate goal is to ensure that users and dependents experience transportation benefits without safety incidents. In particular, these ways of examination not only enrich the information necessary for the formation of the most optimum distribution of available transit system facilities but also provide very relevant and effective instruments for urban development that are both sustainable and efficient.
In this context, classifying railway stations is critical to the design of urban and regional planning strategies. Accurate classification enables planners to tailor development projects to each station’s specific characteristics and needs, ensuring efficient resource allocation and optimal land use. This precision in planning facilitates the integration of transportation networks, promotes seamless connectivity, and supports the creation of cohesive and sustainable urban environments. Furthermore, such classifications provide valuable insights for policymakers, helping them implement policies that align with the goals of TOD and address the unique challenges different regions face.
Literature review
The classification of rail transit systems plays a crucial role in Transit-Oriented Development (TOD) as it profoundly impacts the scale, design, and planning considerations of development projects centered around transit stations. This classification informs decisions on development intensity, station design, infrastructure requirements, and transportation network integration 16 , 17 , 18 . Urban planners and developers leverage this classification to optimize land use, design efficient stations, plan for TOD projects, and create comprehensive transportation networks that seamlessly connect different modes of travel 19 , 20 .
Using clustering methods from mathematics enhances rail transit classification by extracting meaningful patterns and objectively categorizing transit systems. Clustering algorithms enable the recognition of similarities and shared characteristics among systems, employing unsupervised learning to identify inherent structures and relationships within large datasets 21 . By leveraging clustering algorithms, transportation professionals can make data-driven decisions, improving the reliability and scalability of the classification process. Clustering results provide valuable insights for future planning, benchmarking, and resource allocation, facilitating the development of efficient and optimized rail transit systems 22 , 23 .
The application of Artificial Neural Network (ANN), including some aspects of mathematics such as Multiple Linear Regression (MLR), is a hybrid tool to study the relationship between different parameters in a model 15 , 24 , 25 . The utilization of Machine Learning (ML) in rail transit classification is paramount due to its ability to enable data-driven decision-making, handle complex and multidimensional data, uncover hidden patterns, adapt to changing conditions, and support continuous improvement. Machine learning algorithms excel at processing vast volumes of data and extracting meaningful insights that may not be apparent through traditional analysis methods 26 , 27 , 28 , 29 . By leveraging machine learning techniques, transportation professionals can make informed decisions based on the intricate relationships and dependencies identified within rail transit data. These algorithms facilitate the identification of clusters or groups of similar transit systems, leading to more accurate and granular classification outcomes.
Jingru Huang et al. 30 investigated the relationship between the physical environment and subway ridership in Beijing. The results indicated that greater employment density and enhanced accessibility to public transportation contribute to higher ridership during morning peak hours. The research also highlighted variations in the built environment’s impact on ridership across different areas and confirmed the reliability of the model used in the analysis. Liu Yang et al. 31 investigated different methods for categorizing TOD in cities, with Ningbo as the focus. Their research introduced a novel approach to improve TOD based on metro stations, expanding the traditional node-place model with additional attributes and utilizing machine learning for station selection. The study’s findings provided insights for other cities with urban rail transit systems and suggested avenues for future research on analyzing traffic behavior and adjusting land-use strategies in TOD. Enrica Papa et al. 32 classified station areas and promoted public transport use as a solution to mitigate the negative impacts of private car usage in cities. Through cluster analysis, factors influencing these areas were examined, and a method for identifying different station area typologies was proposed. The approach was demonstrated in Naples, showcasing the integration of land-use and transport planning strategies to improve rail stations. Shiliang Su et al. 33 analyzed the specific impact of TOD on metro ridership in Shanghai. The study identified spatial and temporal variations in the influence of TOD factors and neighborhood demographics by combining the node-functionality-place model with interpretable machine learning. The findings underscored the importance of functionality, revealed key interactions, and offered insights for urban planning in high-density cities. Dan Qiang et al. 9 analyzed the metro stations in Shanghai using new urban datasets and identified five clusters based on 15 indicators for TOD. The study found strong correlations between transportation, pedestrian-oriented accessibility, and urban development indicators with ridership, emphasizing the importance of population density in metro passenger traffic.
Xin Yang et al. 34 focused on applying neural networks in TOD and specifically addressed the complex task of short-term prediction of passenger volume in urban rail systems. The study proposed an improved spatiotemporal long short-term memory model (Sp-LSTM) that utilized deep learning techniques and big data, outperforming other prediction methods such as LSTM, ARIMA, and NAR, as demonstrated through a case study on the Beijing Metro Airport Line. In another research 35 , they introduced the Wave-LSTM model, a combination of LSTM and wavelet techniques, which demonstrated superior prediction accuracy compared to other algorithms in an empirical study using practical data from Dongzhimen Station in the Beijing Subway system. The study concluded that the newly proposed model holds great potential as a reliable approach for predicting precise short-term inbound passenger flow in urban rail systems. Jinlei Zhang et al. 36 highlighted the importance of short-term origin-destination (OD) flow prediction in urban rail transit (URT) for real-time operation and management. They introduced a channel-wise attentive split-convolutional neural network (CAS-CNN) that addressed the challenges of data availability, dimensionality, and sparsity. Through testing on real-world datasets from the Beijing Subway, the CAS-CNN model, incorporating innovative components such as channel-wise attention and inflow/outflow-gated mechanisms, outperformed existing benchmarking methods. Chunyan Shuai et al. 37 proposed a pattern match algorithm, TSNE-KNN, for accurate short-term origin-destination (OD) demand prediction in urban rail transit. The TSNE-KNN model outperformed other approaches, including deep neural network models, and identified similarity indicators as universal indicators reflecting the time-space properties of OD flow and the shifting patterns of rail transit stations.
Regarding the limitations in model accuracy, predictive capability, and scalability in the literature, we first acknowledge that previous studies have made significant contributions by proposing methods to enhance accuracy, provide objective evaluations, enable predictive capabilities, and facilitate scalability. However, these limitations and challenges have often been inadequately addressed or only superficially mentioned. Therefore, this study seeks to clarify these aspects and provide concrete details regarding the limitations faced in existing models and methodologies.
Although several studies 9 , 30 , 34 , 36 have demonstrated that machine learning models such as ANN, LSTM, and Sp-LSTM improve predictive accuracy for rail transit systems, the accuracy of these models still faces challenges due to the inherent complexity of urban transit systems. Existing methods, particularly those relying on traditional clustering algorithms (K-Means, DBSCAN), are limited in accurately classifying stations with mixed or transitional characteristics where different clusters overlap. These models may exhibit high accuracy under specific conditions but struggle with highly dynamic and non-linear ridership patterns, especially when faced with new, unseen data. Our approach addresses this limitation by integrating more advanced techniques like deep neural networks (DNNs), which handle complex patterns better, but we also note that model accuracy may still be affected by factors such as data quality and the availability of real-time datasets.
While predictive capabilities in previous research have been advanced by introducing models like Wave-LSTM 34 and CAS-CNN 36 , limitations remain in their ability to predict ridership fluctuations under varying temporal conditions and in stations with irregular passenger volumes. The short-term prediction models, although effective for certain types of data, often fail to generalize across different station types (e.g., those with fluctuating passenger volumes during peak and off-peak hours). Moreover, the scalability of predictive models for short-term origin-destination flow prediction is constrained when moving from small datasets to larger, city-wide applications. Our work seeks to improve this by incorporating predictive models considering a more nuanced range of variables, including socio-economic factors and real-time ridership data, which enhance predictive capabilities over broader datasets. However, predictive accuracy is still limited by the availability of comprehensive data across all stations.
The scalability of rail transit classification methods has been significantly challenged by the complexity of urban transit systems, particularly when expanding from city-level studies to regional or national networks. Studies such as Su et al. 33 and Shuai et al. 37 have focused on specific cities (e.g., Shanghai and Beijing), and the proposed models excel at handling localized datasets but struggle to scale effectively across diverse transit networks with different ridership behaviors and infrastructure designs. Furthermore, the computational demands of machine learning algorithms, such as deep learning models, can become a bottleneck when applied to larger datasets. While our research incorporates scalable algorithms such as GMM and hierarchical clustering methods, we acknowledge that further optimization is necessary to ensure computational efficiency and practicality when applying these models to large-scale TOD planning.
This study builds upon these previous findings by introducing a more systematic and data-driven method to evaluate and classify rail transit stations into clusters based on their characteristics. The research applies clustering algorithms such as K-Means, AGNES, DBSCAN, GMM, and other applicable mathematics and machine learning tools 26 , 27 , 28 , 29 to provide a precise classification method for rail transit stations. By enhancing accuracy, providing objective evaluations, enabling predictive capabilities, and facilitating scalability for large-scale analyses, this innovation empowers urban planners and policymakers with valuable insights for sustainable and efficient urban development, optimizing TOD outcomes. This approach leverages existing methodologies and addresses their limitations by incorporating advanced machine-learning techniques to achieve more accurate and practical results for TOD planning.
Case study and methodology
Chengdu, the capital city of Sichuan province in China, has a well-developed rail transit network that provides efficient and convenient transportation for its residents and visitors. The Chengdu rail transit system consists of metro lines and high-speed rail connections. The Chengdu Metro is the backbone of the city’s urban transportation system. The city center of Chengdu is located around Tianfu Square, which is considered the heart of the urban area. Key commercial districts, cultural landmarks, and government offices are concentrated in this central area. The urban region of Chengdu is densely developed with a mix of high-rise buildings, shopping centers, and residential complexes. Beyond the Third Ring Road, the city transitions into suburban areas with lower-density development and a mix of residential and industrial zones. The Fourth Ring Road generally marks the boundary between the suburban and rural areas, where farmland and small villages become more common. The rail transit system in Chengdu has dramatically improved the city’s transportation efficiency, reducing congestion and providing a convenient way for people to travel within and outside the city. It has played a significant role in enhancing Chengdu’s urban development and supporting its economic growth.
For several compelling reasons, Chengdu’s metro network serves as an excellent case study for Transit-Oriented Development (TOD) and the classification of rail transit stations. Firstly, the metro network in Chengdu boasts extensive coverage, encompassing a vast area that includes urban and suburban regions. The network connects vital city areas, including the city center, central commercial districts, educational institutions, and suburban communities. This extensive reach ensures that many people can access efficient public transportation. This expansive reach provides diverse station locations with unique characteristics and development potential. Secondly, many of Chengdu’s metro stations are strategically located in areas characterized by mixed land use. These areas combine residential, commercial, and recreational facilities, setting the stage for integrated development around the stations. A diverse range of land uses facilitates the creation of walkable neighborhoods and vibrant urban environments. Moreover, Chengdu’s rapid urban growth has presented numerous development opportunities around metro stations. Underutilized or vacant land near stations can be transformed through redevelopment and revitalization initiatives, fostering economic growth and community enhancement.
Chengdu’s commitment to TOD is evident in its urban planning and policy framework. The local government has implemented various measures to encourage the integration of transportation, land use, and urban design. Policies promoting high-density development around metro stations, mixed-use zoning, and pedestrian-friendly infrastructure have been critical to the city’s TOD success. The government has also invested in public amenities, green spaces, and cultural facilities around transit hubs to enhance the quality of life for residents.
The significant passenger volume of Chengdu’s metro system is another compelling aspect. With many daily commuters, the stations attract high levels of foot traffic. This demand creates a favorable market for various businesses and services to thrive around the stations, further stimulating TOD. Additionally, the local government in Chengdu has proactively promoted TOD and urban development around metro stations. Through the implementation of policies and strategies, they encourage the integration of transportation, land use, and urban design. These initiatives aim to create sustainable, livable communities that benefit from well-planned transit-oriented environments.
The success of Chengdu’s metro network and TOD initiatives can be attributed to several factors, including strategic planning, strong government support, and community engagement. The city’s approach to TOD addresses transportation and land use and focuses on creating vibrant, inclusive, and sustainable urban environments. Lessons from Chengdu’s experience can inform TOD projects in other cities, highlighting the importance of comprehensive planning, stakeholder collaboration, and adaptive strategies to local contexts.
Researchers and planners can gain valuable insights into effective station classification and development practices by examining the success of Chengdu’s metro network and its associated TOD efforts. The lessons learned from Chengdu can serve as a valuable guide for future TOD projects in other cities, aiding in creating sustainable and vibrant urban areas. Figure 1 presents the Chengdu rail transit network, the CBDs of Chunxi Road, the Third Tianfu Street, and the boundaries of the rural and urban areas.
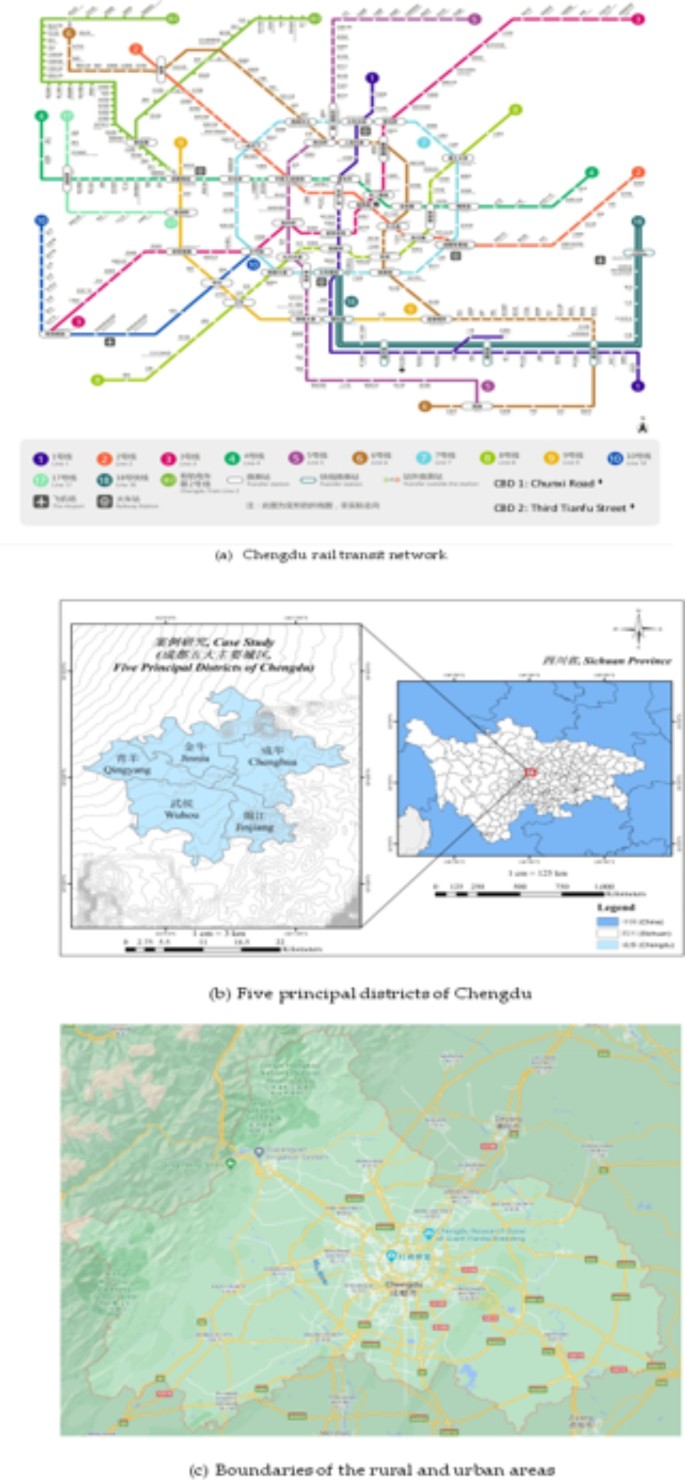
Chengdu: key insights and structure.
Problem statement
By examining the Chengdu Metro system, the study provides a comprehensive understanding of how various factors influence ridership patterns. This paper’s primary focus is divided into ridership fitting and station classification. The first part of the analysis involves fitting ridership data to assess the relationships between ridership levels and variables such as node value, place value, and time factors. This approach helps to elucidate how these variables interact and contribute to the overall ridership figures. In the second part of the study, stations are classified based on a combination of node value, place value, ridership, and time. This classification process reveals patterns and distinctions among stations, offering insights into their operational and functional characteristics. Identifying these commonalities and differences is crucial for tailoring specific strategies to optimize station performance and enhance the overall efficiency of the transit network.
The challenges in solving the problem of predicting subway ridership and categorizing stations stem from the complex, dynamic nature of urban transit systems. Accurately predicting passenger flow at subway stations requires a comprehensive understanding of how factors such as station characteristics, location, and time affect ridership. This study employs different fitting methods to model ridership for Chengdu subway stations, aiming to select the most suitable prediction model. Additionally, subway stations, particularly those intersected by multiple lines, display unique characteristics while sharing common traits. Categorizing these stations through clustering techniques in machine learning helps identify patterns that can inform the design and planning of new stations. However, the unlabeled nature of stations and the varying outcomes from different clustering methods pose challenges, which are addressed by integrating results and using a neural network to classify stations and predict the characteristics of new ones. Figure 2 illustrates the research framework of this study.
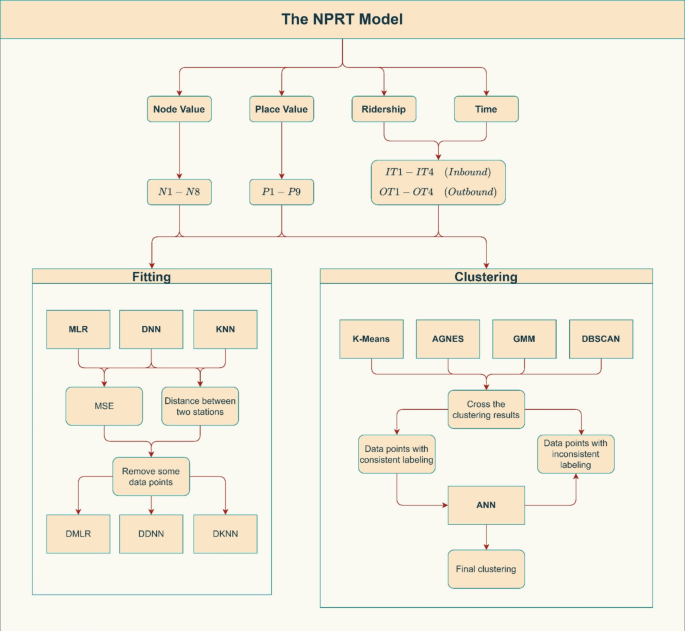
Structured research framework and methodological workflow.
