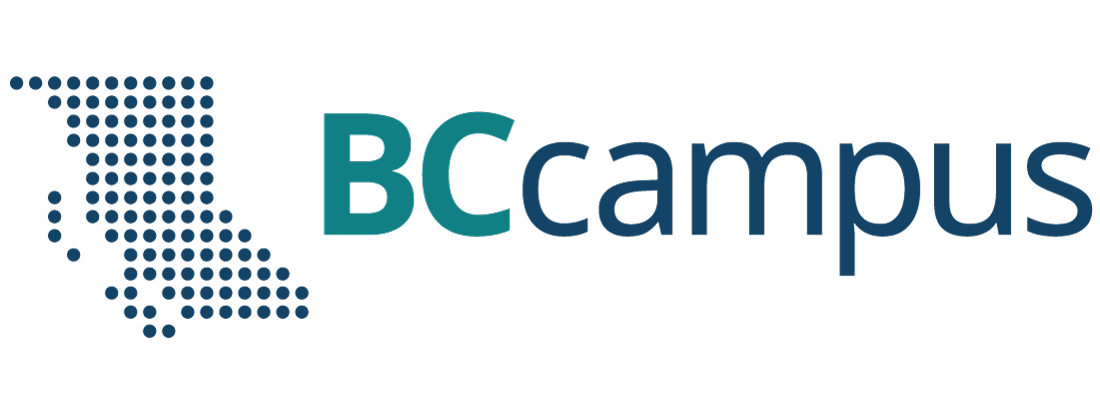
Want to create or adapt books like this? Learn more about how Pressbooks supports open publishing practices.
Chapter 6: Data Collection Strategies
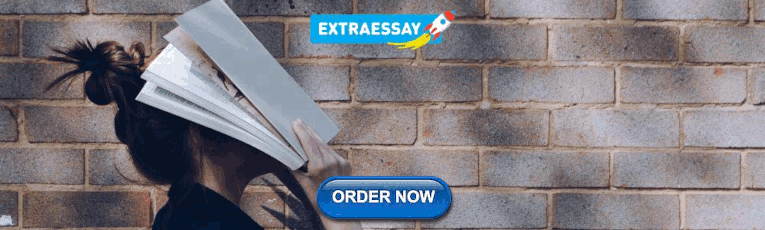
6.1 Experiments
An experiment is a method of data collection designed to test hypotheses under controlled conditions (often in a laboratory), with the goal to eliminate threats to internal validity. Most commonly a quantitative research method, experiments are used more often by psychologists than sociologists, but understanding what experiments are and how they are conducted is useful for all social scientists, whether they actually plan to use this methodology or simply aim to understand findings based on experimental designs.
An experiment is a method of data collection designed to test hypotheses under controlled conditions, with the goal to eliminate threats to internal validity. There are different experiment designs. In the classic experiment , the effect of a stimulus is tested by comparing two groups: one that is exposed to the stimulus (the experimental group ) and another that does not receive the stimulus (the control group ). The control group, often called the comparison group, is treated equally to the experimental group in all respects, except it does not receive the independent variable. The purpose of the control group is to control for rival plausible explanations.
Most experiments take place in a lab or some other controlled environment. In an experiment, the effects of an independent variable upon a dependent variable are tested. Because the researcher’s interest lies in the effects of an independent variable, the researcher must measure participants on the dependent variable before (a pre-test) and after (post-test) the independent variable (or stimulus) is administered. In this type of experiment researchers employ random assignation (often referred to as random assignment), which means that one group is the equivalent of the other. Random assignation is more fully explored in the following section “ 6.1.1 Random Assignation ”.
It is important to note that social sciences research usually takes place in a natural setting, where the researcher will utilize a quasi-experimental design, rather than an experimental design. Similar to an experiment, the independent variable in a quasi-experiment is manipulated. A quasi-experimental design is discussed in more detail in section 6.3 Quasi-experimental research .
Students in research methods classes often use the term “experiment” to describe all kinds of empirical research projects, but in social scientific research the term has a unique meaning and should not be used to describe all research methodologies. In general, designs considered to be “true experiments” contain three key features:
- Independent and dependent variables.
- Pretesting and post-testing.
- Experimental and control groups.
Pretesting and post-testing are both important steps in a classic experiment. Here are a couple of hypothetical examples.
In a study of PTSD, 100 police officer participants from the Winnipeg police department were randomly assigned to either an experiment or control group. All of the police officer participants, from both the experiment and the control group were given the exact same pre-test to assess their levels of PTSD. No significant differences in reported levels of symptoms related to PTSD were found between the experimental and control groups during the pre-test. Participants in the experimental group were then asked to watch a video on scenic travel routes in Manitoba. Both groups then underwent a post-test to re-measure their reported level of symptoms related to PTSD. Upon measuring the scores from the post-test, the researchers discovered that those who had received the experimental stimulus (the video on the car accident) reported greater symptoms of PTSD than those in the control group.
As you can see from Example 1, the dependent variable is reported levels of PTSD symptoms (measured through the pre- and post-test) and the independent variable is visual exposure to trauma (video). Ask yourself: Is the reported level of PTSD symptoms dependent upon visual exposure to trauma (as depicted through the video)? Table 6.1 depicts the design of the study from example 1, above.
01 | XE | 02 |
01 | XC | 02 |
- X stands for the treatment
- E stands for the experimental group (e.g., car accident video)
- C stands for the control or comparison group (e.g., scenic byways of Manitoba video)
- O stands for time, subscripts stand for time: 1=time one; 2=time two.
In one portion of a multifaceted study on depression, all participants were randomly assigned to either an experimental or a control group. All participants were given a pre-test to assess their levels of depression. No significant differences in depression were found between the experimental and control groups during the pre- test. Participants in the experimental group were then asked to read an article suggesting that prejudice against their same racial group is severe and pervasive. Upon measuring depression scores during the post-test period, the researchers discovered that those who had received the experimental stimulus (the article citing the prejudice against their same racial group) reported greater depression than those in the control group (McCoy & Major, 2003).
Now it is your turn. See if you can fill in Table 6.2, based upon what you read in Example 2.
- X stands for the treatment.
- E stands for the experimental group (e.g., ).
- C stands for the control or comparison group (e.g., ).
- O stands for time, subscript stands for ( ).
- The dependent variable is ).
- The independent variable is ).
01 | XE | 02 |
01 | XC | 02 |
- X stands for treatment.
- E stands for the experimental group (e.g., article on severe prejudice within group ).
- C stands for the control or comparison group (e.g., article on severe prejudice outside group).
- O stands for time, 1 and 2 subscripts stand for time: 1=time one; 2=time two.
- The dependent variable is depression .
- The independent variable is feelings that prejudice is a significant issue within your racial group.
Research Methods for the Social Sciences: An Introduction Copyright © 2020 by Valerie Sheppard is licensed under a Creative Commons Attribution-NonCommercial-ShareAlike 4.0 International License , except where otherwise noted.
Share This Book
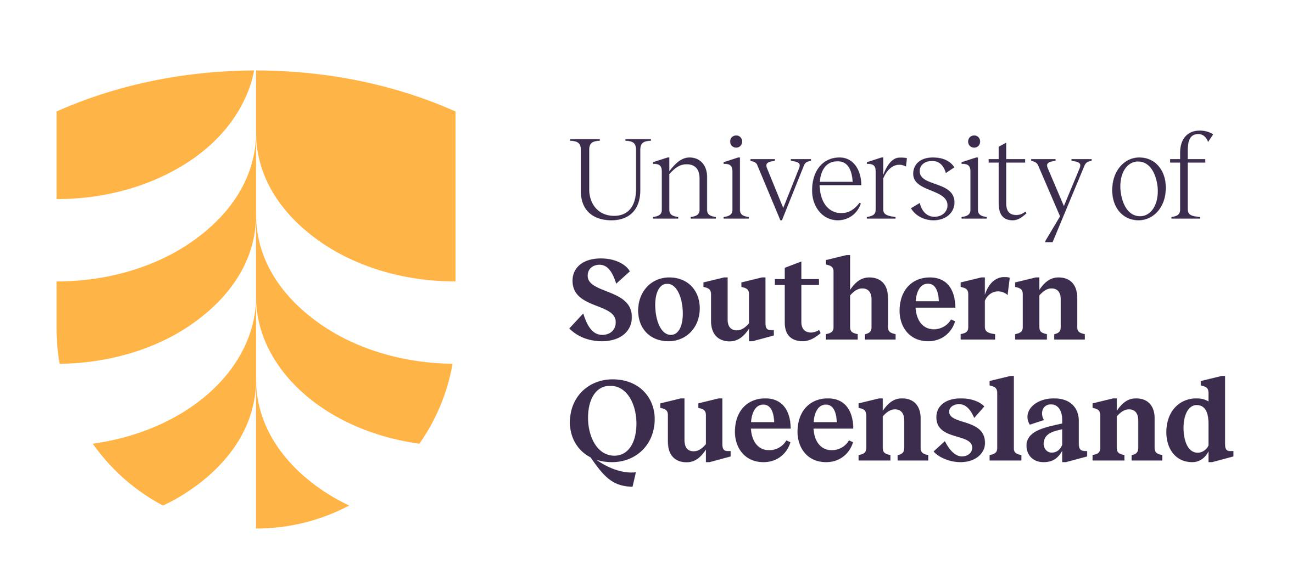
Want to create or adapt books like this? Learn more about how Pressbooks supports open publishing practices.
10 Experimental research
Experimental research—often considered to be the ‘gold standard’ in research designs—is one of the most rigorous of all research designs. In this design, one or more independent variables are manipulated by the researcher (as treatments), subjects are randomly assigned to different treatment levels (random assignment), and the results of the treatments on outcomes (dependent variables) are observed. The unique strength of experimental research is its internal validity (causality) due to its ability to link cause and effect through treatment manipulation, while controlling for the spurious effect of extraneous variable.
Experimental research is best suited for explanatory research—rather than for descriptive or exploratory research—where the goal of the study is to examine cause-effect relationships. It also works well for research that involves a relatively limited and well-defined set of independent variables that can either be manipulated or controlled. Experimental research can be conducted in laboratory or field settings. Laboratory experiments , conducted in laboratory (artificial) settings, tend to be high in internal validity, but this comes at the cost of low external validity (generalisability), because the artificial (laboratory) setting in which the study is conducted may not reflect the real world. Field experiments are conducted in field settings such as in a real organisation, and are high in both internal and external validity. But such experiments are relatively rare, because of the difficulties associated with manipulating treatments and controlling for extraneous effects in a field setting.
Experimental research can be grouped into two broad categories: true experimental designs and quasi-experimental designs. Both designs require treatment manipulation, but while true experiments also require random assignment, quasi-experiments do not. Sometimes, we also refer to non-experimental research, which is not really a research design, but an all-inclusive term that includes all types of research that do not employ treatment manipulation or random assignment, such as survey research, observational research, and correlational studies.
Basic concepts
Treatment and control groups. In experimental research, some subjects are administered one or more experimental stimulus called a treatment (the treatment group ) while other subjects are not given such a stimulus (the control group ). The treatment may be considered successful if subjects in the treatment group rate more favourably on outcome variables than control group subjects. Multiple levels of experimental stimulus may be administered, in which case, there may be more than one treatment group. For example, in order to test the effects of a new drug intended to treat a certain medical condition like dementia, if a sample of dementia patients is randomly divided into three groups, with the first group receiving a high dosage of the drug, the second group receiving a low dosage, and the third group receiving a placebo such as a sugar pill (control group), then the first two groups are experimental groups and the third group is a control group. After administering the drug for a period of time, if the condition of the experimental group subjects improved significantly more than the control group subjects, we can say that the drug is effective. We can also compare the conditions of the high and low dosage experimental groups to determine if the high dose is more effective than the low dose.
Treatment manipulation. Treatments are the unique feature of experimental research that sets this design apart from all other research methods. Treatment manipulation helps control for the ‘cause’ in cause-effect relationships. Naturally, the validity of experimental research depends on how well the treatment was manipulated. Treatment manipulation must be checked using pretests and pilot tests prior to the experimental study. Any measurements conducted before the treatment is administered are called pretest measures , while those conducted after the treatment are posttest measures .
Random selection and assignment. Random selection is the process of randomly drawing a sample from a population or a sampling frame. This approach is typically employed in survey research, and ensures that each unit in the population has a positive chance of being selected into the sample. Random assignment, however, is a process of randomly assigning subjects to experimental or control groups. This is a standard practice in true experimental research to ensure that treatment groups are similar (equivalent) to each other and to the control group prior to treatment administration. Random selection is related to sampling, and is therefore more closely related to the external validity (generalisability) of findings. However, random assignment is related to design, and is therefore most related to internal validity. It is possible to have both random selection and random assignment in well-designed experimental research, but quasi-experimental research involves neither random selection nor random assignment.
Threats to internal validity. Although experimental designs are considered more rigorous than other research methods in terms of the internal validity of their inferences (by virtue of their ability to control causes through treatment manipulation), they are not immune to internal validity threats. Some of these threats to internal validity are described below, within the context of a study of the impact of a special remedial math tutoring program for improving the math abilities of high school students.
History threat is the possibility that the observed effects (dependent variables) are caused by extraneous or historical events rather than by the experimental treatment. For instance, students’ post-remedial math score improvement may have been caused by their preparation for a math exam at their school, rather than the remedial math program.
Maturation threat refers to the possibility that observed effects are caused by natural maturation of subjects (e.g., a general improvement in their intellectual ability to understand complex concepts) rather than the experimental treatment.
Testing threat is a threat in pre-post designs where subjects’ posttest responses are conditioned by their pretest responses. For instance, if students remember their answers from the pretest evaluation, they may tend to repeat them in the posttest exam.
Not conducting a pretest can help avoid this threat.
Instrumentation threat , which also occurs in pre-post designs, refers to the possibility that the difference between pretest and posttest scores is not due to the remedial math program, but due to changes in the administered test, such as the posttest having a higher or lower degree of difficulty than the pretest.
Mortality threat refers to the possibility that subjects may be dropping out of the study at differential rates between the treatment and control groups due to a systematic reason, such that the dropouts were mostly students who scored low on the pretest. If the low-performing students drop out, the results of the posttest will be artificially inflated by the preponderance of high-performing students.
Regression threat —also called a regression to the mean—refers to the statistical tendency of a group’s overall performance to regress toward the mean during a posttest rather than in the anticipated direction. For instance, if subjects scored high on a pretest, they will have a tendency to score lower on the posttest (closer to the mean) because their high scores (away from the mean) during the pretest were possibly a statistical aberration. This problem tends to be more prevalent in non-random samples and when the two measures are imperfectly correlated.
Two-group experimental designs

Pretest-posttest control group design . In this design, subjects are randomly assigned to treatment and control groups, subjected to an initial (pretest) measurement of the dependent variables of interest, the treatment group is administered a treatment (representing the independent variable of interest), and the dependent variables measured again (posttest). The notation of this design is shown in Figure 10.1.

Statistical analysis of this design involves a simple analysis of variance (ANOVA) between the treatment and control groups. The pretest-posttest design handles several threats to internal validity, such as maturation, testing, and regression, since these threats can be expected to influence both treatment and control groups in a similar (random) manner. The selection threat is controlled via random assignment. However, additional threats to internal validity may exist. For instance, mortality can be a problem if there are differential dropout rates between the two groups, and the pretest measurement may bias the posttest measurement—especially if the pretest introduces unusual topics or content.
Posttest -only control group design . This design is a simpler version of the pretest-posttest design where pretest measurements are omitted. The design notation is shown in Figure 10.2.

The treatment effect is measured simply as the difference in the posttest scores between the two groups:
![Rendered by QuickLaTeX.com \[E = (O_{1} - O_{2})\,.\]](https://usq.pressbooks.pub/app/uploads/quicklatex/quicklatex.com-90f2ce47275e7b35def5c5b52b7d7d45_l3.png)
The appropriate statistical analysis of this design is also a two-group analysis of variance (ANOVA). The simplicity of this design makes it more attractive than the pretest-posttest design in terms of internal validity. This design controls for maturation, testing, regression, selection, and pretest-posttest interaction, though the mortality threat may continue to exist.

Because the pretest measure is not a measurement of the dependent variable, but rather a covariate, the treatment effect is measured as the difference in the posttest scores between the treatment and control groups as:
Due to the presence of covariates, the right statistical analysis of this design is a two-group analysis of covariance (ANCOVA). This design has all the advantages of posttest-only design, but with internal validity due to the controlling of covariates. Covariance designs can also be extended to pretest-posttest control group design.
Factorial designs
Two-group designs are inadequate if your research requires manipulation of two or more independent variables (treatments). In such cases, you would need four or higher-group designs. Such designs, quite popular in experimental research, are commonly called factorial designs. Each independent variable in this design is called a factor , and each subdivision of a factor is called a level . Factorial designs enable the researcher to examine not only the individual effect of each treatment on the dependent variables (called main effects), but also their joint effect (called interaction effects).

In a factorial design, a main effect is said to exist if the dependent variable shows a significant difference between multiple levels of one factor, at all levels of other factors. No change in the dependent variable across factor levels is the null case (baseline), from which main effects are evaluated. In the above example, you may see a main effect of instructional type, instructional time, or both on learning outcomes. An interaction effect exists when the effect of differences in one factor depends upon the level of a second factor. In our example, if the effect of instructional type on learning outcomes is greater for three hours/week of instructional time than for one and a half hours/week, then we can say that there is an interaction effect between instructional type and instructional time on learning outcomes. Note that the presence of interaction effects dominate and make main effects irrelevant, and it is not meaningful to interpret main effects if interaction effects are significant.
Hybrid experimental designs
Hybrid designs are those that are formed by combining features of more established designs. Three such hybrid designs are randomised bocks design, Solomon four-group design, and switched replications design.
Randomised block design. This is a variation of the posttest-only or pretest-posttest control group design where the subject population can be grouped into relatively homogeneous subgroups (called blocks ) within which the experiment is replicated. For instance, if you want to replicate the same posttest-only design among university students and full-time working professionals (two homogeneous blocks), subjects in both blocks are randomly split between the treatment group (receiving the same treatment) and the control group (see Figure 10.5). The purpose of this design is to reduce the ‘noise’ or variance in data that may be attributable to differences between the blocks so that the actual effect of interest can be detected more accurately.
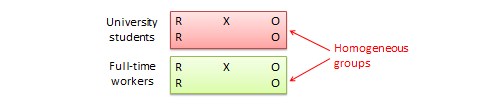
Solomon four-group design . In this design, the sample is divided into two treatment groups and two control groups. One treatment group and one control group receive the pretest, and the other two groups do not. This design represents a combination of posttest-only and pretest-posttest control group design, and is intended to test for the potential biasing effect of pretest measurement on posttest measures that tends to occur in pretest-posttest designs, but not in posttest-only designs. The design notation is shown in Figure 10.6.
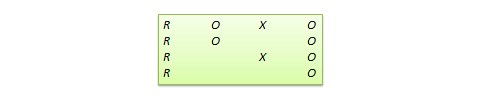
Switched replication design . This is a two-group design implemented in two phases with three waves of measurement. The treatment group in the first phase serves as the control group in the second phase, and the control group in the first phase becomes the treatment group in the second phase, as illustrated in Figure 10.7. In other words, the original design is repeated or replicated temporally with treatment/control roles switched between the two groups. By the end of the study, all participants will have received the treatment either during the first or the second phase. This design is most feasible in organisational contexts where organisational programs (e.g., employee training) are implemented in a phased manner or are repeated at regular intervals.

Quasi-experimental designs
Quasi-experimental designs are almost identical to true experimental designs, but lacking one key ingredient: random assignment. For instance, one entire class section or one organisation is used as the treatment group, while another section of the same class or a different organisation in the same industry is used as the control group. This lack of random assignment potentially results in groups that are non-equivalent, such as one group possessing greater mastery of certain content than the other group, say by virtue of having a better teacher in a previous semester, which introduces the possibility of selection bias . Quasi-experimental designs are therefore inferior to true experimental designs in interval validity due to the presence of a variety of selection related threats such as selection-maturation threat (the treatment and control groups maturing at different rates), selection-history threat (the treatment and control groups being differentially impacted by extraneous or historical events), selection-regression threat (the treatment and control groups regressing toward the mean between pretest and posttest at different rates), selection-instrumentation threat (the treatment and control groups responding differently to the measurement), selection-testing (the treatment and control groups responding differently to the pretest), and selection-mortality (the treatment and control groups demonstrating differential dropout rates). Given these selection threats, it is generally preferable to avoid quasi-experimental designs to the greatest extent possible.

In addition, there are quite a few unique non-equivalent designs without corresponding true experimental design cousins. Some of the more useful of these designs are discussed next.
Regression discontinuity (RD) design . This is a non-equivalent pretest-posttest design where subjects are assigned to the treatment or control group based on a cut-off score on a preprogram measure. For instance, patients who are severely ill may be assigned to a treatment group to test the efficacy of a new drug or treatment protocol and those who are mildly ill are assigned to the control group. In another example, students who are lagging behind on standardised test scores may be selected for a remedial curriculum program intended to improve their performance, while those who score high on such tests are not selected from the remedial program.

Because of the use of a cut-off score, it is possible that the observed results may be a function of the cut-off score rather than the treatment, which introduces a new threat to internal validity. However, using the cut-off score also ensures that limited or costly resources are distributed to people who need them the most, rather than randomly across a population, while simultaneously allowing a quasi-experimental treatment. The control group scores in the RD design do not serve as a benchmark for comparing treatment group scores, given the systematic non-equivalence between the two groups. Rather, if there is no discontinuity between pretest and posttest scores in the control group, but such a discontinuity persists in the treatment group, then this discontinuity is viewed as evidence of the treatment effect.
Proxy pretest design . This design, shown in Figure 10.11, looks very similar to the standard NEGD (pretest-posttest) design, with one critical difference: the pretest score is collected after the treatment is administered. A typical application of this design is when a researcher is brought in to test the efficacy of a program (e.g., an educational program) after the program has already started and pretest data is not available. Under such circumstances, the best option for the researcher is often to use a different prerecorded measure, such as students’ grade point average before the start of the program, as a proxy for pretest data. A variation of the proxy pretest design is to use subjects’ posttest recollection of pretest data, which may be subject to recall bias, but nevertheless may provide a measure of perceived gain or change in the dependent variable.

Separate pretest-posttest samples design . This design is useful if it is not possible to collect pretest and posttest data from the same subjects for some reason. As shown in Figure 10.12, there are four groups in this design, but two groups come from a single non-equivalent group, while the other two groups come from a different non-equivalent group. For instance, say you want to test customer satisfaction with a new online service that is implemented in one city but not in another. In this case, customers in the first city serve as the treatment group and those in the second city constitute the control group. If it is not possible to obtain pretest and posttest measures from the same customers, you can measure customer satisfaction at one point in time, implement the new service program, and measure customer satisfaction (with a different set of customers) after the program is implemented. Customer satisfaction is also measured in the control group at the same times as in the treatment group, but without the new program implementation. The design is not particularly strong, because you cannot examine the changes in any specific customer’s satisfaction score before and after the implementation, but you can only examine average customer satisfaction scores. Despite the lower internal validity, this design may still be a useful way of collecting quasi-experimental data when pretest and posttest data is not available from the same subjects.
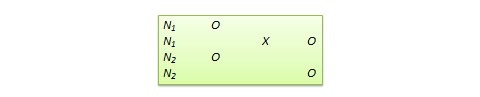
An interesting variation of the NEDV design is a pattern-matching NEDV design , which employs multiple outcome variables and a theory that explains how much each variable will be affected by the treatment. The researcher can then examine if the theoretical prediction is matched in actual observations. This pattern-matching technique—based on the degree of correspondence between theoretical and observed patterns—is a powerful way of alleviating internal validity concerns in the original NEDV design.