Node, place, ridership, and time indicators
Node indicators.
We evaluate the value of a station’s node based on four aspects: the station’s facilities, the availability of nearby transportation options, the accessibility to various destinations, and its importance within the network. Table 1 presents eight indicators that fall under these four aspects.
The station’s facilities are assessed by considering the number of entrances and exits (N1) in each metro station. To determine the accessibility of transits, we consider the number of metro stations (N2) that can be reached within a 20-minute travel time, the number of stations connecting to the central business district (CBD) at Chunxi Road (N3), and the number of stations connecting to the CBD at 3rd Tianfu Street (N4). Since Chengdu has two CBDs, Chunxi Road and 3rd Tianfu Street, we calculated the number of stations and the distance to each CBD. The distance indicates the accessibility of destinations to the CBDs, with Chunxi Road represented by (N5) and 3rd Tianfu Street by (N6). Lastly, the network centrality comprises degree centrality (N7) and closeness centrality (N8).
Using graph modeling, we have employed network centrality to capture the significance of a station within the transit network. To represent the Chengdu rail transit network as a graph \(\:G=(V,E)\) , we assign vertices in \(\:V\) to represent the stations, while the set \(\:E\) consists of edges denoting the connections between stations. The weight of each edge in \(\:E\) is determined by the transit traveling distance.
To measure the degree centrality (N7) of a transit station \(\:v\:\in\:\:V\) in the Chengdu network, we consider the number of links connected to station \(\:v\) in Eq. ( 1 ). Here, \(\:{L}_{vt}\) represents the linkage between station \(\:v\) and another station \(\:t\:\in\:\:V\) , while \(\:K\) represents the total number of stations in set \(\:V\) :
Closeness centrality indicates how close and accessible a node is within the network component. The closeness centrality (N8) measurement for station is determined by the inverse sum of the shortest transit distances from station \(\:v\) to all other stations in set \(\:V\) , as shown in Eq. ( 2 ). Here, \(\:{d}_{vt}\) represents the shortest transit distance between station \(\:v\) and another station \(\:t\:\in\:\:V\) :
Place indicators
To account for the low-density nature of certain areas in Chengdu, we establish a transit catchment area using a radius of 500 m and 1000 m. The evaluation of a station’s place value involves three factors: design, density, and diversity. Table 1 presents nine place indicators categorized under these three factors.
The design aspect is measured using various metrics. These include the average price of office land within the 1000 m-radius catchment area (P1), the average price of commercial land within the 1000 m-radius catchment area (P3), the average price of residential land within the 1000 m-radius catchment area (P5), the number of parking lots within the 500 m-radius catchment area (P8), and the number of bus stops within the 500 m-radius catchment area (P9). Furthermore, design is also assessed by considering the number of offices within 1000 m (P2), the number of shops within 1000 m (P4), and the number of residences within 1000 m (P6). The diversity factor encompasses public facilities such as parks, cultural facilities, schools, and hospitals within the 1000 m-radius catchment area (P7).
Ridership and time indicators
To address the limitations of the NPR model, which fails to consider the impact of time and overlooks variations in ridership between departures and arrivals, we have introduced the recording of tapped-in and tapped-out trips to construct an NPRT model that accounts for different conditions.
As previously mentioned, ridership is closely tied to time. Hence, we have classified passenger traffic into inbound traffic (I) and outbound traffic (O). We have further divided the time into peak, off-peak, regular, and weekends, denoted as T1 to T4. This results in eight distinct conditions. For example, IT1 represents inbound traffic during working hours, IT2 represents inbound traffic during off-peak hours, IT3 represents inbound traffic during the remaining hours of the workday, and IT4 represents inbound traffic on two weekend days. Similarly, OT1 denotes the ridership of passengers leaving the station during working hours, OT2 represents ridership during off-peak hours, OT3 represents ridership during the remaining hours of the workday, and OT4 represents ridership on two weekend days.
Table 2 provides a detailed breakdown of each class and the corresponding time intervals from IT1 to OT4.
Methodology
Information entropy weighting (iew).
To facilitate the analysis of the data and compose the indicators, Information Entropy Weighting ( \(\:IEW\) ) 17 was used to integrate \(\:{N}_{1}-{N}_{8}\) into node value ( \(\:N\) ) and \(\:{P}_{1}-{P}_{9}\) into place value ( \(\:P\) ). When the information entropy is lower, the significance of the index in providing information decreases, resulting in a minor role in the comprehensive evaluation, and thus, a lower weight should be assigned to it. Therefore, information entropy can be used to calculate the weight of each index, taking \(\:N\) as an example. And similarly, we can get \(\:P\) . If there are \(\:n\) stations and \(\:m\) node value indicators, then we get \(\:X\) , where \(\:{x}_{ij}\) represents the value of the indicator \(\:j\) at station \(\:i\) .
Algorithm 1 provides the IEW method applied in this study.
Algorithm 1
Step 1. 0-1 normalized the matrix \(\:X\) .
Step 2. Calculate the proportion of each station for indicator \(\:j\) .
Step 3. Calculate the information entropy of each indicator. If \(\:{p}_{ij}=0\) , specify \(\:\text{ln}\left(0\right)=0\) .
Step 4. Calculate the imbalance coefficient.
Step 5. Calculate the weight of each indicator.
Step 6. The weighted sum of the values for each station is normalized to get the node value.
Tables 3 and 4 , and 5 present the normalized values of node, place, and integrated ridership-time indicators of some subway stations provided by the Min-Max Normalization method.
Cluster methods
Unsupervised learning is necessary when dealing with training samples lacking labeling information. The goal is to assign labels to stations based on node value, place value, and ridership at various times, creating a tagged dataset. This labeled data can be further used to train the rail transit stations’ classification model with classification techniques to predict new stations.
Clustering methods are employed to partition existing stations and unveil the underlying structure of the data to accomplish this objective. The paper utilizes four clustering methods, which are described below.
The K-Means represents a prototyping-based clustering algorithm 4 , 5 . In this algorithm, we randomly select \(\:k\) data from the dataset as the initial centers of the clusters and assign each point in the dataset to the cluster to which the point belongs to the nearest center. A new cluster center can be computed from the most recently delineated cluster; thus, the cluster to which the data point belongs can be reassigned. Repetition of such operations until convergence allows the realization of K-Means. Therefore, when training the model for K-Means, we need to consider tuning the hyperparameters for the number of clusters \(\:k\) .
The AGNES is a hierarchical clustering algorithm that operates on a bottom-up aggregation approach 8 . It begins by treating each object as an individual cluster and subsequently merges clusters step by step based on specific criteria. The similarity matrix can be obtained by calculating the distance between any two clusters, and thus, the two closest clusters \(\:{C}_{i}\) and \(\:{C}_{j}\) can be found. We merge them into the same cluster \(\:{C}_{h}\) and also update the distances related to both to get a new similarity matrix. The process is repeated until the algorithm stops when all data points belong to the same cluster. Since the distance metrics available for calculating the distance between clusters are ward connected, wholly connected, average connected, etc., the distance calculation method \(\:linkage\) needs to be considered in addition to the number of clusters \(\:k\) .
The DBSCAN stands for Density-Based Spatial Clustering of Applications with Noise and is a density-based clustering algorithm 10 , 11 . Its fundamental concept revolves around assessing whether data points belong to the same cluster by identifying adjacent points around the data points and recursively exploring the adjacent points around those neighbors.
Two essential parameters are at the core of the DBSCAN algorithm: radius (ε) and minimum sample size (MinPts). The parameter ε defines the distance threshold for a sample’s neighborhood, while MinPts specifies the minimum number of samples within that neighborhood (defined by ε distance) to be considered part of a cluster.
The GMM (Gaussian Mixture Model) is a probabilistic model that characterizes the cluster prototype. The cluster partition is determined by the prototype corresponding to the posterior probability 12 . GMM uses
where \(\:P\left(\varvec{x}|{\varvec{\mu\:}}_{\varvec{j}},{\varSigma\:}_{j}\right)\) is a Gaussian distribution with mean \(\:{{\upmu\:}}_{j}\) variance \(\:{{\Sigma\:}}_{j}\) and with a non-negative weight \(\:{{\upalpha\:}}_{j}\) constituting the overall distribution \(\:p\left(x|{\Theta\:}\right)\) , and \(\:{\sum\:}_{j=1}^{k}{{\upalpha\:}}_{j}=1\) , \(\:{\Theta\:}=\{{\alpha\:}_{j},{\varvec{\mu\:}}_{\varvec{j}},{{\Sigma\:}}_{j}|j=\text{1,2},\:\dots\:\:,k\}\) .
k represents a parameter to be tuned.
According to the rule, the samples \(\:{x}_{i}\) are assigned to the cluster \(\:{{\uplambda\:}}_{i}\) corresponding to the Gaussian distribution with the highest probability of belonging to it. Based on the dataset \(\:D\) , the parameter \(\:{\Theta\:}\) of the GMM can be solved iteratively by the EM algorithm maximizing its log-likelihood function.
Performance measurements
Two main types of clustering performance measures exist. The first type involves comparing the clustering results with a reference model, which can be the division results provided by domain experts or inherent data categories. This category of measures is referred to as the external index. The second type of measure evaluates the clustering results directly without relying on any reference model, known as the internal index.
External index For data set \(\:D=\{{x}_{1},{x}_{2},\dots\:,{x}_{m}\}\) :
It is assumed that the cluster given by clustering is divided into \(\:C\) , and the cluster given by the reference model is divided into \(\:{C}^{*}\) . Accordingly, \(\:\lambda\:\) and \(\:{\lambda\:}^{*}\) represent the cluster label vectors corresponding to \(\:C\) and \(\:{C}^{*}\) , respectively. When pairing the samples in pairs, the number of unique pairs, denoted by \(\:a+b+c+d=\frac{m(m-1)}{2}\) .
Jaccard Coefficient ( \(\:JC\) ):
Its result is in the interval [0,1], and the larger the value, the better.
Rand Index ( \(\:RI\) ) and Adjusted Rand Index ( \(\:ARI\) ):
\(\:RI\) ranges from \(\:\:\left[\text{0,1}\right]\) . The larger the value, the better. To realize that the index should be close to 0 when the clustering results are generated randomly, \(\:ARI\) is proposed. Its value range is \(\:[-\text{1,1}]\) , and the larger the value, the more consistent the clustering result is with the actual situation.
Internal index:
The Sum of Squared Errors ( \(\:SSE\) ):
It represents the sum of the square loss of the distance from the data in the class to the center of the class, which is the optimization goal of \(\:\text{K}-\text{M}\text{e}\text{a}\text{n}\text{s},\) where \(\:{x}_{i,k}\) denotes the \(\:i\) -th sample point in the \(\:k\) -th class and \(\:{c}_{k}\) is the center point of the \(\:k\) -th class.
Silhouette Coefficient ( \(\:SC\) ):
In Eq. ( 20 ) \(\:{a}_{i}\) represents the average distance of the sample \(\:{x}_{i}\) from other samples in the cluster and \(\:{b}_{i}\) represents the minimum average distance of \(\:{x}_{i}\) from samples in other clusters. The range of \(\:SC\) is \(\:[-\text{1,1}]\) . A clustering result with a higher \(\:SC\) value, closer to 1, indicates a better clustering effect. This is achieved when the distances between samples of the same class are smaller, and the distances between samples of different classes are larger.
Calinski-Harabasz Index ( \(\:CH\) ):
In Eqs. ( 22 ) and ( 23 ) \(\:{B}_{k}\) and \(\:{W}_{k}\) are covariance matrices for inter-cluster and intra-cluster data. \(\:{c}_{q}\) and \(\:{c}_{*}\) represent the center points of cluster \(\:q\) and the data set \(\:D,\) respectively, and \(\:{C}_{q}\) denotes the set of data belonging to cluster \(\:q\) . The \(\:CH\) score represents the ratio between the distance between clusters and the distance within clusters. A higher value indicates a better clustering result because the score has no upper bound. In other words, the larger the \(\:CH\) score, the more distinct and well-separated the clusters are, which is considered a better outcome.
Fitting and classification methods
MLR is a simple linear regression generalization that studies the quantitative dependence between dependent and multiple independent variables 24 , 25 . The multiple linear regression equation estimated by the sample is:
In multiple linear regression analysis, the regression coefficient is estimated by the least square method, that is, finding the appropriate coefficients \(\:\{{b}_{0},{b}_{1},\ldots,{b}_{p}\}\) to minimize the sum of squares of the dependent variable residuals.
DNN (Deep Neural Network) is a neural network comprising multiple hidden layers, categorized as input, hidden, and output layers based on their positions 13 . Typically, the first layer serves as the input layer, the last layer as the output layer, and the intervening layers as hidden layers. Each layer is fully connected, meaning every neuron in a layer is connected to every neuron in the subsequent layer (i.e., layer i is fully connected to layer i + 1). The input layer solely receives external signals without processing, while the hidden and output layers can perform function processing, and the output layer produces the final result. For a fully connected single hidden layer neural network, with the output layer having only one neuron, the output result can be expressed as:
where \(\:g\) represents the activation function of the output layer, \(\:{v}_{k}\) denotes the output weight of the i-th hidden neuron, \(\:\delta\:\) stands for the bias of the output neuron, \(\:{f}_{k}\) is the activation function of the k-th hidden neuron, \(\:{w}_{i}\) shows the output weight of the k-th input neuron and \(\:{\theta\:}_{k}\) is the bias of the k-th hidden neuron.
When utilizing Deep Neural Networks (DNN), careful consideration must be given to the choice of activation function and the configuration of weights and biases. With the advancements in artificial neural networks, a diverse range of activation functions can now be employed in these networks. Activation functions typically produce values from 0 to 1 or -1 to 1, allowing us to select appropriate ones based on specific requirements. For instance, the Tanh function can be suitable for the hidden layer in a binary classification problem, while the Softmax function proves effective for multi-classification tasks. The ReLU function has gained popularity in deep learning due to its ability to avoid the vanishing gradient problem, and the Softplus function, being smoother than ReLU, also finds utility in specific scenarios.
After deciding on the activation function and configuring the network architecture, the next step is determining the optimal weights and biases to achieve the best performance in problem-solving. A loss function is chosen to evaluate the neural network’s performance. Commonly used loss functions include mean square error and cross-entropy error. The neural network is then trained using this loss function, and various training methods can be employed 27 , 28 .
The primary objective during training is to find the proper parameters that minimize the loss function. This optimization process is essentially an optimization problem. A prevalent approach to address this is using gradient or Stochastic Gradient Descent (SGD) strategies. The weights and biases are updated in the negative gradient direction of the objective (loss function) to minimize the loss and improve the network’s performance iteratively. The ultimate goal is to reach a configuration where the neural network performs optimally for the specific task.
K-Nearest Neighbors (KNN) Regression operates based on finding the k nearest neighbors of a given sample and assigning the average value of a specific attribute from these neighbors to the sample 14 , 38 . In contrast to classification, which produces qualitative outputs, regression yields quantitative results. During the training phase of KNN Regression, three crucial factors are considered: the selection of the K value, the method for measuring distances, and the decision-making rules. Decision-making rules primarily come in two forms: the average method and the weighted average method. The step-by-step process of the KNN regression algorithm is presented in Algorithm 2 .
Algorithm 2
KNN Regression.
Step 1. Compute the distance between the point to be predicted and the known points.
Step 2. Sort the known points in ascending order based on their distances from the point to be predicted.
Step 3. Choose the k points with the shortest distances to the predicted point.
Step 4. Return the average or weighted average of the attribute values from the selected k points as the corresponding attribute value for the predicted point.
Results and discussion
Actual data has been collected through investigations to track the inbound and outbound passenger flow in Chengdu subway stations at various time periods. The objective is to aid relevant departments in developing improved plans for constructing new subway stations. To achieve this, it becomes essential to forecast the passenger count entering and exiting the subway stations during different time periods. This prediction can be accomplished by utilizing MLR, DNN, and KNN Regression, which will be employed to fit the data based on the known node indicators and place indicators of the subway stations.
In the context of MLR, we assume the relationship between node indicators, place indicators, and ridership, which is as follows:
The relationship between node indicators, place indicators, and ridership varies due to the differing number of passengers at the same station during various time periods. As a result, the coefficients associated with this relationship differ accordingly.
In the case of DNN utilization, a single hidden layer network is employed to fit the node and place indicators to the ridership data. The input layer comprises 17 neurons, each corresponding to one of the 17 node and place indicators. The hidden layer consists of 10 neurons, and the activation function used for the hidden layer is the ReLU function, as shown in Eq. ( 28 ).
The output layer of the DNN contains a single neuron, representing the ridership, and it uses the LeakyReLU activation function presented in Eq. ( 29 ).
In DNN regression, the loss function employed is the mean square error, and the weights and biases of the DNN are determined through the random gradient descent strategy.
For KNN regression, the Five-Fold Cross-Validation method is utilized. The process involves dividing the training data into five parts, four used as model training sets and the remaining as verification sets. This process is repeated five times to ensure a comprehensive evaluation. The grid search method is then applied to determine the optimal K value, distance measurement method, and decision rules based on the model’s performance on the verification set.
The fitting relationship of different data corresponds to different K values, as depicted in the second column of Table 6 . The distance measurement method is the Manhattan distance, and the decision rule is the average method.