Perils of experimental research
Experimental research is one of the most difficult of research designs, and should not be taken lightly. This type of research is often best with a multitude of methodological problems. First, though experimental research requires theories for framing hypotheses for testing, much of current experimental research is atheoretical. Without theories, the hypotheses being tested tend to be ad hoc, possibly illogical, and meaningless. Second, many of the measurement instruments used in experimental research are not tested for reliability and validity, and are incomparable across studies. Consequently, results generated using such instruments are also incomparable. Third, often experimental research uses inappropriate research designs, such as irrelevant dependent variables, no interaction effects, no experimental controls, and non-equivalent stimulus across treatment groups. Findings from such studies tend to lack internal validity and are highly suspect. Fourth, the treatments (tasks) used in experimental research may be diverse, incomparable, and inconsistent across studies, and sometimes inappropriate for the subject population. For instance, undergraduate student subjects are often asked to pretend that they are marketing managers and asked to perform a complex budget allocation task in which they have no experience or expertise. The use of such inappropriate tasks, introduces new threats to internal validity (i.e., subject’s performance may be an artefact of the content or difficulty of the task setting), generates findings that are non-interpretable and meaningless, and makes integration of findings across studies impossible.
The design of proper experimental treatments is a very important task in experimental design, because the treatment is the raison d’etre of the experimental method, and must never be rushed or neglected. To design an adequate and appropriate task, researchers should use prevalidated tasks if available, conduct treatment manipulation checks to check for the adequacy of such tasks (by debriefing subjects after performing the assigned task), conduct pilot tests (repeatedly, if necessary), and if in doubt, use tasks that are simple and familiar for the respondent sample rather than tasks that are complex or unfamiliar.
In summary, this chapter introduced key concepts in the experimental design research method and introduced a variety of true experimental and quasi-experimental designs. Although these designs vary widely in internal validity, designs with less internal validity should not be overlooked and may sometimes be useful under specific circumstances and empirical contingencies.
Social Science Research: Principles, Methods and Practices (Revised edition) Copyright © 2019 by Anol Bhattacherjee is licensed under a Creative Commons Attribution-NonCommercial-ShareAlike 4.0 International License , except where otherwise noted.
Share This Book
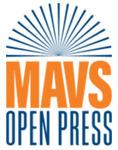
Want to create or adapt books like this? Learn more about how Pressbooks supports open publishing practices.
14.1 What is experimental design and when should you use it?
Learning objectives.
Learners will be able to…
- Describe the purpose of experimental design research
- Describe nomethetic causality and the logic of experimental design
- Identify the characteristics of a basic experiment
- Discuss the relationship between dependent and independent variables in experiments
- Identify the three major types of experimental designs
Pre-awareness check (Knowledge)
What are your thoughts on the phrase ‘experiment’ in the realm of social sciences? In an experiment, what is the independent variable?
The basics of experiments
In social work research, experimental design is used to test the effects of treatments, interventions, programs, or other conditions to which individuals, groups, organizations, or communities may be exposed to. There are a lot of experiments social work researchers can use to explore topics such as treatments for depression, impacts of school-based mental health on student outcomes, or prevention of abuse of people with disabilities. The American Psychological Association defines an experiment as:
a series of observations conducted under controlled conditions to study a relationship with the purpose of drawing causal inferences about that relationship. An experiment involves the manipulation of an independent variable , the measurement of a dependent variable , and the exposure of various participants to one or more of the conditions being studied. Random selection of participants and their random assignment to conditions also are necessary in experiments .
In experimental design, the independent variable is the intervention, treatment, or condition that is being investigated as a potential cause of change (i.e., the experimental condition ). The effect, or outcome, of the experimental condition is the dependent variable. Trying out a new restaurant, dating a new person – we often call these things “experiments.” However, a true social science experiment would include recruitment of a large enough sample, random assignment to control and experimental groups, exposing those in the experimental group to an experimental condition, and collecting observations at the end of the experiment.
Social scientists use this level of rigor and control to maximize the internal validity of their research. Internal validity is the confidence researchers have about whether the independent variable (e.g, treatment) truly produces a change in the dependent, or outcome, variable. The logic and features of experimental design are intended to help establish causality and to reduce threats to internal validity , which we will discuss in Section 14.5 .
Experiments attempt to establish a nomothetic causal relationship between two variables—the treatment and its intended outcome. We discussed the four criteria for establishing nomothetic causality in Section 4.3 :
- plausibility,
- covariation,
- temporality, and
- nonspuriousness.
Experiments should establish plausibility , having a plausible reason why their intervention would cause changes in the dependent variable. Usually, a theory framework or previous empirical evidence will indicate the plausibility of a causal relationship.
Covariation can be established for causal explanations by showing that the “cause” and the “effect” change together. In experiments, the cause is an intervention, treatment, or other experimental condition. Whether or not a research participant is exposed to the experimental condition is the independent variable. The effect in an experiment is the outcome being assessed and is the dependent variable in the study. When the independent and dependent variables covary, they can have a positive association (e.g., those exposed to the intervention have increased self-esteem) or a negative association (e.g., those exposed to the intervention have reduced anxiety).
Since researcher controls when the intervention is administered, they can be assured that changes in the independent variable (the treatment) happens before changes in the dependent variable (the outcome). In this way, experiments assure temporality .
Finally, one of the most important features of experiments is that they allow researchers to eliminate spurious variables to support the criterion of nonspuriousness . True experiments are usually conducted under strictly controlled conditions. The intervention is given in the same way to each person, with a minimal number of other variables that might cause their post-test scores to change.
The logic of experimental design
How do we know that one phenomenon causes another? The complexity of the social world in which we practice and conduct research means that causes of social problems are rarely cut and dry. Uncovering explanations for social problems is key to helping clients address them, and experimental research designs are one road to finding answers.
Just because two phenomena are related in some way doesn’t mean that one causes the other. Ice cream sales increase in the summer, and so does the rate of violent crime; does that mean that eating ice cream is going to make me violent? Obviously not, because ice cream is great. The reality of that association is far more complex—it could be that hot weather makes people more irritable and, at times, violent, while also making people want ice cream. More likely, though, there are other social factors not accounted for in the way we just described this association.
As we have discussed, experimental designs can help clear up at least some of this fog by allowing researchers to isolate the effect of interventions on dependent variables by controlling extraneous variables . In true experimental design (discussed in the next section) and quasi-experimental design, researchers accomplish this w ith a control group or comparison group and the experimental group . The experimental group is sometimes called the treatment group because people in the experimental group receive the treatment or are exposed to the experimental condition (but we will call it the experimental group in this chapter.) The control/comparison group does not receive the treatment or intervention. Instead they may receive what is known as “treatment as usual” or perhaps no treatment at all.
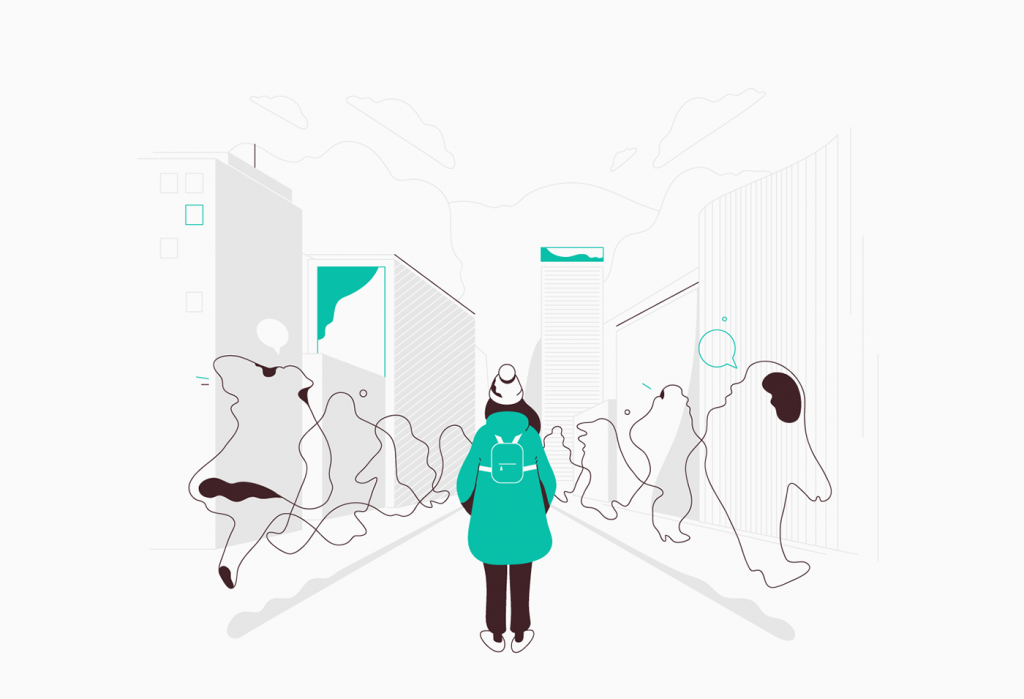
In a well-designed experiment, the control group should look almost identical to the experimental group in terms of demographics and other relevant factors. What if we want to know the effect of CBT on social anxiety, but we have learned in prior research that men tend to have a more difficult time overcoming social anxiety? We would want our control and experimental groups to have a similar portions of men, since ostensibly, both groups’ results would be affected by the men in the group. If your control group has 5 women, 6 men, and 4 non-binary people, then your experimental group should be made up of roughly the same gender balance to help control for the influence of gender on the outcome of your intervention. (In reality, the groups should be similar along other dimensions, as well, and your group will likely be much larger.) The researcher will use the same outcome measures for both groups and compare them, and assuming the experiment was designed correctly, get a pretty good answer about whether the intervention had an effect on social anxiety.
Random assignment [/pb_glossary], also called randomization, entails using a random process to decide which participants are put into the control or experimental group (which participants receive an intervention and which do not). By randomly assigning participants to a group, you can reduce the effect of extraneous variables on your research because there won’t be a systematic difference between the groups.
Do not confuse random assignment with random sampling . Random sampling is a method for selecting a sample from a population and is rarely used in psychological research. Random assignment is a method for assigning participants in a sample to the different conditions, and it is an important element of all experimental research in psychology and other related fields. Random sampling helps a great deal with external validity, or generalizability , whereas random assignment increases internal validity .
Other Features of Experiments that Help Establish Causality
To control for spuriousness (as well as meeting the three other criteria for establishing causality), experiments try to control as many aspects of the research process as possible: using control groups, having large enough sample sizes, standardizing the treatment, etc. Researchers in large experiments often employ clinicians or other research staff to help them. Researchers train their staff members exhaustively, provide pre-scripted responses to common questions, and control the physical environment of the experiment so each person who participates receives the exact same treatment. Experimental researchers also document their procedures, so that others can review them and make changes in future research if they think it will improve on the ability to control for spurious variables.
An interesting example is Bruce Alexander’s (2010) Rat Park experiments. Much of the early research conducted on addictive drugs, like heroin and cocaine, was conducted on animals other than humans, usually mice or rats. The scientific consensus up until Alexander’s experiments was that cocaine and heroin were so addictive that rats, if offered the drugs, would consume them repeatedly until they perished. Researchers claimed this behavior explained how addiction worked in humans, but Alexander was not so sure. He knew rats were social animals and the experimental procedure from previous experiments did not allow them to socialize. Instead, rats were kept isolated in small cages with only food, water, and metal walls. To Alexander, social isolation was a spurious variable, causing changes in addictive behavior not due to the drug itself. Alexander created an experiment of his own, in which rats were allowed to run freely in an interesting environment, socialize and mate with other rats, and of course, drink from a solution that contained an addictive drug. In this environment, rats did not become hopelessly addicted to drugs. In fact, they had little interest in the substance. To Alexander, the results of his experiment demonstrated that social isolation was more of a causal factor for addiction than the drug itself.
One challenge with Alexander’s findings is that subsequent researchers have had mixed success replicating his findings (e.g., Petrie, 1996; Solinas, Thiriet, El Rawas, Lardeux, & Jaber, 2009). Replication involves conducting another researcher’s experiment in the same manner and seeing if it produces the same results. If the causal relationship is real, it should occur in all (or at least most) rigorous replications of the experiment.
Replicability
[INSERT A PARAGRAPH ABOUT REPLICATION/REPRODUCTION HERE. CAN USE/REFERENCE THIS IF IT’S HELPFUL; include glossary definition as well as other general info]
To allow for easier replication, researchers should describe their experimental methods diligently. Researchers with the Open Science Collaboration (2015) [1] conducted the Reproducibility Project , which caused a significant controversy regarding the validity of psychological studies. The researchers with the project attempted to reproduce the results of 100 experiments published in major psychology journals since 2008. What they found was shocking. Although 97% of the original studies reported significant results, only 36% of the replicated studies had significant findings. The average effect size in the replication studies was half that of the original studies. The implications of the Reproducibility Project are potentially staggering, and encourage social scientists to carefully consider the validity of their reported findings and that the scientific community take steps to ensure researchers do not cherry-pick data or change their hypotheses simply to get published.
Generalizability
Let’s return to Alexander’s Rat Park study and consider the implications of his experiment for substance use professionals. The conclusions he drew from his experiments on rats were meant to be generalized to the population. If this could be done, the experiment would have a high degree of external validity , which is the degree to which conclusions generalize to larger populations and different situations. Alexander argues his conclusions about addiction and social isolation help us understand why people living in deprived, isolated environments may become addicted to drugs more often than those in more enriching environments. Similarly, earlier rat researchers argued their results showed these drugs were instantly addictive to humans, often to the point of death.
Neither study’s results will match up perfectly with real life. There are clients in social work practice who may fit into Alexander’s social isolation model, but social isolation is complex. Clients can live in environments with other sociable humans, work jobs, and have romantic relationships; does this mean they are not socially isolated? On the other hand, clients may face structural racism, poverty, trauma, and other challenges that may contribute to their social environment. Alexander’s work helps understand clients’ experiences, but the explanation is incomplete. Human existence is more complicated than the experimental conditions in Rat Park.
Effectiveness versus Efficacy
Social workers are especially attentive to how social context shapes social life. This consideration points out a potential weakness of experiments. They can be rather artificial. When an experiment demonstrates causality under ideal, controlled circumstances, it establishes the efficacy of an intervention.
How often do real-world social interactions occur in the same way that they do in a controlled experiment? Experiments that are conducted in community settings by community practitioners are less easily controlled than those conducted in a lab or with researchers who adhere strictly to research protocols delivering the intervention. When an experiment demonstrates causality in a real-world setting that is not tightly controlled, it establishes the effectiveness of the intervention.
The distinction between efficacy and effectiveness demonstrates the tension between internal and external validity. Internal validity and external validity are conceptually linked. Internal validity refers to the degree to which the intervention causes its intended outcomes, and external validity refers to how well that relationship applies to different groups and circumstances than the experiment. However, the more researchers tightly control the environment to ensure internal validity, the more they may risk external validity for generalizing their results to different populations and circumstances. Correspondingly, researchers whose settings are just like the real world will be less able to ensure internal validity, as there are many factors that could pollute the research process. This is not to suggest that experimental research findings cannot have high levels of both internal and external validity, but that experimental researchers must always be aware of this potential weakness and clearly report limitations in their research reports.
Types of Experimental Designs
Experimental design is an umbrella term for a research method that is designed to test hypotheses related to causality under controlled conditions. Table 14.1 describes the three major types of experimental design (pre-experimental, quasi-experimental, and true experimental) and presents subtypes for each. As we will see in the coming sections, some types of experimental design are better at establishing causality than others. It’s also worth considering that true experiments, which most effectively establish causality , are often difficult and expensive to implement. Although the other experimental designs aren’t perfect, they still produce useful, valid evidence and may be more feasible to carry out.
) | ||
A. One-group pretest posttest | A. Pre- and posttests are administered, but no comparison group | XXXX |
B. One-shot case study | B. No pretest | What is the average level of loneliness among graduates of a peer support training program? What percent of graduates rate their social support as “good” or “excellent”? |
) | ||
C. Nonequivalent comparison group design | C. Similar to classical experimental design only without random assignment | XXXX |
D. Static-group design | D. No pretest, posttest administered after the intervention
| |
E. Natural experiments | E. Naturally occurring event becomes “experimental condition”; observational study in which some cases are exposed to condition (which becomes the “experimental condition”) and others are not; changes in “experimental” group can be assessed; | |
( ) | XXXX | |
F. Classical experimental design | F. Pre- and posttest; control group | |
G. Posttest only control group | G. Does not use a pretest and assumes random assignment results in equivalent groups | |
H. Solomon four group design | H. Random assignment, two experimental and two control groups, pretests for half of the groups and posttests for all |
Key Takeaways
- Experimental designs are useful for establishing causality, but some types of experimental design do this better than others.
- Experiments help researchers isolate the effect of the independent variable on the dependent variable by controlling for the effect of extraneous variables .
- Experiments use a control/comparison group and an experimental group to test the effects of interventions. These groups should be as similar to each other as possible in terms of demographics and other relevant factors.
- True experiments have control groups with randomly assigned participants; quasi-experimental types of experiments have comparison groups to which participants are not randomly assigned; pre-experimental designs do not have a comparison group.
TRACK 1 (IF YOU ARE CREATING A RESEARCH PROPOSAL FOR THIS CLASS):
- Think about the research project you’ve been designing so far. How might you use a basic experiment to answer your question? If your question isn’t explanatory, try to formulate a new explanatory question and consider the usefulness of an experiment.
- Why is establishing a simple relationship between two variables not indicative of one causing the other?
TRACK 2 (IF YOU AREN’T CREATING A RESEARCH PROPOSAL FOR THIS CLASS):
Imagine you are interested in studying child welfare practice. You are interested in learning more about community-based programs aimed to prevent child maltreatment and to prevent out-of-home placement for children.
- Think about the research project stated above. How might you use a basic experiment to look more into this research topic? Try to formulate an explanatory question and consider the usefulness of an experiment.
- Open Science Collaboration. (2015). Estimating the reproducibility of psychological science. Science, 349 (6251), aac4716. Doi: 10.1126/science.aac4716 ↵
an operation or procedure carried out under controlled conditions in order to discover an unknown effect or law, to test or establish a hypothesis, or to illustrate a known law.
treatment, intervention, or experience that is being tested in an experiment (the independent variable) that is received by the experimental group and not by the control group.
Ability to say that one variable "causes" something to happen to another variable. Very important to assess when thinking about studies that examine causation such as experimental or quasi-experimental designs.
circumstances or events that may affect the outcome of an experiment, resulting in changes in the research participants that are not a result of the intervention, treatment, or experimental condition being tested
causal explanations that can be universally applied to groups, such as scientific laws or universal truths
as a criteria for causal relationship, the relationship must make logical sense and seem possible
when the values of two variables change at the same time
as a criteria for causal relationship, the cause must come before the effect
an association between two variables that is NOT caused by a third variable
variables and characteristics that have an effect on your outcome, but aren't the primary variable whose influence you're interested in testing.
the group of participants in our study who do not receive the intervention we are researching in experiments with random assignment
the group of participants in our study who do not receive the intervention we are researching in experiments without random assignment
in experimental design, the group of participants in our study who do receive the intervention we are researching
The ability to apply research findings beyond the study sample to some broader population,
This is a synonymous term for generalizability - the ability to apply the findings of a study beyond the sample to a broader population.
performance of an intervention under ideal and controlled circumstances, such as in a lab or delivered by trained researcher-interventionists
The performance of an intervention under "real-world" conditions that are not closely controlled and ideal
the idea that one event, behavior, or belief will result in the occurrence of another, subsequent event, behavior, or belief
Doctoral Research Methods in Social Work Copyright © by Mavs Open Press. All Rights Reserved.
Share This Book
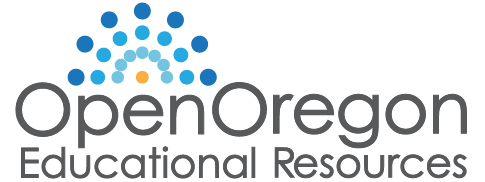
21 13. Experimental design
Chapter outline.
- What is an experiment and when should you use one? (8 minute read)
- True experimental designs (7 minute read)
- Quasi-experimental designs (8 minute read)
- Non-experimental designs (5 minute read)
- Critical, ethical, and critical considerations (5 minute read)
Content warning : examples in this chapter contain references to non-consensual research in Western history, including experiments conducted during the Holocaust and on African Americans (section 13.6).
13.1 What is an experiment and when should you use one?
Learning objectives.
Learners will be able to…
- Identify the characteristics of a basic experiment
- Describe causality in experimental design
- Discuss the relationship between dependent and independent variables in experiments
- Explain the links between experiments and generalizability of results
- Describe advantages and disadvantages of experimental designs
The basics of experiments
The first experiment I can remember using was for my fourth grade science fair. I wondered if latex- or oil-based paint would hold up to sunlight better. So, I went to the hardware store and got a few small cans of paint and two sets of wooden paint sticks. I painted one with oil-based paint and the other with latex-based paint of different colors and put them in a sunny spot in the back yard. My hypothesis was that the oil-based paint would fade the most and that more fading would happen the longer I left the paint sticks out. (I know, it’s obvious, but I was only 10.)
I checked in on the paint sticks every few days for a month and wrote down my observations. The first part of my hypothesis ended up being wrong—it was actually the latex-based paint that faded the most. But the second part was right, and the paint faded more and more over time. This is a simple example, of course—experiments get a heck of a lot more complex than this when we’re talking about real research.
Merriam-Webster defines an experiment as “an operation or procedure carried out under controlled conditions in order to discover an unknown effect or law, to test or establish a hypothesis, or to illustrate a known law.” Each of these three components of the definition will come in handy as we go through the different types of experimental design in this chapter. Most of us probably think of the physical sciences when we think of experiments, and for good reason—these experiments can be pretty flashy! But social science and psychological research follow the same scientific methods, as we’ve discussed in this book.
As the video discusses, experiments can be used in social sciences just like they can in physical sciences. It makes sense to use an experiment when you want to determine the cause of a phenomenon with as much accuracy as possible. Some types of experimental designs do this more precisely than others, as we’ll see throughout the chapter. If you’ll remember back to Chapter 11 and the discussion of validity, experiments are the best way to ensure internal validity, or the extent to which a change in your independent variable causes a change in your dependent variable.
Experimental designs for research projects are most appropriate when trying to uncover or test a hypothesis about the cause of a phenomenon, so they are best for explanatory research questions. As we’ll learn throughout this chapter, different circumstances are appropriate for different types of experimental designs. Each type of experimental design has advantages and disadvantages, and some are better at controlling the effect of extraneous variables —those variables and characteristics that have an effect on your dependent variable, but aren’t the primary variable whose influence you’re interested in testing. For example, in a study that tries to determine whether aspirin lowers a person’s risk of a fatal heart attack, a person’s race would likely be an extraneous variable because you primarily want to know the effect of aspirin.
In practice, many types of experimental designs can be logistically challenging and resource-intensive. As practitioners, the likelihood that we will be involved in some of the types of experimental designs discussed in this chapter is fairly low. However, it’s important to learn about these methods, even if we might not ever use them, so that we can be thoughtful consumers of research that uses experimental designs.
While we might not use all of these types of experimental designs, many of us will engage in evidence-based practice during our time as social workers. A lot of research developing evidence-based practice, which has a strong emphasis on generalizability, will use experimental designs. You’ve undoubtedly seen one or two in your literature search so far.
The logic of experimental design
How do we know that one phenomenon causes another? The complexity of the social world in which we practice and conduct research means that causes of social problems are rarely cut and dry. Uncovering explanations for social problems is key to helping clients address them, and experimental research designs are one road to finding answers.
As you read about in Chapter 8 (and as we’ll discuss again in Chapter 15 ), just because two phenomena are related in some way doesn’t mean that one causes the other. Ice cream sales increase in the summer, and so does the rate of violent crime; does that mean that eating ice cream is going to make me murder someone? Obviously not, because ice cream is great. The reality of that relationship is far more complex—it could be that hot weather makes people more irritable and, at times, violent, while also making people want ice cream. More likely, though, there are other social factors not accounted for in the way we just described this relationship.
Experimental designs can help clear up at least some of this fog by allowing researchers to isolate the effect of interventions on dependent variables by controlling extraneous variables . In true experimental design (discussed in the next section) and some quasi-experimental designs, researchers accomplish this w ith the control group and the experimental group . (The experimental group is sometimes called the “treatment group,” but we will call it the experimental group in this chapter.) The control group does not receive the intervention you are testing (they may receive no intervention or what is known as “treatment as usual”), while the experimental group does. (You will hopefully remember our earlier discussion of control variables in Chapter 8 —conceptually, the use of the word “control” here is the same.)
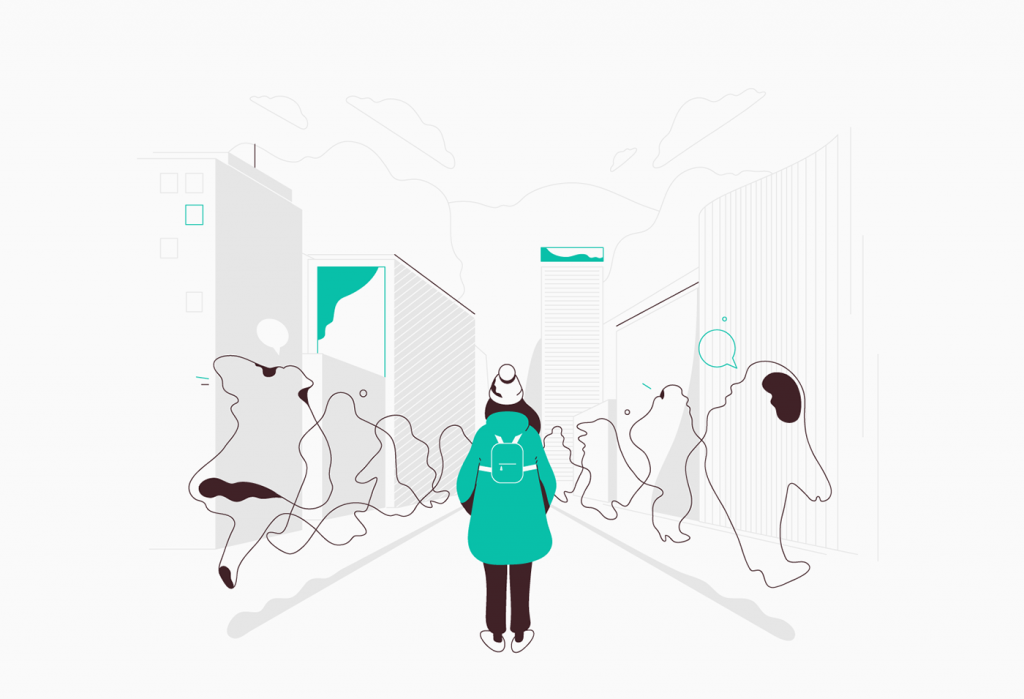
In a well-designed experiment, your control group should look almost identical to your experimental group in terms of demographics and other relevant factors. What if we want to know the effect of CBT on social anxiety, but we have learned in prior research that men tend to have a more difficult time overcoming social anxiety? We would want our control and experimental groups to have a similar gender mix because it would limit the effect of gender on our results, since ostensibly, both groups’ results would be affected by gender in the same way. If your control group has 5 women, 6 men, and 4 non-binary people, then your experimental group should be made up of roughly the same gender balance to help control for the influence of gender on the outcome of your intervention. (In reality, the groups should be similar along other dimensions, as well, and your group will likely be much larger.) The researcher will use the same outcome measures for both groups and compare them, and assuming the experiment was designed correctly, get a pretty good answer about whether the intervention had an effect on social anxiety.
You will also hear people talk about comparison groups , which are similar to control groups. The primary difference between the two is that a control group is populated using random assignment, but a comparison group is not. Random assignment entails using a random process to decide which participants are put into the control or experimental group (which participants receive an intervention and which do not). By randomly assigning participants to a group, you can reduce the effect of extraneous variables on your research because there won’t be a systematic difference between the groups.
Do not confuse random assignment with random sampling. Random sampling is a method for selecting a sample from a population, and is rarely used in psychological research. Random assignment is a method for assigning participants in a sample to the different conditions, and it is an important element of all experimental research in psychology and other related fields. Random sampling also helps a great deal with generalizability , whereas random assignment increases internal validity .
We have already learned about internal validity in Chapter 11 . The use of an experimental design will bolster internal validity since it works to isolate causal relationships. As we will see in the coming sections, some types of experimental design do this more effectively than others. It’s also worth considering that true experiments, which most effectively show causality , are often difficult and expensive to implement. Although other experimental designs aren’t perfect, they still produce useful, valid evidence and may be more feasible to carry out.
Key Takeaways
- Experimental designs are useful for establishing causality, but some types of experimental design do this better than others.
- Experiments help researchers isolate the effect of the independent variable on the dependent variable by controlling for the effect of extraneous variables .
- Experiments use a control/comparison group and an experimental group to test the effects of interventions. These groups should be as similar to each other as possible in terms of demographics and other relevant factors.
- True experiments have control groups with randomly assigned participants, while other types of experiments have comparison groups to which participants are not randomly assigned.
- Think about the research project you’ve been designing so far. How might you use a basic experiment to answer your question? If your question isn’t explanatory, try to formulate a new explanatory question and consider the usefulness of an experiment.
- Why is establishing a simple relationship between two variables not indicative of one causing the other?
13.2 True experimental design
- Describe a true experimental design in social work research
- Understand the different types of true experimental designs
- Determine what kinds of research questions true experimental designs are suited for
- Discuss advantages and disadvantages of true experimental designs
True experimental design , often considered to be the “gold standard” in research designs, is thought of as one of the most rigorous of all research designs. In this design, one or more independent variables are manipulated by the researcher (as treatments), subjects are randomly assigned to different treatment levels (random assignment), and the results of the treatments on outcomes (dependent variables) are observed. The unique strength of experimental research is its internal validity and its ability to establish ( causality ) through treatment manipulation, while controlling for the effects of extraneous variable. Sometimes the treatment level is no treatment, while other times it is simply a different treatment than that which we are trying to evaluate. For example, we might have a control group that is made up of people who will not receive any treatment for a particular condition. Or, a control group could consist of people who consent to treatment with DBT when we are testing the effectiveness of CBT.
As we discussed in the previous section, a true experiment has a control group with participants randomly assigned , and an experimental group . This is the most basic element of a true experiment. The next decision a researcher must make is when they need to gather data during their experiment. Do they take a baseline measurement and then a measurement after treatment, or just a measurement after treatment, or do they handle measurement another way? Below, we’ll discuss the three main types of true experimental designs. There are sub-types of each of these designs, but here, we just want to get you started with some of the basics.
Using a true experiment in social work research is often pretty difficult, since as I mentioned earlier, true experiments can be quite resource intensive. True experiments work best with relatively large sample sizes, and random assignment, a key criterion for a true experimental design, is hard (and unethical) to execute in practice when you have people in dire need of an intervention. Nonetheless, some of the strongest evidence bases are built on true experiments.
For the purposes of this section, let’s bring back the example of CBT for the treatment of social anxiety. We have a group of 500 individuals who have agreed to participate in our study, and we have randomly assigned them to the control and experimental groups. The folks in the experimental group will receive CBT, while the folks in the control group will receive more unstructured, basic talk therapy. These designs, as we talked about above, are best suited for explanatory research questions.
Before we get started, take a look at the table below. When explaining experimental research designs, we often use diagrams with abbreviations to visually represent the experiment. Table 13.1 starts us off by laying out what each of the abbreviations mean.
R | Randomly assigned group (control/comparison or experimental) |
O | Observation/measurement taken of dependent variable |
X | Intervention or treatment |
X | Experimental or new intervention |
X | Typical intervention/treatment as usual |
A, B, C, etc. | Denotes different groups (control/comparison and experimental) |
Pretest and post-test control group design
In pretest and post-test control group design , participants are given a pretest of some kind to measure their baseline state before their participation in an intervention. In our social anxiety experiment, we would have participants in both the experimental and control groups complete some measure of social anxiety—most likely an established scale and/or a structured interview—before they start their treatment. As part of the experiment, we would have a defined time period during which the treatment would take place (let’s say 12 weeks, just for illustration). At the end of 12 weeks, we would give both groups the same measure as a post-test .
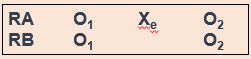
In the diagram, RA (random assignment group A) is the experimental group and RB is the control group. O 1 denotes the pre-test, X e denotes the experimental intervention, and O 2 denotes the post-test. Let’s look at this diagram another way, using the example of CBT for social anxiety that we’ve been talking about.

In a situation where the control group received treatment as usual instead of no intervention, the diagram would look this way, with X i denoting treatment as usual (Figure 13.3).
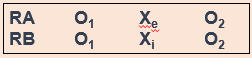
Hopefully, these diagrams provide you a visualization of how this type of experiment establishes time order , a key component of a causal relationship. Did the change occur after the intervention? Assuming there is a change in the scores between the pretest and post-test, we would be able to say that yes, the change did occur after the intervention. Causality can’t exist if the change happened before the intervention—this would mean that something else led to the change, not our intervention.
Post-test only control group design
Post-test only control group design involves only giving participants a post-test, just like it sounds (Figure 13.4).
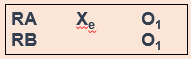
But why would you use this design instead of using a pretest/post-test design? One reason could be the testing effect that can happen when research participants take a pretest. In research, the testing effect refers to “measurement error related to how a test is given; the conditions of the testing, including environmental conditions; and acclimation to the test itself” (Engel & Schutt, 2017, p. 444) [1] (When we say “measurement error,” all we mean is the accuracy of the way we measure the dependent variable.) Figure 13.4 is a visualization of this type of experiment. The testing effect isn’t always bad in practice—our initial assessments might help clients identify or put into words feelings or experiences they are having when they haven’t been able to do that before. In research, however, we might want to control its effects to isolate a cleaner causal relationship between intervention and outcome.
Going back to our CBT for social anxiety example, we might be concerned that participants would learn about social anxiety symptoms by virtue of taking a pretest. They might then identify that they have those symptoms on the post-test, even though they are not new symptoms for them. That could make our intervention look less effective than it actually is.
However, without a baseline measurement establishing causality can be more difficult. If we don’t know someone’s state of mind before our intervention, how do we know our intervention did anything at all? Establishing time order is thus a little more difficult. You must balance this consideration with the benefits of this type of design.
Solomon four group design
One way we can possibly measure how much the testing effect might change the results of the experiment is with the Solomon four group design. Basically, as part of this experiment, you have two control groups and two experimental groups. The first pair of groups receives both a pretest and a post-test. The other pair of groups receives only a post-test (Figure 13.5). This design helps address the problem of establishing time order in post-test only control group designs.
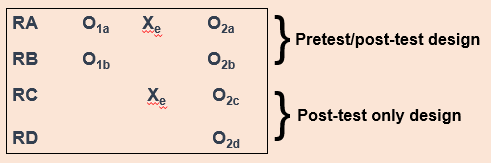
For our CBT project, we would randomly assign people to four different groups instead of just two. Groups A and B would take our pretest measures and our post-test measures, and groups C and D would take only our post-test measures. We could then compare the results among these groups and see if they’re significantly different between the folks in A and B, and C and D. If they are, we may have identified some kind of testing effect, which enables us to put our results into full context. We don’t want to draw a strong causal conclusion about our intervention when we have major concerns about testing effects without trying to determine the extent of those effects.
Solomon four group designs are less common in social work research, primarily because of the logistics and resource needs involved. Nonetheless, this is an important experimental design to consider when we want to address major concerns about testing effects.
- True experimental design is best suited for explanatory research questions.
- True experiments require random assignment of participants to control and experimental groups.
- Pretest/post-test research design involves two points of measurement—one pre-intervention and one post-intervention.
- Post-test only research design involves only one point of measurement—post-intervention. It is a useful design to minimize the effect of testing effects on our results.
- Solomon four group research design involves both of the above types of designs, using 2 pairs of control and experimental groups. One group receives both a pretest and a post-test, while the other receives only a post-test. This can help uncover the influence of testing effects.
- Think about a true experiment you might conduct for your research project. Which design would be best for your research, and why?
- What challenges or limitations might make it unrealistic (or at least very complicated!) for you to carry your true experimental design in the real-world as a student researcher?
- What hypothesis(es) would you test using this true experiment?
13.4 Quasi-experimental designs
- Describe a quasi-experimental design in social work research
- Understand the different types of quasi-experimental designs
- Determine what kinds of research questions quasi-experimental designs are suited for
- Discuss advantages and disadvantages of quasi-experimental designs
Quasi-experimental designs are a lot more common in social work research than true experimental designs. Although quasi-experiments don’t do as good a job of giving us robust proof of causality , they still allow us to establish time order , which is a key element of causality. The prefix quasi means “resembling,” so quasi-experimental research is research that resembles experimental research, but is not true experimental research. Nonetheless, given proper research design, quasi-experiments can still provide extremely rigorous and useful results.
There are a few key differences between true experimental and quasi-experimental research. The primary difference between quasi-experimental research and true experimental research is that quasi-experimental research does not involve random assignment to control and experimental groups. Instead, we talk about comparison groups in quasi-experimental research instead. As a result, these types of experiments don’t control the effect of extraneous variables as well as a true experiment.
Quasi-experiments are most likely to be conducted in field settings in which random assignment is difficult or impossible. They are often conducted to evaluate the effectiveness of a treatment—perhaps a type of psychotherapy or an educational intervention. We’re able to eliminate some threats to internal validity, but we can’t do this as effectively as we can with a true experiment. Realistically, our CBT-social anxiety project is likely to be a quasi experiment, based on the resources and participant pool we’re likely to have available.
It’s important to note that not all quasi-experimental designs have a comparison group. There are many different kinds of quasi-experiments, but we will discuss the three main types below: nonequivalent comparison group designs, time series designs, and ex post facto comparison group designs.
Nonequivalent comparison group design
You will notice that this type of design looks extremely similar to the pretest/post-test design that we discussed in section 13.3. But instead of random assignment to control and experimental groups, researchers use other methods to construct their comparison and experimental groups. A diagram of this design will also look very similar to pretest/post-test design, but you’ll notice we’ve removed the “R” from our groups, since they are not randomly assigned (Figure 13.6).
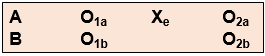
Researchers using this design select a comparison group that’s as close as possible based on relevant factors to their experimental group. Engel and Schutt (2017) [2] identify two different selection methods:
- Individual matching : Researchers take the time to match individual cases in the experimental group to similar cases in the comparison group. It can be difficult, however, to match participants on all the variables you want to control for.
- Aggregate matching : Instead of trying to match individual participants to each other, researchers try to match the population profile of the comparison and experimental groups. For example, researchers would try to match the groups on average age, gender balance, or median income. This is a less resource-intensive matching method, but researchers have to ensure that participants aren’t choosing which group (comparison or experimental) they are a part of.
As we’ve already talked about, this kind of design provides weaker evidence that the intervention itself leads to a change in outcome. Nonetheless, we are still able to establish time order using this method, and can thereby show an association between the intervention and the outcome. Like true experimental designs, this type of quasi-experimental design is useful for explanatory research questions.
What might this look like in a practice setting? Let’s say you’re working at an agency that provides CBT and other types of interventions, and you have identified a group of clients who are seeking help for social anxiety, as in our earlier example. Once you’ve obtained consent from your clients, you can create a comparison group using one of the matching methods we just discussed. If the group is small, you might match using individual matching, but if it’s larger, you’ll probably sort people by demographics to try to get similar population profiles. (You can do aggregate matching more easily when your agency has some kind of electronic records or database, but it’s still possible to do manually.)
Time series design
Another type of quasi-experimental design is a time series design. Unlike other types of experimental design, time series designs do not have a comparison group. A time series is a set of measurements taken at intervals over a period of time (Figure 13.7). Proper time series design should include at least three pre- and post-intervention measurement points. While there are a few types of time series designs, we’re going to focus on the most common: interrupted time series design.

But why use this method? Here’s an example. Let’s think about elementary student behavior throughout the school year. As anyone with children or who is a teacher knows, kids get very excited and animated around holidays, days off, or even just on a Friday afternoon. This fact might mean that around those times of year, there are more reports of disruptive behavior in classrooms. What if we took our one and only measurement in mid-December? It’s possible we’d see a higher-than-average rate of disruptive behavior reports, which could bias our results if our next measurement is around a time of year students are in a different, less excitable frame of mind. When we take multiple measurements throughout the first half of the school year, we can establish a more accurate baseline for the rate of these reports by looking at the trend over time.
We may want to test the effect of extended recess times in elementary school on reports of disruptive behavior in classrooms. When students come back after the winter break, the school extends recess by 10 minutes each day (the intervention), and the researchers start tracking the monthly reports of disruptive behavior again. These reports could be subject to the same fluctuations as the pre-intervention reports, and so we once again take multiple measurements over time to try to control for those fluctuations.
This method improves the extent to which we can establish causality because we are accounting for a major extraneous variable in the equation—the passage of time. On its own, it does not allow us to account for other extraneous variables, but it does establish time order and association between the intervention and the trend in reports of disruptive behavior. Finding a stable condition before the treatment that changes after the treatment is evidence for causality between treatment and outcome.
Ex post facto comparison group design
Ex post facto (Latin for “after the fact”) designs are extremely similar to nonequivalent comparison group designs. There are still comparison and experimental groups, pretest and post-test measurements, and an intervention. But in ex post facto designs, participants are assigned to the comparison and experimental groups once the intervention has already happened. This type of design often occurs when interventions are already up and running at an agency and the agency wants to assess effectiveness based on people who have already completed treatment.
In most clinical agency environments, social workers conduct both initial and exit assessments, so there are usually some kind of pretest and post-test measures available. We also typically collect demographic information about our clients, which could allow us to try to use some kind of matching to construct comparison and experimental groups.
In terms of internal validity and establishing causality, ex post facto designs are a bit of a mixed bag. The ability to establish causality depends partially on the ability to construct comparison and experimental groups that are demographically similar so we can control for these extraneous variables .
Quasi-experimental designs are common in social work intervention research because, when designed correctly, they balance the intense resource needs of true experiments with the realities of research in practice. They still offer researchers tools to gather robust evidence about whether interventions are having positive effects for clients.
- Quasi-experimental designs are similar to true experiments, but do not require random assignment to experimental and control groups.
- In quasi-experimental projects, the group not receiving the treatment is called the comparison group, not the control group.
- Nonequivalent comparison group design is nearly identical to pretest/post-test experimental design, but participants are not randomly assigned to the experimental and control groups. As a result, this design provides slightly less robust evidence for causality.
- Nonequivalent groups can be constructed by individual matching or aggregate matching .
- Time series design does not have a control or experimental group, and instead compares the condition of participants before and after the intervention by measuring relevant factors at multiple points in time. This allows researchers to mitigate the error introduced by the passage of time.
- Ex post facto comparison group designs are also similar to true experiments, but experimental and comparison groups are constructed after the intervention is over. This makes it more difficult to control for the effect of extraneous variables, but still provides useful evidence for causality because it maintains the time order[ /pb_glossary] of the experiment.
- Think back to the experiment you considered for your research project in Section 13.3. Now that you know more about quasi-experimental designs, do you still think it's a true experiment? Why or why not?
- What should you consider when deciding whether an experimental or quasi-experimental design would be more feasible or fit your research question better?
13.5 Non-experimental designs
Learners will be able to...
- Describe non-experimental designs in social work research
- Discuss how non-experimental research differs from true and quasi-experimental research
- Demonstrate an understanding the different types of non-experimental designs
- Determine what kinds of research questions non-experimental designs are suited for
- Discuss advantages and disadvantages of non-experimental designs
The previous sections have laid out the basics of some rigorous approaches to establish that an intervention is responsible for changes we observe in research participants. This type of evidence is extremely important to build an evidence base for social work interventions, but it's not the only type of evidence to consider. We will discuss qualitative methods, which provide us with rich, contextual information, in Part 4 of this text. The designs we'll talk about in this section are sometimes used in [pb_glossary id="851"] qualitative research, but in keeping with our discussion of experimental design so far, we're going to stay in the quantitative research realm for now. Non-experimental is also often a stepping stone for more rigorous experimental design in the future, as it can help test the feasibility of your research.
In general, non-experimental designs do not strongly support causality and don't address threats to internal validity. However, that's not really what they're intended for. Non-experimental designs are useful for a few different types of research, including explanatory questions in program evaluation. Certain types of non-experimental design are also helpful for researchers when they are trying to develop a new assessment or scale. Other times, researchers or agency staff did not get a chance to gather any assessment information before an intervention began, so a pretest/post-test design is not possible.
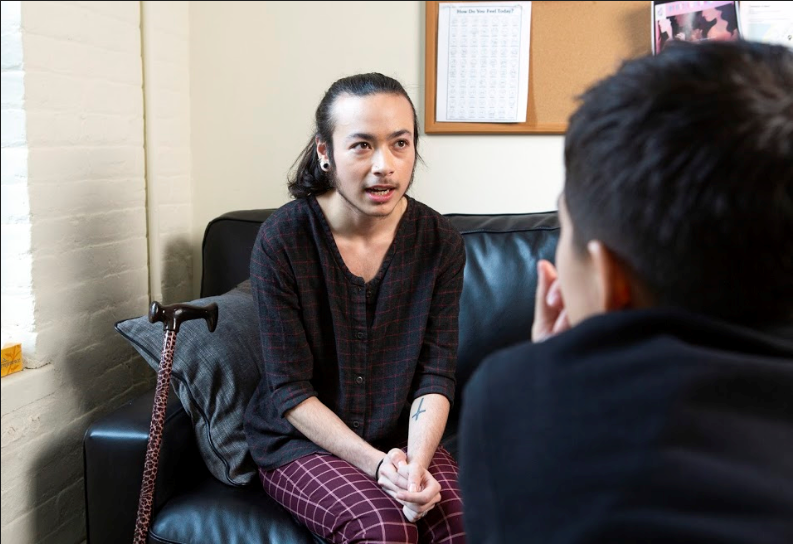
A significant benefit of these types of designs is that they're pretty easy to execute in a practice or agency setting. They don't require a comparison or control group, and as Engel and Schutt (2017) [3] point out, they "flow from a typical practice model of assessment, intervention, and evaluating the impact of the intervention" (p. 177). Thus, these designs are fairly intuitive for social workers, even when they aren't expert researchers. Below, we will go into some detail about the different types of non-experimental design.
One group pretest/post-test design
Also known as a before-after one-group design, this type of research design does not have a comparison group and everyone who participates in the research receives the intervention (Figure 13.8). This is a common type of design in program evaluation in the practice world. Controlling for extraneous variables is difficult or impossible in this design, but given that it is still possible to establish some measure of time order, it does provide weak support for causality.

Imagine, for example, a researcher who is interested in the effectiveness of an anti-drug education program on elementary school students’ attitudes toward illegal drugs. The researcher could assess students' attitudes about illegal drugs (O 1 ), implement the anti-drug program (X), and then immediately after the program ends, the researcher could once again measure students’ attitudes toward illegal drugs (O 2 ). You can see how this would be relatively simple to do in practice, and have probably been involved in this type of research design yourself, even if informally. But hopefully, you can also see that this design would not provide us with much evidence for causality because we have no way of controlling for the effect of extraneous variables. A lot of things could have affected any change in students' attitudes—maybe girls already had different attitudes about illegal drugs than children of other genders, and when we look at the class's results as a whole, we couldn't account for that influence using this design.
All of that doesn't mean these results aren't useful, however. If we find that children's attitudes didn't change at all after the drug education program, then we need to think seriously about how to make it more effective or whether we should be using it at all. (This immediate, practical application of our results highlights a key difference between program evaluation and research, which we will discuss in Chapter 23 .)
After-only design
As the name suggests, this type of non-experimental design involves measurement only after an intervention. There is no comparison or control group, and everyone receives the intervention. I have seen this design repeatedly in my time as a program evaluation consultant for nonprofit organizations, because often these organizations realize too late that they would like to or need to have some sort of measure of what effect their programs are having.
Because there is no pretest and no comparison group, this design is not useful for supporting causality since we can't establish the time order and we can't control for extraneous variables. However, that doesn't mean it's not useful at all! Sometimes, agencies need to gather information about how their programs are functioning. A classic example of this design is satisfaction surveys—realistically, these can only be administered after a program or intervention. Questions regarding satisfaction, ease of use or engagement, or other questions that don't involve comparisons are best suited for this type of design.
Static-group design
A final type of non-experimental research is the static-group design. In this type of research, there are both comparison and experimental groups, which are not randomly assigned. There is no pretest, only a post-test, and the comparison group has to be constructed by the researcher. Sometimes, researchers will use matching techniques to construct the groups, but often, the groups are constructed by convenience of who is being served at the agency.
Non-experimental research designs are easy to execute in practice, but we must be cautious about drawing causal conclusions from the results. A positive result may still suggest that we should continue using a particular intervention (and no result or a negative result should make us reconsider whether we should use that intervention at all). You have likely seen non-experimental research in your daily life or at your agency, and knowing the basics of how to structure such a project will help you ensure you are providing clients with the best care possible.
- Non-experimental designs are useful for describing phenomena, but cannot demonstrate causality.
- After-only designs are often used in agency and practice settings because practitioners are often not able to set up pre-test/post-test designs.
- Non-experimental designs are useful for explanatory questions in program evaluation and are helpful for researchers when they are trying to develop a new assessment or scale.
- Non-experimental designs are well-suited to qualitative methods.
- If you were to use a non-experimental design for your research project, which would you choose? Why?
- Have you conducted non-experimental research in your practice or professional life? Which type of non-experimental design was it?
13.6 Critical, ethical, and cultural considerations
- Describe critiques of experimental design
- Identify ethical issues in the design and execution of experiments
- Identify cultural considerations in experimental design
As I said at the outset, experiments, and especially true experiments, have long been seen as the gold standard to gather scientific evidence. When it comes to research in the biomedical field and other physical sciences, true experiments are subject to far less nuance than experiments in the social world. This doesn't mean they are easier—just subject to different forces. However, as a society, we have placed the most value on quantitative evidence obtained through empirical observation and especially experimentation.
Major critiques of experimental designs tend to focus on true experiments, especially randomized controlled trials (RCTs), but many of these critiques can be applied to quasi-experimental designs, too. Some researchers, even in the biomedical sciences, question the view that RCTs are inherently superior to other types of quantitative research designs. RCTs are far less flexible and have much more stringent requirements than other types of research. One seemingly small issue, like incorrect information about a research participant, can derail an entire RCT. RCTs also cost a great deal of money to implement and don't reflect “real world” conditions. The cost of true experimental research or RCTs also means that some communities are unlikely to ever have access to these research methods. It is then easy for people to dismiss their research findings because their methods are seen as "not rigorous."
Obviously, controlling outside influences is important for researchers to draw strong conclusions, but what if those outside influences are actually important for how an intervention works? Are we missing really important information by focusing solely on control in our research? Is a treatment going to work the same for white women as it does for indigenous women? With the myriad effects of our societal structures, you should be very careful ever assuming this will be the case. This doesn't mean that cultural differences will negate the effect of an intervention; instead, it means that you should remember to practice cultural humility implementing all interventions, even when we "know" they work.
How we build evidence through experimental research reveals a lot about our values and biases, and historically, much experimental research has been conducted on white people, and especially white men. [4] This makes sense when we consider the extent to which the sciences and academia have historically been dominated by white patriarchy. This is especially important for marginalized groups that have long been ignored in research literature, meaning they have also been ignored in the development of interventions and treatments that are accepted as "effective." There are examples of marginalized groups being experimented on without their consent, like the Tuskegee Experiment or Nazi experiments on Jewish people during World War II. We cannot ignore the collective consciousness situations like this can create about experimental research for marginalized groups.
None of this is to say that experimental research is inherently bad or that you shouldn't use it. Quite the opposite—use it when you can, because there are a lot of benefits, as we learned throughout this chapter. As a social work researcher, you are uniquely positioned to conduct experimental research while applying social work values and ethics to the process and be a leader for others to conduct research in the same framework. It can conflict with our professional ethics, especially respect for persons and beneficence, if we do not engage in experimental research with our eyes wide open. We also have the benefit of a great deal of practice knowledge that researchers in other fields have not had the opportunity to get. As with all your research, always be sure you are fully exploring the limitations of the research.
- While true experimental research gathers strong evidence, it can also be inflexible, expensive, and overly simplistic in terms of important social forces that affect the resources.
- Marginalized communities' past experiences with experimental research can affect how they respond to research participation.
- Social work researchers should use both their values and ethics, and their practice experiences, to inform research and push other researchers to do the same.
- Think back to the true experiment you sketched out in the exercises for Section 13.3. Are there cultural or historical considerations you hadn't thought of with your participant group? What are they? Does this change the type of experiment you would want to do?
- How can you as a social work researcher encourage researchers in other fields to consider social work ethics and values in their experimental research?
- Engel, R. & Schutt, R. (2016). The practice of research in social work. Thousand Oaks, CA: SAGE Publications, Inc. ↵
- Sullivan, G. M. (2011). Getting off the “gold standard”: Randomized controlled trials and education research. Journal of Graduate Medical Education , 3 (3), 285-289. ↵
an operation or procedure carried out under controlled conditions in order to discover an unknown effect or law, to test or establish a hypothesis, or to illustrate a known law.
explains why particular phenomena work in the way that they do; answers “why” questions
variables and characteristics that have an effect on your outcome, but aren't the primary variable whose influence you're interested in testing.
the group of participants in our study who do not receive the intervention we are researching in experiments with random assignment
in experimental design, the group of participants in our study who do receive the intervention we are researching
the group of participants in our study who do not receive the intervention we are researching in experiments without random assignment
using a random process to decide which participants are tested in which conditions
The ability to apply research findings beyond the study sample to some broader population,
Ability to say that one variable "causes" something to happen to another variable. Very important to assess when thinking about studies that examine causation such as experimental or quasi-experimental designs.
the idea that one event, behavior, or belief will result in the occurrence of another, subsequent event, behavior, or belief
An experimental design in which one or more independent variables are manipulated by the researcher (as treatments), subjects are randomly assigned to different treatment levels (random assignment), and the results of the treatments on outcomes (dependent variables) are observed
a type of experimental design in which participants are randomly assigned to control and experimental groups, one group receives an intervention, and both groups receive pre- and post-test assessments
A measure of a participant's condition before they receive an intervention or treatment.
A measure of a participant's condition after an intervention or, if they are part of the control/comparison group, at the end of an experiment.
A demonstration that a change occurred after an intervention. An important criterion for establishing causality.
an experimental design in which participants are randomly assigned to control and treatment groups, one group receives an intervention, and both groups receive only a post-test assessment
The measurement error related to how a test is given; the conditions of the testing, including environmental conditions; and acclimation to the test itself
a subtype of experimental design that is similar to a true experiment, but does not have randomly assigned control and treatment groups
In nonequivalent comparison group designs, the process by which researchers match individual cases in the experimental group to similar cases in the comparison group.
In nonequivalent comparison group designs, the process in which researchers match the population profile of the comparison and experimental groups.
a set of measurements taken at intervals over a period of time
Graduate research methods in social work Copyright © 2021 by Matthew DeCarlo, Cory Cummings, Kate Agnelli is licensed under a Creative Commons Attribution-NonCommercial-ShareAlike 4.0 International License , except where otherwise noted.
Share This Book
- Skip to secondary menu
- Skip to main content
- Skip to primary sidebar
Statistics By Jim
Making statistics intuitive
Experimental Design: Definition and Types
By Jim Frost 3 Comments
What is Experimental Design?
An experimental design is a detailed plan for collecting and using data to identify causal relationships. Through careful planning, the design of experiments allows your data collection efforts to have a reasonable chance of detecting effects and testing hypotheses that answer your research questions.
An experiment is a data collection procedure that occurs in controlled conditions to identify and understand causal relationships between variables. Researchers can use many potential designs. The ultimate choice depends on their research question, resources, goals, and constraints. In some fields of study, researchers refer to experimental design as the design of experiments (DOE). Both terms are synonymous.

Ultimately, the design of experiments helps ensure that your procedures and data will evaluate your research question effectively. Without an experimental design, you might waste your efforts in a process that, for many potential reasons, can’t answer your research question. In short, it helps you trust your results.
Learn more about Independent and Dependent Variables .
Design of Experiments: Goals & Settings
Experiments occur in many settings, ranging from psychology, social sciences, medicine, physics, engineering, and industrial and service sectors. Typically, experimental goals are to discover a previously unknown effect , confirm a known effect, or test a hypothesis.
Effects represent causal relationships between variables. For example, in a medical experiment, does the new medicine cause an improvement in health outcomes? If so, the medicine has a causal effect on the outcome.
An experimental design’s focus depends on the subject area and can include the following goals:
- Understanding the relationships between variables.
- Identifying the variables that have the largest impact on the outcomes.
- Finding the input variable settings that produce an optimal result.
For example, psychologists have conducted experiments to understand how conformity affects decision-making. Sociologists have performed experiments to determine whether ethnicity affects the public reaction to staged bike thefts. These experiments map out the causal relationships between variables, and their primary goal is to understand the role of various factors.
Conversely, in a manufacturing environment, the researchers might use an experimental design to find the factors that most effectively improve their product’s strength, identify the optimal manufacturing settings, and do all that while accounting for various constraints. In short, a manufacturer’s goal is often to use experiments to improve their products cost-effectively.
In a medical experiment, the goal might be to quantify the medicine’s effect and find the optimum dosage.
Developing an Experimental Design
Developing an experimental design involves planning that maximizes the potential to collect data that is both trustworthy and able to detect causal relationships. Specifically, these studies aim to see effects when they exist in the population the researchers are studying, preferentially favor causal effects, isolate each factor’s true effect from potential confounders, and produce conclusions that you can generalize to the real world.
To accomplish these goals, experimental designs carefully manage data validity and reliability , and internal and external experimental validity. When your experiment is valid and reliable, you can expect your procedures and data to produce trustworthy results.
An excellent experimental design involves the following:
- Lots of preplanning.
- Developing experimental treatments.
- Determining how to assign subjects to treatment groups.
The remainder of this article focuses on how experimental designs incorporate these essential items to accomplish their research goals.
Learn more about Data Reliability vs. Validity and Internal and External Experimental Validity .
Preplanning, Defining, and Operationalizing for Design of Experiments