By employing the three methods mentioned above, we have fitted eight sets of data for IT1-IT4 and OT1-OT4. In each case, 10% of the data is reserved as the test set, while 90% is used for training the models. The effectiveness of the models is assessed by calculating the Mean Squared Error (MSE) on the test set. Figure 3 displays the variation of MSE for different models across the eight test sets.
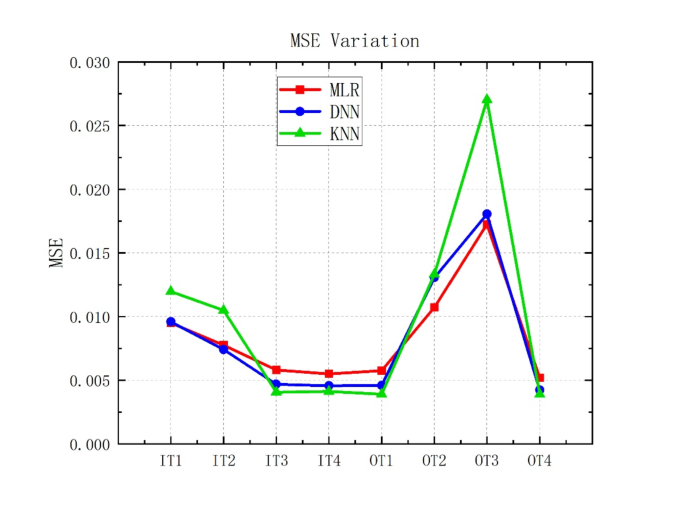
Line plots of MSE variation on the test set under MLR, DNN, and KNN models for each time period (IT1-OT4).
The outcome is not entirely satisfactory, particularly concerning OT3. To gain insights, we observe the data distribution for this specific case. Subsequently, the distance between the independent variables and the dependent variable of the two stations is calculated using the following methods:
After computing the distance mentioned above between each pair of stations, we can visualize the distribution histograms as shown in Fig. 4 .
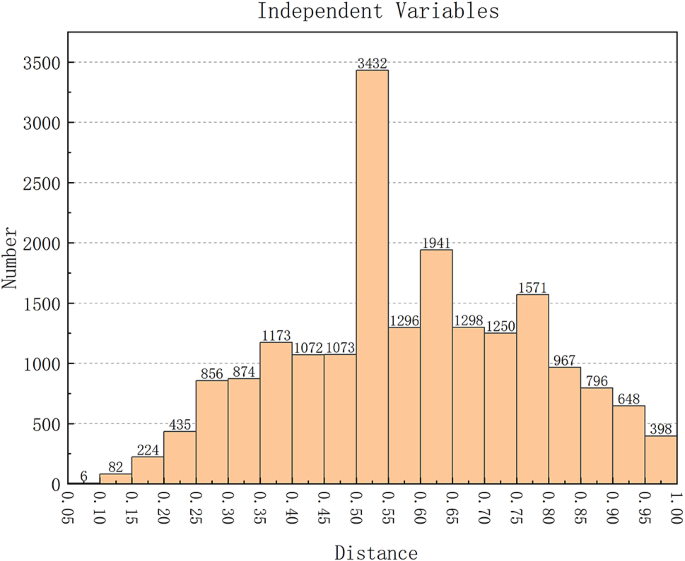
Histogram of the frequency distribution of the distance between the independent variables (N1-N8 and P1-P9).
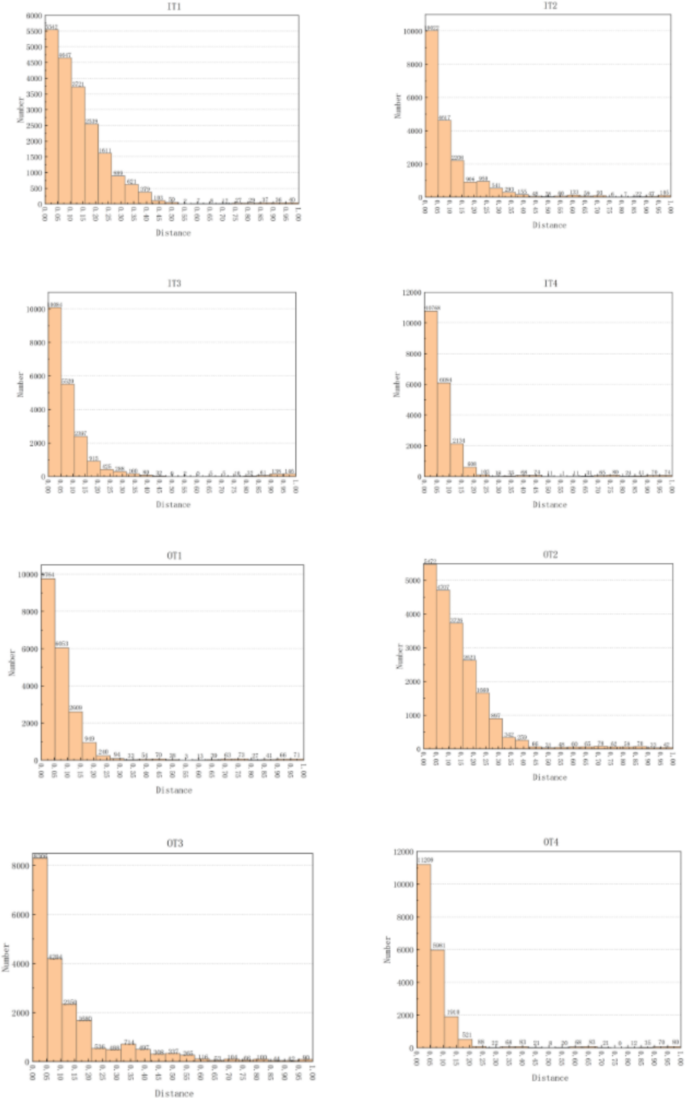
Histogram of the frequency distribution of the distance between the dependent variable (i.e., ridership) at any two stations for different time periods (IT1-OT4).
As shown in Fig. 5 , distribution histograms were drawn to depict the distances between data corresponding to every pair of stations within each time span. The data for each time span were then analyzed using these distribution histograms. For OT3, the distance between the variables is predominantly distributed in the range of [0.50, 0.55]. Additionally, the ridership between stations is generally close, except for a few stations where significant differences in ridership exist. It appears that some stations have similar independent variables but substantially different ridership.
To achieve a more effective fitting outcome, we remove the stations where independent variables are very close, yet ridership differs significantly. This process is repeated for each group, resulting in the number of deleted stations for each group, which is indicated in the third column of Table 6 .
After removing some stations, the data was fitted using MLR, DNN, and KNN. The different K values corresponding to the eight data groups are presented in the fourth column of Table 6 . Figure 6 shows a comparison diagram of MSE on test sets for different models with distinct data. Specifically, DMLR represents the fitting results with MLR after eliminating contradictory stations, while DDNN and DKNN denote the corresponding results for DNN and KNN, respectively.
From the observations in Fig. 6 , it can be inferred that the overall fitting effects of the MLR, DNN, and KNN models are relatively similar. Notably, the performances of all three models on the IT1, OT2, and OT3 test set improved after removing a certain number of contradictory stations, especially for OT3. However, the MSE values for IT2, OT2, and OT3 are relatively higher when compared to the other five groups of data. Upon analyzing the ridership distribution in Fig. 7 for these three groups, it becomes evident that the ridership still deviates from the distribution range of most stations, indicating relatively poor fitting effects for the models.
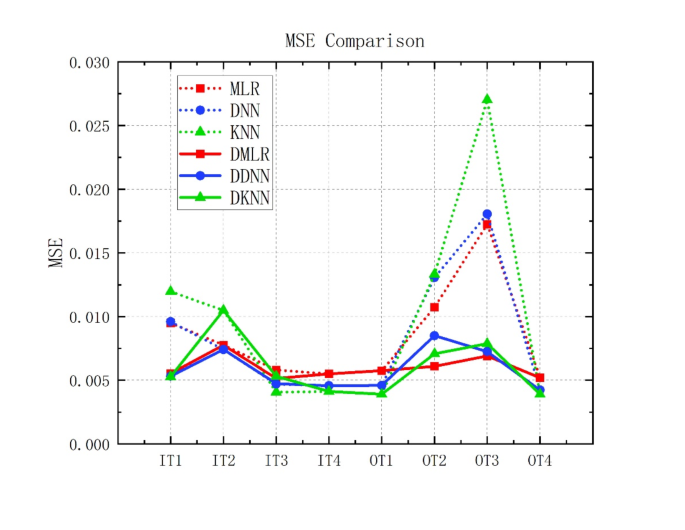
Line plots of MSE changes in the test set for each time period (IT1-OT4) under MLR, DNN, and KNN, as well as the model with some contradictory stations removed (DMLR, DDNN, DKNN).
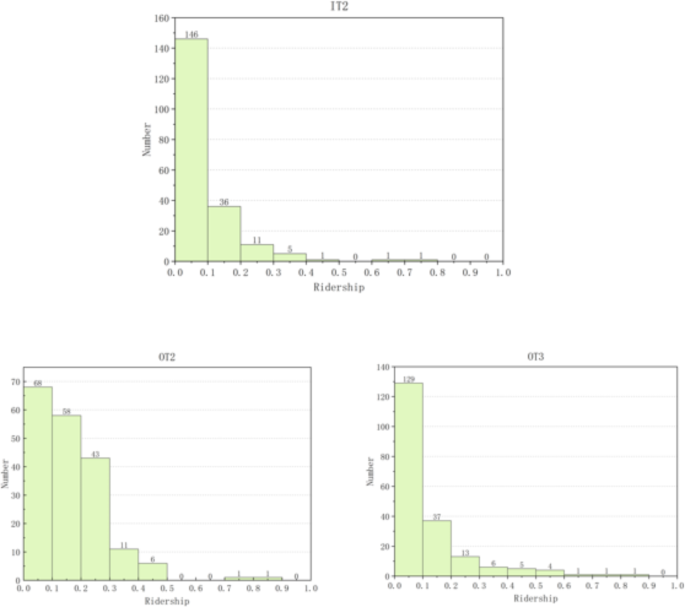
Histogram of the frequency distribution of ridership under IT2, OT2, and OT3 time periods for outliers selection.
Figure 8 displays the point plots of MLR, DNN, and KNN-fitted ridership alongside the actual ridership at selected stations for each time period (IT1-OT4). These plots allow for a comparison between the fitted results and the actual ridership. By observing Fig. 8 , it is evident that the machine learning model is ineffective in predicting stations with many passengers. However, when the number of passengers at a station is small, the model can accurately predict the passenger count. Therefore, deleting the abnormal stations with similar independent variables but significantly higher passenger numbers can further improve the model’s fitting ability. Additionally, the distribution of actual ridership for IT2, OT2, and OT3 remains scattered compared to other time periods, even after removing the abnormal stations. This observation aligns with Fig. 8 and explains why the MSE for these three time periods in Fig. 7 is relatively large.
We obtained promising results on the test set after applying MLR, DNN, and KNN to fit the node indicators, place indicators, and ridership by excluding contradictory stations from the eight data groups. This success allows us to accurately predict station ridership when equipped with the station’s node and place indicators at different times.
However, two approaches can be adopted to enhance ridership predictions’ accuracy. Firstly, we can train the model with more extensive station data. Additional data will help the model capture broader patterns and behaviors, leading to improved predictions. Secondly, investigating other crucial factors that influence ridership is essential. Identifying and incorporating these additional factors into the model can significantly enhance its predictive capabilities and provide more comprehensive insights into the ridership patterns of subway stations.
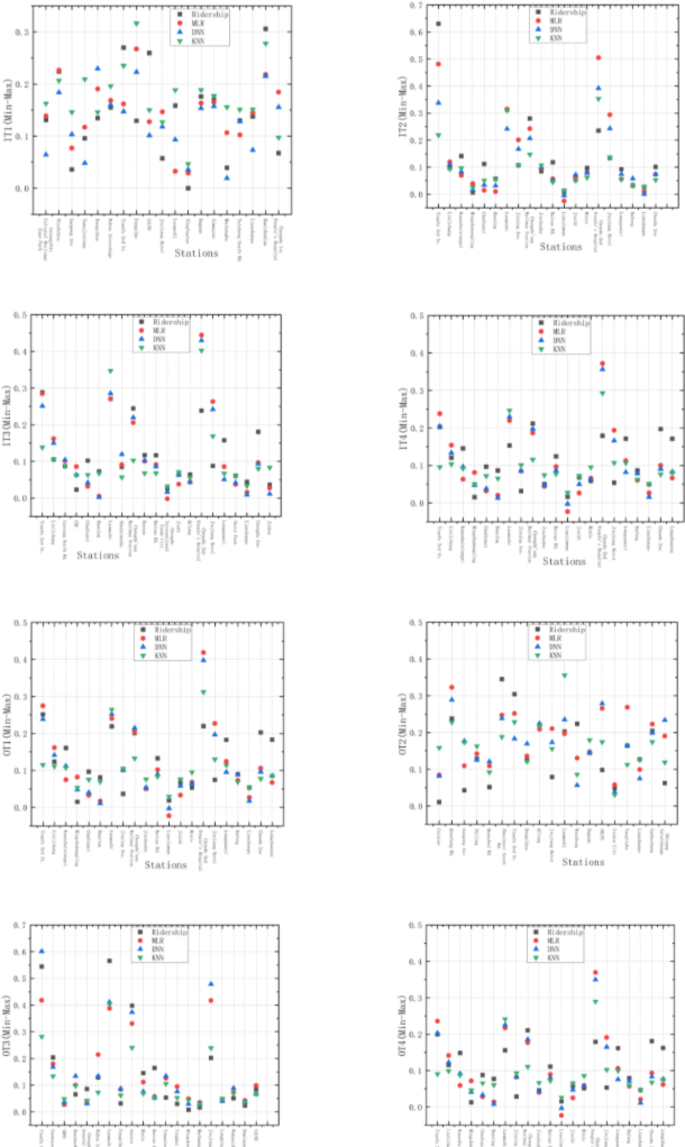
Comparison and analysis of different fitting results.
Model training and selection
Since stations are unlabeled, the categorization process involves clustering in machine learning, an unsupervised learning technique. Different clustering methods operate on different principles, resulting in varied clustering outcomes. To minimize this effect, the results of multiple clustering methods are integrated. A neural network is trained using consistently labeled results, allowing the trained model to classify stations with inconsistent labeling (thus converting it to a supervised learning task) and predict the class of new stations.
To label the data, we separately employ four clustering methods (K-Means, AGNES, DBSCAN, GMM) and conduct a preliminary evaluation of the clustering effectiveness using internal indexes SC and CH. The ridership data is divided into eight groups based on the time span (T), namely IT1-OT4. The process remains the same for each group, and for illustration purposes, we will use the IT1 data as an example.
The methodology section provided a brief description of the clustering algorithm. The principles of the algorithm indicate that each one has hyperparameters that influence the clustering effect, with different parameter choices potentially impacting the results positively or negatively. Based on this, the most satisfactory clustering results and their corresponding parameter values are selected by adjusting the parameters during model training and observing changes in the internal index values.
Python is utilized to cluster the given data. The models can be enhanced by adjusting hyperparameters in the algorithms, adhering to the principles of clustering methods. For K-Means and GMM, we need to adjust the number of clusters (k) as the critical parameter. In the case of AGNES, both an appropriate k-value and a suitable distance definition method (linkages) between clusters must be determined. Regarding DBSCAN, the maximum neighborhood radius (eps) and the minimum number of samples within the domain radius (min_samples) that form the core object must be adjusted for optimum results.
To achieve more satisfactory final classification results, better clustering outcomes for each clustering algorithm, which involves parameter selection, are needed. Larger values of the internal indexes SC and CH indicate better model results. The internal index values for models under different parameter settings are calculated separately to select the best model for each algorithm. Taking IT1 as an example, the results of the four basic clustering algorithms are displayed in Tables 7 , 8 and 9 , and 10 . Based on these results, line graphs, presented in Fig. 9 , are drawn to intuitively identify the optimal parameter for each algorithm. The diagrams in Fig. 9 show the internal index changes corresponding to the four clustering methods during parameter adjustment. The optimal parameters for each algorithm are then selected, and the model is trained using the clustering results under those parameter settings as the subsequent results for that algorithm. For example, with K-Means, the internal indicators SC and CH reach their maximum when k = 3, indicating that clustering subway stations into three categories is most appropriate. For AGNES, although SC is slightly higher at k = 2, CH is significantly higher at k = 3, suggesting that k = 3 should be chosen as the number of clustering categories for AGNES.
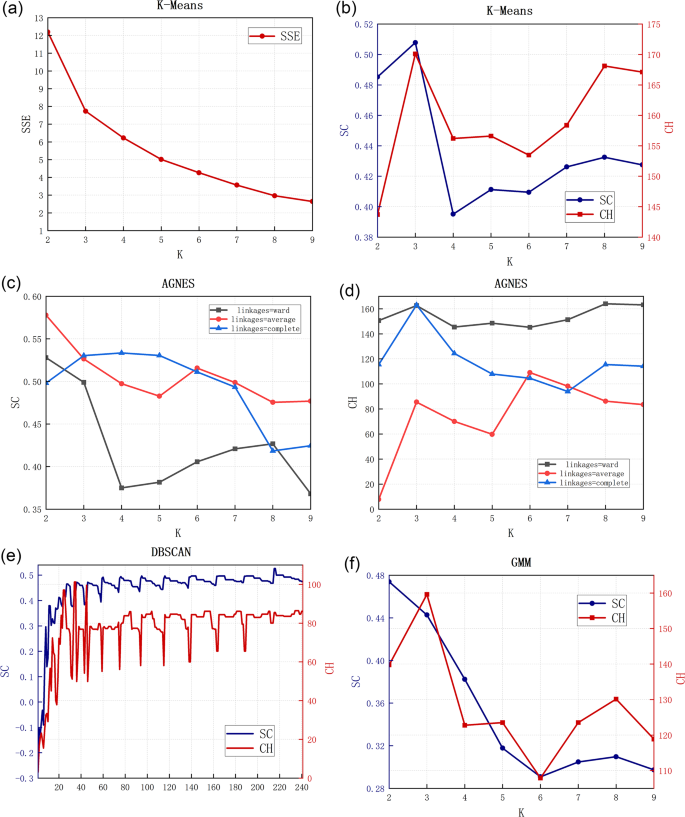
IT1 parameter-based evaluation of clustering methods.