This phase of the design of experiments helps you identify critical variables, know how to measure them while ensuring reliability and validity, and understand the relationships between them. The review can also help you find ways to reduce sources of variability, which increases your ability to detect treatment effects. Notably, the literature review allows you to learn how similar studies designed their experiments and the challenges they faced.
Operationalizing a study involves taking your research question, using the background information you gathered, and formulating an actionable plan.
This process should produce a specific and testable hypothesis using data that you can reasonably collect given the resources available to the experiment.
- Null hypothesis : The jumping exercise intervention does not affect bone density.
- Alternative hypothesis : The jumping exercise intervention affects bone density.
To learn more about this early phase, read Five Steps for Conducting Scientific Studies with Statistical Analyses .
Formulating Treatments in Experimental Designs
In an experimental design, treatments are variables that the researchers control. They are the primary independent variables of interest. Researchers administer the treatment to the subjects or items in the experiment and want to know whether it causes changes in the outcome.
As the name implies, a treatment can be medical in nature, such as a new medicine or vaccine. But it’s a general term that applies to other things such as training programs, manufacturing settings, teaching methods, and types of fertilizers. I helped run an experiment where the treatment was a jumping exercise intervention that we hoped would increase bone density. All these treatment examples are things that potentially influence a measurable outcome.
Even when you know your treatment generally, you must carefully consider the amount. How large of a dose? If you’re comparing three different temperatures in a manufacturing process, how far apart are they? For my bone mineral density study, we had to determine how frequently the exercise sessions would occur and how long each lasted.
How you define the treatments in the design of experiments can affect your findings and the generalizability of your results.
Assigning Subjects to Experimental Groups
A crucial decision for all experimental designs is determining how researchers assign subjects to the experimental conditions—the treatment and control groups. The control group is often, but not always, the lack of a treatment. It serves as a basis for comparison by showing outcomes for subjects who don’t receive a treatment. Learn more about Control Groups .
How your experimental design assigns subjects to the groups affects how confident you can be that the findings represent true causal effects rather than mere correlation caused by confounders. Indeed, the assignment method influences how you control for confounding variables. This is the difference between correlation and causation .
Imagine a study finds that vitamin consumption correlates with better health outcomes. As a researcher, you want to be able to say that vitamin consumption causes the improvements. However, with the wrong experimental design, you might only be able to say there is an association. A confounder, and not the vitamins, might actually cause the health benefits.
Let’s explore some of the ways to assign subjects in design of experiments.
Completely Randomized Designs
A completely randomized experimental design randomly assigns all subjects to the treatment and control groups. You simply take each participant and use a random process to determine their group assignment. You can flip coins, roll a die, or use a computer. Randomized experiments must be prospective studies because they need to be able to control group assignment.
Random assignment in the design of experiments helps ensure that the groups are roughly equivalent at the beginning of the study. This equivalence at the start increases your confidence that any differences you see at the end were caused by the treatments. The randomization tends to equalize confounders between the experimental groups and, thereby, cancels out their effects, leaving only the treatment effects.
For example, in a vitamin study, the researchers can randomly assign participants to either the control or vitamin group. Because the groups are approximately equal when the experiment starts, if the health outcomes are different at the end of the study, the researchers can be confident that the vitamins caused those improvements.
Statisticians consider randomized experimental designs to be the best for identifying causal relationships.
If you can’t randomly assign subjects but want to draw causal conclusions about an intervention, consider using a quasi-experimental design .
Learn more about Randomized Controlled Trials and Random Assignment in Experiments .
Randomized Block Designs
Nuisance factors are variables that can affect the outcome, but they are not the researcher’s primary interest. Unfortunately, they can hide or distort the treatment results. When experimenters know about specific nuisance factors, they can use a randomized block design to minimize their impact.
This experimental design takes subjects with a shared “nuisance” characteristic and groups them into blocks. The participants in each block are then randomly assigned to the experimental groups. This process allows the experiment to control for known nuisance factors.
Blocking in the design of experiments reduces the impact of nuisance factors on experimental error. The analysis assesses the effects of the treatment within each block, which removes the variability between blocks. The result is that blocked experimental designs can reduce the impact of nuisance variables, increasing the ability to detect treatment effects accurately.
Suppose you’re testing various teaching methods. Because grade level likely affects educational outcomes, you might use grade level as a blocking factor. To use a randomized block design for this scenario, divide the participants by grade level and then randomly assign the members of each grade level to the experimental groups.
A standard guideline for an experimental design is to “Block what you can, randomize what you cannot.” Use blocking for a few primary nuisance factors. Then use random assignment to distribute the unblocked nuisance factors equally between the experimental conditions.
You can also use covariates to control nuisance factors. Learn about Covariates: Definition and Uses .
Observational Studies
In some experimental designs, randomly assigning subjects to the experimental conditions is impossible or unethical. The researchers simply can’t assign participants to the experimental groups. However, they can observe them in their natural groupings, measure the essential variables, and look for correlations. These observational studies are also known as quasi-experimental designs. Retrospective studies must be observational in nature because they look back at past events.
Imagine you’re studying the effects of depression on an activity. Clearly, you can’t randomly assign participants to the depression and control groups. But you can observe participants with and without depression and see how their task performance differs.
Observational studies let you perform research when you can’t control the treatment. However, quasi-experimental designs increase the problem of confounding variables. For this design of experiments, correlation does not necessarily imply causation. While special procedures can help control confounders in an observational study, you’re ultimately less confident that the results represent causal findings.
Learn more about Observational Studies .
For a good comparison, learn about the differences and tradeoffs between Observational Studies and Randomized Experiments .
Between-Subjects vs. Within-Subjects Experimental Designs
When you think of the design of experiments, you probably picture a treatment and control group. Researchers assign participants to only one of these groups, so each group contains entirely different subjects than the other groups. Analysts compare the groups at the end of the experiment. Statisticians refer to this method as a between-subjects, or independent measures, experimental design.
In a between-subjects design , you can have more than one treatment group, but each subject is exposed to only one condition, the control group or one of the treatment groups.
A potential downside to this approach is that differences between groups at the beginning can affect the results at the end. As you’ve read earlier, random assignment can reduce those differences, but it is imperfect. There will always be some variability between the groups.
In a within-subjects experimental design , also known as repeated measures, subjects experience all treatment conditions and are measured for each. Each subject acts as their own control, which reduces variability and increases the statistical power to detect effects.
In this experimental design, you minimize pre-existing differences between the experimental conditions because they all contain the same subjects. However, the order of treatments can affect the results. Beware of practice and fatigue effects. Learn more about Repeated Measures Designs .
Assigned to one experimental condition | Participates in all experimental conditions |
Requires more subjects | Fewer subjects |
Differences between subjects in the groups can affect the results | Uses same subjects in all conditions. |
No order of treatment effects. | Order of treatments can affect results. |
Design of Experiments Examples
For example, a bone density study has three experimental groups—a control group, a stretching exercise group, and a jumping exercise group.
In a between-subjects experimental design, scientists randomly assign each participant to one of the three groups.
In a within-subjects design, all subjects experience the three conditions sequentially while the researchers measure bone density repeatedly. The procedure can switch the order of treatments for the participants to help reduce order effects.
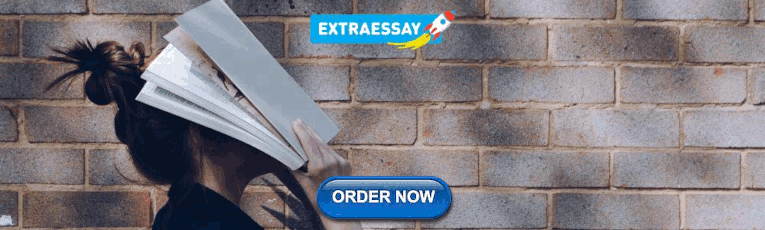
Matched Pairs Experimental Design
A matched pairs experimental design is a between-subjects study that uses pairs of similar subjects. Researchers use this approach to reduce pre-existing differences between experimental groups. It’s yet another design of experiments method for reducing sources of variability.
Researchers identify variables likely to affect the outcome, such as demographics. When they pick a subject with a set of characteristics, they try to locate another participant with similar attributes to create a matched pair. Scientists randomly assign one member of a pair to the treatment group and the other to the control group.
On the plus side, this process creates two similar groups, and it doesn’t create treatment order effects. While matched pairs do not produce the perfectly matched groups of a within-subjects design (which uses the same subjects in all conditions), it aims to reduce variability between groups relative to a between-subjects study.
On the downside, finding matched pairs is very time-consuming. Additionally, if one member of a matched pair drops out, the other subject must leave the study too.
Learn more about Matched Pairs Design: Uses & Examples .
Another consideration is whether you’ll use a cross-sectional design (one point in time) or use a longitudinal study to track changes over time .
A case study is a research method that often serves as a precursor to a more rigorous experimental design by identifying research questions, variables, and hypotheses to test. Learn more about What is a Case Study? Definition & Examples .
In conclusion, the design of experiments is extremely sensitive to subject area concerns and the time and resources available to the researchers. Developing a suitable experimental design requires balancing a multitude of considerations. A successful design is necessary to obtain trustworthy answers to your research question and to have a reasonable chance of detecting treatment effects when they exist.
Share this:

Reader Interactions
March 23, 2024 at 2:35 pm
Dear Jim You wrote a superb document, I will use it in my Buistatistics course, along with your three books. Thank you very much! Miguel
March 23, 2024 at 5:43 pm
Thanks so much, Miguel! Glad this post was helpful and I trust the books will be as well.
April 10, 2023 at 4:36 am
What are the purpose and uses of experimental research design?
Comments and Questions Cancel reply
- A-Z Publications
Annual Review of Sociology
Volume 43, 2017, review article, field experiments across the social sciences.
- Delia Baldassarri 1 , and Maria Abascal 2
- View Affiliations Hide Affiliations Affiliations: 1 Department of Sociology, New York University, New York, New York 10012; email: [email protected] 2 Department of Sociology, Columbia University, New York, New York 10027; email: [email protected]
- Vol. 43:41-73 (Volume publication date July 2017) https://doi.org/10.1146/annurev-soc-073014-112445
- First published as a Review in Advance on May 22, 2017
- © Annual Reviews
Using field experiments, scholars can identify causal effects via randomization while studying people and groups in their naturally occurring contexts. In light of renewed interest in field experimental methods, this review covers a wide range of field experiments from across the social sciences, with an eye to those that adopt virtuous practices, including unobtrusive measurement, naturalistic interventions, attention to realistic outcomes and consequential behaviors, and application to diverse samples and settings. The review covers four broad research areas of substantive and policy interest: first, randomized controlled trials, with a focus on policy interventions in economic development, poverty reduction, and education; second, experiments on the role that norms, motivations, and incentives play in shaping behavior; third, experiments on political mobilization, social influence, and institutional effects; and fourth, experiments on prejudice and discrimination. We discuss methodological issues concerning generalizability and scalability as well as ethical issues related to field experimental methods. We conclude by arguing that field experiments are well equipped to advance the kind of middle-range theorizing that sociologists value.
Article metrics loading...
Full text loading...
Literature Cited
- Abascal M . 2015 . Us and them: black–white relations in the wake of Hispanic population growth. Am. Sociol. Rev. 80 : 789– 813 [Google Scholar]
- Adida CL , Laitin DD , Valfort MA . 2016 . Why Muslim Integration Fails in Christian-Heritage Societies Cambridge, MA: Harvard Univ. Press [Google Scholar]
- Ahmed AM , Hammarstedt M . 2008 . Discrimination in the rental housing market: a field experiment on the Internet. J. Urban Econ. 64 : 362– 72 [Google Scholar]
- Ahmed AM , Hammarstedt M . 2009 . Detecting discrimination against homosexuals: evidence from a field experiment on the Internet. Economica 76 : 599– 97 [Google Scholar]
- Arceneaux K , Nickerson DW . 2009 . Who is mobilized to vote? A re-analysis of 11 field experiments. Am. J. Political Sci. 53 : 1– 16 [Google Scholar]
- Attanasio O , Augsburg B , De Haas R , Fitzsimons E , Harmgart H . 2012 . Group lending or individual lending? Evidence from a randomised field experiment in Mongolia. Work. Pap. No. 136, Eur. Bank Reconstr. Dev. [Google Scholar]
- Attanasio O , Pellerano L , Reyes SP . 2009 . Building trust? Conditional cash transfer programmes and social capital. Fiscal Stud. 30 : 139– 77 [Google Scholar]
- Avdeenko A , Gilligan MG . 2015 . International interventions to build social capital: evidence from a field experiment in Sudan. Am. Political Sci. Rev. 109 : 427– 49 [Google Scholar]
- Ayres I , Siegelman P . 1995 . Race and gender discrimination in bargaining for a new car. Am. Econ. Rev. 85 : 304– 21 [Google Scholar]
- Baldassarri D . 2015 . Cooperative networks: altruism, group solidarity, and reciprocity in Ugandan farmer organizations. Am. J. Sociol. 121 : 355– 95 [Google Scholar]
- Baldassarri D . 2016 . Prosocial behavior across communities: evidence from a nationwide lost-letter experiment Presented at Advances with Field Experiments Conf., Sept. 16, Univ Chicago: [Google Scholar]
- Banerjee A , Bertrand M , Datta S , Mullainathan S . 2009 . Labor market discrimination in Delhi: evidence from a field experiment. J. Comp. Econ. 37 : 14– 27 [Google Scholar]
- Banerjee A , Duflo E . 2009 . The experimental approach to development economics. Annu. Rev. Econ. 1 : 151– 78 [Google Scholar]
- Banerjee A , Duflo E . 2011 . Poor Economics: A Radical Rethinking of the Way to Fight Global Poverty. New York: Public Affairs [Google Scholar]
- Banerjee A , Duflo E , Glennerster R , Kothari D . 2010a . Improving immunization coverage in rural India: Clustered randomized controlled immunisation campaigns with and without incentives. Br. Med. J. 340:c2220 [Google Scholar]
- Banerjee A , Duflo E , Glennerster R , Kinnan C . 2010b . The miracle of microfinance? Evidence from a randomized evaluation. Work. Pap. No. 13-09, Dep. Econ., MIT [Google Scholar]
- Barr A . 2003 . Trust and expected trustworthiness: experimental evidence from Zimbabwean villages. Econ. J. 113 : 614– 30 [Google Scholar]
- Bauchet J , Marshall C , Starita L , Thomas J , Yalouris A . 2011 . Latest findings from randomized evaluations of microfinance. Access Finance Forum Rep. 2 : 1– 27 [Google Scholar]
- Beath A , Christia F , Enikolopov R . 2013 . Empowering women: evidence from a field experiment in Afghanistan. Am. Political Sci. Rev. 107 : 540– 57 [Google Scholar]
- Benson PL , Karabenick SA , Lerner RM . 1976 . Pretty pleases: the effects of physical attractiveness, race, and sex on receiving help. J. Exp. Soc. Psychol. 12 : 409– 15 [Google Scholar]
- Benz M , Meier S . 2008 . Do people behave in experiments as in the field? Evidence from donations. Exp. Econ. 11 : 278– 81 [Google Scholar]
- Bertrand M , Karlan D , Mullainathan S , Shafir E , Zinman J . 2010 . What's advertising content worth? Evidence from a consumer credit marketing field experiment. Q. J. Econ. 125 : 263– 306 [Google Scholar]
- Bertrand M , Mullainathan S . 2004 . Are Emily and Greg more employable than Lakisha and Jamal? A field experiment on labor market discrimination. Am. Econ. Rev. 94 : 991– 1013 [Google Scholar]
- Besbris M , Faber JW , Rich P , Sharkey P . 2015 . Effect of neighborhood stigma on economic transitions. PNAS 112 : 4994– 98 [Google Scholar]
- Bettinger EP . 2012 . Paying to learn: the effect of financial incentives on elementary school test scores. Rev. Econ. Stat. 94 : 686– 98 [Google Scholar]
- Bigoni M , Bortolotti S , Casari M , Gambetta D , Pancotto F . 2016 . Amoral familism, social capital, or trust? The behavioural foundations of the Italian north–south divide. Econ. J. 126 : 1318– 41 [Google Scholar]
- Blommaert L , Coenders M , van Tubergen F . 2014 . Discrimination of Arabic-named applicants in the Netherlands: an Internet-based field experiment examining different phases in online recruitment procedures. Soc. Forces 92 : 957– 82 [Google Scholar]
- Bond RM , Fariss CJ , Jones JJ , Kramer AD , Marlow C . et al. 2012 . A 61-million-person experiment in social influence and political mobilization. Nature 489 : 295– 98 [Google Scholar]
- Bosch M , Carnero MA , Farré L . 2010 . Information and discrimination in the rental housing market: evidence from a field experiment. Reg. Sci. Urban Econ. 40 : 11– 19 [Google Scholar]
- Brearley HC . 1931 . Experimental sociology in the United States. Soc. Forces 10 : 196– 99 [Google Scholar]
- Butler DM , Broockman DE . 2011 . Do politicians racially discriminate against constituents? A field experiment on state legislators. Am. J. Political Sci. 55 : 463– 77 [Google Scholar]
- Butler DM , Nickerson DW . 2011 . Can learning constituency opinion affect how legislators vote? Results from a field experiment. Q. J. Political Sci. 6 : 55– 83 [Google Scholar]
- Camerer C . 2003 . Behavioral Game Theory: Experiments in Strategic Interaction New York, NY: Russell Sage Found. [Google Scholar]
- Cardenas J , Carpenter J . 2008 . Behavioural development economics: lessons from field labs in the developing world. J. Dev. Stud. 44 : 337– 64 [Google Scholar]
- Casey K , Glennerster R , Miguel E . 2012 . Reshaping institutions: evidence on external aid and local collective action. Q. J. Econ. 127 : 1755– 812 [Google Scholar]
- Castilla EJ , Benard S . 2010 . The paradox of meritocracy in organizations. Adm. Sci. Q. 55 : 543– 676 [Google Scholar]
- Centola D . 2010 . The spread of behavior in an online social network experiment. Science 329 : 1194– 97 [Google Scholar]
- Charness G , Gneezy U . 2009 . Incentives to exercise. Econometrica 77 : 909– 31 [Google Scholar]
- Chetty R , Hendren N , Katz LF . 2015 . The effects of exposure to better neighborhoods on children: new evidence from the moving to opportunity experiment. Work. Pap. 21156, NBER, Cambridge, MA [Google Scholar]
- Chong D , Junn J . 2011 . Politics from the perspective of minority populations. Cambridge Handbook of Experimental Political Science JN Druckman, DP Green, JH Kuklinski, A Lupia, 602– 33 Cambridge, UK: Cambridge Univ. Press [Google Scholar]
- Cialdini RB , Ascani K . 1976 . Test of a concession procedure for inducing verbal, behavioral, and further compliance with a request to give blood. J. Pers. Soc. Psychol. 61 : 295– 300 [Google Scholar]
- Cialdini RB , Vincent JE , Lewis SK , Catalan J , Wheeler D , Darby BL . 1975 . Reciprocal concessions procedure for inducing compliance: the door-in-the-face technique. J. Pers. Soc. Psychol. 31 : 206– 15 [Google Scholar]
- Clampet-Lundquist S , Massey DS . 2008 . Neighborhood effects on economic self-sufficiency: a reconsideration of the Moving to Opportunity experiment. Am. J. Sociol. 114 : 107– 43 [Google Scholar]
- Cohen J , Dupas P . 2010 . Free distribution or cost-sharing? Evidence from a randomized malaria prevention experiment. Q. J. Econ. 125 : 1– 40 [Google Scholar]
- Cole S , Giné X , Tobacman J , Topalova P , Townsend R , Vickery J . 2013 . Barriers to household risk management: evidence from India. Am. Econ. J. Appl. Econ. 5 : 104– 35 [Google Scholar]
- Cook TD , Shadish WR . 1994 . Social experiments: some developments over the past fifteen years. Annu. Rev. Psychol. 45 : 545– 80 [Google Scholar]
- Correll SJ , Benard S , Paik I . 2007 . Getting a job: is there a motherhood penalty?. Am. J. Sociol. 112 : 1297– 339 [Google Scholar]
- Cox D . 1958 . Planning of Experiments New York: Wiley [Google Scholar]
- Crépon B , Devoto F , Duflo E , Parienté W . 2011 . Impact of microcredit in rural areas of Morocco: evidence from a randomized evaluation. Work. Pap., Dep. Econ., MIT [Google Scholar]
- Cross H , Kenney GM , Mell J , Zimmerman W . 1990 . Employer hiring practices: differential treatment of Hispanic and Anglo job seekers. Tech. rep., Urban Inst., Washington, DC [Google Scholar]
- Deaton A . 2010 . Instruments, randomization, and learning about development. J. Econ. Lit. 48 : 424– 55 [Google Scholar]
- Dehejia R , Pop-Eleches C , Samii C . 2015 . From local to global: external validity in a fertility natural experiment. Work. Pap. 21459, NBER, Cambridge, MA [Google Scholar]
- Doob AN , Gross AE . 1968 . Status as an inhibitor of horn-honking responses. J. Soc. Psychol. 76 : 213– 18 [Google Scholar]
- Druckman JN , Green DP , Kuklinski JH , Lupia A . 2011 . Cambridge Handbook of Experimental Political Science Cambridge, UK: Cambridge Univ. Press [Google Scholar]
- Duflo E , Kremer M , Robinson J . 2008 . How high are rates of return to fertilizer? Evidence from field experiments in Kenya. Am. Econ. Rev. 98 : 482– 88 [Google Scholar]
- Duflo E , Kremer M , Robinson J . 2011 . Nudging farmers to use fertilizer: theory and experimental evidence from Kenya. Am. Econ. Rev. 101 : 2350– 90 [Google Scholar]
- Dunn EW , Aknin LB , Norton MI . 2008 . Spending money on others promotes happiness. Science 319 : 1687– 88 [Google Scholar]
- Dunning T . 2012 . Natural Experiments in the Social Sciences: A Design-Based Approach Cambridge, UK: Cambridge Univ. Press [Google Scholar]
- Dupas P . 2009 . What matters (and what does not) in households’ decision to invest in malaria prevention?. Am. Econ. Rev. 99 : 224– 30 [Google Scholar]
- Dupas P . 2011 . Do teenagers respond to HIV risk information? Evidence from a field experiment in Kenya. Am. Econ. J. Appl. Econ. 3 : 1– 34 [Google Scholar]
- Dupas P . 2014 . Short-run subsidies and long-run adoption of new health products: evidence from a field experiment. Econometrica 82 : 197– 228 [Google Scholar]
- Dupas P , Robinson J . 2011 . Savings constraints and microenterprise development: evidence from a field experiment in Kenya. Work. Pap. 14693, NBER, Cambridge, MA [Google Scholar]
- Emswiller T , Deaux K , Willits JE . 1971 . Similarity, sex, and requests for small favors. J. Appl. Soc. Psychol. 1 : 284– 91 [Google Scholar]
- Enos RD . 2014 . Causal effect of intergroup contact on exclusionary attitudes. PNAS 111 : 3699– 704 [Google Scholar]
- Enos RD , Fowler A , Vavreck L . 2014 . Increasing inequality: the effect of GOTV mobilization on the composition of the electorate. J. Polit. 76 : 273– 88 [Google Scholar]
- Fearon JD , Humphreys M , Weinstein JM . 2009 . Can development aid contribute to social cohesion after civil war? Evidence from a field experiment in post-conflict Liberia. Am. Econ. Rev. 99 : 287– 91 [Google Scholar]
- Fearon JD , Humphreys M , Weinstein JM . 2015 . How does development assistance affect collective action capacity? Results from a field experiment in post-conflict Liberia. Am. J. Political Sci. 109 : 450– 69 [Google Scholar]
- Fershtman C , Gneezy U . 2001 . Discrimination in a segmented society: an experimental approach. Q. J. Econ. 116 : 351– 77 [Google Scholar]
- Fisher RA . 1935 . The Design of Experiments New York: Hafner [Google Scholar]
- Fiszbein A , Schady N . 2009 . Conditional cash transfers: reducing present and future poverty. World Bank Policy Res. Rep., World Bank Washington, DC: [Google Scholar]
- Forbes GB , Gromoll HF . 1971 . The lost letter technique as a measure of social variables: some exploratory findings. Soc. Forces 50 : 113– 15 [Google Scholar]
- Freedman JL , Fraser SC . 1966 . Compliance without pressure: the foot-in-the-door technique. J. Pers. Soc. Psychol. 4 : 195– 202 [Google Scholar]
- Freese J , Peterson D . 2017 . Replication in social science. Annu. Rev. Sociol. 43. In press [Google Scholar]
- Fryer R . 2011 . Financial incentives and student achievement: evidence from randomized trials. Q. J. Econ. 126 : 1755– 98 [Google Scholar]
- Gaddis SM . 2015 . Discrimination in the credential society: an audit study of race and college selectivity in the labor market. Soc. Forces 93 : 1451– 79 [Google Scholar]
- Gaddis SM , Ghoshal R . 2015 . Arab American housing discrimination, ethnic competition, and the contact hypothesis. Ann. Am. Acad. Political Soc. Sci. 660 : 282– 99 [Google Scholar]
- Galster G , Constantine P . 1991 . Discrimination against female-headed households in rental housing: theory and exploratory evidence. Rev. Soc. Econ. 49 : 76– 100 [Google Scholar]
- Gantner L . 2007 . PROGRESA: An integrated approach to poverty alleviation in Mexico. Case Studies in Food Policy for Developing Countries: Policies for Health, Nutrition, Food Consumption, and Poverty P Pinstrup-Andersen, F Cheng, Vol 1 211– 20 Ithaca, NY: Cornell Univ. Press [Google Scholar]
- Garfinkel H . 1967 . Studies in Ethnomethodology Englewood Cliffs, NJ: Prentice-Hall [Google Scholar]
- Gelman A . 2014 . Experimental reasoning in social science. Field Experiments and Their Critics: Essays on the Uses and Abuses of Experimentation in the Social Sciences DL Teele 185– 95 New Haven, CT: Yale Univ. Press [Google Scholar]
- Gerber AS . 2011 . Field experiments in political science. Cambridge Handbook of Experimental Political Science JN Druckman, DP Green, JH Kuklinski, A Lupia 115– 38 Cambridge, UK: Cambridge Univ. Press [Google Scholar]
- Gerber AS , Green DP . 2000 . The effects of canvassing, telephone calls, and direct mail on voter turnout: a field experiment. Am. Political Sci. Rev. 94 : 653– 63 [Google Scholar]
- Gerber AS , Green DP . 2012 . Field Experiments New York: Norton [Google Scholar]
- Gerber AS , Green DP , Larimer CW . 2008 . Social pressure and voter turnout: evidence from a large scale field experiment. Am. Political Sci. Rev. 102 : 33– 48 [Google Scholar]
- Gerber AS , Green DP , Shachar R . 2003 . Voting may be habit-forming: evidence from a randomized field experiment. Am. J. Political Sci. 47 : 540– 50 [Google Scholar]
- Gil-White F . 2004 . Ultimatum game with an ethnicity manipulation: results from Kohvdiin Bulgan Sum, Mongolia. Foundations of Human Sociality: Economic Experiments and Ethnographic Evidence from Fifteen Small-Scale Societies J Henrich, R Boyd, S Bowles, C Camerer, E Fehr, H Gintis, 260– 304 Oxford, UK: Oxford Univ. Press [Google Scholar]
- Gilligan MJ , Pasquale BJ , Samii C . 2014 . Civil war and social cohesion: lab-in-the-field evidence from Nepal. Am. J. Political Sci. 58 : 604– 19 [Google Scholar]
- Giné X , Karlan D . 2014 . Group versus individual liability: short and long term evidence from Philippine-microcredit lending groups. J. Dev. Econ. 107 : 65– 83 [Google Scholar]
- Giné X , Karlan D , Zinman J . 2010 . Put your money where your butt is: a commitment contract for smoking cessation. Am. Econ. J. Appl. Econ. 213– 35 [Google Scholar]
- Gneezy U , List J , Price MK . 2012 . Toward an understanding of why people discriminate: evidence from a series of natural field experiments. Work. Pap. 17855, NBER, Cambridge, MA [Google Scholar]
- Gneezy U , Meier S , Rey-Biel P . 2011 . When and why incentives (don't) work to modify behavior. J. Econ. Perspect. 25 : 191– 210 [Google Scholar]
- Gneezy U , Rey-Biel P . 2014 . On the relative efficiency of performance pay and noncontingent incentives. J. Eur. Econ. Assoc. 12 : 62– 72 [Google Scholar]
- Gneezy U , Rustichini A . 2000 . A fine is a price. J. Legal Stud. 29 : 1– 17 [Google Scholar]
- Goel V . 2014 . Facebook tinkers with users’ emotions in news feed experiment, stirring outcry. New York Times , June 30 B1
- Gosnell HF . 1927 . Getting Out the Vote: An Experiment in the Stimulation of Voting Chicago: Chicago Univ. Press [Google Scholar]
- Green DP , Gerber A . 2008 . Get Out the Vote: How to Increase Voter Turnout Washington, DC: Brookings Inst. Press. 2nd ed. [Google Scholar]
- Green DP , Wong J . 2009 . Tolerance and the contact hypothesis: a field experiment. The Political Psychology of Democratic Citizenship 228– 46 Oxford, UK: Oxford Univ. Press [Google Scholar]
- Greenberg D , Shroder M . 2004 . The Digest of Social Experiments. Washington, DC: Urban Inst. Press [Google Scholar]
- Grose CR . 2014 . Field experimental work on political institutions. Annu. Rev. Political Sci. 17 : 355– 70 [Google Scholar]
- Grossman G , Baldassarri D . 2012 . The impact of elections on cooperation: evidence from a lab in the field experiment in Uganda. Am. J. Political Sci. 56 : 964– 85 [Google Scholar]
- Grossman G , Paler L . 2015 . Using experiments to study political institutions. Handbook of Comparative Political Institutions J Gandhi, R Ruiz-Rufino 84– 97 London: Routledge [Google Scholar]
- Habyarimana J , Humphreys M , Posner DN , Weinstein JM . 2009 . Coethnicity: Diversity and the Dilemmas of Collective Action New York: Russell Sage Found. [Google Scholar]
- Harrison GW . 2013 . Field experiments and methodological intolerance. J. Econ. Methodol. 20 : 103– 17 [Google Scholar]
- Harrison GW , List JA . 2004 . Field experiments. J. Econ. Lit. 42 : 1009– 55 [Google Scholar]
- Hausman JA , Wise DA . 1985 . Social Experimentation Chicago: Chicago Univ. Press [Google Scholar]
- Heckman JJ . 1992 . Randomization and social policy evaluation. Evaluating Welfare and Training Programs CF Manski, I Garfinkel 201– 30 Cambridge, MA: Harvard Univ. Press [Google Scholar]
- Heckman JJ . 1998 . Detecting discrimination. J. Econ. Perspect. 12 : 101– 16 [Google Scholar]
- Heckman JJ , Siegelman P . 1993 . The Urban Institute audit studies: their methods and findings. Clear and Convincing Evidence: Measurement of Discrimination in America M Fix, RJ Struyk 187– 258 Washington, DC: Urban Inst. Press [Google Scholar]
- Henrich J , Boyd R , Bowles S , Camerer C , Fehr E . et al. 2001 . In search of homo economicus: behavioral experiments in 15 small-scale societies. Am. Econ. Rev. 91 : 73– 78 [Google Scholar]
- Henrich J , Ensminger J , McElreath R , Barr A , Barrett C . et al. 2010 . Markets, religion, community size, and the evolution of fairness and punishment. Science 327 : 1480– 84 [Google Scholar]
- Henrich J , McElreath R , Barr A , Ensminger J , Barrett C . et al. 2006 . Costly punishment across human societies. Science 312 : 1767– 70 [Google Scholar]
- Henry PJ . 2008 . College sophomores in the laboratory redux: influences of a narrow data base on social psychology's view of the nature of prejudice. Psychol. Inq. 19 : 49– 71 [Google Scholar]
- Herberich DH , List JA , Price MK . 2011 . How many economists does it take to change a light bulb? A natural field experiment on technology adoption Work. Pap., Univ. Chicago [Google Scholar]
- Heyman J , Ariely D . 2004 . Effort for payment: a tale of two markets. Psychol. Sci. 15 : 787– 93 [Google Scholar]
- Holland J , Silva AS , Mace R . 2012 . Lost letter measure of variation in altruistic behaviour in 20 neighbourhoods. PLOS ONE 7 : e43294 [Google Scholar]
- Houlette MA , Gaertner SL , Johnson KM , Banker BS , Riek BM , Dovidio JF . 2004 . Developing a more inclusive social identity: an elementary school intervention. J. Soc. Issues 60 : 35– 55 [Google Scholar]
- Humphreys M , Sanchez de la Sierra R , van der Windt P . 2013 . Fishing, commitment, and communication: a proposal for comprehensive nonbinding research registration. Polit. Anal. 21 : 1– 20 [Google Scholar]
- Imbens G , Wooldridge J . 2009 . Recent developments in the econometrics of program evaluation. J. Econ. Lit. 47 : 5– 86 [Google Scholar]
- Isen AM , Levin PF . 1972 . Effect of feeling good on helping: cookies and kindness. J. Pers. Soc. Psychol. 21 : 384– 88 [Google Scholar]
- Jackson M , Cox DR . 2013 . The principles of experimental design and their application in sociology. Annu. Rev. Sociol. 39 : 27– 49 [Google Scholar]
- Jensen R , Miller N . 2008 . Giffen behavior and subsistence consumption. Am. Econ. Rev. 98 : 1553– 77 [Google Scholar]
- Kamenica E . 2012 . Behavioral economics and psychology of incentives. Annu. Rev. Econ. 4 : 427– 52 [Google Scholar]
- Karlan D . 2005 . Using experimental economics to measure social capital and predict financial decisions. Am. Econ. Rev. 95 : 1688– 99 [Google Scholar]
- Karlan D , Appel J . 2011 . More Than Good Intentions: Improving the Ways the World's Poor Borrow, Save, Farm, Learn, and Stay Healthy New York: Penguin [Google Scholar]
- Karlan D , Goldberg N . 2011 . Microfinance evaluation strategies: notes on methodology and findings. The Handbook of Microfinance B Armendáriz, M Labie 17– 58 London: World Scientific [Google Scholar]
- Karlan D , McConnell M , Mullainathan S , Zinman J . 2014 . Getting to the top of mind: how reminders increase saving. Manag. Sci. 62 : 3393– 3411 [Google Scholar]
- Karlan D , Osei-Akoto I , Osei R , Udry C . 2010 . Examining underinvestment in agriculture: measuring returns to capital and insurance. Work. Pap., Abdul Latif Jameel Poverty Action Lab. https://www.poverty-action.org/sites/default/files/Panel3-3-Farmers-Returns-Capital.pdf [Google Scholar]
- Karlan D , Zinman J . 2011 . Microcredit in theory and practice: using randomized credit scoring for impact. Science 332 : 1278– 84 [Google Scholar]
- Keizer K , Lindenberg S , Steg L . 2008 . The spreading of disorder. Science 322 : 1681– 85 [Google Scholar]
- Kelly E , Moena P , Oakes J , Fan W , Okechukwu C . et al. 2014 . Changing work and work-family conflict: evidence from the work, family, and health network. Am. Sociol. Rev. 79 : 485– 516 [Google Scholar]
- Kling JR , Liebman JB , Katz LF . 2007 . Experimental analysis of neighborhood effects. Econometrica 75 : 83– 119 [Google Scholar]
- Kotran A . 2015 . Opower and utility partners save over eight terawatt-hours of energy power and utility partners save over eight terawatt-hours of energy. News release, May 21
- Kramer ADI , Guillory JE , Hancock JT . 2014 . Experimental evidence of massive-scale emotional contagion through social networks. PNAS 111 : 8788– 90 [Google Scholar]
- Kremer M . 2003 . Randomized evaluations of educational programs in developing countries: some lessons. Am. Econ. Rev. 93 : 102– 6 [Google Scholar]
- Kremer M , Brannen C , Glennerster R . 2013 . The challenge of education and learning in the developing world. Science 340 : 297– 300 [Google Scholar]
- Kremer M , Leino J , Miguel E , Zwane AP . 2011 . Spring cleaning: rural water impacts, valuation, and property rights institutions. Q. J. Econ. 126 : 145– 205 [Google Scholar]
- Kugelmass H . 2016 . “Sorry, I'm not accepting new patients”: an audit study of access to mental health care. J. Health Soc. Behav. 57 : 168– 83 [Google Scholar]
- Lacetera N , Macis M . 2010 . Do all material incentives for pro-social activities backfire? The response to cash and non-cash incentives for blood donations. J. Econ. Psychol. 31 : 738– 48 [Google Scholar]
- Lacetera N , Macis M , Slonim R . 2013 . Economic rewards to motivate blood donations. Science 340 : 927– 28 [Google Scholar]
- Landry CE , Lange A , List JA , Price MK , Rupp NG . 2010 . Is a donor in hand better than two in the bush? Evidence from a natural field experiment. Am. Econ. Rev. 100 : 958– 83 [Google Scholar]
- Langer EJ , Rodin J . 1976 . The effects of choice and enhanced responsibility for the aged: a field experiment in an institutional setting. J. Pers. Soc. Psychol. 34 : 191– 98 [Google Scholar]
- Lauster N , Easterbrook A . 2011 . No room for new families? A field experiment measuring rental discrimination against same-sex couples and single parents. Soc. Probl. 58 : 389– 409 [Google Scholar]
- Leuven E , Oosterbeek H , van der Klaauw B . 2010 . The effect of financial rewards on students’ achievement: evidence from a randomized experiment. J. Eur. Econ. Assoc. 8 : 1243– 65 [Google Scholar]
- Levine M , Prosser A , Evans D , Reicher S . 2005 . Identity and emergency intervention: how social group membership and inclusiveness of group boundaries shape helping behavior. Pers. Soc. Psychol. Bull. 31 : 443– 53 [Google Scholar]
- Levitt SD , List JA . 2009 . Field experiments in economics: the past, the present, and the future. Eur. Econ. Rev. 53 : 1– 18 [Google Scholar]
- Levitt SD , List JA , Neckerman S , Sadoff S . 2012 . The behavioralist goes to school: leveraging behavioral economics to improve educational performance. Work. Pap. 18165, NBER Cambridge, MA: [Google Scholar]
- List JA . 2007 . Field experiments: a bridge between lab and naturally occurring data. B.E. J. Econ. Anal. Policy 5 : 2 [Google Scholar]
- Lucas JW . 2003 . Theory-testing, generalization, and the problem of external validity. Sociol. Theory 21 : 236– 53 [Google Scholar]
- Ludwig J , Duncan GJ , Gennetian LA , Katz LF , Kessler RC . et al. 2013 . Long-term neighborhood effects on low-income families: evidence from moving to opportunity. Am. Econ. Rev. 103 : 226– 31 [Google Scholar]
- Ludwig J , Liebman JB , Kling JR , Duncan GJ , Katz LF . et al. 2008 . What can we learn about neighborhood effects from the moving to opportunity experiment?. Am. J. Sociol. 114 : 144– 88 [Google Scholar]
- Marwell G , Ames RE . 1979 . Experiments on the provision of public goods: resources, interest, group size, and the free-rider problem. Am. J. Sociol. 84 : 1335– 60 [Google Scholar]
- Massey DS , Lundy G . 2001 . Use of Black English and racial discrimination in urban housing markets: new methods and findings. Urban Aff. Rev. 36 : 452– 69 [Google Scholar]
- McDermott R . 2011 . Internal and external validity. Cambridge Handbook of Experimental Political Science JN Druckman, DP Green, JH Kuklinski, A Lupia, 27– 40 Cambridge, UK: Cambridge Univ. Press [Google Scholar]
- McEwan PJ . 2015 . Improving learning in primary schools of developing countries: a meta-analysis of randomized experiments. Rev. Educ. Res. 85 : 353– 94 [Google Scholar]
- McNutt M . 2015 . Editorial retraction of Lacour & Green. Science 346 : 1366– 69 Science 348 : 1100 [Google Scholar]
- Merton RK . 1945 . Sociological theory. Am. J. Sociol. 50 : 462– 73 [Google Scholar]
- Michelson M , Nickerson DW . 2011 . Voter Mobilization Cambridge, UK: Cambridge Univ. Press [Google Scholar]
- Miguel E , Kremer M . 2004 . Worms: identifying impacts on education and health in the presence of treatment externalities. Econometrica 72 : 159– 217 [Google Scholar]
- Milgram S , Liberty HJ , Toledo R , Wackenhut J . 1986 . Response to intrusion into waiting lines. J. Pers. Soc. Psychol. 51 : 683– 89 [Google Scholar]
- Milgram S , Mann L , Hartner S . 1965 . The lost letter technique: a tool of social research. Public Opin. Q. 29 : 437– 38 [Google Scholar]
- Milkman KL , Akinola M , Chugh D . 2015 . What happens before? A field experiment exploring how pay and representation differentially shape bias on the pathway into organizations. J. Appl. Psychol. 100 : 1678– 712 [Google Scholar]
- Milkman KL , Beshears J , Choi JJ , Laibson D , Madrian BC . 2011 . Using implementation intentions prompts to enhance influenza vaccination rates. PNAS 108 : 10415– 20 [Google Scholar]
- Morgan S , Winship C . 2007 . Counterfactuals and Causal Inference Cambridge, UK: Cambridge Univ. Press [Google Scholar]
- Morton R , Williams K . 2010 . Experimental Political Science and the Study of Causality Cambridge, UK: Cambridge Univ. Press [Google Scholar]
- Moss-Racusin CA , Dovidio JF , Brescoll V , Graham MJ , Handelsman J . 2012 . Science faculty's subtle gender biases favor male students. PNAS 109 : 16474– 79 [Google Scholar]
- Munnell AH . 1986 . Lessons from the Income Maintenance Experiments Boston: Fed. Res. Bank of Boston [Google Scholar]
- Mutz DC . 2011 . Population-Based Survey Experiments Princeton, NJ: Princeton Univ. Press [Google Scholar]
- Nagda BRA , Tropp LR , Paluck EL . 2006 . Looking back as we look ahead: integrating research, theory, and practice on intergroup relations. J. Soc. Issues 62 : 439– 51 [Google Scholar]
- Neumark D , Bank RJ , Nort KDV . 1996 . Sex discrimination in restaurant hiring: an audit study. Q. J. Econ. 111 : 915– 41 [Google Scholar]
- Nickerson DW . 2008 . Is voting contagious? Evidence from two field experiments. Am. Political Sci. Rev. 102 : 49– 57 [Google Scholar]
- Nolan JM , Kenefick J , Schultz PW . 2011 . Normative messages promoting energy conservation will be underestimated by experts unless you show them the data. Soc. Influence 6 : 169– 80 [Google Scholar]
- Nolan JM , Schultz PW , Cialdini RB , Goldstein NJ , Griskevicius V . 2008 . Normative social influence is underdetected. Pers. Soc. Psychol. Bull. 34 : 913– 23 [Google Scholar]
- Nosek B , Aarts A , Anderson J , Anderson C , Attridge P . et al. 2015a . Estimating the reproducibility of psychological science. Science 349 : 943– 51 [Google Scholar]
- Nosek B , Alter G , Banks G , Borsboom D , Bowman S . et al. 2015b . Promoting an open research culture. Science 348 : 1422– 25 [Google Scholar]
- Olken B . 2007 . Monitoring corruption: evidence from a field experiment in Indonesia. J. Political Econ. 115 : 200– 49 [Google Scholar]
- Olken B . 2010 . Direct democracy and local public goods: evidence from a field experiment in Indonesia. Am. Political Sci. Rev. 104 : 243– 67 [Google Scholar]
- Pager D . 2003 . The mark of a criminal record. Am. J. Sociol. 108 : 937– 75 [Google Scholar]
- Pager D . 2007 . The use of field experiments for studies of employment discrimination: contributions, critiques, and directions for the future. Ann. Am. Acad. Political Soc. Sci. 609 : 104– 33 [Google Scholar]
- Pager D , Quillian L . 2005 . Walking the talk: what employers say versus what they do. Am. Sociol. Rev. 70 : 355– 80 [Google Scholar]
- Pager D , Western B , Bonikowski B . 2009 . Discrimination in a low-wage labor market: a field experiment. Am. Sociol. Rev. 74 : 777– 99 [Google Scholar]
- Paluck EL . 2009 . Reducing intergroup prejudice and conflict using the media: a field experiment in Rwanda. Interpers. Relat. Group Process. 96 : 574– 87 [Google Scholar]
- Paluck EL , Cialdini RB . 2014 . Field research methods. Handbook of Research Methods in Social and Personality Psychology HT Reis, CM Judd 81– 97 New York: Cambridge Univ. Press, 2nd ed.. [Google Scholar]
- Paluck EL , Green DP . 2009 . Prejudice reduction: what works? A review and assessment of research and practice. Annu. Rev. Psychol. 60 : 339– 67 [Google Scholar]
- Paluck EL , Shepherd H . 2012 . The salience of social referents: a field experiment on collective norms and harassment behavior in a school social network. J. Pers. Soc. Psychol. 103 : 899– 915 [Google Scholar]
- Paluck EL , Shepherd H , Aronow PM . 2016 . Changing climates of conflict: a social network driven experiment in 56 schools. PNAS 113 : 566– 71 [Google Scholar]
- Pedulla DS . 2016 . Penalized or protected? Gender and the consequences of non-standard and mismatched employment histories. Am. Sociol. Rev. 81 : 262– 89 [Google Scholar]
- Pettigrew TF . 1998 . Intergroup contact theory. Annu. Rev. Psychol. 49 : 65– 85 [Google Scholar]
- Riach PA , Rich J . 2002 . Field experiments of discrimination in the market place. Econ. J. 112 : 480– 518 [Google Scholar]
- Rodríguez-Planas N . 2012 . Longer-term impacts of mentoring, educational services, and learning incentives: evidence from a randomized trial in the United States. Am. Econ. J. Appl. Econ. 4 : 121– 39 [Google Scholar]
- Rondeau D , List JA . 2008 . Matching and challenge gifts to charity: evidence from laboratory and natural field experiments. Exp. Econ. 11 : 253– 67 [Google Scholar]
- Ross SL , Turner MA . 2005 . Housing discrimination in metropolitan America: explaining changes between 1989 and 2000. Soc. Probl. 52 : 152– 80 [Google Scholar]
- Rossi PH , Berk RA , Lenihan KJ . 1980 . Money, Work, and Crime: Experimental Evidence New York: Academic Press [Google Scholar]
- Rossi PH , Berk RA , Lenihan KJ . 1982 . Saying it wrong with figures: a comment on Zeisel. Am. J. Sociol. 88 : 390– 93 [Google Scholar]
- Rossi PH , Lyall KC . 1978 . An overview evaluation of the NIT experiment. Eval. Stud. Rev. 3 : 412– 28 [Google Scholar]
- Sabin N . 2015 . Modern microfinance: a field in flux. Social Finance Nicholls A, Paton R, Emerson J Oxford, UK: Oxford Univ. Press [Google Scholar]
- Salganik MJ , Dodds PS , Watts DJ . 2006 . Experimental study of inequality and unpredictability in an artificial cultural market. Science 311 : 854– 56 [Google Scholar]
- Sampson RJ . 2008 . Moving to inequality: neighborhood effects and experiments meet social structure. Am. J. Sociol. 114 : 189– 231 [Google Scholar]
- Sampson RJ . 2012 . Great American City: Chicago and the Enduring Neighborhood Effect Chicago, IL: Chicago Univ. Press [Google Scholar]
- Schuler SR , Hashemi SM , Badal SH . 1998 . Men's violence against women in rural Bangladesh: undermined or exacerbated by microcredit programmes?. Dev. Pract. 8 : 148– 57 [Google Scholar]
- Schultz P . 2004 . School subsidies for the poor: evaluating the Mexican Progresa poverty program. J. Dev. Econ. 74 : 199– 250 [Google Scholar]
- Shadish WR , Cook TD . 2009 . The renaissance of field experimentation in evaluating interventions. Annu. Rev. Psychol. 607– 29 [Google Scholar]
- Shadish WR , Cook TD , Campbell DT . 2002 . Experimental and Quasi-experimental Designs for Generalized Causal Inference. New York: Houghton, Mifflin and Company [Google Scholar]
- Simpson BT , McGrimmon T , Irwin K . 2007 . Are blacks really less trusting than whites? Revisiting the race and trust question. Soc. Forces 86 : 525– 52 [Google Scholar]
- Sniderman PM , Grob DB . 1996 . Innovations in experimental design in attitude surveys. Annu. Rev. Sociol. 22 : 377– 99 [Google Scholar]
- Steinpreis RE , Anders KA , Ritzke D . 1999 . The impact of gender on the review of the curricula vitae of job applicants and tenure candidates: a national empirical study. Sex Roles 41 : 509– 28 [Google Scholar]
- Stutzer A , Goette L , Zehnder M . 2011 . Active decisions and prosocial behaviour: a field experiment on blood donations. Econ. J. 121 : 476– 93 [Google Scholar]
- Teele DL . 2014 . Reflections on the ethics of field experiments. Field Experiments and Their Critics: Essays on the Uses and Abuses of Experimentation in the Social Sciences DL Teele 115– 40 New Haven, CT: Yale Univ. Press [Google Scholar]
- Thornton RL . 2008 . The demand for, and impact of, learning HIV status. Am. Econ. Rev. 98 : 1829– 63 [Google Scholar]
- Tilcsik A . 2011 . Pride and prejudice: employment discrimination against openly gay men in the United States. Am. J. Sociol. 117 : 586– 626 [Google Scholar]
- Travers J , Milgram S . 1969 . An experimental study of the small world problem. Sociometry 32 : 425– 43 [Google Scholar]
- Turner MA , Bednarz BA , Herbig C , Lee SJ . 2003 . Discrimination in metropolitan housing markets phase 2: Asians and Pacific Islanders Tech. rep., Urban Inst., Washington, DC [Google Scholar]
- Turner MA , Fix M , Struyk RJ . 1991 . Opportunities Denied, Opportunities Diminished: Racial Discrimination in Hiring Washington, DC: Urban Inst. Press [Google Scholar]
- Turner MA , Ross SL , Galster GC , Yinger J . 2002 . Discrimination in metropolitan housing markets: national results from phase 1 of the Housing Discrimination Study (HDS) Tech. rep., Urban Inst Washington, DC: [Google Scholar]
- Van Bavel JJ , Mende-Siedlecki P , Brady WJ , Reinero DA . 2016 . Contextual sensitivity in scientific reproducibility. PNAS 113 : 6454– 59 [Google Scholar]
- Van de Rijt A , Kang SM , Restivo M , Patil A . 2014 . Field experiments of success-breeds-success dynamics. PNAS 111 : 6934– 39 [Google Scholar]
- Van Der Merwe WG , Burns J . 2008 . What's in a name? Racial identity and altruism in post-apartheid South Africa. South Afr. J. Econ. 76 : 266– 75 [Google Scholar]
- Vermeersch C , Kremer M . 2005 . School Meals, Educational Achievement, and School Competition: Evidence from a Randomized Evaluation. New York: World Bank [Google Scholar]
- Volpp KG , Troxel AB , Pauly MV , Glick HA , Puig A . et al. 2009 . A randomized, controlled trial of financial incentives for smoking cessation. N. Engl. J. Med. 360 : 699– 709 [Google Scholar]
- Whitt S , Wilson RK . 2007 . The dictator game, fairness and ethnicity in postwar Bosnia. Am. J. Political Sci. 51 : 655– 68 [Google Scholar]
- Wienk RE , Reid CE , Simonson JC , Eggers FJ . 1979 . Measuring racial discrimination in American housing markets: the housing market practices survey. Tech. Rep. HUD-PDR-444(2), Dep. Hous. Urban Dev Washington, DC: [Google Scholar]
- Williams WM , Ceci SJ . 2015 . National hiring experiments reveal 2:1 faculty preference for women on STEM tenure track. PNAS 112 : 5360– 65 [Google Scholar]
- Yamagishi T . 2011 . Trust: The Evolutionary Game of Mind and Society New York: Springer [Google Scholar]
- Yamagishi T , Cook KS , Watabe M . 1998 . Uncertainty, trust, and commitment formation in the United States and Japan. Am. J. Sociol. 104 : 165– 94 [Google Scholar]
- Zeisel H . 1982 . Disagreement over the evaluation of a controlled experiment. Am. J. Sociol. 88 : 378– 89 [Google Scholar]
Data & Media loading...
- Article Type: Review Article
Most Read This Month
Most cited most cited rss feed, birds of a feather: homophily in social networks, social capital: its origins and applications in modern sociology, conceptualizing stigma, framing processes and social movements: an overview and assessment, organizational learning, the study of boundaries in the social sciences, assessing “neighborhood effects”: social processes and new directions in research, social exchange theory, culture and cognition, focus groups.
Reactivity in social scientific experiments: what is it and how is it different (and worse) than a Placebo effect?