After carefully evaluating the results, we selected four models trained by K-Means (k = 3), AGNES (k = 3, linkages = complete), DBSCAN (eps = 0.32, min_samples = 3, resulting in two clusters), and GMM (k = 3). Using three-dimensional scatter plots, we visually represented the clustering effects of the models under their optimal parameters.
As shown in Table 11 ; Fig. 10 , based on the evaluation index, it is evident that the K-Means model with k = 3 provides the ideal clustering result for the data in the IT1 period. Therefore, this clustering result can be chosen as the label for the data. Figure 10 shows that subway stations are divided into three categories by the K-Means, AGNES, and GMM clustering methods and into two categories by the DBSCAN method. The results from each method have similar N, P, and R values, which verify the rationality of the clustering model and the selection of specific clustering numbers.
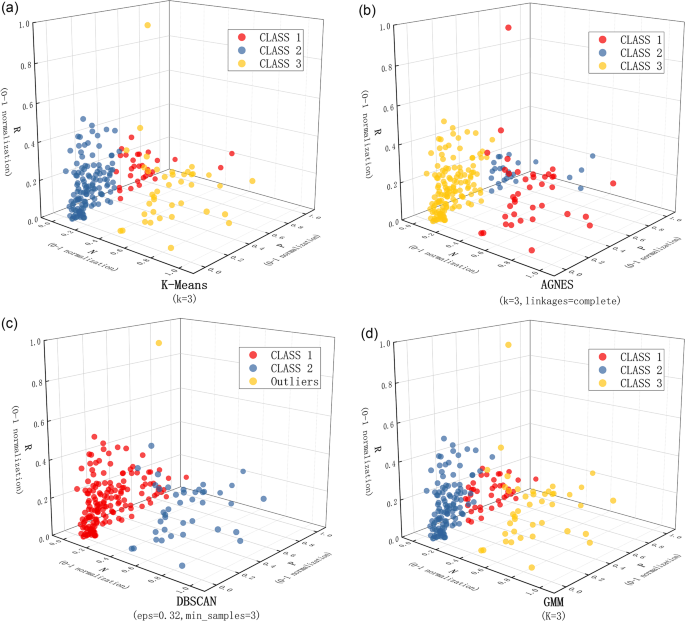
Scatterplots of the IT1 clustering under the optimal models selected by K-Means, AGNES, DBSCAN, GMM.
Enhancements to data labeling
During the model selection process, it is evident that choosing the best-performing algorithm’s result as the data label is a viable approach. However, since different clustering methods divide the data based on distinct principles, they yield varying clustering outcomes. As a result, we can explore methods to combine the results from multiple algorithms. To achieve this, we can first identify samples that consistently belong to the same cluster across all clustering algorithms. These samples can be directly labeled without any modifications. On the other hand, if a data point is assigned to different clusters by different methods, we can temporarily mark it with a distinct symbol and further process it using alternative approaches.
In the provided results, DBSCAN classifies the data into two clusters, while K-Means, AGNES, and GMM classify the data into three clusters. Considering the abovementioned approach, we focus on K-Means, AGNES, and GMM results. Based on this, we label the points that do not consistently fall into the same cluster across these three methods. The figure depicts 25 red points in IT1 with different labels due to the discrepancies in the clustering algorithms. These points are not included in the three clusters formed by the other points. Figure 11 presents the scatter plot of cluster results after crossing. Appendices 1, 2, 3, 4, 5, 6, 7, and 8 illustrate the complete results from IT1 to OT4.
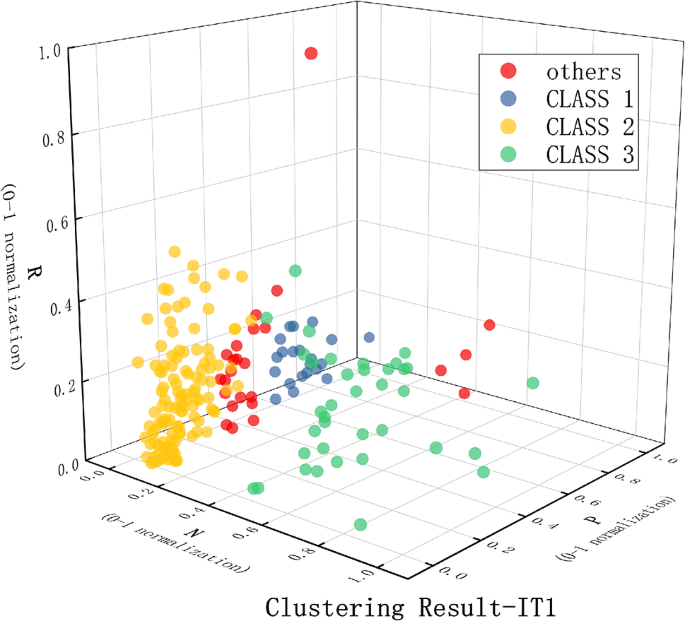
Scatter plot representation of cluster results: post-crossing.
The remaining data points are categorized into three clusters, leaving 25 points without corresponding labels. These points may have suboptimal clustering performance, and their obtained labels may lack strong interpretability. We can employ supervised learning using the accurately labeled data to train a classification model to address this. Subsequently, this model can be used to predict the labels of the unlabeled points, providing them with more accurate labels.
Using the classification algorithm trained on data generated from the results of multiple clustering algorithms, we can group these poorly classified points into three clusters. As a result, all the data points in the dataset will have their respective classes. This approach leads to improved classification results compared to a single algorithm alone. Through this process, we effectively leverage limited data information to enhance the accuracy and interpretability of the labels assigned to these data points.
Classification
In the previous section, data points with consistent labels were clustered, while those with inconsistent labels were set aside. However, further classification methods are necessary to cluster all stations accurately, including those with inconsistent labels. To address this, a Deep Neural Network (DNN) is employed for station classification. The model was trained using consistently labeled data points, with 70% of the data serving as the training set, and its performance was evaluated based on accuracy on the validation set.
The DNN architecture consists of an input layer with 3 neurons to accommodate the 3-dimensional data features, namely N, P, and R. It includes a hidden layer with 10 neurons that utilize the LeakyReLU activation function. The output layer has 3 neurons, each representing one of the three clusters into which the stations were grouped. This layer employs the Softmax activation function for gradient logarithmic normalization. The Cross-Entropy loss function was used during training to optimize the model’s performance.
The weights and biases in the DNN are determined using the stochastic gradient descent strategy, which iteratively updates them based on the data. With iterations set to 10,000, the neural network model is trained under each time period (IT1-OT4) based on the above architecture. Figure 12 visualizes the architecture of the neural network used in this paper.
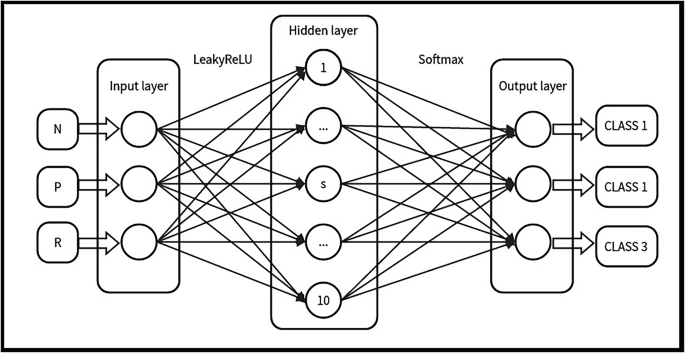
The basic architecture of a neural network for training consistently labeled stations and predicting inconsistently labeled stations.
Table 12 summarizes the accuracy results for eight data groups on the validation set.
The models have shown exceptional performance on the test set, with seven out of the eight groups achieving an impressive 100% accuracy, except for OT3. This demonstrates the model’s excellent training and reaffirms the effectiveness of the clustering methodology employed in the previous section.
Given this success, the model can accurately classify new stations when provided with relevant information. The trained model can also classify unlabeled sites and compare the results with various clustering methods. This comparative analysis will offer valuable insights into the model’s superior performance and advantages over alternative clustering approaches.
Table 13 presents the final cluster results for 30 randomly selected stations for different time periods. These classifiers were trained using the DNN on test sets, ensuring robust evaluation across various time spans. The DNN model demonstrates its ability to make accurate predictions for the selected stations, providing valuable insights into the performance of the classifiers over different periods. This data allows for assessing the model’s effectiveness in handling temporal variations and offers crucial information for understanding the overall stability and reliability of the predictions.
The stations were clustered using each of the three clustering methods. A neural network was then used to re-classify the data points labeled inconsistently under the three algorithms, ensuring that all subway stations had a cluster to which they belonged. This final clustering result is believed to combine some of the clustering results of the three algorithms, especially for the inconsistently labeled data.
Different clustering algorithms produce different results based on their principles. However, it is unclear which set of clustering results is superior. The intersection of the clustering results of the three algorithms was taken to leverage all the clustering results. Data points in the same cluster under all three algorithms were clustered together. Data points that did not fit into the same cluster were considered to be placed into different clusters by algorithms based on different principles.
To address this, a neural network was trained with consistently labeled points. This involved training the model with more accurately clustered points to predict the clusters of those currently unlabeled points. This approach maximized the accuracy of station prediction by using the clustering results of the three algorithms.
Verification of clustering results
As the initial dataset lacks labels, we are limited to evaluating the cluster performance using internal rather than external indexes. However, by applying the neural network, we could label each point in the dataset, reasonably assuming that these labels are relatively accurate. Leveraging this labeled dataset as a reference model, we can now employ external indexes such as JC (Jaccard Coefficient) and ARI (Adjusted Rand Index) to assess the performance of each clustering algorithm in reverse.
Using the results obtained from the DNN classifier, we calculate the external indexes for the three clustering methods, as presented in Table 14 . This approach allows us to verify and compare the performance of each algorithm based on the reference labels generated by the neural network.
All of the external index values for the clustering results are relatively large, indicating a difference, but not a significant one, between the final clustering results and those of a single algorithm. The Jaccard coefficient (JC) and Adjusted Rand Index (ARI) show that K-Means’ results are superior, suggesting that K-Means substantially influences the final clustering results.
Table 14 shows that all three clustering algorithms significantly influence the final clustering results. Most data is consistently labeled under all three algorithms, and the DNN prediction utilizes this information. Consequently, the predicted results are partially similar to the clustering results of the individual algorithms. This further confirms the validity and accuracy of our station classification approach.
A portion of the consistently labeled data was selected as a validation set. Since this data has corresponding classes, its results can be used to test the effectiveness of the DNN. For the inconsistently labeled stations, they are used as a test set to predict the classes using the trained neural network. However, since clustering results from three different algorithms are available, the predicted results can be compared against them. The results in Table 14 show that the difference between the predicted and actual clustering results is minimal, verifying the feasibility of the predictions to some extent.
Additionally, the DNN model trained earlier can be used to predict classes for new unclassified stations, ensuring the model’s usability. The DNN model can also be updated as station data is continuously updated to achieve better results. Scaling up the training data by constantly adding new inputs improves the neural network’s predictive power, enabling it to better predict the classes of new stations and adapt to new changes and trends.
The results of this study have a great deal of meaning for urban planners and policymakers, especially in the case of TOD. The novel classification model explained not only enhances the precision of station classification but also gives implementable ideas that may support more efficient and environmentally friendly urban planning.
With the help of the reborn version of the classification model, town planners, and policyholders can now make informed decisions about new station planning and its improvement. By looking at the unique combinations of property values and the on-time performance for the various station types, the most effective recommendations can be made to be more efficient in land use and the area’s transit. The station pair result directs highlights of a set of stations that possess analogous features. Thus, roads and sidewalks, bus stops, and cycle lanes can be constructed whenever necessary. Train stations must be invested only when high in demand rather than just building them all over the city. This step implies the creation of the future city, where technology and GI (geographic information) are used to improve the quality of life of residents, businesses, and the natural environment with less car dependency.
The authorities must apply adaptive TOD strategies that consider the transition of urban growth and transit usage patterns. The estimates of the model can be used to forecast future ridership trends that will enable the change of TOD plans to accommodate the volatile downtown urban structure. If the classification model with the expanded sustainability schemes is combined, then the Sustainable Development Goals (SDGs) will be supported. By concentrating on stations that help use public transport and, on the other hand, do not force people to possess personal vehicles, TOD strategies could lessen urban congestion and carbon emissions.
Another necessary part of community engagement is that local authorities, along with urban designers, can get the community to participate in their projects with the aid of the sorting model. Urban planners can analyze information from the sorting model to interact with local societies efficiently. Planners can get the public’s opinion by providing precise and data-supported information about the advantages and future constructions around traffic stations. In addition, they can ensure that the TOD projects align with peoples’ needs and preferences.
The main concern is setting up a system for continuously monitoring and examining rail facilities using the classification model. Routine appraisals may help to unearth the new patterns and potential mishaps earlier, which could provide better interventions to keep the efficiency and effectiveness of TOD strategies at the desired level. By encouraging other cities and areas to adopt the classification model, the model will be improved and made accessible to other cities and areas. With the help of the best practices and outcomes from the Chengdu case study that can serve as a model for the same urban environment, promoting a uniform mode of transit station classification and TOD planning would be encouraged.
Urban policies and rules are set to blend and synchronize various urban developments on different scales by transcoding the data from the robot into the already existing urban planning paperwork. The decomplication of these documents can facilitate clarity when trying to find a way to arrange various instruments to achieve sustainable development. Toward this end, urban practitioners working in conjunction with local government leaders and land use stakeholders need to realize that before entering its practical application, the application of the input code is put through deformation, noise addition, erasing some parts, and other special operations. Followed by the deployment of these core measures, by the planners’ and politicians’ willingness, the classification model is being led to its full potential, which leads to the pulling up of the already inefficient process and the creation of a new and streamlined urban growth utilizing applied Transit-Oriented Development strategy. Global warming and environmental degradation are major issues that must be addressed immediately to prevent the problem from worsening. The necessary proteins are injected into the programming code to produce options for the local government to develop.
According to Table 13 , the classification results obtained from the DNN model provide a comprehensive understanding of the ridership patterns across rail transit stations during different time periods. These classifications have significant implications for TOD policymaking and planning, offering valuable insights into how temporal variations in passenger traffic can influence various stages of urban transit planning. The model helps assess the effectiveness of transit infrastructure and services, providing planners with data-driven approaches to improve the efficiency and sustainability of urban transit systems.
One of the key applications of this classification is in demand management and capacity planning. For example, stations like North Railway Station and Renmin Rd. North exhibit consistently high traffic (classification 3) across all time periods (IT1 to OT4). This suggests that these stations require additional capacity during peak and off-peak hours. The classification data can guide transportation planners in making decisions such as adjusting the frequency of trains, redesigning station layouts to accommodate higher volumes of passengers, and adding amenities to meet increased demand. The insights gained from these classifications allow for better resource allocation, helping to prevent over-crowding at crucial transit hubs and ensuring that stations are equipped to handle peak demand efficiently.
Additionally, the classification results are instrumental in peak-hour optimization. Stations such as Tianfu Square and Sichuan Gymnasium show high ridership during working hours but a decline in traffic during off-peak or weekend times (OT3, IT4). This pattern indicates the need for peak-hour optimization strategies, such as increasing service frequency during high-demand periods or improving station accessibility to better handle the flow of passengers during peak times. During off-peak and weekend hours, TOD strategies might consider repurposing station spaces for other uses, such as hosting community events or establishing retail pop-ups, which would keep the area vibrant even when ridership decreases.
The classification data also offers insights into infrastructure investments and upgrades. For instance, stations such as Wenshu Monastery and Jinjiang Hotel consistently show low traffic (classification 1) across all time periods, indicating that these stations experience low passenger volumes even during peak hours. This information is valuable for urban planners when considering prioritizing infrastructure investments. Instead of focusing significant resources on low-traffic stations, planners might decide to allocate more funds to high-demand stations, while low-traffic stations could be maintained with lighter service routes or alternative modes of transportation such as biking or walking. Furthermore, these low-traffic stations could be targeted with TOD policies to increase ridership through development incentives, such as constructing residential or commercial properties nearby to draw more passengers.
The classification system also provides a framework for service flexibility and scheduling. For example, the Hi-Tech Zone shows varying ridership patterns, with low outbound traffic during off-peak hours (OT2) but higher outbound ridership during working hours (OT1). This variation allows transportation planners to adjust transit schedules based on real-time data, reducing operational costs during off-peak hours while maintaining rider satisfaction during periods of high demand. Flexible scheduling, such as reduced service during off-peak times and increased frequency during peak hours, can improve the efficiency of transit systems and better match passenger needs without overburdening the network.
Another critical application of these classifications is in weekend transit planning. Stations like Tongzilin and Financial City experience notable differences between weekday and weekend ridership patterns, with lower inbound traffic on weekends (IT4 = 1) compared to higher weekday traffic. Understanding these differences helps planners optimize weekend service routes by reducing service frequency or developing special routes catering to weekend commuters and leisure travelers. This flexibility ensures that resources are allocated efficiently, with transit services tailored to match the specific demands of each station during different time periods.
The classification data is also essential for supporting mixed-use development in high-traffic areas. Stations like South Railway Station show consistently high ridership across all time periods (classification 3 for IT1 to OT4). Such stations serve as key commuter hubs and prime locations for mixed-use development, combining residential, commercial, and recreational spaces. TOD strategies could promote the development of high-traffic areas into vibrant, multi-use communities, fostering economic growth while supporting commuters’ daily needs. TOD policies can encourage private investment in real estate, retail, and entertainment in these areas, ensuring that the station is a focal point for residents and visitors.
The classification system also highlights opportunities for public-private partnerships. Stations like Century City exhibit consistent high traffic across all time periods (classification 3), indicating their importance as key transit hubs. These high-traffic stations are ideal candidates for public-private partnerships (PPP) that could promote commercial ventures or real estate projects, leveraging the high footfall to attract private investment. By working with private stakeholders, TOD strategies can integrate retail spaces, office buildings, and residential complexes into the transit network, maximizing these high-demand stations’ economic and social benefits.
Finally, these classifications contribute to the development of sustainability and environmental policies. Stations with moderate but consistent traffic, such as Weijianian and Shengxian Lake (classification 2 across all conditions), can be focal points for eco-friendly initiatives. By understanding which stations experience moderate traffic, planners can design policies to reduce the carbon footprint of transit systems. These stations may be integrated with pedestrian-friendly zones, bicycle lanes, or green spaces to encourage environmentally sustainable transportation options. Furthermore, TOD policies can promote using renewable energy sources and energy-efficient technologies in transit stations, contributing to broader sustainability goals while ensuring that these stations continue to serve the community’s needs.
According to the mentioned strategies and examples, classifying passenger traffic into distinct categories based on time periods and traffic types provides valuable insights into various aspects of TOD planning. These classifications help address challenges in demand management, peak-hour optimization, infrastructure investments, service flexibility, and sustainability. By leveraging this data, urban planners can create more efficient, resilient, and sustainable transit systems that cater to the evolving needs of commuters and align with long-term urban development goals.
Classifying rail transit stations is crucial for shaping Transit-Oriented Development (TOD) and fostering sustainable urban growth. This paper presented a pioneering classification model that seamlessly blends traditional methodologies with state-of-the-art machine learning (ML) algorithms to analyze comprehensively and cluster rail transit stations. By incorporating mathematical models and supervised learning techniques, the model achieves a refined evaluation of station attributes, thereby enhancing the precision of classification techniques in TOD. This holistic approach enables rigorous station performance analysis and facilitates effective planning that aligns with the principles of TOD.
Several results stemming from this study include:
MLR, DNN, and KNN regression models were trained using the existing data, and the resulting fitting outcomes can be observed in Fig. 3 . Some data clusters display slight generality, prompting the construction of a distribution map illustrating variable distances. Consequently, contradictory points in the data—where independent variables exhibit close distances, yet corresponding dependent variables are significantly distant—were identified and removed. Following the removal process, the three methods mentioned above were applied to fit the data, and the corresponding results are displayed in Fig. 6 . Notably, the Mean Squared Error (MSE) for each time interval’s data fitting remains below 0.012. This result is satisfactory in comparison to the situation before anomalous point deletion. As a result, accurate ridership forecasts can be generated independently using these well-trained models.
K-Means, AGNES, DBSCAN, and GMM algorithms were employed for data clustering. Except for DBSCAN, all algorithms grouped the stations into three distinct categories. Evaluation using internal indices is presented in Table 11 . The outcomes indicate that, in terms of the Silhouette Coefficient, the ranking is AGNES > DBSCAN > K-Means > GMM, while for the CH score, the order is K-Means > AGNES > GMM > DBSCAN.
Distinct algorithms yield varying clustering outcomes. Consequently, we cross-reference the K-Means, AGNES, and GMM results to categorize points exhibiting consistent clustering patterns. Points with incongruent clustering outcomes are temporarily unlabeled. Refer to Table 12 for the outcomes of the neural network trained on labeled data. Among the neural networks corresponding to the eight temporal spans, only OT3 attains an accuracy of 98.15%. The remaining networks achieve 100% accuracy, indicating satisfactory performance on the test sets. Thus, we employ this neural network to predict the classification of unlabeled data and assign labels to all instances.
After labeling the data, the clustering effectiveness is assessed by computing the external indices ARI and JC. Refer to Table 14 for the outcomes. Notably, for both external indices, K-Means > AGNES > GMM. This alignment between the site classification predictions from the neural network and the clustering algorithms highlights the method’s rationality and accuracy from an alternative perspective.
Possible directions for future studies
Several intriguing avenues for future research can be explored to enhance further the accuracy and depth of rail transit station classification within the realm of Transit-Oriented Development (TOD).
One promising direction involves the integration of Partial Differential Equations (PDE) or other advanced mathematical models to capture the intricate spatial and temporal dynamics of station performance, enabling a more comprehensive understanding of the underlying factors influencing TOD outcomes. Additionally, a fruitful path for future investigation lies in harnessing the power of deep learning techniques, such as Convolutional Neural Networks (CNNs) or Recurrent Neural Networks (RNNs), to extract nuanced patterns and correlations from complex station data, potentially uncovering hidden insights that contribute to more refined classification and planning strategies. Furthermore, an interdisciplinary approach that fuses transportation engineering, urban planning, and environmental science could yield a holistic framework for station classification, considering factors like environmental impact, accessibility, and social equity in conjunction with performance metrics. Lastly, exploring the potential integration of real-time data streams, such as crowd flow patterns and energy consumption, could provide a dynamic dimension to rail transit station classification, enabling adaptive and responsive TOD strategies that evolve with changing urban dynamics.
Exploring new research areas can enhance our knowledge of the diverse characteristics of rail transit stations and support the ongoing development of successful and sustainable TOD approaches.
Data availability
The datasets used and/or analyzed during the current study are available from the corresponding author upon reasonable request.
Abbreviations
Agglomerative Nesting
Artificial Neural Network
Adjusted Rand Index
Calinski-Harabasz Index
Density-Based Spatial Clustering of Applications with Noise
DNN after deleting outliers
KNN after deleting outliers
MLR after deleting outliers
Deep-Learning Neural Network
Gaussian Mixture Model
Information entropy weighting
Jaccard Coefficient
K-means clustering
K-nearest Neighbor
Machine Learning
Multiple Linear Regression
Mean of Squared Errors
Place value
Ridership value
Silhouette Coefficient
Sum of Squared Errors
- Transit Oriented Development
Bojing Liao, Pauline, E. W., van den Berg, P. J. V., van Wesemael, T. A. & Arentze Empirical analysis of walkability using data from the Netherlands. Transp. Res. Part D . 85 , 102390. https://doi.org/10.1016/j.trd.2020.102390 (2020).
Article Google Scholar
Shiliang Su, H., Zhang, M., Wang, M., Weng, M. & Kang Transit-oriented development (TOD) typologies around metro station areas in urban China: a comparative analysis of five typical megacities for planning implications. J. Transp. Geogr. 90 , 102939. https://doi.org/10.1016/j.jtrangeo.2020.102939 (2021).
Hongzan, J., Huang, S. & Zhou, Y. Understanding the land use function of station areas based on spatiotemporal similarity in rail transit ridership: a case study in Shanghai, China. J. Transp. Geogr. Volume . 109 , 103568. https://doi.org/10.1016/j.jtrangeo.2023.103568 (May 2023).
Mohiuddin Ahmed, R. & Seraj Syed Mohammed Shamsul Islam. The k-means Algorithm: A Comprehensive Survey and Performance Evaluation. Electronics 2020, 9, 1295; https://doi.org/10.3390/electronics9081295
Ningning Dong, T. et al. A method for short-term passenger flow prediction in urban rail transit based on deep learning. Multimedia Tools Appl. 83 , 61621–61643. https://doi.org/10.1007/s11042-023-14388-z (2024).
Chaojun Dong, Y., Su & Xiankun Liu. Research on TOD Based on Isomap and K-means Clustering Algorithm. 2009 Sixth International Conference on Fuzzy Systems and Knowledge Discovery. https://doi.org/10.1109/FSKD.2009.788
Niu, S. et al. Measuring the built environment of green transit-oriented development: a factor-cluster analysis of rail station areas in Singapore. Front. Architectural Res. , https://doi.org/10.1016/j.foar.2021.03.005
Fionn Murtagh, P. & Contreras Algorithms for hierarchical clustering: an overview. WIREs Data Min. Knowl. Discov . https://doi.org/10.1002/widm.53 (2011).
Qiang, D., Zhang, L. & Huang, X. Quantitative evaluation of TOD Performance based on Multi-source Data: a case study of Shanghai. Front. Public. Health . 10 , 820694. https://doi.org/10.3389/fpubh.2022.820694 (2022).
Article PubMed PubMed Central Google Scholar
Schubert, E., Sander, J., Ester, M., Kriegel, H. P. & Xu, X. DBSCAN Revisited, Revisited: why and how you should (still) use DBSCAN. ACM Trans. Database Syst. 42 https://doi.org/10.1145/3068335 (2017). Article 19 (July 2017),.
Yibin Ren, G., Chen, Y., Han, H. & Zheng Extracting potential bus lines of Customized City Bus Service based on public transport big data. IOP Conf. Series: Earth Environ. Sci. 46 , 012017. https://doi.org/10.1088/1755-1315/46/1/012017 (2016).
Zhenyu Mei, J., Gong, C., Feng, L., Kong, Z. & Zhu Assessment of carbon emissions from TOD subway first/last mile trips based on level classification. Travel Behav. Soc. 36 , 100792. https://doi.org/10.1016/j.tbs.2024.100792 (2024).
Abiodun, O. I., Jantan, A. & Omolara, A. E. Kemi Victoria Dada, Nachaat AbdElatif Mohamed, Humaira Arshad. State-of-theart in artificial neural network applications: a survey. Heliyon . 4 , e00938. https://doi.org/10.1016/j.heliyon.2018.e00938 (2018).
AlKhereibi, A. H., Wakjira, T. G., Kucukvar, M. & Onat, N. C. Predictive Machine Learning Algorithms for Metro Ridership based on Urban Land Use policies in support of transit-oriented development. Sustainability . 15 , 1718. https://doi.org/10.3390/su15021718 (2023).
Shiliang Su, C., Zhao, H., Zhou, B., Li, M. & Kang Unraveling the relative contribution of TOD structural factors to Metro ridership: a novel localized modeling approach with implications on spatial planning. J. Transp. Geogr. 100 , 103308. https://doi.org/10.1016/j.jtrangeo.2022.103308 (2022).
Pishro, A. A. et al. Lili Zhang. The Integrated ANN-NPRT-HUB Algorithm for Rail-Transit Networks of Smart Cities: A TOD Case Study in Chengdu. Buildings 13, 1944. (2023). https://doi.org/10.3390/buildings13081944
Pishro, A. A. et al. WeiYu Li; Node, Place, Ridership, and Time Model for Rail-Transit Stations: A Case Study 1216120 (Scientific Report, 2022). https://doi.org/10.1038/s41598-022-20209-4
Zhejing Cao, Y. & Asakura, Z. Coordination between node, place, and ridership: comparing three transit operators in Tokyo. Transp. Res. Part D . 87 , 102518. https://doi.org/10.1016/j.trd.2020.102518 (2020).
Zemp, S., Stauffacher, M., Lang, D. J. & Scholz, R. W. Classifying railway stations for strategic transport and land use planning: Context matters! Journal of Transport Geography 19 670–679. https://doi.org/10. 1016/j. jtran geo. 2010. 08. 008 (2011).
Chorus, P. & Bertolini, L. An application of the node place model to explore the spatial development dynamics of station areas in Tokyo. J. Transp. Land. Use . 4 (1), 45–58. https://doi.org/10.5198/jtlu.v4i1.145 (2011).
Shaofei Niu, A., Shen, H. Z., Huang, Y. & Mou, Y. Measuring the built environment of green transit-oriented development: a factor-cluster analysis of rail station areas in Singapore. Front. Architectural Res. 10 , 652e668. https://doi.org/10.1016/j.foar.2021.03.005 (2021).
Weiye, X. Yehua Dennis Wei. Assess the non-linear relationship between built environment and active travel around light-rail transit stations. Appl. Geogr. Volume . 151 , 102862. https://doi.org/10.1016/j.apgeog.2022.102862 (2023).
Yu, Z., Zhu, X. & Liu, X. Characterizing metro stations via urban function: thematic evidence from transit-oriented development (TOD) in Hong Kong. J. Transp. Geogr. Volume . 99 , 103299 (2022).
Pishro, A. A. et al. & Qihong Yang; Application of Artificial Neural Networks and Multiple Linear Regression on Local Bond Stress Equation of UHPC and Reinforcing Steel Bars; Scientific Reports; 11:15061 ; : 2045–2322; (2021). https://doi.org/10.1038/s41598-021-94480-2
Pishro, A. A. et al. Comprehensive Equation of Local Bond Stress Between UHPC and Reinforcing Steel Bars; Construction and Building Materials; 262 119942; ISSN: 0950 – 0618 ; (2020). https://doi.org/10.1016/j.conbuildmat.2020.119942
Pishro, A. A. et al. Yuandi Zhao; advancing ultimate bond stress–slip model of UHPC structures through a novel hybrid machine learning approach. Structures . 62 , 106162. https://doi.org/10.1016/j.istruc.2024.106162 (2024).
Pishro, A. A. et al. Siti Jahara Matlan; UHPC-PINN-Parallel Micro element system for the local bond stress–slip model subjected to monotonic loading. Structures . 46 , 570–597. https://doi.org/10.1016/j.istruc.2022.10.053 (2022).
Pishro, A. A. et al. Qihong Yang; Structural Performance of EB-FRP-Strengthened RC T-Beams Subjected to Combined Torsion and Shear 15(14), 4852 ; (2022). https://doi.org/10.3390/ma15144852
Pishro, A. A. et al. Victor Postel; structural behavior of FRP-Retrofitted RC beams under combined torsion and bending. Materials . 15 , 3213. https://doi.org/10.3390/ma15093213 (2022).
Article ADS Google Scholar
Jingru Huang, S., Chen, Q., Xu, Y., Chen, J. & Hu Relationship between built environment characteristics of TOD and subway ridership: a causal inference and regression analysis of the Beijing subway. J. Rail Transp. Plann. Manage. 24 , 100341. https://doi.org/10.1016/j.jrtpm.2022.100341 (2022).
Liu Yang, X. & Song TOD Typology based on Urban Renewal: a classification of Metro Stations for Ningbo City. Urban Rail Transit. 7 (3), 240–255. https://doi.org/10.1007/s40864-021-00153-8 (2021).
Article PubMed Google Scholar
Papa, E., Carpentieri, G. & Angiello, G. A TOD classification of Metro stations: an application in Naples. In: (eds Papa, R., Fistola, R. & Gargiulo, C.) Smart Planning: Sustainability and Mobility in the Age of Change. Green Energy and Technology. Springer, Cham. https://doi.org/10.1007/978-3-319-77682-8_17 (2018).
Chapter Google Scholar
Shiliang Su, Z., Wang, B., Li, M. & Kang Deciphering the influence of TOD on Metro ridership: an integrated approach of extended node-place model and interpretable machine learning with planning implications. J. Transp. Geogr. Volume . 104 , 103455. https://doi.org/10.1016/j.jtrangeo.2022.103455 (2022).
Xin Yang, Q., Xue, M., Ding, J., Wu, Z. & Gao Short-term prediction of passenger volume for urban rail systems: a deep learning approach based on smart-card data. Int. J. Prod. Econ. Volume . 231 , 107920. https://doi.org/10.1016/j.ijpe.2020.107920 (January 2021).
Xin Yang, Q. et al. A novel prediction model for the inbound passenger flow of urban rail transit. Inf. Sci. 566 , 347–363. https://doi.org/10.1016/j.ins.2021.02.036 (2021).
Article MathSciNet Google Scholar
Zhang, J., Che, H., Chen, F., Ma, W. & He, Z. Short-term origin-destination demand prediction in urban rail transit systems: a channel-wise attentive split-convolutional neural network method. Transp. Res. Part C . 124 , 102928. https://doi.org/10.1016/j.trc.2020.102928 (2021).
Chunyan Shuai, J. et al. Relationship analysis of short-term origin–destination prediction performance and spatiotemporal characteristics in urban rail transit. Transp. Res. Part. A: Policy Pract. Volume . 164 , 206–223. https://doi.org/10.1016/j.tra.2022.08.006 (October 2022).
Zhang, M. L. & Zhi-Hua Zhou ML-KNN:Alazy learning approach to multi-label learning. Pattern Recogn. 40 , 2038–2048. https://doi.org/10.1016/j.patcog.2006.12.019 (2007).
Download references
Acknowledgements
Acknowledgments: The authors acknowledge the Sichuan University of Science and Engineering (SUSE), Sichuan University, Université Gustave Eiffel, University of the West of England (UWE Bristol), Staffordshire University London, University of Leeds, and Université de Lille.
This research was supported by the following: (1) Science and Technology Department of Sichuan Province (2022YFWZ0010). (2) Bridge Non-destructive Testing (NDT) and Engineering Computation Sichuan Provincial University Key Laboratory (2022QYY01). (3) The Key Laboratory of Colleges and Universities in Sichuan Province (2020QYJ01).
Author information
Authors and affiliations.