- Paper in the Philosophy of the Social Sciences and Humanities
- Open access
- Published: 20 April 2021
- Volume 11 , article number 42 , ( 2021 )
Cite this article
You have full access to this open access article
- María Jiménez-Buedo 1
7025 Accesses
12 Citations
5 Altmetric
Explore all metrics
Reactivity, or the phenomenon by which subjects tend to modify their behavior in virtue of their being studied upon, is often cited as one of the most important difficulties involved in social scientific experiments, and yet, there is to date a persistent conceptual muddle when dealing with the many dimensions of reactivity. This paper offers a conceptual framework for reactivity that draws on an interventionist approach to causality. The framework allows us to offer an unambiguous definition of reactivity and distinguishes it from placebo effects. Further, it allows us to distinguish between benign and malignant forms of the phenomenon, depending on whether reactivity constitutes a danger to the validity of the causal inferences drawn from experimental data.
Similar content being viewed by others
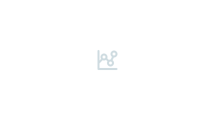
Reactivity in the human sciences
Towards an account of the placebo effect: a critical evaluation alongside current evidence, an overview of scientific reproducibility: consideration of relevant issues for behavior science/analysis.
Avoid common mistakes on your manuscript.
1 Introduction
The surge in social scientific experimentation of the last years has been in great part driven by the success of experimental and behavioral economics. It is natural then that the methodological discussions around new experimental practices in the social sciences have often been shaped by the debates that were taking place among practicing experimental economists.
In the first few decades after the emergence of new experimental practices in the social sciences, then, the question of reactivity, or the phenomenon that occurs when individuals alter their behavior because of their awareness of being studied, has not been central to the discussions of methodologists or practitioners, partly because economists were not crucially concerned by it.
With their clear-cut methodological stance shaped most importantly by a tenacious control over the incentives faced by participants in the experimental setting, experimental economists may have initially felt that their experiments were shielded from the worries associated with subjects’ reactivity that had long haunted their fellow social psychologist experimenters. More recently, experimental economists gradually moved in their study toward topics in which economic incentives no longer dominated the structure of a given game, but instead were intermingled with normative considerations (such as in the study of altruism, punishment, or social norms). Following this developments, a corresponding interest in the problem of reactivity has ensued among experimental economists.
In particular, the question of reactivity, under its multiple conceptual variants, has gained the attention of important experimentalists regarding the Dictator Game (DG) Footnote 1 and other similarly abstract designs aimed at measuring the normative inclinations of subjects. While the standard DG results, in which a number of “dictators” share their money with complete strangers has traditionally been interpreted widely as evidence of prosocial behavior, a number of important works that came out around the same time started disputing this interpretation, and instead suggested that the high level of donations observed was more likely indicative of the existence of artefacts: thus, for authors such as Bardsley ( 2008 ), Zizzo ( 2010 ), Dana et al ( 2007 ), and List ( 2007 ), the fact that a majority of DG subjects were willing to share a significant amount of their endowments with their fellow players was because the game was too transparently "about giving", and thus experimental subjects could easily guess what was expected of them and acted accordingly..
In this way, and according to critics, players in the DG are merely trying to perform the role of “good subjects” by adjusting their behavior to expectations, or, more specifically, adjusting to what they think it is expected of them as subjects (Bardsley 2008 ; Zizzo 2010 ). Alternatively, others have argued that relevant inferences from the DG and other similarly abstract games are still possible: both in the lab and in the field, subjects' behavior depends on other people's expectations and thus the DG provides a useful setting to study how subjects choose to adhere to the normative cues that the experimental setting provides (Levitt and List 2007 , Jimenez-Buedo and Guala 2016 ).
Despite the shadow of the artifact over the DG, the game continues to be enacted in the growing number of social science experimental labs that have been set up in the last few years, coinciding with the extraordinary growth of experimental methods across the social scientific disciplines. There remains an open question regarding what can be inferred, if anything, from subjects’ behavior in the standard DG or its variants. Can the DG results be used to explain phenomena outside the lab, and if so, which are those phenomena? Can we use the DG results to explain why people do things like give money to charities or is the behavior of DG players only meaningful (and relevant) inside the lab?
This paper argues that the debate about the validity of results of the DG and related games is stymied by the ambiguities that surround the concept of reactivity, as there are a number of unresolved conceptual issues regarding the phenomenon of reactivity. In this paper, we address two of these conceptual ambiguities.
First, there are a number of terms that are used to refer to what we here conceptualize as the phenomenon of reactivity, though they often are used without clarifying their definitions and, more importantly, they are often used interchangeably. In this way, Hawthorne effects, placebo effects, demand effects of experimentation, experimenter demand effects, methodological artifact, social desirability bias, are all terms that are often used in a loose way to invoke what we refer to as reactivity, or the phenomenon by which subjects in an experiment tend to modify their behavior in virtue of their awareness of being under study.
For example, and as we will see again in the next section, this is apparent in the debate around the validity of inferences from the DG, where an array of terms have been used often interchangeably to refer, in turn, to an array of ambiguously defined phenomena related to reactivity. In this way, and though there are many possible mechanisms for what we here call reactivity (such as the desire of subjects to comply with experimenter's expectations; their capacity to correctly guess the object of the experiment; the queasiness or apprehension of subjects to being evaluated; the fact that some subjects may try to deceive experimenters about their true motives for action; and the fact that experimenters or experimental designs may involuntarily give out cues about what behavior is expected of subjects), we here try to provide a unifying framework that subsumes the commonalities of these phenomena under the umbrella term of reactivity.
Second, and relatedly, there is the issue of whether the type of phenomena or mechanisms mentioned above invalidate an experiment’s inferential import or whether instead, they only constitute a potential threat to the validity of inferences from an experiment. Again, because the definitions of terms such as Hawthorne effects, demand effects, and the like are often used without being standardized or operationalized, these terms are used interchangeably both to define the phenomenon associated with reactivity and to refer to the invalidation of an experiment’s results due to the existence of reactive effects. This, again, creates confusion around the validity of experiments whenever we know or suspect that reactivity is at work in any given experiment. Here, we provide a framework in which we specify the conditions under which the existence of reactivity poses a threat to our capacity to draw causal conclusions from experiments.
In the pages that follow we provide a behavioral definition of reactivity. We offer an interventionist framework (Woodward 2003 ) that subsumes the phenomena associated with reactivity under a unifying conceptual scheme.. This framework allows us both to define unambiguously the notion of reactivity, and to analyse the challenges that reactivity can pose to causal inference in experiments with humans. Footnote 2 To this avail, we introduce a distinction between malignant and benign forms of reactivity, in terms of the effects that reactivity can have on the validity of causal inferences drawn from experimental results. We argue that malignant forms of reactivity have the potential to render findings causally uninterpretable and we have reason to suspect that they do so whenever the effects of reactivity are idiosyncratic, i.e., whenever reactive effects cannot be assumed to be equal across the control and the treatment groups. Finally, our framework allows us to differentiate between reactivity and placebo effects.
Our paper also argues that clarifying this concept and the related set of phenomena that it describes therefore constitutes a valuable contribution to the debate about the limits of social science experimentation.
2 Reactivity again
In the early years of the experimental economics, when the focus was exclusively on the study of market institutions, experimental economists may have felt that their experiments were shielded from the worries associated with subjects’ reactivity. This was due partly to the fact that experimental economics, born as a means to study the economic phenomena such as the clearing of markets, could adhere to a series of methodological principles, synthesized by Vernon Smith’s precepts (Smith 1982), Footnote 3 and meant as a list of rules that provided sufficient conditions for the validity of experiments. Of these six principles, four of them were related to the need of adherence to strictly structured monetary incentives. Most importantly, the principle of dominance dictated that incentives had to dominate over any other subjective costs associated with participation in the experiment, thus creating a stark methodological barrier between the practices of economists and other more traditional experimental practices in psychology.
Gradually, the practices of experimental economists converged with those of behavioral economists (who themselves had a history of cross-collaboration with psychologists) and this convergence crystallized in a methodological synthesis in which there was a clear relaxation of some of the Smithian precepts. Yet, there was still the perception that economists and psychologists differed systematically in their methodological practices, as summarized in the classic Hertwig and Ortmann piece ( 2001 ). Following Hertwig and Ortmann, these practices (the proscription of deception, the use of well-defined scripts, and the repetition of tasks), together with the use of monetary incentives, were defining features of the experiments in economics, as compared to those of psychologists. None of these practices were in themselves warrants against reactivity but they may have, collectively, during some years, given a sense of protection against the perils of reactivity to a profession that was gradually and increasingly adopting experimental practices.
As experiments became common within the discipline of economics, experimenters in economics broadened the array of topics that they dealt with. Gradually their topics included, prominently, questions regarding pro-social behavior, but in these games, by construction, monetary incentives needed to be weighed against other (pro-social) considerations: they could no longer completely dominate the incentives of the players (Jimenez-Buedo 2015 ). Against this background, and as we already pointed out in the introduction, the success of games such as the DG and the ensuing debate over the correct interpretation of its results eventually brought the question of reactivity to the fore of the methodological discussion among economists.
Initially, the critics of standard interpretations of the Dictator Game results resorted to standard terminology used in more traditionally experimental disciplines, such as psychology. For example, as already mentioned above, Bardsley ( 2008 ) resorted to the concept of Hawthorne effects in his criticism of altruistic interpretations of the DG results. The term of Hawthorne effects, with origins in industrial organizational studies, is normally used to refer to the fact that subjects may try to “overperform” when they are being observed. Footnote 4 Because its definition is not standardized, it is also often used to refer to the subject’s sensivity to being observed and sometimes also to refer to the behavioral changes that are considered to be a direct response to the experimenter’s scrutiny.
Among the DG critics, Zizzo ( 2010 ) provides his own conceptual approach to the issue, and coined what is now the standard terminology in economics. Zizzo defined experimenter demand effects (2010) as the changes in behavior by experimental subjects due to cues about what constitutes appropriate behavior. According to Zizzo, experimenter demand effects can be either purely cognitive (when an experimental participant tries to figure out what she is expected to do as an experimental subject), or they can also have an additional social layer, when that elucidation is additionally shaped by a sense of social adequateness.
Moreover, Zizzo’s conceptual scheme also provided an account of the way in which experimenter demand effects could affect the validity of experiments. According to his framework experimenter demand effects are a problem for the validity of experiments whenever experimental participants can correctly guess the true experimental objectives.
The term experimenter demand effects has been very extremely influential among experimental economists, and due to the influence of economics in the new wave of social science experimentalism, it is already permeating the language of experimentalists in other social sciences, such as sociology and political science, thus constituting the new conceptual standard. The term experimenter demand effects constitutes in itself a sort of terminological synthesis with respect to preexisting terms in social psychology, by merging two classic terms: experimenter effects, and demand effects of experimentation. These other two terms constituted two important tenets in the lingo that originated in social psychology in the 1960s and 1970s and that has conformed, for years, the vocabulary of social scientific experimentalists: the synthesis would come from merging together, in one term, Orne’s demand characteristics of experimentation and Rosenthal’s experimenter (expectancy) effects. In the case of the former, Orne ( 1962 , 1969 ) studied, both theoretically and empirically, how experimental subjects actively contribute to complete and construe the experimental task by enquiring and hypothesizing what is expected of them as experimental subjects. For Orne, this is an inherent feature of social scientific experimentation, since experimental instructions are necessarily incomplete: the experiment is itself a social situation that exerts implicit demands on the social actors involved in it. These implicit demands are worthy of study by social psychologists (thus, Orne’s and other’s project of a Social Psychology of Experimentation). More practically, Orne also considered that these demands need to be analyzed by experimenters because they have the potential to interfere with the (more explicit) experimental task that is the experimentalist’s primary object of research. Rosenthal’s experimenter expectancy effects (1968), in turn, refer to the set of cues regarding the experiment’s objectives or hypotheses that experimenters can inadvertently send to participants, and that can end up affecting the experiment’s results. In this way, and by focusing on experimenter demand effects, Zizzo merges both of these traditions in how he conceptualizes these effects: these are changes in the behavior of experimental subjecs due to (experimenter) cues about what constitutes appropriate behavior (“demanded” from them).
Zizzo classifies demand effects on the basis of whether subjects correctly or incorrectly guess the true goal of the experiment. Thus, depending on the coincidence between what the subjects believe about the experiment and what the experiment really is meant to test, we have three possible cases:
Uncorrelated expected and true objectives
Negatively correlated expected and true objectives
Positively correlated expected and true objectives
Zizzo argues that only the third case is truly problematic: demand effects in this case act as a confound, preventing the researcher from distinguishing the causal role of the treatment from that of the demand. This is, according to him, the case of the standard Dictator Game: the experimenter’s demand is correlated with the true purpose of the experiment, because subjects can easily guess that the experiment is about “giving.” Zizzo’s terminological effort is commendable, among other things, for trying to offer an account of the conditions under which experimenter demand effects affect the validity of experiments. But Zizzo’s specification remains unsatisfactory for the reasons discussed below.
A look at some standard practices in the more orthodox practices of experimental economics suffices in order to see why Zizzo’s diagnosis regarding the effects of experimental demand effects on validity lacks generality: monetary incentives (especially when or if they are dominant) are often used, precisely, to align the motivation of experimental subject with the (true) objectives of experimenters in a given game. In other words, they are used to signal to participants what the real objectives of a given experiment are. This is the case, for example, in those instances in which experimenters create an environment where income maximization is expected and demanded from participants. The coincidence between the true experimental objectives and those guessed by participants is in these cases, rather than a problem, a precondition for success in the experiment. This is a weakness in Zizzo’s diagnosis regarding the relation between reactivity and the validity of experiments.
The interventionist account that we introduce next avoids this problem by bypassing any reference to the “experiment’s true objectives “, a notion that can be vague and hard to operationalize. Yet, our account still provides a way to distinguish between situations in which reactivity is not problematic for experimental validity versus situations in which it potentially poses a threat. As we mentioned in the introduction, to properly discern between these two situations is important terminologically, as this is one of the ambiguities that hinders discussions on reactivity by producing misunderstandings: most of the terms that we use to refer to the general phenomenon of reacitivity (such as experimenter effects, demand effects, placebo effects, Hawthorne effects, or methodological artifacts) are often used without distinguishing between two different aspects of the phenomenon: these terms are used to refer both to the mechanisms that have the potential to bias an experiment and to the biases that can (or not) result from these mechanisms.
As we have already mentioned, some of the terms that are normally linked to reactivity-related phenomena have, in some contexts, some more specific meanings. This is the case, for example, for the term Hawthorne effects, which in some contexts can refer to the fact that experimental participants often feel motivated to display their best performance at a given task (and in this sense, better than they would under normal conditions), as a result of their being under study. Yet, in other contexts (as was the case in the DG debate), the term is also used in a different sense, to refer to the participants’ motivation to adapt their behavior to whatever they think the experimenter expects of them. While these two different types of attitudes can coincide in some contexts (e.g., whenever experimenters expect participants to perform at their “best” and subjects anticipate it), there are scenarios in which these two types of participant attitudes would lead to diverging behavioral responses. Footnote 5 For this reason, using the same term to refer to both phenomena can lead to confusion.
Here we defend an approach that unifies all reactivity-related phenomena under the same label, by focusing on the common aspects of the different mechanisms that can lead to reactivity. This does not preclude that further studies focus on more specific mechanisms, but rather, we contend that in an area where terminological ambiguity abounds, providing first a unifying framework is a useful first step.
3 Interventionism and social scientific experiments
In this section we characterize reactivity and the challenges that it poses to causal inference by using an interventionist or manipulationist account of causation (Woodward 2003 , Spirtes, Glymour and Scheines 2000,[1993]). For this, we will first describe the basic tenets of causal interventionism to then characterize a common type of behavioral experiment using an interventionist framework. We then analyze the possible meanings of reactivity through an interventionist lens.
An interventionist conception of causation conceives causal relationships as relationships that describe what will happen to some variables (effects, or dependent variables) when we manipulate or intervene on others (causes, or independent variables). For an interventionist to say that a relationship is causal is thus to say that it is exploitable for purposes of manipulation and control in a way that merely correlational relationships are not. The choice for this framework given our present problem (i.e., reactivity and how it affects causal inference from experimental data) seems natural for three reasons:
First, the interventionist notion of cause is often justified, precisely, as one that is especially fitting to the logic of the controlled experiment, which in turn is regarded as a method privileged in its capacity to allow for the testing of causal claims (pp. 22–23 Woodward 2003 ). In fact, interventionism can also be interpreted as a methodology to find out about causes, rather than as an approach committed to any particular ontology of causation (see Woodward 2015 ). Understood as a methodology, interventionism associates causal claims with the outcomes of hypothetical experiments in which the value of the variable representing the putative effect is set by means of intervening (only) on the putative cause.
Second, interventionism as conceived by Woodward has been especially concerned with the identification and clarification of ambiguous causal claims as they come up in (often social) scientific contexts, such as the assertion that "being female causes one to be discriminated against in hiring/salary" (p. 115, Woodward 2003 ). Woodward has tried to clarify such claims by linking them to potential or actual experimental manipulations. As we will show, the ambiguity in some of the assertions involving the phenomenon of reactivity comes, precisely, from a lack of clarity regarding what types of manipulations are attainable in different experimental settings involving humans.
Third, although Woodward has dealt with psychological and social science experiments that study social preferences (2007, 2008), the question of reactivity has not been systematically analysed under an interventionist framework: though Woodward has studied some well-known economic experiments such as the Ultimatum and the DG, his discussions have dealt with the robustness and external validity of their findings, but he has not, to date, specifically dealt with the phenomenon of reactivity and the question of how it can affect the causal claims we can validly infer from these games. The present paper thus contributes both to the literature on interventionism in social scientific experimentation and more broadly to the methodological and philosophical debates around experimental social science.
According to Woodward’s well-known manipulationist definition of cause:
(M) X causes Y iff (1) it is possible to intervene on X and (2) under some such possible intervention on X, changes in the value of X are associated with changes in the value of Y. Interventions must in turn fulfill the following conditions (see Fig. 1 ):
IN-i The intervention I completely disrupts the causal relationship between X and its previous causes. The value of X is set entirely by I.
IN-ii The intervention I should not itself be produced by any process that affects Y via a route that does not go through X.
IN-iii The intervention I leaves the values taken by any causes of X except those that are on the path from I to X to Y unchanged.
IN-iv The intervention I must not directly cause Y via a route that does not go through X.
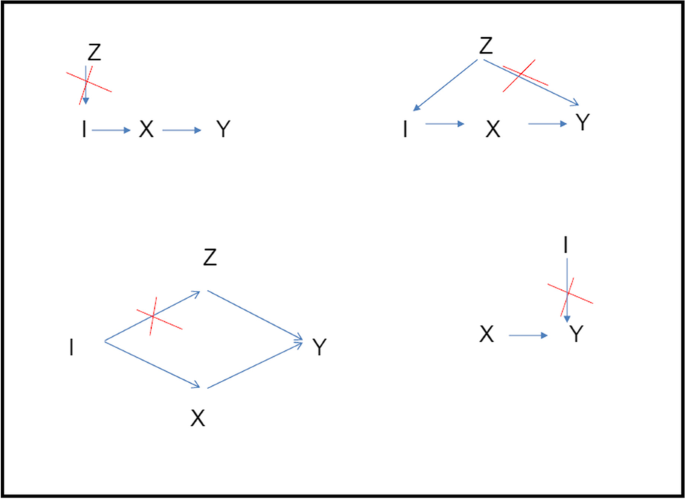
Conditions IN-i to IN-iv for an ideal intervention (left to right, top to bottom)
In more recent work Woodward ( 2007 ) has relaxed condition IN-i, which defines hard or arrow-breaking interventions in order to accommodate processes in which the value of X does not come entirely under the control of the intervention. This happens when there are other endogenous causal influences on X that cannot be broken by the intervention. In those cases. IN-i can be relaxed to IN-i’, where the intervention supplies an appropriately exogenous and uncorrelated source of variation to the variable X intervened on, rather than a complete disruption or breaking of all other causal influences on X. Thus, in soft interventions thus defined, the variation supplied by the intervention I should not be correlated with other causes of X or with causes of Y besides those that are on the route from I to X to Y.
The relaxation of this condition is crucial to accommodate experiments in many areas in which proper surgical interventions are not possible. In the case of the behavioral sciences, the impossibility is often determined by the fact that some form of mental causation is involved: as it has been argued by Campbell ( 2007 ), condition IN-i would entail that whenever we want to intervene on the mental state of an agent, we must ensure the removal of all the other causes of that agent’s mental state (thus suspending the rational autonomy of the individual).
Now that the main elements of an interventionist framework are laid out, we can use it to represent some economics experiments. In particular, we want to focus on the type of experiments that have sparked some of the recent discussions about reactivity in experimental economics. For this reason, we will use the DG as an example, as it is a well-known game with a very simple structure facilitating exposition, and has the additional advantage of having been extensively discussed by leading experimentalists in regard to reactivity-related issues.
By introducing modifications to the basic structure of the game, The DG design has been used to test different types of hypotheses. Here we focus on a well-established use of the DG design: the testing of subjects’ sensitivity to the manipulation of the normative framework applicable to the experimental situation (Guala and Mittone, 2010 ). Typically, in this kind of experimental exercise the basic DG is played as a control against a modified DG that constitutes the treatment, where the modification consists of the introduction of a normative-relevant cue. For example, in a well-known example, subjects in the treatment group play the DG in a room in which a picture of a pair of eyes is set, in order to bring to the subjects’ imagination the possibility of someone observing their actions (Haley and Fessler ( 2005 )). Other well-known modifications of the DG include introducing a modification in the identity of the Recipient (from an anonymous player to a well-known NGO, for example), or introducing an element of merit in deciding who, among two given players, gets to be the Dictator.
To be sure, both the standard DG (acting as a control or baseline) and the modified DG (acting as the treatment of interest) expose experimental subjects to an “unusual” normative setting, but the assumption is that by further modifying the normative environment, we can test whether an additional normative cue further affects the subject’s willingness to donate. The difference in the mean allocation between the two games is then interpreted as reflecting the impact of the introduction of the experimental manipulation in the modified DG: in terms of the causal hypothesis being tested, the difference in the mean allocation (from Dictators to Recipients) in the two experimental settings is seen as being caused by the introduction of the normative cue.
We can thus conceptualize this experiment, in more formal terms, as one based on a double intervention, where we must compare the results of each intervention to draw a conclusion about the causal impact of our putative cause on the putative effect (or the impact of the independent variable on the dependent variable). For this, we compare a control group playing the standard DG (X 0 ), with a treatment group exposed to the introduction of a DG that includes an additional normative cue (X 1 ). The causal impact of the normative change in the environment (X 1 -X 0 ) is thus measured by the difference in the mean allocation (Y 1 -Y 0 ) See Fig. 2 below. Footnote 6
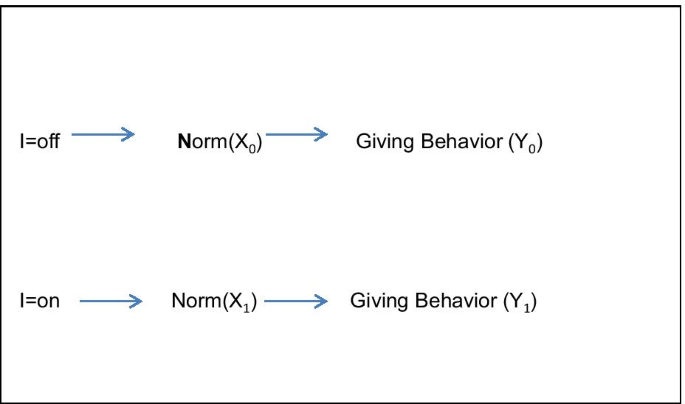
The Dictator Game from an Interventionist Perspective
We are now in a position to offer a suitable conceptualization of reactivity from an interventionist perspective. Recall that reactivity does not need to be restricted to experiments, as it is usually understood as the change in the subject’s behavior that results from his or her awareness of being studied, where this is also applicable to observational studies. In an observational environment, the change in behavior will come as a result of a subject´s awareness of being studied, or as a result of the operation of whatever measurement device is used. In the case of experimental studies there is an added layer of complexity, since by its own nature, the experiment provides the subject with a stimulus that is often supposed and expected to cause a behavioral change in participants (by exposing them to the treatment, or putative cause). Thus, when specifically applied to experiments, most definitions of reactivity-related phenomena can be seen as somewhat elliptical: reactivity is the change in the subject’s behavior as a direct result of her being studied, rather than as a result of the operation of our variable of interest, although the second part of the sentence is often not explicitly mentioned.
In terms of the categories deployed in an interventionist scheme, reactivity can thus be defined as a byproduct of an experimental intervention due to the subject’s awareness of taking part in that intervention. This byproduct takes place outside the causal path that goes from the independent variable or putative cause to the dependent variable or putative effect: we intervene on X (the putative cause) in order to assess its effect on Y (some aspect of the subject’s behavior), but by intervening experimentally, we also affect the subject’s behavior via some other route that does not go through X (i.e., the subject´s behavior gets altered because of his or her awareness of being under study).
Figures 3 and 4 represent cases of reactivity associated with experiments with settings akin to that of the DG: reactivity occurs when an intervention produces a change in the subject’s behavior through a route different from the one that goes from the putative cause to the putative effect (from I to X to Y).
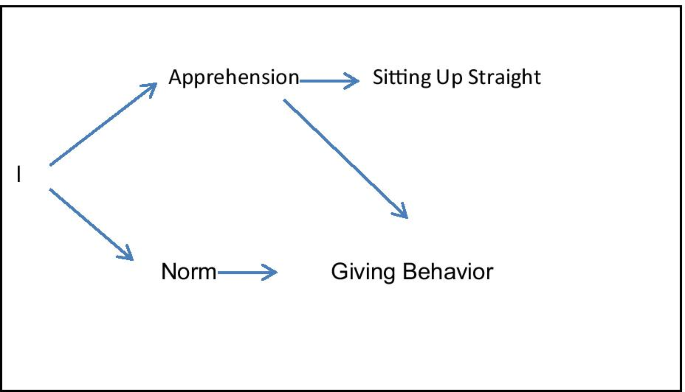
An example of Reactivity
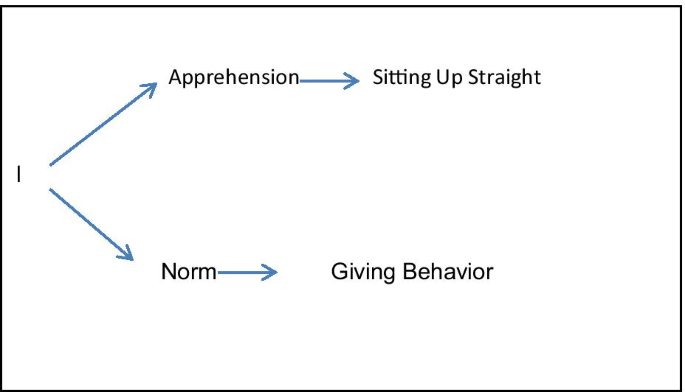
Another example of Reactivity
Let us illustrate this definition with our DG example, where an intervention introduces a normative cue in the environment in order to test for its causal effect on the subject’s “giving behavior”. Reactivity would occur if the intervention also results in inducing in the participant, for example, a sense of apprehension (such as a sensation of queasiness over feeling observed or studied upon) and if, in turn, the participant reacts to this apprehension by modifying his behavior (such as, for example, sitting up straight in his chair as a response to the feeling observed). Note that the apprehension is not attributable to the introduction of the normative cue per se, but to some other aspect imbued in the experimental setting, such as the fact of being under observation (see Figs. 3 and 4 above). It should be noted that apprehension to evaluation is only one of the many potential triggers of reactivity, where other common, well-known manifestations or mechanisms include the subjects’ reactions to the perceived authority of the experimenter, the participant’s zeal for being “a good subject” (or the opposite uncooperative desire to “boycott” an experiment), or the pervasive and understandable participants’ active search for cues and second guesses about what the experiment is really about (Jimenez-Buedo and Guala 2016 ).
By conceptualizing the phenomenon of reactivity in this way, we can better see what distinguishes reactivity in an experimental context from the more encompassing, general phenomenon of reactivity in observational research. Reactivity occurs when by studying subjects, we modify their behavior. However, in an experimental context there is always an intended intervention on the subjects’ environment, often purposefully directed at behavioral change. Reactivity is thus the uncontrolled, unintended effect on the subjects’ behavior that results as a byproduct of the intervention put in place to test for the causal effects of the experimental treatment. As we will see in the next section, our interventionist framework allows us, precisely, to discern when and why the intervention’s behavioral byproduct poses risks to our capacity to draw causal inferences from the experimental data.
4 Benign and malignant forms of reactivity
Now that we have defined reactive behavior within an interventionist framework, we can distinguish between two types of reactivity, depending on whether the type of reactive behavior violates or complies with the conditions for an ideal intervention.
Benign reactivity occurs when the intervention’s impact on the subject’s behavior does not affect the output variable of interest in the experiment. It is thus benign , in the sense that it does not pose in itself any problems to the causal inferential process as conceived by interventionism. Figure 4 shows an example of benign reactivity: intervening to set the value of the putative cause triggers an additional behavioral effect (sitting up differently than we normally would). This effect, however, operates outside of the causal path going from X to Y, and does not affect Y in any way.
By not violating any of the conditions of an ideal intervention, benign reactivity does not pose any particular challenges to causal inference. In our DG example, benign reactivity would mean that the apprehension that DG players can experience causes them to sit differently in their chairs (or makes them more prone to smiling, or causes their heart to beat faster) but to retain its benign character that same apprehension cannot affect the players’ “giving behavior”.
We can define malignant reactivity, in contrast, as occurring when the experimental manipulation not only changes the value of the putative effect Y by setting in motion the putative cause X, but additionally, it gives rise to an additional causal path that also affects the output variable of interest Y. This violates condition IN-iii above, so manipulations in which malignant reactivity occurs do not constitute ideal interventions in the Woodwardian sense.
Figure 3 represents graphically a case of malignant reactivity: the intervention sets in motion some reactive mechanism in Dictators (such as apprehension) and this apprehension affects, in turn, their willingness to donate to Recipients. In this case, the Dictator’s donating behavior is influenced both by the manipulation of the normative framework and by the participants’ apprehension toward the experimental evaluation of their behavior. Malignant reactivity thus constitutes an obstacle to causal inference through the violation of the IN-iii condition: if the level of donations we observe is suspected to be due not only to our introduction of a normative cue (the putative cause) but also influenced by some concomitant factor (in this case evaluation apprehension), then the effect that we observe on donations when we intervene on the normative cue cannot be attributed solely to it.
Note that the introduction of the distinction between malignant and benign forms of reactivity solves an extant ambiguity in the way that the relevant literature treats the relation between reactivity and experimental validity: the many terms that are employed to refer to reactivity-related phenomena are normally used to designate both the phenomenon itself and its potential for undermining the validity of experimental inferences. In this way, it is often the case that terms such as Hawthorne effects, are used ambiguously to refer both to the phenomenon by which a subject, for example, may be motivated to perform his or her best in an experimental context, and to refer to the experimental artifact that a particular reactive behavior may cause in a particular experiment. The problem with this ambiguity is that if it goes unnoticed it implicitly amounts to assuming that any reactivity-related phenomenon ipso facto invalidates any experimental inference that we wish to make. Yet, the two need not go together, as we might well be in situations in which, for example, we want, as experimenters, to motivate participants to perform at their best level, having no reason to think that their doing so poses a problem to the validity of our inferences from the experiment.
Because we also know that some form of reactivity or another is always present in any social scientific experiment, the implicit automatic connection between reactivity and artifact is likely to play no small role in the thinking of those that see social scientific experimentation as an enterprise doomed to fail. Yet, most social scientists and commentators tend to think, more plausibly, that reactivity does not irremediably lead to the invalidation of an experiment, yet the systematic discussion on the conditions under which it would are often absent.
In this regard, Zizzo’s more ambitious conceptual project is careful: in his framework, experimenter demand effects are not in themselves a problem but have the potential to create one whenever experimental subjects can correctly guess the objectives of the experiment, yet, as Jimenez-Buedo and Guala ( 2016 ) have argued, this approach neglects that often many economic experiments successfully align the incentives of subjects and experimenters through monetary rewards that are meant, precisely, to inform experimental subjects what exactly is sought of them, or in other words, what the objective of the experiment really is. Thus, and although Zizzo’s identification of this condition seems to fit the DG case nicely, it does not constitute the best grounds for a general elucidation of these conditions.
Our definition of reactivity and our distinction between benign and malignant forms of reactivity solves this problem: reactivity can but does not necessarily cause problems for causal inference. In its benign form, reactivity does not in itself pose difficulties in terms of the causal inferences that we can draw from experiments. In contrast, malignant reactivity constitutes an obstacle to the inference of causality from experimental data.
5 Is malignant reactivity lethal to causal inference? Placebo effects versus reactivity
The previous section ends on a somber note regarding the damage that malignant reactivity can do to experimental exercises aimed at inferring the causal impact of a given variable through controlled interventions. Yet, the reader may immediately consider the parallels between malignant forms of reactivity and what routinely occurs in Randomized Controlled Trials when placebo effects are present (i.e., when expectations about treatment have an effect on the recovery of patients). After all, the interventions that normally take place in RCTs often include, via placebo effects, a violation of condition IN-iii: the placebo effect created by exposure to any treatment (active or placebo) can improve our mood or expectations in ways that in turn impact our health. Yet, as we know, the introduction of control groups routinely solves whatever problems this may create for causal inferential purposes.
In fact, Woodward ( 2008 ) has discussed how an interventionist account can analytically deal with the presence of placebo effects in drug-testing RCTs. He has done so in the context of his response to Cartwright’s criticism of the interventionist’s assumption of modularity. Woodward argues that even though, as Cartwright rightly points out, placebo effects make surgical interventions impossible, interventionism can account for the strategies employed for inferring causality despite the impossibility (2008, p. 212)). In the presence of the placebo effects an intervenionist approach provides the rationale for the introduction of a control group that receives a placebo (a drug that resembles the treatment in all but its active ingredient). The aim of this placebo control group is to provide a base-line that allows us to measure the net causal effect of the drug we are testing by means of comparing the output result in the control trial and drug trials. The difference between the two trials is thus assumed to be an accurate representation of what would have happened if the drug had been administered in the absense of a placebo effect. In an interventionist account this subtraction or net effect represents or stands for the results of a counterfactual trial in which a surgical, ideal intervention would be possible. If the solution is readily available in the case of placebos, can we not use it to deal with the case of malignant reactivity in social scientific experiments?
In Fig. 5 we can see how the structure of the problem is formally similar in both the DG in the presence of reactivity and in an RCT with placebo effects. In both cases we see how malignant reactivity is present. However, there is a crucial difference between both situations: whereas in the case of RCTs the assumption of placebo effects that are equivalent across treatments seems generally valid (or at least valid for all those experiments in which the treatment can be administered in ways where blinding is effective, Footnote 7 such as in the intake of pills), it seems much harder to satisfy in the case of treatments involving some form of mental causation. Footnote 8
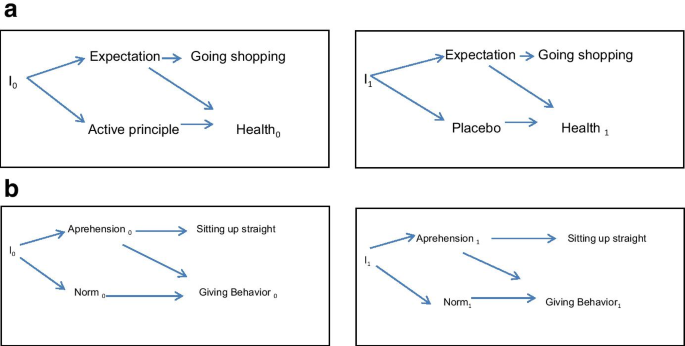
a RCTs and the placebo effect. b Reactivity in social scientific experiments
The reason is that in the case of social scientific experiments, a given treatment (or placebo) needs to be embedded in an experimental script, to which subjects then react. In some ways the experimental script carries the variable of interest like a pill may carry (or not) an active treatment: the variable of interest (say, a normative cue) is embedded in a given script like an active principle is embedded in a pill. Yet, this “carrying” also differs in important ways: in the case of experimental treatments involving mental causation, the script that “carries” a given treatment also embodies it, in a way in which the script and the treatment in which it is embedded become an inseparable bundle to which the subject reacts. For this reason, whatever reactive behavior occurs, it is likely to be the joint product of all the experiment’s elements in conjunction and this, in turn, implies that each script has the potential to give rise to its own unique, idiosyncratic reactivity: even if the treatment and control protocols differ in only one element (i.e., the presence or absence of our intended independent variable of interest), we cannot rule out that this differential element is enough to alter the participants’ perception of the whole experimental experience. This means that even the part of the script that remains the same across treatments can be perceived differently (as part of a different whole) by the experimental subjects.
When we add an active principle to a pill in the control group, the active principle alone can explain the difference between the responses in the treatment and control groups. In contrast, when we add (for example) an additional normative cue to an experimental script, the difference between the respones in the treatment and control group is the result of the interaction of the normative cue with the script. Put in other words, the inclusion of an element whose causal impact we want to test (e.g., a normative cue) has the potential to modify the effect of the same base script across the experimental groups, since the normative cue and the script that embeds it will be received inseparably by the experimental subjects. The same script used on its own (in the control group), and used in conjunction with the treatment (in the treatment group), might be received differently. This stands in contrast with the case of an RCT testing the efficacy of an active ingredient: once we assume that blinding across treatments is effective, we can safely assume that the excipient in the pill ha the same (placebo) effect across the treatment and the control groups.
In social scientific experiments, when a design tries to isolate the causal effect of a treatment embedded in a script, we must however at least conceive of the possibility (in cases where we suspect that there is malignant reactivity) that the differences in behavior across groups may be due not only to the treatment itself (understood here again as the variable of interest) but also, that this difference across treatments may be also due to the differences (across treatments) in the reactive behavior. This means that even if we introduce a minute change in the treatment group (minute with respect to the control group), we may also be modifying differentially across treatments, things like the participant´s eargerness to cooperate with what she thinks is the experiment’s objective, or her apprehension to the experimenter’s evaluation.
The reason for this lies in the holistic nature of meaning in social interactions: because any minute difference in a script has the potential to alter the meaning of a social interaction, a small difference in a script can transform the subjects’ interpretation of the experiment and thus can change the reactive behavior associated with it.
This has an important implication for social scientific experiments aiming at testing causal hypotheses through the comparison of control and treatment groups: if we cannot assume generally that these two interventions give rise to the same type of reactivity, then we cannot assume generally that a standard control group will suffice in order to correctly identify and isolate the causal impact of treatments net of reactivity. This will be the case even i ( as it is often the case), the control and the treatment differ in only one minute element, for that minute element has the potential to change the interpretation of the whole experiment and to induce different types of reactivity in both the control and the treatment groups. As we have shown, this aspect of social scientific experimentation can be well represented and conceptualized through an interventionist framework.
This paper thus clarifies the phenomenon of reactivity by subsuming it under this well-known framework. An interventionist framework allows us to provide a behavioral definition of the phenomenon of reactivity, subsuming its different mechanisms under a general scheme. It allows us, further, to distinguish between benign and malignant forms of reactivity, by differentiating between situations in which reactivity affects the variable of interest, from those in which the reactive behavior is orthogonal to the variable of interest.
In this section we have also seen how an interventionist framework can allow us to differentiate between situations in which malignant reactivity can be remedied with a control group (as it is routinely the case in RCTs dealing with placebo effects), from those situations in which malignant reactivity may be “resistant” to the standard procedure of contrasting the treatment and control groups. The latter can happen whenever reactivity may be idyosincratic, meaning that it is unique to the particular script enacted in each experiment. If reactivity is of this type, it cannot be subtracted away by comparing the treatment and the control group, even if the treatment and control differ in only one element. Summing up, an interventionist framework thus allows us to show that experimental reactivity can pose a threat to the inferential import of experiments. According to this framework this will happen in cases in which this reactivity is both malignant and idiosyncratic.
An interventionist framework thus provides a clear account of cases in which reactivity is present, but benign to the validity of an experiment, and it further provides a clear account of situations in which, in contrast, reactivity poses a threat to validity even if we have a (placebo) control group. Footnote 9 This contrasts with previous analysis of some aspects of the phenomena, and especially, with Zizzo’s account of experimenter demand effects, in which they are supposedly a threat to validity in cases in which experimental subjects can correctly identify the true objectives of the experiment.
Let us illustrate this analysis with our example contrasting the use of placebo in a properly blinded RCT with the case of a DG in which we assume malignant idiosyncratic reactivity (examples also depicted in Fig. 5 ):
If in an RCT set up to test the effectiveness of a new drug a given participant’s mood is improved merely by taking part in the study (i.e., if he or she is subject to a placebo effect), then we can safely assume that this improvement in mood will be equivalent across the treatment and control groups, in so far as blinding of the treatment is effective.
In contrast, consider the case of a standard DG used as a control and a modified DG used as the treatment of interest. If a participant is feeling apprehensive regarding the scrutiny of her behavior in a standard DG, this apprehension will be linked to her interpretation of the experiment’s meaning, which in turn will be determined jointly by her overall experience as a participant, i.e., by all the elements consisting of the experimental setting. In the standard DG subjects might feel queasiness regarding the fact that the standard DG is a “mysterious”, or an unusual game, where it is not totally clear what sort of behavior is expected of them. If we add an additional stimulus to the game in a modified DG (such as, for example, revealing the identity of the Recipient as being a charitable organization) we may, as experimenters, be using this stimulus as the carrier of a normative cue, the effects of which we want to test. However, the stimulus will also be the likely carrier of its own particular form of reactivity, one that has the potential to differ systematically from the type of reactivity associated with a standard DG. A modified DG can perhaps provide clearer signals to participants about the normative expectations at play, thus turning the environment into a more familiar one. At the same time, however, the range of phenomena linked to reactivity, (i.e., the behavioral response that is due to elements other than the intended treatment) is also likely to differ from that of a standard DG, and might, for example, have more to do with uncontrolled expectations regarding how to appear as a good subject.
In other words, to the extent that any two treatments involving social interactions are different (e.g., the baseline and the treatment of interest) we can expect (or at least consider the possibility) that their associated reactivity can be, in principle, unique and intrinsic to each treatment. The methodological consequence of this is clear, and applies as well to our DG example: the difference in the donation levels across treatments (the output variable of interest net of the baseline or control) can not thus be automatically assumed to be an accurate representation of what would have happened if the treatment of interest had been administered in the absense of reactivity.
To sum up, a variation in the script needed to modify a standard DG in order to carry a treatment (as, e.g., when introducing a normative cue in a modified DG) is likely to carry with itself a new bundle of reactive phenomena. If this reactivity is of the malignant sort, i.e., if it carries behavioral effects onto our output variable of interest, and, if we think it is idyosyncratic (i.e., if we think it depends on the particular script we are enacting), then we may not have any obvious means to know what would be the effect of our treatment variable, net of reactivity.
And yet, in the case of the DG, a significant difference in means between a standard DG (baseline) and a modified DG (treatment) is routinely presented in the relevant literature as proof of the effect on donations of whatever modification in the game. It should be noted that this implies that this difference in means can be interpreted as representing the effect of the introduction of the normative cue (the treatment) on donation levels, net of reactivity. However, as we have shown, this operation rests on endorsing at least one of the assumptions below:
There is no reactivity involved either in the standard DG or on its modified version.
Whatever reactivity there is, it is of the benign sort for both the standard DG and its modified version.
If there is malignant reactivity on the DG or its modified version, this malignant reactivity is behaviorally equivalent in its impact on the variable output of interest (the level of donations), i.e., it is not idysincratic to the treatment.
While any of the above assumptions can in principle be true for any given experiment, they cannot be assumed to hold generally across all social scientific settings, especially in cases in which we have reason to think that some forms of reactivity are likely, as in the case of the DG and related games. Our framework shows why it is necessary to justify or discuss each of these assumptions in every instance, and for each intervention, when presenting social scientific experimental results.
Note that the case in which reactivity is both malignant and idiosyncratic is the truly challenging one, for what we call here malignant reactivity can otherwise be routinely treated through the use of control groups, as it normally is. Our goal here is to provide an account of reactivity that can clarify why these situations can happen (and why they cannot be solved by the standard practice of having control groups). Our aim here is theoretical and conceptual rather than stritctly practical, meaning that we try to provide the definitions and distinctions that can be of help to further research aiming at systematically articulating what concrete experimental settings tend to bring about these problems. Though our aim is not here to provide a guide that identifies the concrete conditions under which reactivity can be either malignant or malignant and idosyncratic, we can hypothesize that there are a number of experimental situations where we can suspect that we are in this predicament. In particular, the DG can provide some cues regarding some of the scenarios that can make reactivity of the malignant idyosincratic kind more likely to emerge.
The DG provides an example of a setting where we have a game that, having very little structure, produces very different results depending on the introduction of different cues or variations in the context. Put differently, the interpretation of the DG’s “meaning” seems to depend on minute context variation. We can tentatively hypothesize that scenarios where results are very “sensitive” to slight changes in the experimental script might also be candidates for being scenarios where slight changes in the script can bring about strong changes in the part of the behavior that is properly “reactive”. In these cases, we might suspect that the reactive behavior might not be the same across the treatment and the control groups, provided that we think that the sensitivity of the design affects, not only the behavior in the treatment’s causal path, but also, the part of the behavior that is properly “reactive”.
As discussed in the introduction, the DG is a game in which, by construction, monetary incentives in the game do not dominate behavior (needless to say, if they did, the DG results would be incredibly boring, with zero donations across the board, irrespective of the particular designs). It seems to us that the DG exemplifies one of the obvious costs of abandoning dominance as a methodological precept: when economic incentives do not dominate the game, there is room for other considerations, including “reactive” ones, to affect the behavior of the participants in an experiment. But abandoning the principle of dominance is necessary if economists are interested in studying social behavior that relates to normative or ethical motivations, for the study of these through monetary incentives is done, precisely, by weighing monetary incentives against these other social (e.g., purely normative) considerations. In this sense, economists, once they have abandoned dominance as a guiding precept, have had to deal with reactivity as much as their experimental colleagues in other social sciences.
The framework developed here thus seems to provide a promising route to finding out what makes results like those of the DG and similar games, particulary debatable. We restrict our analysis to the conceptual and theoretical clarification of the phenomenon through an interventionist framework, rather than devote this piece to the particular methodological analysis of a given design. We contend, however, that our conceptual contribution can be valuable to future applied research.
6 Conclusions
In social scientific experiments, the putative causes tested by the interventions come embedded in experimental scripts, rather than in pills, and thus operate through mental causation and social meaning, where this meaning is interpreted holistically. Experimental scripts embodying the treatment often give rise to some type of reactivity, whereby subjects modify their behavior as a result of some characteristics of the intervention, other than those related to the variable of interest. Whether this reactivity is suspected to be benign (if it does not have an effect on the relevant dependent variable) or malignant (if it does) will depend on the way those particular experimental scripts are processed and conceived by subjects. Moreover, this reactivity can, sometimes, be unique to each intervention, and thus, inseparable from each experimental script when the difference in outcomes between the control and the treatment group cannot guarantee that results are net of reactivity related input.
When we contrast the output of the treatment intervention with the control intervention (as in a modified DG versus a standard DG) in order to draw causal conclusions, we are implicitly assuming that the reactivity generated by each experimental script is benign or that, if it is malignant, it is equivalent across treatments (i.e., not idiosyncratic). While any of these assumptions may be true for any given intervention, they may not always hold in all cases. By stressing the need to specify the conditions under which these assumptions can hold, our analysis aims to contribute to the debate over the limits of social scientific experimentation and specifically, about the validity of causal inferences generated by social experiments like the DG.
Ultimately, our intuitions about reactivity hinge upon, but also affect one’s methodological position in the debate regarding the powers and the limits of social scientific experimentation. Indeed, while reactivity is traditionally considered by some a problem that can be either prevented by the use of control groups, or accounted for in the interpretation of results, it has represented for others a definitive obstacle to the mere possibility of investigating the social world experimentally (Harré and Secord 1972 ). A tension has traditionally existed between two seemingly irreconcilable views on the relationship between experimentation and the issue of reactivity: the experiment seen as the best environment to create the type of control that is needed to separate behavior into some of its relevant causal components, and. the view that experimentation is severely hindered by the fact that all social reality, including the experimental site is a thick, layered environment charged with social meaning, where that social meaning can only be interpreted holistically. Here we try to show that although reactivity is very likely a constitutive part of social experimentation, it is often benign. When it is not, it is often solvable through the standard practice of including a control group. Yet, we have also shown that when reactivity is not benign and is idiosyncratic, then it does pose problems to the inferential import of experiments. We have offered a conceptual framework to understand reactivity and argue that elucidating this concept provides a useful groundwork upon which we can build more nuanced, methodologically driven, case by case analyses.
In the DG, the experimenter allocates some fixed quantity of money with player 1, the Dictator, who then has to decide how much, if any, he or she wants to share with player 2, the Respondent. The results of the standard DG show that roughly half of the Dictators depart from the earnings maximizing strategy and choose to give some money, the mean allocation being 20% of the initial endowment. Moreover, a consistent minority of dictators choose to split the sum in two similar sizes (Camerer 2003 ).
It is perhaps opportune to underline once more that the framework for reactivity we provide, in which it is used as an umbrella term, does not intend to distinguish among different mechanisms of reactivity. It instead unifies the phenomenon in order to explore the problems that it can create to causal identification and experimental validity.
Vernon Smith’s precepts were the following: the proscription of deception, the principle of parallelism, or the idea of “similarity” between the lab setting and the target phenomena, and finally, a series of requirements regarding the structure of the incentives faced by subjects. These included: (i)nonsatiation (where the medium of payment should not “satiate” participants, in the way, more money does typically not satiate); (ii) saliency (where the reward must increase or decrease according to the way in which an outcome is consider good or bad, or correct or incorrect); (iii) dominance (where the rewards must dominate any subjective costs associated with participation in the experiment), and (iv)privacy (in that each subject in an experiment receives info only about her own payoffs).
The origin of the term comes from the Hawthorne Works, in Illinois, a factory in which, in the context of a series of studies on productivity, a group of assembly employees seemed to paradoxically increase their productivity as researchers dimmed the lights. This puzzling result was interpreted as the result of the perception of workers of being under study (Adair 1984 ).
Note, as an example of how the two phenomena may differ in a concrete example: In the original Hawthorne Works study, employees responded by overperforming as lights became dimmer, though it is unlikely that they would have thought that experimenters expected them to do so.
The representacional convention (I = on and off) is borrowed from Eberhardt and Scheines ( 2007 ).
For an analysis on the relevance of blinding see Teira and Reiss ( 2013 ) Teira ( 2019 ).
To be sure, placebo effects also , and rather obviously, involve mental causation, but on this point we are contrasting the treatments that are being administered, not the secondary effects (both of which -placebo and reactivity alike- involve mental causation). In the case of the social sciences treatments, they will almost always involve some form of mental causation, in contrast with the case of the medical placebo if the treatment is administered through the intake of a pill.
It should be noted that malignant idiosyncratic reactivity threatens not only external validity, but also, internal validity. Regarding external validity, the existence of reactive effects that can not be substracted away via a control group, undoubtedly poses problems to the extrapolation of results from the lab to non experimental conditions. The problem, however, is also one of external validity: proper causal identification through isolation is not possible in the presence of malignant idiosyncratic reactivity, and thus, internal validity cannot be attained. I thank reviewers for pressing me on this point.
Adair, J. G. (1984). The Hawthorne effect: a reconsideration of the methodological artifact. Journal of Applied Psychology, 69, 334–345.
Bardsley, N. (2008). Dictator game giving: altruism or artefact? Experimental Economics, 11 (2), 122–133.
Article Google Scholar
Camerer, C. F. (2003). Behavioral game theory: Experiments in strategic interaction . Princeton: Princeton University Press.
Google Scholar
Campbell, J. (2007). An interventionist approach to causation in psychology. Causal learning: Psychology, philosophy, and computation , 58-66.
Dana, J., Weber, R. A., & Kuang, J. X. (2007). Exploiting moral wiggle room: experiments demonstrating an illusory preference for fairness. Economic Theory, 33 (1), 67–80.
Eberhardt, F., & Scheines, R. (2007). Interventions and causal inference. Philosophy of Science, 74 (5), 981–995.
Guala, F., & Mittone, L. (2010). Paradigmatic experiments: the dictator game. The Journal of Socio-Economics, 39 (5), 578–584.
Haley, K. J., & Fessler, D. M. (2005). Nobody’s watching?: Subtle cues affect generosity in an anonymous economic game. Evolution and Human behavior, 26 (3), 245–256.
Harré, R., & Secord, P. F. (1972). The explanation of social behaviour.
Hertwig, R., & Ortmann, A. (2001). Experimental practices in economics: A methodological challenge for psychologists? Behavioral and Brain Sciences, 24 (3), 383–403.
Jimenez-Buedo, M. (2015). The last dictator game? Dominance, reactivity, and the methodological artefact in experimental economics. International Studies in the Philosophy of Science, 29 (3), 295–310.
Jimenez-Buedo, M., & Guala, F. (2016). Artificiality, reactivity, and demand effects in experimental economics. Philosophy of the Social Sciences, 46 (1), 3–23.
Levitt, S. D., & List, J. A. (2007). What do laboratory experiments measuring social preferences reveal about the real world? Journal of Economic Perspectives, 21 (2), 153–174.
List, J. A. (2007). On the interpretation of giving in dictator games. Journal of Political Economy, 115 , 482–492.
Orne, M. T. (1962). On the social psychology of the psychological experiment: with particular reference to demand characteristics and their Implications. American Psychologist, 17, 776–783.
Orne, M. T. (1969). Demand characteristics and the concept of quasi-controls. In R. Rosenthal & R. Rosnow (Eds.), Artifact in Behavioral Research (pp. 143–179). New York: Academic Press.
Rosenthal, R. (1964). Experimenter outcome-orientation and the results of the psychological experiment. Psychological Bulletin, 61, 405.
Rosenthal, R. (1968). On the social psychology of the psychological experiment: 1, 2 the experimenter's hypothesis as unintended determinant of experimental results. American Scientist, 51 (2), 268–283.
Spirtes, P., Glymour, C. N., Scheines, R., & Heckerman, D. (2000). Causation, prediction, and search. MIT press.
Teira, D., & Reiss, J. (2013). Blinding and the non-interference assumption in field experiments. Philosophy of the Social Sciences, 43 (3), 358–372.
Teira, D. (2019). Placebo trials without mechanisms: How far can we go? Studies in History and Philosophy of Science Part C: Studies in History and Philosophy of Biological and Biomedical Sciences, 77, 101177.
Woodward, J. (2003). Making things happen: A causal theory of explanation . Oxford: Oxford University Press.
Woodward, J. (2007). Causation with a human Face. In Price and Corry (Eds.), Causation and the constitution of reality . Oxford University Press: 66–105
Woodward, J. (2008). Invariance, modularity, and all that. In S. Hartman, C. Hoefer, & L. Bovens (Eds.), Nancy cartwright’s philosophy of science (pp. 198–237). Taylor & Francis.
Woodward, J. (2015). Methodology, ontology, and interventionism. Synthese, 192 (11), 3577–3599.
Zizzo, D. J. (2010). Experimenter demand effects in economic experiments. Experimental Economics, 13 (1), 75–98.
Download references
Acknowledgements
This paper was first presented at the Departamental Seminar in Uned, May 2016, the PSA 2016 Inem session, in Atlanta, and several other venues thereafter (including a Workshop on Reactivity organized at Bergen University in March 2020). I would like to thank the participants of these sessions for input and encouragement, as well as the reviewers and editors of EJPS.
MECABIOSOC. FFI2017-89639-P. Ministerio de Ciencia, Innovación y Universidades.
Author information
Authors and affiliations.
Dpto. de Lógica, Historia y Filosofía de la Ciencia, UNED, UNED, Paseo de senda del rey 7, 28040, Madrid, Spain
María Jiménez-Buedo
You can also search for this author in PubMed Google Scholar
Corresponding author
Correspondence to María Jiménez-Buedo .
Ethics declarations
Ethical approval.
non applicable.
Informed consent
Conflict of interest, additional information, publisher’s note.
Springer Nature remains neutral with regard to jurisdictional claims in published maps and institutional affiliations.
Rights and permissions
Open Access This article is licensed under a Creative Commons Attribution 4.0 International License, which permits use, sharing, adaptation, distribution and reproduction in any medium or format, as long as you give appropriate credit to the original author(s) and the source, provide a link to the Creative Commons licence, and indicate if changes were made. The images or other third party material in this article are included in the article's Creative Commons licence, unless indicated otherwise in a credit line to the material. If material is not included in the article's Creative Commons licence and your intended use is not permitted by statutory regulation or exceeds the permitted use, you will need to obtain permission directly from the copyright holder. To view a copy of this licence, visit http://creativecommons.org/licenses/by/4.0/ .
Reprints and permissions
About this article
Jiménez-Buedo, M. Reactivity in social scientific experiments: what is it and how is it different (and worse) than a Placebo effect?. Euro Jnl Phil Sci 11 , 42 (2021). https://doi.org/10.1007/s13194-021-00350-z
Download citation
Received : 08 May 2019
Accepted : 25 January 2021
Published : 20 April 2021
DOI : https://doi.org/10.1007/s13194-021-00350-z
Share this article
Anyone you share the following link with will be able to read this content:
Sorry, a shareable link is not currently available for this article.
Provided by the Springer Nature SharedIt content-sharing initiative
- Dictator game
- Experimental economics
- Experimenter demand effects
- Social science experiments
- Hawthorne effect
- Interventionism
- Placebo effect
Advertisement
- Find a journal
- Publish with us
- Track your research
- Institutional Login
Access to the full content is only available to members of institutions that have purchased access. If you belong to such an institution, please log in or find out more about how to order .
Loading content
We were unable to load the content
Article Summary
- content locked 1 The logic of the experiment
- content locked 2 Variable analysis
- content locked 3 Problems with the experimental model
- content locked Bibliography
Experiments in social science
- Hughes, John A.
Within social science the experiment has an ambiguous place. With the possible exception of social psychology, there are few examples of strictly experimental studies. The classic study still often cited is the Hawthorne experiments, which began in 1927, and is used mainly to illustrate what became known as the ‘Hawthorne Effect’, that is, the unintended influence of the research itself on the results of the study. Yet, experimental design is often taken within social research as the embodiment of the scientific method which, if the social sciences are to reach the maturity of the natural sciences, social research should seek to emulate. Meeting this challenge meant trying to devise ways of applying the logic of the experiment to ‘non-experimental’ situations where it was not possible directly to manipulate the experimental conditions. Criticisms have come from two main sources: first, from researchers who claim that the techniques used to control factors within non-experimental situations are unrealizable with current statistical methods and, second, those who reject the very idea of hypothesis-testing as an ambition for social research.
Related Searches
- Philosophy of social science
Related Articles
- Experiment By Morrison, Margaret C.
- Statistics and social science By Spirtes, Peter
What Is an Experiment? Definition and Design
The Basics of an Experiment
- Chemical Laws
- Periodic Table
- Projects & Experiments
- Scientific Method
- Biochemistry
- Physical Chemistry
- Medical Chemistry
- Chemistry In Everyday Life
- Famous Chemists
- Activities for Kids
- Abbreviations & Acronyms
- Weather & Climate
- Ph.D., Biomedical Sciences, University of Tennessee at Knoxville
- B.A., Physics and Mathematics, Hastings College
Science is concerned with experiments and experimentation, but do you know what exactly an experiment is? Here's a look at what an experiment is... and isn't!
Key Takeaways: Experiments
- An experiment is a procedure designed to test a hypothesis as part of the scientific method.
- The two key variables in any experiment are the independent and dependent variables. The independent variable is controlled or changed to test its effects on the dependent variable.
- Three key types of experiments are controlled experiments, field experiments, and natural experiments.
What Is an Experiment? The Short Answer
In its simplest form, an experiment is simply the test of a hypothesis . A hypothesis, in turn, is a proposed relationship or explanation of phenomena.
Experiment Basics
The experiment is the foundation of the scientific method , which is a systematic means of exploring the world around you. Although some experiments take place in laboratories, you could perform an experiment anywhere, at any time.
Take a look at the steps of the scientific method:
- Make observations.
- Formulate a hypothesis.
- Design and conduct an experiment to test the hypothesis.
- Evaluate the results of the experiment.
- Accept or reject the hypothesis.
- If necessary, make and test a new hypothesis.
Types of Experiments
- Natural Experiments : A natural experiment also is called a quasi-experiment. A natural experiment involves making a prediction or forming a hypothesis and then gathering data by observing a system. The variables are not controlled in a natural experiment.
- Controlled Experiments : Lab experiments are controlled experiments , although you can perform a controlled experiment outside of a lab setting! In a controlled experiment, you compare an experimental group with a control group. Ideally, these two groups are identical except for one variable , the independent variable .
- Field Experiments : A field experiment may be either a natural experiment or a controlled experiment. It takes place in a real-world setting, rather than under lab conditions. For example, an experiment involving an animal in its natural habitat would be a field experiment.
Variables in an Experiment
Simply put, a variable is anything you can change or control in an experiment. Common examples of variables include temperature, duration of the experiment, composition of a material, amount of light, etc. There are three kinds of variables in an experiment: controlled variables, independent variables and dependent variables .
Controlled variables , sometimes called constant variables are variables that are kept constant or unchanging. For example, if you are doing an experiment measuring the fizz released from different types of soda, you might control the size of the container so that all brands of soda would be in 12-oz cans. If you are performing an experiment on the effect of spraying plants with different chemicals, you would try to maintain the same pressure and maybe the same volume when spraying your plants.
The independent variable is the one factor that you are changing. It is one factor because usually in an experiment you try to change one thing at a time. This makes measurements and interpretation of the data much easier. If you are trying to determine whether heating water allows you to dissolve more sugar in the water then your independent variable is the temperature of the water. This is the variable you are purposely controlling.
The dependent variable is the variable you observe, to see whether it is affected by your independent variable. In the example where you are heating water to see if this affects the amount of sugar you can dissolve , the mass or volume of sugar (whichever you choose to measure) would be your dependent variable.
Examples of Things That Are Not Experiments
- Making a model volcano.
- Making a poster.
- Changing a lot of factors at once, so you can't truly test the effect of the dependent variable.
- Trying something, just to see what happens. On the other hand, making observations or trying something, after making a prediction about what you expect will happen, is a type of experiment.
- Bailey, R.A. (2008). Design of Comparative Experiments . Cambridge: Cambridge University Press. ISBN 9780521683579.
- Beveridge, William I. B., The Art of Scientific Investigation . Heinemann, Melbourne, Australia, 1950.
- di Francia, G. Toraldo (1981). The Investigation of the Physical World . Cambridge University Press. ISBN 0-521-29925-X.
- Hinkelmann, Klaus and Kempthorne, Oscar (2008). Design and Analysis of Experiments, Volume I: Introduction to Experimental Design (Second ed.). Wiley. ISBN 978-0-471-72756-9.
- Shadish, William R.; Cook, Thomas D.; Campbell, Donald T. (2002). Experimental and quasi-experimental designs for generalized causal inference (Nachdr. ed.). Boston: Houghton Mifflin. ISBN 0-395-61556-9.
- 10 Things You Need To Know About Chemistry
- Chemistry 101 - Introduction & Index of Topics
- How to Clean Lab Glassware
- How To Design a Science Fair Experiment
- Understanding Experimental Groups
- What Is a Control Group?
- Examples of Independent and Dependent Variables
- How to Write a Lab Report
- The Difference Between Control Group and Experimental Group
- Scientific Method Lesson Plan
- Pre-Lab Prep for Chemistry Lab
- Difference Between Independent and Dependent Variables
- Which Is Faster: Melting Ice in Water or Air?
- What Is the Difference Between Hard and Soft Science?
- 5 Top Reasons Why Students Fail Chemistry
- What Is a Dependent Variable?