School of Civil Engineering, Sichuan University of Science and Engineering, Zigong, 643000, China
Ahad Amini Pishro & Zhengrui Zhang
School of Mathematics, Sichuan University, Chengdu, 610065, China
Shiquan Zhang, Yuetong Liu & Qixiao Hu
Univ. Gustave Eiffel, Ecole des Ponts, LVMT, Marne-la-Vallée, 77454, France
Ahad Amini Pishro & Alain L’Hostis
School of Environment and Technology, University of the West of England, Bristol, BS16 1QY, UK
Farzad Hejazi
Staffordshire University London, Queen Elizabeth Olympic Park, Here East, London, E20 3BS, UK
Maryam Shahpasand
School of Civil Engineering, Faculty of Engineering and Physical Sciences, University of Leeds, Leeds, UK
Univ. Lille, CNRS, Centrale Lille, UMR 9013 - LaMcube - Laboratoire de mécanique, multiphysique, multiéchelle, Lille, 59000, France
Abdelbacet Oueslati
You can also search for this author in PubMed Google Scholar
Contributions
Author Contributions: Conceptualization, Ahad Amini Pishro, Shiquan Zhang, Alain L’Hostis; Data curation, Ahad Amini Pishro, Qixiao Hu, Yuetong Liu; Formal analysis, Ahad Amini Pishro, Shiquan Zhang, Qixiao Hu, Yuetong Liu, Farzad Hejazi, Maryam Shahpasand; Funding acquisition, Ahad Amini Pishro; Investigation, Ahad Amini Pishro, Shiquan Zhang; Methodology, Ahad Amini Pishro, Shiquan Zhang, Alain L’Hostis, Maryam Shahpasand; Resources, Ahad Amini Pishro; Software, Qixiao Hu, Yuetong Liu, Farzad Hejazi; Supervision, Ahad Amini Pishro, Shiquan Zhang, Alain L’Hostis, Farzad Hejazi, Maryam Shahpasand; Validation, Ahad Amini Pishro, Shiquan Zhang, Alain L’Hostis, Farzad Hejazi, Maryam Shahpasand, Ali Rahman, Abdelbacet Oueslati, Zhengrui Zhang; Visualization, Qixiao Hu, Yuetong Liu; Writing – original draft, Ahad Amini Pishro, Qixiao Hu, Yuetong Liu; Writing – review & editing, Ahad Amini Pishro, Shiquan Zhang, Alain L’Hostis, Farzad Hejazi, Maryam Shahpasand, Ali Rahman, Abdelbacet Oueslati, Zhengrui Zhang.
Corresponding author
Correspondence to Yuetong Liu .
Ethics declarations
Competing interests.
The authors declare no competing interests.
Additional information
Publisher’s note.
Springer Nature remains neutral with regard to jurisdictional claims in published maps and institutional affiliations.
Supplementary Information
Supplementary information., rights and permissions.
Open Access This article is licensed under a Creative Commons Attribution-NonCommercial-NoDerivatives 4.0 International License, which permits any non-commercial use, sharing, distribution and reproduction in any medium or format, as long as you give appropriate credit to the original author(s) and the source, provide a link to the Creative Commons licence, and indicate if you modified the licensed material. You do not have permission under this licence to share adapted material derived from this article or parts of it. The images or other third party material in this article are included in the article’s Creative Commons licence, unless indicated otherwise in a credit line to the material. If material is not included in the article’s Creative Commons licence and your intended use is not permitted by statutory regulation or exceeds the permitted use, you will need to obtain permission directly from the copyright holder. To view a copy of this licence, visit http://creativecommons.org/licenses/by-nc-nd/4.0/ .
Reprints and permissions
About this article
Cite this article.
Amini Pishro, A., Zhang, S., L’Hostis, A. et al. Machine learning-aided hybrid technique for dynamics of rail transit stations classification: a case study. Sci Rep 14 , 23929 (2024). https://doi.org/10.1038/s41598-024-75541-8
Download citation
Received : 24 May 2024
Accepted : 07 October 2024
Published : 13 October 2024
DOI : https://doi.org/10.1038/s41598-024-75541-8
Share this article
Anyone you share the following link with will be able to read this content:
Sorry, a shareable link is not currently available for this article.
Provided by the Springer Nature SharedIt content-sharing initiative
- Rail Transit Station Classification
- Machine Learning algorithms
- Clustering methods
- Regression models
Quick links
- Explore articles by subject
- Guide to authors
- Editorial policies
Sign up for the Nature Briefing: AI and Robotics newsletter — what matters in AI and robotics research, free to your inbox weekly.

Information
- Author Services
Initiatives
You are accessing a machine-readable page. In order to be human-readable, please install an RSS reader.
All articles published by MDPI are made immediately available worldwide under an open access license. No special permission is required to reuse all or part of the article published by MDPI, including figures and tables. For articles published under an open access Creative Common CC BY license, any part of the article may be reused without permission provided that the original article is clearly cited. For more information, please refer to https://www.mdpi.com/openaccess .
Feature papers represent the most advanced research with significant potential for high impact in the field. A Feature Paper should be a substantial original Article that involves several techniques or approaches, provides an outlook for future research directions and describes possible research applications.
Feature papers are submitted upon individual invitation or recommendation by the scientific editors and must receive positive feedback from the reviewers.
Editor’s Choice articles are based on recommendations by the scientific editors of MDPI journals from around the world. Editors select a small number of articles recently published in the journal that they believe will be particularly interesting to readers, or important in the respective research area. The aim is to provide a snapshot of some of the most exciting work published in the various research areas of the journal.
Original Submission Date Received: .
- Active Journals
- Find a Journal
- Journal Proposal
- Proceedings Series
- For Authors
- For Reviewers
- For Editors
- For Librarians
- For Publishers
- For Societies
- For Conference Organizers
- Open Access Policy
- Institutional Open Access Program
- Special Issues Guidelines
- Editorial Process
- Research and Publication Ethics
- Article Processing Charges
- Testimonials
- Preprints.org
- SciProfiles
- Encyclopedia
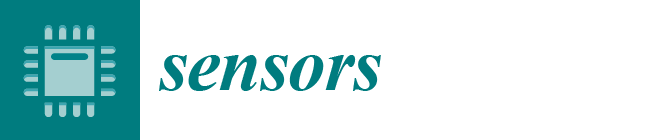
Article Menu

- Subscribe SciFeed
- Recommended Articles
- Google Scholar
- on Google Scholar
- Table of Contents
Find support for a specific problem in the support section of our website.
Please let us know what you think of our products and services.
Visit our dedicated information section to learn more about MDPI.
JSmol Viewer
Monitoring the impact of artificial structure on hydrogeological environment: a case study of hydraulic tunnel at pirot hydropower plant.
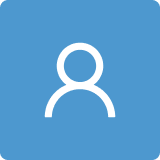
1. Introduction
2. materials and methods, 2.1. study area, geological conditions, 2.2. methods, 2.2.1. groundwater piezometry, 2.2.2. meteorological data acquisition, 2.2.3. hydrological and hydrogeological monitoring, 2.2.4. physicochemical monitoring, 2.2.5. cross-correlation, 3.1. meteorological data, 3.2. discharge regimes, 3.3. physicochemical properties of the springs, 3.4. hydrometric station assessment, 3.5. impact of artificial structure on hydrogeological environment, 3.6. cross-correlation analyses, 4. discussion, 5. conclusions, author contributions, informed consent statement, data availability statement, acknowledgments, conflicts of interest.
- Guida, D.; Longobardi, A.; Villani, P. Hydrological modelling for river basin management in a highly hydro-geological conditioned environment. In Geo-Environment and Landscape Evolution II ; WIT Transactions on Ecology and the Environment; WIT Press: Billerica MA, USA, 2006; Volume 89, pp. 283–292. Available online: https://www.witpress.com/elibrary/wit-transactions-on-ecology-and-the-environment/89/16234 (accessed on 21 July 2024).
- Schicht, R.J.; Walton, W.C. Hydrologic Budgets for Three Small Watersheds in Illinois ; Repos. of Investigation 40; Illinois State Water Survey: Champaign, IL, USA, 1961; Volume 40, pp. 1–40. [ Google Scholar ]
- Toth, J. A theoretical analysis of groundwater flow in small drainage basins. J. Geophys. Res. 1963 , 68 , 4795–4812. [ Google Scholar ] [ CrossRef ]
- Condon, L.E.; Kollet, S.; Bierkens, M.F.P.; Fogg, G.E.; Maxwell, R.M.; Hill, M.C.; Hendricks Fransen, H.-J.; Verhoef, A.; Van Loon, A.F.; Sulis, M.; et al. Global groundwater modeling and monitoring: Opportunities and challenges. Water Resour. Res. 2021 , 57 , e2020WR029500. [ Google Scholar ] [ CrossRef ]
- Hiscock, K.M. Hydrogeology: Principles and Practice , 1st ed.; Blackwell Publishing: Malden, MA, USA, 2005; p. 389. [ Google Scholar ]
- Goldscheider, N.; Madl-Szonyi, J.; Eross, A.; Schill, E. Thermal water resources in carbonate rock aquifers. Hydrogeol. J. 2010 , 18 , 1303–1318. [ Google Scholar ] [ CrossRef ]
- Shuster, E.; White, W. Seasonal fluctuations in the chemistry of limestone springs: A possible means for characterizing carbonate aquifer. J. Hydrol. 1971 , 14 , 93–128. [ Google Scholar ] [ CrossRef ]
- Bianchetti, G.; Roth, P.; Vuataz, F.D.; Vergain, J. Deep groundwater circulation in the Alps: Relations between water infiltration, induced seismicity and thermal springs: The case of Val d’Illiez, Wallis, Switzerland. Eclogae Geol. Helvet. 1992 , 85 , 291–305. [ Google Scholar ]
- Tóth, J.; Hayashi, M. The theory of basinal gravity flow of groundwater and its impacts on hydrology in Japan. J. Groundw. Hydrol. 2010 , 52 , 335–354. [ Google Scholar ] [ CrossRef ]
- Čokorilo-Ilić, M.; Mladenović, A.; Ćuk, M.; Jemcov, I. The importance of detailed groundwater monitoring for underground structure in karst (Case study: HPP Pirot, Southeastern Serbia). Water 2019 , 11 , 603. [ Google Scholar ] [ CrossRef ]
- Milanović, P. Geological Engineering in Karst ; Energoprojekt: Beograd, Serbia, 1999; pp. 26–27. (In Serbian) [ Google Scholar ]
- Li, X.; Cao, L.; Zhao, X. Assessment of potential impact of tunneling on the groundwater in Epi-Fissure-Karst-Zone and ecological environment. Environ. Earth Sci. 2012 , 66 , 967–976. [ Google Scholar ] [ CrossRef ]
- Jain, S. Fundamentals of Physical Geology ; Springer Geology: Cham, Switzerland, 2015; pp. 1–488. [ Google Scholar ] [ CrossRef ]
- Vincenzi, V.; Gargini, A.; Goldscheider, N.; Piccinini, L. Differential hydrogeological effects of draining tunnels through the northern Apennines, Italy. Rock Mech. Rock. Eng. 2014 , 47 , 947–965. [ Google Scholar ] [ CrossRef ]
- Li, L.; Tu, W.; Shi, S.; Chen, J.; Zhang, Y. Mechanism of water inrush in tunnel construction in karst area. Geomatics Nat. Hazards Risk 2016 , 7 , 35–46. [ Google Scholar ] [ CrossRef ]
- Wang, Y.; Jing, H.; Yu, L.; Su, H.; Luo, N. Set pair analysis for risk assessment of water inrush in karst tunnels. Bull. Eng. Geol. Environ. 2017 , 76 , 1199–1207. [ Google Scholar ] [ CrossRef ]
- Wang, T.; Zhan, S.; Chen, C.; Su, W. Characterizing fractures to mitigate inrush of water into a shaft using hydrogeological approaches. Tunn. Undergr. Space Technol. 2017 , 61 , 205–220. [ Google Scholar ] [ CrossRef ]
- Zheng, X.; Yang, Z.; Wang, S.; Chen, Y.F.; Hu, R.; Zhao, X.J.; Wu, X.L.; Yang, X.L. Evaluation of hydrogeological impact of tunnel engineering in a karst aquifer by coupled discrete-continuum numerical simulations. J. Hydrol. 2021 , 597 , 125765. [ Google Scholar ] [ CrossRef ]
- Gisbert, J.; Vallejos, A.; Gonzalez, A.; Pulido-Bosch, A. Environmental and hydrogeological problems in karstic terrains crossed by tunnels: A case study. Environ. Geol. 2009 , 58 , 347–357. [ Google Scholar ] [ CrossRef ]
- Hu, H.; Zhang, B.; Zuo, Y.; Zhang, C.; Wang, Y.; Guo, Z. The mechanism and numerical simulation analysis of water bursting in filling karst tunnel. Geotech. Geol. Eng. 2018 , 36 , 1197–1205. [ Google Scholar ] [ CrossRef ]
- Lv, Y.; Jiang, Y.; Hu, W.; Cao, M.; Mao, Y. A review of the effects of tunnel excavation on the hydrology, ecology, and environment in karst areas: Current status, challenges, and perspectives. J. Hydrol. 2020 , 586 , 124891. [ Google Scholar ] [ CrossRef ]
- Liu, J.; Shen, L.; Wang, Z.; Duan, S.; Wu, W.; Peng, X.; Jiang, Y. Response of plants water uptake patterns to tunnels excavation based on stable isotopes in a karst trough valley. J. Hydrol. 2019 , 571 , 485–493. [ Google Scholar ] [ CrossRef ]
- Li, H.; Kagami, H. Groundwater level and chemistry changes resulting from tunnel construction near Matsumoto City. Japan Environ. Geol. 1997 , 31 , 76–84. [ Google Scholar ] [ CrossRef ]
- Vincenzi, V.; Piccinini, L.; Gargini, A.; Sapigni, M. Parametric and numerical modelling tools to forecast hydrogeological impacts of a tunnel. Acque Sotter.–Ital. J. Groundw. 2010 , 1 , 135–154. [ Google Scholar ] [ CrossRef ]
- Piña, A.P.; Donado, L.D.; Blake, S.; Cramer, T. Compositional multivariate statistical analysis of hydrogeochemical processes in a fractured massif: La Línea Tunnel project, Colombia. Appl. Geochem. 2018 , 95 , 1–18. [ Google Scholar ] [ CrossRef ]
- Saberinasr, A.; Morsali, M.; Hashemnejad, A.; Hassanpour, J. Determining the origin of groundwater elements using hydrochemical data (case study: Kerman water conveyance tunnel). Environ. Earth Sci. 2019 , 78 , 198. [ Google Scholar ] [ CrossRef ]
- Ćuk, M.; Jemcov, I.; Mladenović, A.; Čokorilo-Ilić, M. Hydrochemical impact of the hydraulic tunnel on groundwater in the complex aquifer system in Pirot, Serbia. Carbonates Evaporites 2020 , 35 , 31. [ Google Scholar ] [ CrossRef ]
- Raposo, J.R.; Molinero, J.; Dafonte, J. Quantitative evaluation of hydrogeological impact produced by tunnel construction using water balance models. Eng. Geol. 2010 , 116 , 323–332. [ Google Scholar ] [ CrossRef ]
- Bailly-Comte, V.; Martin, J.B.; Jourde, H.; Screaton, E.J.; Pistre, S.; Langston, A. Water exchange and pressure transfer between conduits and matrix and their influence on hydrodynamics of two karst aquifers with sinking streams. J. Hydrol. 2010 , 386 , 55–66. [ Google Scholar ] [ CrossRef ]
- Schmidt, S.; Geyer, T.; Guttman, J.; Marei, A.; Ries, F.; Sauter, M. Characterisation and modelling of conduit restricted karst aquifers—Example of the Auja spring, Jordan Valley. J. Hydrol. 2014 , 511 , 750–763. [ Google Scholar ] [ CrossRef ]
- Worthington, S.R.H.; Soley, R.W.N. Identifying turbulent flow in carbonate aquifers. J. Hydrol. 2017 , 552 , 70–80. [ Google Scholar ] [ CrossRef ]
- Chen, Z.; Goldscheider, N. Modeling spatially and temporally varied hydraulic behavior of a folded karst system with dominant conduit drainage at catchment scale, Hochifen-Gottesacker, Alps. J. Hydrol. 2014 , 514 , 41–52. [ Google Scholar ] [ CrossRef ]
- Malago, A.; Efstathiou, D.; Bouraoui, F.; Nikolaidis, N.P.; Franchini, M.; Bidoglio, G.; Kritsotakis, M. Regional scale hydrologic modeling of a karst-dominant geomorphology: The case study of the Island of Crete. J. Hydrol. 2016 , 540 , 64–81. [ Google Scholar ] [ CrossRef ]
- Panno, S.V.; Hacklez, K.C.; Hwang, H.H.; Greenberg, S.; Krapac, I.G.; Landsberger, S.; O’Kelly, D.J. Source identification of sodium and chloride contamination in natural waters: Preliminary results. In Proceedings of the 12th Annual Conference of the Illinois Groundwater Consortium, Makanda, IL, USA, 22 April 2002; pp. 1–26. [ Google Scholar ]
- Čokorilo Ilić, M.; Mladenović, A.; Ćuk, M.; Radosavljević, J.; Šišović, J.; Jemcov, I. Analiza uticaja tunela HE 'Pirot' na kvantitativne karakteristike podzemnih voda. In Zbornik 8 ; Simpozijuma o zaštiti karsta: Pirot, Serbia, 2018; pp. 7–17. [ Google Scholar ]
- Ristić Vakanjac, V. Forecasting Long-Term Spring Discharge. In Monography: Karst Aquifers—Characterization and Engineering ; Stevanović, Z., Ed.; Professional Practice in Earth Science Series; Springer International Publishing: Cham, Switzerland, 2015; pp. 435–454. [ Google Scholar ]
- Anđelković, J.; Krstić, B.; Ćirić, D.; Martinović, D.; Bogdanović, P. National Geological Map of Yugoslavia ; 1:100000, sheets K 34—34: Pirot and interpretations Federal Geological Institute: Belgrade, Serbia, 1975. (In Serbian) [ Google Scholar ]
- Chaulya, S.K.; Prasad, G.M. Sensing and Monitoring Technologies for Mines and Hazardous Areas. Monitoring and Prediction Technologies ; Elsevier: Amsterdam, The Netherlands; London, UK; Cambridge, MA, USA, 2016; Chapter 1.7.4.5.5. [ Google Scholar ] [ CrossRef ]
- Kresic, N.; Stevanovic, Z. Groundwater Hydrology of Springs: Engineering, Theory, Management and Sustainability ; Butterworth-Heinemann: Amsterdam, The Netherlands; Boston, MA, USA, 2010; 573p. [ Google Scholar ]
- Han, G.; Tang, Y.; Wu, Q.; Tan, Q. Chemical and strontium isotope characterization of rainwater in karst virgin forest, Southwest China. Atmos. Environ. 2010 , 44 , 174–181. [ Google Scholar ] [ CrossRef ]
- Chaddock, R.E. Principles and Methods of Statistics , 1st ed.; Houghton Miffin Company, The Riverside Press: Cambridge, UK, 1925. [ Google Scholar ]
- Čokorilo Ilić, M.; Bajić, D.; Popović, M. Simulation of Hydrogeological Environmental Discharge in Case of Interruption Constant Observations. In Proceedings of the Sinteza—International Scientific Conference on Information Technology, Computer Science, and Data Science, Belgrade, Serbia, 16 May 2024; pp. 288–294. [ Google Scholar ]
- Wang, Y.; Tian, Y.; Cao, Y. Dam siting: A review. Water 2021 , 13 , 2080. [ Google Scholar ] [ CrossRef ]
- Attard, G.; Winiarski, T.; Rossier, Y.; Eisenlohr, L. Review: Impact of underground structures on the flow of urban groundwater. Hydrogeol. J. 2016 , 24 , 5. [ Google Scholar ] [ CrossRef ]
Click here to enlarge figure
Phase | Tunnel Operation Regime | Beginning–End of the Observation Period | Total Duration of the Phase (Days) |
---|---|---|---|
A | Operating mode/pressurized tunnel/in operation | 28 August 2016–19 September 2016 | 23 days |
B | Tunnel out of operation | 19 September 2016–24 October 2016 | 35 days |
C | Tunnel back in operation/resumed operating mode | 24 October 2016–10 November 2016 (13 December 2016) | 50 days (83 days) |
measurement range | From 0.1 to 99,900 L/s |
precision | <5% |
repetitivity | ±1% |
tracer type | NaCl |
tracer amount | from 10 g to 100 kg of salt |
ideal tracer mix | between 5 and 20 g of salt per L/s of assessed flow rate |
charging | 3 × 1.5 V alcaline batteries |
durability | ~100 h (at standard conditions) |
connection | over link RS-232 |
device dimensions/weight | 230 × 150 × 80 mm/620 g |
water resistivity | IP65 |
salt concentration | |
measurement range | 0 to 3200 mg/L |
sensitivity | 1 mg/L |
precision | <1% |
temperature | |
measurement range | 0 to +40 °C |
precision | ±0.2 °C |
pH | Temperature, °C | ORP, mV | DO, % | EC, mS/cm | TDS, g/L | |
---|---|---|---|---|---|---|
measurement range | 0.00–14.00 | −5.00–55.00 | ±2000.0 | 0.0–500.0 | 0–200 | 0–400 |
resolution | 0.001 | 0.01 | 0.1 | 0.1 | 0.001–400.0 | 0.001–400.0 |
accuracy | ±0.02 | ±0.15 | ±1.0 | ±1.5–3.0 | ±1.0% | ±1.0% |
calibration | * | automatic (in one adapted point) | based on EC calibration |
mg/L | Methods |
---|---|
HCO | volumetry |
CO | volumetry |
Cl | potentiometry |
SO | turbidimetry |
NO | spectrophotometry |
Na | AAS—atomic absorption spectrophotometry, flame technology, acetylene-air |
K | AAS—atomic absorption spectrophotometry, flame technology, acetylene-air |
Ca | AAS—atomic absorption spectrophotometry, flame technology, acetylene-nitrous oxide |
Mg | AAS—atomic absorption spectrophotometry, flame technology, acetylene-nitrous oxide |
Fe | AAS—atomic absorption spectrophotometry, flame technology, acetylene-air |
Sr | AAS—atomic absorption spectrophotometry, flame technology, acetylene-nitrous oxide |
Li | AAS—atomic absorption spectrophotometry, flame technology, acetylene-air |
H S | gas volumetry |
Tunnel Operation Regime Phase | Name of the Spring | Type of Groundwater | Tunnel Operation Regime Phase | Name of the Spring | Type of Groundwater |
---|---|---|---|---|---|
A | Dobrodolska River | Ca-Mg-HCO | A | Dag Banjica | Ca-Mg-HCO |
B | Ca-HCO | B | Ca-Mg-HCO | ||
C | Ca-Mg-HCO | C | Ca-Mg-HCO | ||
A | Izvor | Ca-HCO | A | Berilovac | Ca-HCO |
B | Ca-HCO | B | Ca-HCO | ||
C | Ca-HCO | C | Ca-Mg-HCO | ||
A | Nisor 1 | Ca-HCO | A | Nisor 2 | Ca-HCO |
B | Ca-HCO | B | Ca-HCO | ||
C | Ca-HCO | C | Ca-HCO | ||
A | Dobri Do | Ca-HCO | A | Glame | Ca-HCO -SO |
B | Ca-HCO | B | Ca-HCO -SO | ||
C | Ca-HCO | C | Ca-HCO -SO |
Coefficient of Determination | Interpretation |
---|---|
0.00 | no correlation |
0.00–0.25 | weak correlation |
0.25–0.64 | moderate correlation |
0.64–1.00 | strong correlation |
1.00 | total correlation |
The statements, opinions and data contained in all publications are solely those of the individual author(s) and contributor(s) and not of MDPI and/or the editor(s). MDPI and/or the editor(s) disclaim responsibility for any injury to people or property resulting from any ideas, methods, instructions or products referred to in the content. |
Share and Cite
Čokorilo Ilić, M.; Popović, M.P.; Bajić, D.; Matović, V.; Abramović, F.; Alimpić, F. Monitoring the Impact of Artificial Structure on Hydrogeological Environment: A Case Study of Hydraulic Tunnel at Pirot Hydropower Plant. Sensors 2024 , 24 , 6578. https://doi.org/10.3390/s24206578
Čokorilo Ilić M, Popović MP, Bajić D, Matović V, Abramović F, Alimpić F. Monitoring the Impact of Artificial Structure on Hydrogeological Environment: A Case Study of Hydraulic Tunnel at Pirot Hydropower Plant. Sensors . 2024; 24(20):6578. https://doi.org/10.3390/s24206578
Čokorilo Ilić, Marina, Miroslav P. Popović, Dragoljub Bajić, Vesna Matović, Filip Abramović, and Filip Alimpić. 2024. "Monitoring the Impact of Artificial Structure on Hydrogeological Environment: A Case Study of Hydraulic Tunnel at Pirot Hydropower Plant" Sensors 24, no. 20: 6578. https://doi.org/10.3390/s24206578
Article Metrics
Article access statistics, further information, mdpi initiatives, follow mdpi.
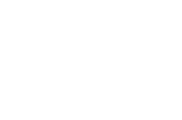
Subscribe to receive issue release notifications and newsletters from MDPI journals
Multifactor Coupled Evaluation Model of Fracability Based on a Combinatorial Weighting Method: A Case Study of the Low-Permeability Sandstone Reservoirs of Yanchang Formation, Southwestern Ordos Basin, China
- Original Paper
- Published: 13 October 2024
Cite this article
- Yongming Yang 1 ,
- Xiao Li 1 ,
- Yang Ju ORCID: orcid.org/0000-0003-4297-4455 2 ,
- Gang Li 1 &
- Xiwen Li 1
The accurate evaluation of the fracability of low-permeability rocks is crucial for designing and optimizing hydraulic fracturing strategies in low-permeability reservoirs. In this study, fracability was defined as a property that measures the ability of low-permeability sandstones to form complicated fractures through hydraulic fracturing. Therefore, we investigated the influence of mechanical characteristics, physical properties, and geological conditions on the breakdown pressure and fracture morphology in low-permeability sandstones. To address the limitations of existing fracability evaluation models, a combinatorial weighting method (CWM) combining subjective and objective weighting methods was proposed. Based on this weighting method, we established a multifactor coupled evaluation model (MCEM) for evaluating the fracability of low-permeability sandstones and introduced a new fracability index to quantify the results derived from this model. The effectiveness of the evaluation model was validated through comparative analysis with existing fracability evaluation models. Using this evaluation method, we evaluated the fracability of Yanchang Formation’s low-permeability sandstone reservoirs in the southwestern Ordos Basin to identify the best candidates. The evaluation results were consistent with the daily oil production from the field reservoirs. The multifactor coupled evaluation model based on the combinatorial weighting method (MCEM–CWM) comprehensively considers the effects of physical, mechanical, and geological factors on fracability, and combines the subjective and objective weighting methods to overcome the shortcomings of the single weighting method of existing fracability evaluation models. The study provides a novel approach for designing and optimizing hydraulic fracturing stimulation strategies in low-permeability reservoirs.
Influences of multiple fators on the fracability of low-permeability reservoirs were revealed.
A multifactor coupled evaluation model based on the combinatorial weighting method was established.
A method to classify low-permeability sandstone reservoirs fracability was proposed.
The fracability of low-permeability sandstone reservoirs of Yanchang Formation was evaluated.
This is a preview of subscription content, log in via an institution to check access.
Access this article
Subscribe and save.
- Get 10 units per month
- Download Article/Chapter or eBook
- 1 Unit = 1 Article or 1 Chapter
- Cancel anytime
Price includes VAT (Russian Federation)
Instant access to the full article PDF.
Rent this article via DeepDyve
Institutional subscriptions
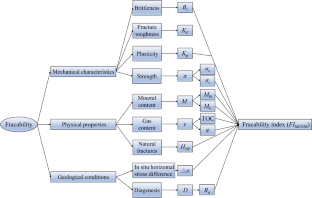
Data Availability
The data are available from the corresponding author on reasonable request.
Abbreviations
Combinatorial weighing method
Combination-ordered weighted averaging
Criteria importance through the intercriteria correlation
- Multifactor coupled evaluation model
- Combinatorial weighting method
Natural fractures
Hydraulic fractures
Horizontal stress difference
Fracability index
Brittleness index
Elastic modulus (MPa)
Poisson's ratio
Normalized brittleness index
Normalized elastic modulus
Normalized Poisson’s ratio
Maximum value of E
Minimum value of E
Maximum value of μ
Minimum values of μ
Fracture toughness
Fracture toughness of type I
Fracture toughness of type II
Plasticity coefficient
Strength (MPa)
Uniaxial compressive strength (MPa)
Tensile strength (MPa)
Mineral composition (%)
Brittle mineral content (%)
Clay mineral content (%)
Gas content (m 3 /t)
Adsorbed gas content (m 3 /t)
Free gas content (m 3 /t)
Effective porosity (%)
Natural fracture development index
3D fractal dimension
Normalized relative surface area of the NFs
Reverse indicator
Surface area of the fractures (m 2 )
Volume of the fractures (m 3 )
Vertical in situ stress (MPa)
Maximum horizontal in situ stress (MPa)
Minimum horizontal in situ stress (MPa)
In situ horizontal stress difference (MPa)
Vitrinite reflectance (%)
Subjective relative weights
Volume of information
Standard deviation
Objective relative weight
Combinatorial weight
Abdulaziz AM, Hawary SS (2020) Prediction of carbonate diagenesis from well logs using artificial neural network: an innovative technique to understand complex carbonate systems. Ain Shams Eng J 11(4):1387–1401. https://doi.org/10.1016/j.asej.2020.01.010
Article Google Scholar
Amiri H, Ramezanzadeh A, Chamanzad M, Parhizgar M (2021) Recognizing the best intervals for hydraulic fracturing using a new fracability index. J Pet Explor Prod Tech 11:3193–3201. https://doi.org/10.1007/s13202-021-01173-7
Awaji H, Sato S (1978) Combined mode fracture toughness measure by disk test. J Eng Mater Tech 100:175–182. https://doi.org/10.1115/1.3443468
Cao H, Zhao Y, Gao Q, Bao T, Sun PH (2021) An insight into shale’s time-dependent brittleness based on the dynamic mechanical characteristics induced by fracturing fluid invasion. J Nat Gas Sci Eng 96:104312. https://doi.org/10.1016/j.jngse.2021.104312
Cheng W, Jin Y, Chen M (2015) Reactivation mechanism of natural fractures by hydraulic fracturing in naturally fractured shale reservoirs. J Nat Gas Sci Eng 23:431–439. https://doi.org/10.1016/j.jngse.2015.01.031
Diakoulaki D, Mavrotas G, Papayannakis L (1995) Determining Objective weights in multiple criteria problems: The CRITIC method. Comput Oper Res 22(7):763–770. https://doi.org/10.1016/0305-0548(94)00059-H
Dou LB, Yang HJ, Xiao YJ, Gao H, Li TT, Sun HB (2021) Probability study of formation brittleness and new quantitative evaluation of fracability for shale reservoirs. Prog Geophys 36(2):0576–0584. https://doi.org/10.6038/pg2021EE0460
Dou LB, Zuo XD, Qu L, Xiao YJ, Bi G, Wang R, Zhang M (2022) A new method of quantitatively evaluating fracability of tight sandstone reservoirs using geomechanics characteristics and in situ stress field. Processes 10(5):1040. https://doi.org/10.3390/pr10051040
Article CAS Google Scholar
Fan WC, Xu ZM, Wu B, He YS, Zhang ZF (2022) Structural multi-objective topology optimization and application based on the criteria importance through intercriteria correlation method. Eng Optimiz 54(5):830–846. https://doi.org/10.1080/0305215X.2021.1901087
Fu HJ, Wang XZ, Zhang LX, Gao RM, Li ZT, Zhu XL, Xu W, Li Q, Xu T (2015) Geological controls on artificial fracture networks in continental shale and its fracability evaluation: a case study in the Yanchang Formation, Ordos Basin, China. J Nat Gas Sci Eng 26:1285–1293. https://doi.org/10.1016/j.jngse.2015.08.034
Gong DG, Qu ZQ, Li JX, Qu GZ, Cao YC, Guo TK (2016) Extended finite element simulation of hydraulic fracture based on ABAQUS platform. Rock Soil Mech 37(5):1512–1520. https://doi.org/10.16285/j.rsm.2016.05.036
Guan ZC, Chen TG (2017) Theory and technology of drilling engineering. China University of Petroleum Press, Dongying
Google Scholar
Guo JL, Jia CY, He DB, Meng FK (2021) Classification and evaluation on shale gas reservoir for Wufeng-Longmaxi formation in Chuannan area, Sichuan Basin. Lithosphere 1:3364731. https://doi.org/10.2113/2021/3364731
Huang C, Xu TY, Ju YW, Zhu HJ, Ju LT, Li WY (2019) Fracability evaluation of shale of the Wufeng-Longmaxi Formation in the Changning Area, Sichuan Basin. Acta Geol Sin-Engl 93(4):996–1004. https://doi.org/10.1111/1755-6724.13877
Huo XS, Xue H, Jiao LD (2023) Risk management of retrofit project in old residential areas under green development. Energ Build 279:112708. https://doi.org/10.1016/j.enbuild.2022.112708
Iyare UC, Ramsook R, Blake OO (2021) Fracability evaluation of the upper Cretaceous Naparima Hill Formation, Trinidad. J Pet Sci Eng 208(D):109599. https://doi.org/10.1016/j.petrol.2021.109599
Jiang HY (2020) Study on rock mechanical characteristics and volume fracturing “Sweet spot” of tight sandstone reservoir in Area X. Xi’an Shiyou University, Xi’an
Jin XC, Shah SN, Roegiers JC, Zhang B (2015) An integrated petrophysics and geomechanics approach for fracability evaluation in shale reservoirs. SPE J 20(3):518–526. https://doi.org/10.2118/168589-PA
Ju Y, Wu GJ, Wang YL, Liu P, Yang YM (2021) 3D Numerical model for hydraulic fracture propagation in tight ductile reservoirs, considering multiple influencing factors via the entropy weight method. SPE J 26(5):2685–2702. https://doi.org/10.2118/205385-PA
Lai FQ, Luo H, Qin DY, Xia WX, Gong DJ (2018) Crushability evaluation of shale gas reservoir based on analytic hierarchy process. Spec Oil Gas Reserv 25(03):154–159. https://doi.org/10.3969/j.issn.1006-6535.2018.03.031
Li XH, Zhang LY, Zhang RR, Yang M, Li H (2021) A semi-quantitative methodology for risk assessment of university chemical laboratory. J Loss Prevent Proc 72:104553. https://doi.org/10.1016/j.jlp.2021.104553
Li Z, Li G, Yu H, Jiang ZJ, Li HZ, Gong HB (2022) Fracability evaluation based on the three-dimensional geological numerical simulation of in situ stress: case study of the Longmaxi Formation in the Weirong shale gas field, southwestern China. Math Geosci 54:1069–1096. https://doi.org/10.1007/s11004-022-10001-5
Liu XQ, Zhang WD, Qu ZQ, Guo TK, Sun Y, Rabiei M, Cao QY (2020) Feasibility evaluation of hydraulic fracturing in hydrate-bearing sediments based on analytic hierarchy process-entropy method (AHP-EM). J Nat Gas Sci Eng 81:103434. https://doi.org/10.1016/j.jngse.2020.103434
Liu JG, Qu LS, Song ZY, Li J, Liu C, Feng YC, Sun HH (2021) Fracability evaluation method and influencing factors of the tight sandstone reservoir. Geofluids 2021:7092143. https://doi.org/10.1155/2021/7092143
Lu C, Ma L, Guo JC, Li XY, Zheng YC, Ren Y, Yin CB, Li JF, Zhou GQ, Wang JD, Du ZJ, Meng XB, Song MS, Yang B (2021) Novel method and case study of a deep shale fracability evaluation based on the brittleness index. Energ Explor Exploit 40(1):442–459. https://doi.org/10.1177/01445987211033656
Luo ZH, Yuan GY, Cen K, Li W (2022) Evaluation of the oil-bearing drilling cuttings processing technology in petrochemical industry under cleaner production: a case study in China. J Clean Prod 375:134041. https://doi.org/10.1016/j.jclepro.2022.134041
Meng SW, Li DX, Wang Q, Tao JP, Su J, Li HQ, Jin X, Yang L (2021) Fracability evaluation of the upper Cretaceous Naparima Hill Formation, Trinidad. Front Earth Sci 9:797405. https://doi.org/10.3389/feart.2021.797405
Nguyen ND, Nguyen TT, Vamplew P, Dazeley R, Nahavandi S (2021) A Prioritized objective actor-critic method for deep reinforcement learning. Neural Comput Appl 33(16):10335–10349. https://doi.org/10.1007/s00521-021-05795-0
Noack K (1998) Control of gas emissions in underground coal mines. Int J Coal Geol 35:57–82. https://doi.org/10.1016/S0166-5162(97)00008-6
Peng CY, Zhou J, Wu JS, Jiang M, Zhang H, Yin B, Liu SY, Zhang Y (2023) Evaluation of tight sandstone mechanical properties and fracability: An experimental study of reservoir sand-stones from Lufeng Sag, Pearl River Mouth Basin, northern south China Sea. Processes 11(7):2135. https://doi.org/10.3390/pr11072135
Reinicke A, Rybacki E, Stanchits S, Huenges E, Dresen G (2010) Hydraulic fracturing stimulation techniques and formation damage mechanisms-Implications from laboratory testing of tight sandstone-proppant systems. Chem Erdr-Geochem 70(s3):107–117. https://doi.org/10.1016/j.chemer.2010.05.016
Rickman R, Mullen M, Petre E, Grieser B (2008) A practical use of shale petro-physics for stimulation design optimization: all shale plays are not clones of the Barnett Shale. In: SPE annual technical conference and exhibition, 21–24 September, Colorado, USA. https://doi.org/10.2118/115258-MS
Shen C, Zhao JZ, Xie J, Fu YQ, Wu JF, Ren L (2021) The main controlling factors and evaluation method of the reservoir stimulation potential: a case study of the Changning shale gas field, Southern Sichuan Basin, SW China. Front Earth Sci 9:738668. https://doi.org/10.3389/feart.2021.738668
Shen C, Ren L, Zhao JZ, Tan XC, Wu LZ (2017) A comprehensive evaluation index for shale reservoirs and its application: A case study of the Ordovician Wufeng Formation to Silurian Longmaxi Formation in southeastern margin of Sichuan Basin, SW China. Petrol Explor Dev+ 44(4):686–695. https://doi.org/10.1016/S1876-3804(17)30078-2
Shi HT, Li YF, Jiang ZN, Zhang J (2021) Comprehensive power quality evaluation method of microgrid with dynamic weighting based on CRITIC. Meas Control 54(5–6):1097–1104. https://doi.org/10.1177/00202940211016092
Song YB, Lu WY, Bai EH (2020) Numerical simulation of the influence of natural fractures on hydraulic fracture propagation. Geofluids 2020:8878548. https://doi.org/10.1155/2020/8878548
Sui LL, Ju Y, Yang YM, Yang Y, Li AS (2016) A quantification method for shale fracability based on analytic hierarchy process. Energy 115:637–645. https://doi.org/10.1016/j.energy.2016.09.035
Sui HY, Gao W, Hu RL (2019) A new evaluation method for the fracability of a shale reservoir based on the structural properties. Geofluids 2019:2079458. https://doi.org/10.1155/2019/2079458
Sun SN, Chen YY, Wang AL, Liu XJ (2022) An evaluation model of carbon emission reduction effect of prefabricated buildings based on cloud model from the perspective of construction supply chain. Buildings 12(10):1534. https://doi.org/10.3390/buildings12101534
Tang Y, Xing Y, Li LZ, Zhang BH, Jiang SX (2012) Influence factors and evaluation methods of the gas shale fracability. Earth Sci Front 19(5):356–363
CAS Google Scholar
Wang DB, Ge HK, Wang XQ, Wang JB, Meng FB, Suo Y, Han P (2015) A novel experimental approach for fracability evaluation in tight-gas reservoirs. J Nat Gas Sci Eng 23:239–249. https://doi.org/10.1016/j.jngse.2015.01.039
Wang XQ, Ge HK, Wang DB, Wang JB, Chen H (2017) A comprehensive method for fracability evaluation of shale combined with brittleness and stress sensitivity. J Geophys Eng 14(6):1420–1429. https://doi.org/10.1088/1742-2140/aa7ffd
Wang W, Wu LG, Li X, Qu FF, Li WB, Ma YY, Ma DH (2023) An evaluation method for automated vehicles combining subjective and objective factors. Machines 11(6):597. https://doi.org/10.3390/machines11060597
Wu JJ, Zhang SH, Luo X (2017) Fracability evaluation of shale gas reservoir-A case study in the Lower Cambrian Niutitang formation, northwestern Hunan, China. J Petrol Sci Eng 164:675–684. https://doi.org/10.1016/j.petrol.2017.11.055
Xie HP, Xue XQ (1997) The mathematical foundation and method of fractal application. Science Press, Beijing
Xie HP (1993) Fractals in rock mechanics. A. A. Balkema, Netherlands
Xing C, Yao LH, Wang YD, Hu ZJ (2022) Suitability evaluation of the lining form based on combination weighting-set pair analysis. Appl Sci-Basel 12(10):4896. https://doi.org/10.3390/app12104896
Xu ZS (2006) A C-OWA operator-based approach to decision making with interval fuzzy preference relation. Int J Intell Syst 21(12):1289–1298. https://doi.org/10.1002/int.20184
Xu WJ, Zhao JZ, Xu JG (2021) Fracability evaluation method for tight sandstone oil reservoirs. Nat Resour Res 30(6):4277–4295. https://doi.org/10.1007/s11053-021-09907-4
Yager RR (1988) On ordered weighted averaging aggregation operators in multicriteria decision making. IEEE Trans Syst Man Cybern 18(1):183–190. https://doi.org/10.1109/21.87068
Yager RR (1993) Families of OWA operators. Fuzzy Set Syst 59(2):125–148. https://doi.org/10.1016/0165-0114(93)90194-M
Yalcin N, Unlu U (2018) A multi-criteria performance analysis of initial public offering (IPO) firms using critic and vikor methods. Technol Econ Dev Ecol 24(2):534–560. https://doi.org/10.3846/20294913.2016.1213201
Yang BC, Xue L, Duan YT, Wang MM (2021) Correlation study between fracability and brittleness of shale-gas reservoir. Geomech Geophys Geol 7(2):31. https://doi.org/10.1007/s40948-021-00231-y
Yang YM, Li X, Fu ZP, Ju Y (2022a) Study of preparing artificial cores and propagation of hydraulic fractures in plastic sandstones. Geomech Geophys Geol 8(6):178. https://doi.org/10.1007/s40948-022-00493-0
Yang YM, Li X, Ju Y (2022b) Influence mechanisms of plasticity and horizontal stress difference on the fracture propagation in plastic reservoir rocks: a 3D XFEM-based plastic fracturing model. Geomech Geophys Geo 8(5):145. https://doi.org/10.1007/s40948-022-00453-8
Zhai WB, Li J, Zhou YC (2019) Application of catastrophe theory to fracability evaluation of deep shale reservoir. In: 4th International Conference on computational and experimental science and engineering (ICCESEN), Antalya, Turkey. 12(5):161. https://doi.org/10.1007/s12517-019-4332-1
Zhai MY, Li LC, Wang ZL, Zhang LY, Li AS, Zhang ZL, Zheng BT, Huang B (2021) Three-Dimensional numerical simulation and analysis of geomechanical controls of hydraulic fracturing in heterogeneous formations. Arab J Sci Eng 47(9):11533–11552. https://doi.org/10.1007/s13369-021-06225-y
Zhang JB, Wu CF (2012) Application status and improvement of coal bed methane development technology. Coal Sci Tech 40(8):88–91+96. https://doi.org/10.13199/j.cst.2012.08.91.zhangjb.005
Zhou J, Zhang LQ, Braun A, Han ZH (2017) Investigation of processes of interaction between hydraulic and natural fractures by PFC modeling comparing against laboratory experiments and analytical models. Energies 10(7):1001. https://doi.org/10.3390/en10071001
Download references
Acknowledgements
This work was supported by the National Natural Science Foundation of China [grant number 51874309, 52121003], National Key Research and Development Project of China (2022YFC3004602), and Basic research expenses of China University of Mining and Technology Beijing-Top Innovative Talents Cultivation Fund for doctoral students [grant number BBJ2023050].
This work was funded by the National Natural Science Foundation of China [grant number 51874309, 52121003], National Key Research and Development Project of China (2022YFC3004602), and Basic research expenses of China University of Mining and Technology Beijing-Top Innovative Talents Cultivation Fund for doctoral students [grant number BBJ2023050].
Author information
Authors and affiliations.
School of Mechanical and Civil Engineering, China University of Mining and Technology-Beijing, D11 Xueyuan Road, Beijing, 100083, China
Yongming Yang, Xiao Li, Gang Li & Xiwen Li
State Key Laboratory of Coal Resources and Safe Mining, China University of Mining and Technology at Beijing, Beijing, 100083, China
You can also search for this author in PubMed Google Scholar
Contributions
Yongming Yang: investigation, formal analysis, methodology, software, writing–original draft. Xiao Li: data curation, investigation, formal analysis, software, writing–original draft. Yang Ju: conceptualization, methodology, project administration, supervision, funding acquisition, writing–editing and review. Gang Li: formal analysis, validation. Xiwen Li: investigation, formal analysis, visualization.
Corresponding author
Correspondence to Yang Ju .
Ethics declarations
Conflict of interest.
The authors declare that they have no known competing financial interests or personal relationships that could have appeared to influence the work reported in this paper.
Additional information
Publisher's note.
Springer Nature remains neutral with regard to jurisdictional claims in published maps and institutional affiliations.
Rights and permissions
Springer Nature or its licensor (e.g. a society or other partner) holds exclusive rights to this article under a publishing agreement with the author(s) or other rightsholder(s); author self-archiving of the accepted manuscript version of this article is solely governed by the terms of such publishing agreement and applicable law.
Reprints and permissions
About this article
Yang, Y., Li, X., Ju, Y. et al. Multifactor Coupled Evaluation Model of Fracability Based on a Combinatorial Weighting Method: A Case Study of the Low-Permeability Sandstone Reservoirs of Yanchang Formation, Southwestern Ordos Basin, China. Rock Mech Rock Eng (2024). https://doi.org/10.1007/s00603-024-04193-x
Download citation
Received : 19 May 2023
Accepted : 07 September 2024
Published : 13 October 2024
DOI : https://doi.org/10.1007/s00603-024-04193-x
Share this article
Anyone you share the following link with will be able to read this content:
Sorry, a shareable link is not currently available for this article.
Provided by the Springer Nature SharedIt content-sharing initiative
- Fracability
- Low-permeability reservoirs
- Find a journal
- Publish with us
- Track your research
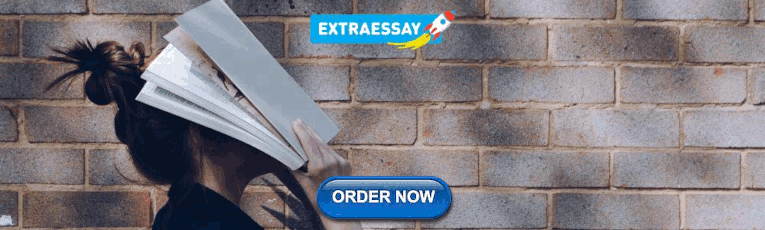
COMMENTS
A case study is a detailed study of a specific subject, such as a person, group, place, event, organization, or phenomenon. Case studies are commonly used in social, educational, clinical, and business research. A case study research design usually involves qualitative methods, but quantitative methods are sometimes also used.
Defnition: A case study is a research method that involves an in-depth examination and analysis of a particular phenomenon or case, such as an individual, organization, community, event, or situation. It is a qualitative research approach that aims to provide a detailed and comprehensive understanding of the case being studied.
Case studies are in-depth investigations of a person, group, event, or community. Typically, data is gathered from various sources using several methods (e.g., observations & interviews). The case study research method originated in clinical medicine (the case history, i.e., the patient's personal history). In psychology, case studies are ...
Definition, Methods, and Examples. Case study methodology offers researchers an exciting opportunity to explore intricate phenomena within specific contexts using a wide range of data sources and collection methods. It is highly pertinent in health and social sciences, environmental studies, social work, education, and business studies.
The purpose of case study research is twofold: (1) to provide descriptive information and (2) to suggest theoretical relevance. Rich description enables an in-depth or sharpened understanding of the case. It is unique given one characteristic: case studies draw from more than one data source. Case studies are inherently multimodal or mixed ...
Jean Piaget's observations of his own children are good examples of how an intrinsic case study can contribute to the development of a psychological theory. The three main case study types often used are intrinsic, instrumental, and collective. Intrinsic case studies are useful for learning about unique cases.
A case study protocol outlines the procedures and general rules to be followed during the case study. This includes the data collection methods to be used, the sources of data, and the procedures for analysis. Having a detailed case study protocol ensures consistency and reliability in the study.
A case study is defined as an in-depth analysis of a particular subject, often a real-world situation, individual, group, or organization. It is a research method that involves the comprehensive examination of a specific instance to gain a better understanding of its complexities, dynamics, and context.
Key Features. Presents a definition of case study research that can be used in different fields of study; Describes case study as a research strategy rather than as a single tool for decision making and inquiry; Guides rather than dictates, readers understanding and applications of case study research; Includes a critical summary in each entry ...
Case study method is the most widely used method in academia for researchers interested in qualitative research (Baskarada, 2014). Research students select the case study as a method without understanding array of factors that can affect the outcome of their research. ... Characteristics, prevalence and tensions of critical thinking in Indon ...
A case study, in common parlance, documents a particular situation or event in detail in a specific sociopolitical context. The particular can be a person, a classroom, an institution, a program, or a policy. Below I identify different ways in which case study is used before focusing on qualitative case study research in particular.
ase study method (Yin, 2006, 2009b, and 2011a).3To maintain its brevity, the refresher gives less attention to the reporting phase of case studies, although a few words of advice are still offe. ed with regard to presenting case study evidence.The refresher concludes by discussing the positioning of the case study method among other social ...
The term "case study" refers to both a specific research design or methodology, and a method of analysis for examining a problem. Mills et al. (2010) note that case study, both as a methodology and as a method—unlike many qualitative methodologies—is frequently used to generalize across populations.
Case study method is founded on the principle that each case should be regarded as a complete and unique phenomenon if we are to understand its internal dynamics. Regardless of the number of cases analyzed, any study based on this method requires a thorough knowledge of each case (Stake 2005).
Case Study Method. The case study method is a very popular form of qualitative analysis and involves a careful and complete observation of a social unit, be that unit a person, a family, an institution, a cultural group, or even the entire community. It is a method of study in depth rather than breadth.
The definitions of case study evolved over a period of time. Case study is defined as "a systematic inquiry into an event or a set of related events which aims to describe and explain the phenomenon of interest" (Bromley, 1990).Stoecker defined a case study as an "intensive research in which interpretations are given based on observable concrete interconnections between actual properties ...
A case study is one of the most commonly used methodologies of social research. This article attempts to look into the various dimensions of a case study research strategy, the different epistemological strands which determine the particular case study type and approach adopted in the field, discusses the factors which can enhance the effectiveness of a case study research, and the debate ...
According to the book Understanding Case Study Research, case studies are "small scale research with meaning" that generally involve the following: The study of a particular case, or a number of cases. That the case will be complex and bounded. That it will be studied in its context. That the analysis undertaken will seek to be holistic.
A case study is a detailed study of a specific subject, such as a person, group, place, event, organisation, or phenomenon. Case studies are commonly used in social, educational, clinical, and business research. A case study research design usually involves qualitative methods, but quantitative methods are sometimes also used.
Beyond teaching specific subject matter, the case study method excels in instilling meta-skills in students. This article explains the importance of seven such skills: preparation, discernment ...
Researchers, economists, and others frequently use case studies to answer questions across a wide spectrum of disciplines, from analyzing decades of climate data for conservation efforts to developing new theoretical frameworks in psychology. Learn about the different types of case studies, their benefits, and examples of successful case studies.
Book Features: A straightforward introduction to the science of doing case study research. ... This book presents a disciplined, qualitative exploration of case study methods by drawing from ...
Definitions of qualitative case study research. Case study research is an investigation and analysis of a single or collective case, intended to capture the complexity of the object of study (Stake, 1995).Qualitative case study research, as described by Stake (), draws together "naturalistic, holistic, ethnographic, phenomenological, and biographic research methods" in a bricoleur design ...
Background The private healthcare sector has become an essential component of healthcare systems globally. This interest has increased with the universal health coverage agenda. However, in most low- and middle-income countries, few classificatory studies of the private hospital sector were carried out. Methods This study describes the private hospital sector in a developing country setup and ...
This study builds upon these previous findings by introducing a more systematic and data-driven method to evaluate and classify rail transit stations into clusters based on their characteristics.
This paper uses the lattice Boltzmann method (LBM) to study the droplet impact behavior on different microstructured surfaces: right-triangle, semicircular, and rectangular microstructures. The formulas for calculating the surface wetting characteristics of different microstructures are derived theoretically, the difference between simulation ...
Artificial objects, particularly tunnels used for water transport under pressure, impact the geological and hydrogeological environment to a greater or lesser extent, and it is vital to assess their contributions to groundwater quality. Although tunnels are typically lined with concrete, their interaction with the hydrogeological environment intensifies over time. In this study, the detailed ...
The accurate evaluation of the fracability of low-permeability rocks is crucial for designing and optimizing hydraulic fracturing strategies in low-permeability reservoirs. In this study, fracability was defined as a property that measures the ability of low-permeability sandstones to form complicated fractures through hydraulic fracturing. Therefore, we investigated the influence of ...