1 What are the social sciences?
Learning Objectives for this Chapter
After reading this Chapter, you should be able to:
- understand what the social sciences are, including some fundamental concepts and values,
- understand and apply the concept of ‘phronesis’ to thinking about the purpose and value of the social sciences.
History and philosophy of the social sciences
Some of the earliest written and spoken accounts of human action, values, and the structure of society can be found in Ancient Greek, Islamic, Chinese and indigenous cultures. For example, Ibn Khaldoun , a 14th-century North African philosopher, is considered a pioneer in the field of social sciences. He wrote the book Muqaddimah , which is regarded as the first comprehensive work in the social sciences. It charts an attempt to create a universal history based on studying and explaining the economic, social, and political factors that shape society and discussed the cyclical rise and fall of civilisations. Moreover, indigenous peoples across the world have contributed in various and significant ways to the development of scientific knowledge and practices (e.g., see this recent article by Indigenous scholar, Jesse Popp – How Indigenous knowledge advances modern science and technology ). Indeed, contemporary social science has much to learn from indigenous knowledges and methodologies (e.g., Quinn 2022 ), as well as much reconciling to do in terms of its treatment of indigenous peoples the world over (see Coburn, Moreton-Robinson, Sefa Dei, and Stewart-Harawira, 2013 ).
Nevertheless, the dominant Western European narrative of the achievements of the enlightenment still tends to overlook and discredit much of this knowledge. Additionally, male thinkers have tended to dominate within the Western social sciences, while women have historically been excluded from academic institutions and their perspectives largely omitted from social science history and texts. Therefore, much of the history of the social sciences represent a predominantly white, masculine viewpoint. That is not to say that the concepts and theories developed by these male social scientists should be outright discredited. Nevertheless, in engaging with them we must understand this context; they are not the only voices, nor necessarily the most important. Indeed, it is crucial therefore that the history of the social sciences is continually re-examined through a critical lens, to identify gaps within social scientific knowledge bases and allow space for critical revisions that broaden existing concepts and theories beyond an exclusively masculine, Western-centric perspective. We seek to adopt such an approach throughout this book. However, to critique and question Western social scientific perspectives, we must first understand them.
Social sciences in the Western world
The study of the social sciences, as developed in the Western world, can be said to emerge from the Age of Enlightenment in the late 17th Century. Beginning with René Descartes (1596-1650), both the natural and social sciences developed from the concept of the rational, thinking individual. These early Enlightenment thinkers argued that human beings use reason to understand the world, rather than only referring to religion. Other thinkers around this time such as Jean-Jacques Rousseau (1712-1778), M. de Voltaire (1694-1778) and Denis Diderot (1713-1784), began to develop different methodologies to scientifically explain processes in the body, the structure of society, and the limits of human knowledge. It was during this period that the social sciences grew out of moral philosophy, which asks ‘how people ought to live’, and political philosophy, which asks ‘what form societies ought to take’. Rather than only focusing on descriptive scientific questions about ‘how things are’, the social sciences also sought answers to normative questions about ‘how things could be’. This is one of the central differences between the natural sciences and the social sciences. This era of Enlightenment marked an important turning point in history that gave way to further developments in both the natural and social sciences.
Immanuel Kant (1724-1804) is often regarded as one of the most influential philosophers for the development of the social sciences. In his work, Kant develops an epistemology that accounts for the objective validity of knowledge, due to the capacities of the human mind. In other words, how can we as individual people come to know facts about the world that are true for all of us. Social scientists, such as Émile Durkheim (1858-1917) and Max Weber (1864-1920) critically developed the work of Kant to explain social relations between individuals.
Émile Durkheim prioritised the validity of social facts over the values themselves, continuing the tradition of ‘ positivism ‘ (an ontological position that we discuss later in this Chapter). Durkheim argued that there is a distinction between social facts and individual facts. Rather than viewing the structure of the human mind as the basis for knowledge like Kant, Durkheim argued that it is society itself that forms the basis for the social experience of individuals. Social facts should therefore, “be treated as natural objects and can be classified, compared and explained according to the logic of any natural science” (Rose, 1981: 19). Durkheim developed his methodology using analogies to the natural sciences. For example, he borrowed concepts from biology to understand society as a living organism.
TRIGGER WARNING
The following section contains content which may be triggering for certain people. It focuses on the sociology of suicide, including discussion of self-harm and different forms of suicide as it exists within society.
Durkheim and Suicide
Emile Durkheim’s 1879 text ‘Suicide: a Study in Sociology’ is a foundational work for the study of social facts. Durkheim explores the phenomenon of suicide across different time periods, nationalities, religions, genders, and economic groups. Durkheim argues that the problem of suicide can not be explained through purely biological, psychological or environmental means. Suicide must, he concludes, “necessarily depend upon social causes and be in itself a collective phenomenon” (Durkheim 1897: 97). It was and continues to be a work of great impact that demonstrates that, what most would consider an individual act is actually enmeshed in social factors.
In his text, Durkheim identifies some of the different forms suicide can take within society, four of which we discuss below.
Egoistic Suicide
Egoistic suicide is caused by what Durkheim terms “excessive individuation” (Durkheim 1897: 175). A lack of integration within a particular community or society at large leads human beings to feel isolated and disconnected from others. Durkheim argues that “suicide increases with knowledge”(Durkheim 1897: 123). This is not to say that a particular human being kills themselves because of their knowledge; rather it is because of the decline of organised religion that human beings desire knowledge outside of religion. It is thus, for Durkheim the weakening organisation of religion that detaches people from their (religious) community, increasing social isolation. According to Durkheim, the capacity of religion to prevent suicide does not result from a stricter prohibition of self-harm. Religion has the power to prevent someone from committing suicide because it is a community, or a ‘society’ in Durkheim’s words. The collective values of religion increases social integration and is just one example of the importance of community in decreasing rates of suicide. Isolation of individuals, for Durkheim, is a fundamental cause of suicide: “The bond attaching man [sic] to life relaxes because that attaching him [sic] to society is itself slack” (Durkheim 1897: 173).
Altruistic Suicide
Durkheim notes another kind of suicide that stems from “insufficient individuation” (Durkheim 1897: 173). This occurs in social situations where an individual identifies so strongly with their beliefs of a group that they are willing to sacrifice themselves for what they perceive to be the greater good. Examples of altruistic suicide include suicidal sacrifice in certain cultures to honour their particular God, soldiers who go to war and die in honour of their country, or the ancient tradition of Hara-kiri in Japan. As such, Durkheim notes that some people have even refused to consider altruistic suicide a form of self-destruction, because it resembles “some categories of action which we are used to honouring with our respect and even admiration”(Durkheim 1897: 199).
Anomic Suicide
The third kind of suicide Durkheim identifies is termed anomic suicide. This type is the result of the activity of human beings “lacking regulation”, and “the consequent sufferings” that are felt from this situation (Durkheim 1897: 219). Durkheim notes the similarities between egoistic and anomic suicide, however he notes an important distinction: “In egoistic suicide it is deficient in truly collective activity, thus depriving the latter of object and meaning. In anomic suicide, society’s influence is lacking in the basically individual passions, thus leaving them without a check-rein” (Durkheim 1897: 219).
Fatalistic Suicide
There is a fourth type of suicide for Durkheim, one that has more historical meaning than current relevance. Fatalistic suicide is opposed to anomic, and is the result of “excessive regulation, that of persons with futures pitilessly blocked and passions violently choked by oppressive discipline” (Durkheim 1897: 239). These regulations occur during moments of crises, including economic and social upheaval, that destabilise the individual’s sense of meaning. It is the impact of external factors onto the individual, where meaning is thrown to the wind for the individual, that characterises fatalistic suicide.
Durkheim’s sociological study of suicide was a groundbreaking work for social sciences. His methodology, multivariate analysis, provided a way to understand numerous interrelated factors and how they relate to a particular social fact. His findings, particularly the higher suicide rates of Protestants, compared to Jewish and Catholic people, was correlated to the higher rates to individualised consciousness and the lower social control. This study, despite criticisms of the generalisations drawn from the results, has had a remarkable impact on sociology and remains a seminal text for those interested in the social sciences.
Max Weber was also influenced by the work of Kant. Unlike Durkheim, Weber “transformed the paradigm of validity and values into a sociology by giving values priority over validity” (Rose, 1981: 19). Culture is thus understood as a value that structures our understanding of the world. According to Weber, values cannot be spoken about in terms of their truth content. The separation between values and validity means that values can only be discussed in terms of faith rather than scientific reason. For Weber, only when a culture’s underpinning values are defined can facts about the social world be understood.
The philosophy of G.W.F. Hegel (1770-1831) also greatly shaped the development of the social sciences. As argued by Herbert Marcuse (1941: 251-257), Hegel instigated the shift from abstract philosophy to theories of society. According to Hegel, human beings are not restricted to the pre-existing social order and can understand and change the social world. Our natural ability to reason allows human beings to create theories about our world that are universal and true.
Karl Marx (1818-1883), often regarded as the founder of conflict theory, was deeply influenced by the philosophy of Hegel. For example, Hegel emphasises that labour and alienation are essential characteristics of human experience, and Marx applies this idea more concretely to a material analysis of society, dividing human history along the lines of the forces of production. In other words, Marx understood that labour was divided in capitalist society according to two classes that developed society through a perpetual state of conflict: the working class, or ‘ proletariat’ , and the class of ownership, or ‘ bourgeoisie’ (we talk more about Marx’s conflict theory in Chapter 3).
Overall, the social sciences have a long and complex history, influenced by many different philosophical perspectives. As alluded to earlier, however, any account of the historical beginnings of the social sciences must be understood to be embedded within dominant systems of power, including for example colonisation, patriarchy, and capitalism. Indeed, any history of the social sciences is already situated within a narrative, or ‘discourse’. Maintaining a critical lens will allow for a deeper understanding of the genesis of the social sciences, as well as the important ability to question social scientific approaches, understandings, findings, and methods. It is this disposition that we seek to cultivate throughout this book. After all, as Marx famously wrote, “The philosophers have only interpreted the world, in various ways. The point, however, is to change it.”
Defining Key Terms
Descriptive : A descriptive claim or question seeks to explain how things work, what causes them to work that way, and how things relate to one another.
Normative : A normative claim or question seeks to explain how things ought to work, why they should work a certain way, and what should change for things to work differently.
Labour : For Marx, labour is the natural capacity of human beings to work and create things. Under capitalism, labour primarily produces profits for the ruling class. (Please note, we return to the notion of labour in later chapters, and explore other understandings and definitions of this term.)
Alienation : Workers, separated from the products of their labour and replaceable in the production process, become separated or ‘alienated’ from their creative human essence. (Please also see Chapter 3 for a further explanation of the concept of alienation under Marxism.)
What are the social sciences?
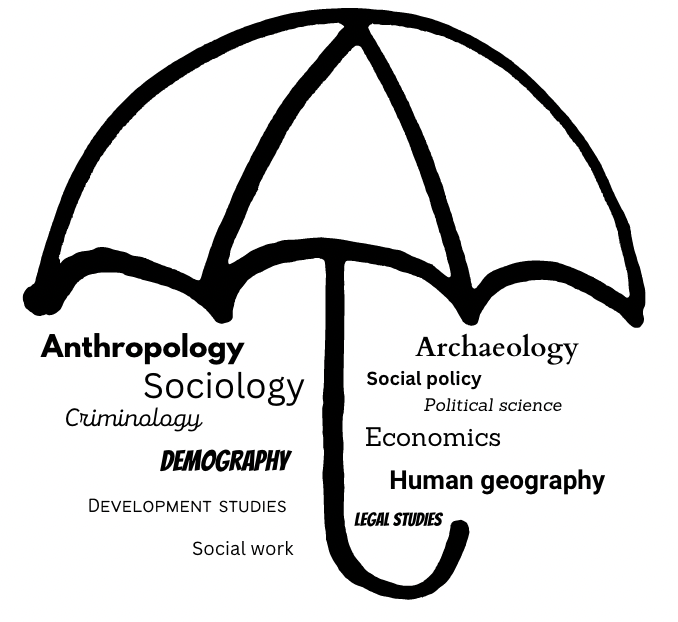
The social sciences are a ‘broad church’, including lots of different disciplinary and sub-disciplinary areas. These include, for example, sociology, anthropology, criminology, archaeology, social policy, human geography, and many more. At their core, they apply the ‘scientific method’ to the analysis of people, societies, power, and social change.
Before we move on, let’s touch briefly on what we mean by the scientific method . At its core, the scientific method is essentially a series of steps that scientists take in order to build and test scientific knowledge. These steps include:
- Observation : Scientists observe the world around them, in order to better understand it.
- Question : Scientists ask ‘research questions’ about how the world works.
- Hypothesis: Scientists come up with ideas or theories about how they think the world works, which they then seek to test through their research.
- Experiment: In experimental research, scientists use a specific experimental design (which includes a control and experimental group) to test hypotheses. This is not always possible or desirable in the social sciences, so social scientists tend to rely on a broader array of methods to collect data that can help them test their hypotheses about the social world.
- Analysis: Scientists use various different approaches to analyse the data they collect; the approach to analysis depends on the kind of data collected, and what questions are being asked of the data.
- Conclusions: Scientists develop conclusions, based on the results of their analyses. They consider how these either reinforce or further develop existing knowledge and understandings, as well as what there is left to find out (the latter of which informs future research endeavours).
Over time, social scientists have developed their own ontological and epistemological leanings, which in many ways represent a departure from the typical positivist approaches of the natural sciences. While the natural sciences tend to assume there are objective ‘truths’ waiting to be discovered through, for instance, sensory experience (seeing, looking), social scientists tend to understand truth as being socially constructed. Thus, social scientists tend to adopt interpretivist and constructivist approaches to understanding the world, seeing knowledge as being co-constructed, rooted in context, and an important source/expression of power.
Consolidate your learning: ‘Introduction to the social sciences’ video
To consolidate your understanding of the social sciences, watch the following short video – Introduction to the social sciences (YouTube, 8:34) .
Flyvbjerg (2001) referred to the ‘science wars’, by which he meant the ongoing battle between the natural and social sciences. Often in public and political discourse, the natural sciences are seen as being more ‘scientific’ and a source of ‘stronger’ or ‘more objective’ knowledge than the social sciences. However, the reality is that both have equally important but different things to offer. As Flyvbjerg (2001: 3) argued:
…the social sciences are strongest where the natural sciences are weakest: just as the social sciences have not contributed much to explanatory and predictive theory, neither have the natural sciences contributed to the reflexive analysis and discussion of values and interests…
As Flyvbjerg (2001) sees it, social scientists should not try to replicate the natural sciences but should instead embrace their ability to take a different ontological and epistemological outlook, which enables deep, reflexive, and contextualised analysis about people and societies as a point of departure for values-based action . He called this ‘phronetic social science’ (which we elaborate on later in the Chapter).
Defining key terms
‘Ontology’: Ontology is the study of reality and being. When we refer to ‘ontology’, we are not just talking about people’s views of the world, but also their lived experience and actual being in the world, as well as their beliefs and claims about the nature of their existence. Some key questions are ‘what and who exists in the world?’ and ‘what are the relationships between them’?
‘Epistemology’ : Epistemology concerns the origin and nature of knowledge, including how knowledge claims are built and made. Some key questions are ‘what is knowledge?’ and ‘how is knowledge acquired’?
Positivism: Positivism is an ontology that assumes there is an objective ‘truth’ waiting to be discovered. Positivism involves, therefore, the search for a universal/generalisable ‘truth’.
Constructivism: Constructivism is an ontology that assumes that there are multiple ‘truths’ that are subjective and socially constructed. Truths are not, therefore, universal but are instead rooted in social, historical, and geographical context. These ‘truths’ are also bound up with power. For instance, those who hold power get to say what is ‘true’ and what isn’t.
In addition to the above, Argentine-Canadian philosopher Mario Bunge ‘s (2003: 285ff) glossary of key terms includes a range of ontological concepts used in the social sciences that are useful to think with:
“Definitions of Twelve Ontological Concepts
- Ontology: The philosophical study of being and becoming.
- Realism (ontological): The thesis that the world outside the student exists on its own.
- Phenomenalism (ontological): The philosophical view that there are only phenomena (appearances to someone).
- Constructivism (ontological): The view that the world is a human (individual or social) construction.
- Dialectics: The ontological doctrine, due to Hegel and adopted by Marx and his followers, according to which every item is at once the unity and struggle of opposites.
- Materialism: The family of naturalist ontologies according to which all existents are material.
- Naturalism: The family of ontologies that assert that all existents are natural-hence none are supernatural.
- Idealism. The family of ontologies according to which ideas pre-exist and dominate everything else.
- Subjectivism. The family of philosophies according to which everything is in a subject’s mind (subjective idealism).
- Holism: The family of doctrines according to which all things come in unanalyzable wholes.
- Individualism: The view that the universe is an aggregate of separate individuals: that wholes and emergence are illusory.
- Systemism (ontological): The view that everything is either a system or a component of some system.”
Source: Bunge, M. (2003). E mergence and Convergence: Qualitative Novelty and the Unity of Knowledge . University of Toronto Press. Pp. 285ff
Reflection exercise
Take a few moments to think about what you have read above. Then, write a short (~100 word) reflection explaining:
- primary ways in which the natural and social sciences differ, and
- some things that the social sciences offer that the natural sciences cannot.
Why study the social sciences?
In their 2019 publication, Carré asked, ‘what are the social sciences for’? In response, they propose a framework for thinking about the different approaches and contributions of social science research, which encompasses three continuums: 1) return on investment versus intrinsic value; 2) citizen (societal) relevance versus academic relevance; and 3) applied research versus basic research (see the Figure below, adapted from Carré [2019: 23]).
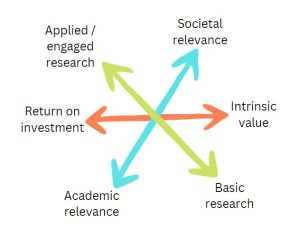
While Carré (2019) argues that social scientists move along these continuums, he also suggests that there is good justification for finding middle grounds between the extremes. For instance, while applied research will tend to focus on and find solutions for specific social issues (e.g. youth crime), ‘basic’ research tends to adopt a more high-level theoretical approach to shaping how we understand the world, which can lead to longer-term substantive change (such as changing the way we think about and understand youth crime). As Carré (2019: 22) explains: “either research is conducted to directly solve pressing social issues, or it takes a full step back from the social word, in order to reflect about it without directly meddling [and] being involved in its events and discussions.” However, both are incredibly useful for moving knowledge forward and making crucial contributions. Similarly, they can have important symbiotic relationships; applied research might be informed and guided by the knowledge created through basic research, and conversely, applied research studies might be meta-analysed (a type of combined analysis) to inform broader theoretical development that is often the purview of basic research.
A central question raised by Carré (2019) is, what should social science ‘give back’ to the society that supports it? Take a piece of paper and write down some responses to this, based on your own views and beliefs.
According to Flyvbjerg (2001), and as also covered by Schram (2012), the concept of ‘phronetic social science’ can help bring social scientists back to the central value of the social sciences, rather than seeing them try to emulate the natural sciences and their search for universal and generalisable theories and truths. Instead, phronetic social science recognises that ‘truth’ is dependent on context, is in constant flux, and is bound up with power. This is not to say that we live in a ‘post-truth’ world where anything goes, but merely that we need to interrogate how knowledge and truth are created and how societies and social structures can play a role in this. Famous sociologist, Michel Foucault (1926-1984) referred to this as a ‘politics of truth’: something we’ll continue to discuss in greater detail over coming chapters.
‘Phronetic’ social science
Phronetic social science draws on the concept of phronesis, a term coined by Aristotle (384-322 BC) to refer to practical wisdom that arises from experience. Thus, phronetic social science “is designed not to substitute for, but instead to supplement, practice wisdom and to do so in ways that can improve society” (Schram 2012: 16). In terms of improving society, phronetic social science is then also concerned with praxis, or the practical application of knowledge to the betterment of society. Finally, phronetic social science is not attached to particular methods (e.g. quantitative versus qualitative), instead being “open to relying on a diversity of data collection methods in order to best inform attempts to promote change related to the issues being studied” (Schram 2012: 20).
Schram (2012: 18-19) presents four justifications for phronetic social science as follows:
- “Given the dynamic nature of human interaction in the social world, social inquiry is best practiced when it does not seek general laws of action that can be used to predict courses of action, but instead offer a critical assessment of values, norms and structures of power and dominance. Social inquiry is better when it is linked to questions of the good life, that is, to questions of what we ought to do.
- While the social world is dynamic, social research is best seen as dialogical. Social inquiry is not a species of theoretical reason but of practical reason. Practical reason stays within a horizon of involvements in social life. For Flyvbjerg, this entails a context-dependent view of social inquiry that rests on the capacity for judgement. Understanding can never be grasped analytically; it is a holistic character. Understanding also has intrinsic subjective elements requiring researchers to forgo a disinterested position of detachment and enter into dialogue with those they study.
- As the study of dynamic social life, dialogical social inquiry is best practiced when we give up traditional notions of objectivity and truth and put aside the fact-value distinction. Instead, we should emphasise a contextual notion of truth that is pluralistic and culture-bound, further necessitating involvement with those we study.
- Dialogical social inquiry into a dynamic and changing social world provides a basis for emphasising that interpretation is itself a practice of power, one that if conducted publicly and in ways that engage the public can also challenge power and inform efforts to promote social change.”
This concept of phronetic social science is a helpful means of understanding how the social sciences differ to the natural sciences, and can add value in different ways. However, it doesn’t tell us how to do social science, or how to be social scientists. What tools, for instance, might we use to undertake the sort of dialogical social inquiry that Schram refers to above? And how might we start ‘thinking’ like social scientists? We turn to these questions in the chapter that follows.
‘Phronesis’: Described by Aristotle as ‘practical wisdom’, and juxtaposed with techn é (‘know how’ of practice) and epistem é (abstract and universal knowledge).
‘Dialogical’: Exploring the meaning of things and creating knowledge through dialogue/conversation.
‘Quantitative’ : A term used to describe research methods that typically involve measurement and counting of phenomena, regularly involving numerical data.
‘Qualitative’: A term used to describe research methods that typically involve understanding and interpretation of lived experiences (how people think, feel, act), regularly involving textual data.
Think about the concept of phronetic social science. Write a short paragraph (~30-40 words) to explain it in your own words. Then read back over the content in this chapter content to check your understanding.
Resources to support further learning
Relevant readings:
- Gorton, W. ‘ The Philosophy of Social Science .’
- Flyvbjerg, B. 2001. ‘The science wars: a way out.’ In. Flyvbjerg, B. Making social science matter, chapter 1. Cambridge University Press: Cambridge.
- Carré, D. 2019. ‘ Social sciences, what for? On the manifold directions for social research .’ In. Valsiner, J. (Ed.) Social philosophy of science for the social sciences, pp. 13-29. Springer: Cham.
- Schram, S. 2012. ‘Phronetic social science: an idea whose time has come.’ In Flyvbjerg, B., Landman, T. and Schram, S. (Eds.) Real social science: applied phronesis. Cambridge University Press: Cambridge.
- Bunge, M. (2003). E mergence and Convergence: Qualitative Novelty and the Unity of Knowledge . University of Toronto Press.
Other resources:
- Video: Soomo, ‘An animated introduction to social science’ (YouTube, 4:35) .
- Video: ‘Introduction to the social sciences’ (YouTube, 8:34) .
- Podcast: Theory and Philosophy Podcast, ‘Bent Flyvbjerg – Making Social Science Matter’ (YouTube, 44:06) . (Note, discussion of phronesis starts at 7:51)
- Video: ‘Importance of social science with Professor Cary Cooper’ (YouTube, 4:13) .
Introduction to the Social Sciences Copyright © 2023 by The University of Queensland is licensed under a Creative Commons Attribution 4.0 International License , except where otherwise noted.
Share This Book
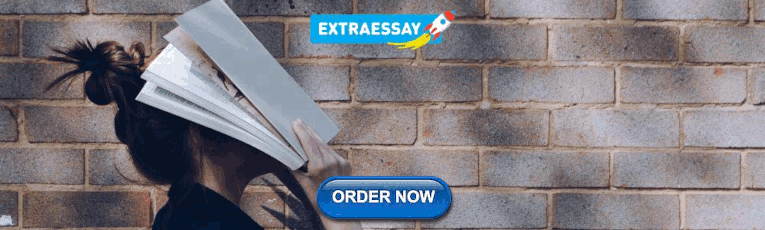
IMAGES
COMMENTS
An experiment is a method of data collection designed to test hypotheses under controlled conditions, with the goal to eliminate threats to internal validity. There are different experiment designs. In the classic experiment, the effect of a stimulus is tested by comparing two groups: one that is exposed to the stimulus (the experimental group ...
An experiment is a method of data collection designed to test hypotheses under controlled conditions. In social scientific research, the term experiment has a precise meaning and should not be used to describe all research methodologies. Experiments have a long and important history in social science.
Sociology. A social experiment is a method of psychological or sociological research that observes people's reactions to certain situations or events. The experiment depends on a particular social approach where the main source of information is the participants' point of view and knowledge. To carry out a social experiment, specialists usually ...
10 Experimental research. 10. Experimental research. Experimental research—often considered to be the 'gold standard' in research designs—is one of the most rigorous of all research designs. In this design, one or more independent variables are manipulated by the researcher (as treatments), subjects are randomly assigned to different ...
Trying out a new restaurant, dating a new person - we often call these things "experiments." However, a true social science experiment would include recruitment of a large enough sample, random assignment to control and experimental groups, exposing those in the experimental group to an experimental condition, and collecting observations ...
experiments in political science and use many examples from political science. That said, the arguments I make and the suggestions I offer apply to any social science application of an experiment - which as will be discussed in detail - I define as a study where an intervention (by
The rise of experiments in the social sciences1.1. Tracking the increasing presence of experiments in top social science journals. The experimental method has long been part of social scientists' methodological toolkit (for a history of the experimental method in various contexts, see Druckman et al., 2006; Salsburg 2001; Thye 2014a).
Social Experiments, History of. Trudy Dehue, in Encyclopedia of Social Measurement, 2005. Social Experiments in the 19th Century Early Definitions. The RCT is a fairly recent development. Before the 1910s, no expert on social research advanced the idea of comparing artificially composed experimental and control groups, and before the 1920s none of them proposed to compose groups on the basis ...
Key Takeaways. Experimental designs are useful for establishing causality, but some types of experimental design do this better than others. Experiments help researchers isolate the effect of the independent variable on the dependent variable by controlling for the effect of extraneous variables.; Experiments use a control/comparison group and an experimental group to test the effects of ...
A social experiment is the random assignment of human subjects to two groups to examine the effects of social policies. One group, called the " treatment group, " is offered or required to participate in a new program, while a second group, the " control group, " receives the existing program. The two groups are monitored over time to ...
This overview of the experiment in the social sciences addresses the differing definitions but also focuses on some of the conceptual themes and foundations common across more specialized and applied topics in experimental methodology (e.g., field experiments, laboratory experiments, and quasi-experiments) and across disciplines.
Experiment Definition in Science. By definition, an experiment is a procedure that tests a hypothesis. A hypothesis, in turn, is a prediction of cause and effect or the predicted outcome of changing one factor of a situation. Both the hypothesis and experiment are components of the scientific method. The steps of the scientific method are:
Experimental research is best suited for explanatory research—rather than for descriptive or exploratory research—where the goal of the study is to examine cause-effect relationships. It also works well for research that involves a relatively limited and well-defined set of independent variables that can either be manipulated or controlled.
Various methods are applied to experimental social psychology. Traditional methods include laboratory experiment, natural experiment, systematic observation, archival research, test, statistics (including correlation), simulation, interview, questionnaire, etc. Experimental social psychology study adopts either one of the above methods or several methods at the same time, thus producing ...
An experiment is a data collection procedure that occurs in controlled conditions to identify and understand causal relationships between variables. Researchers can use many potential designs. The ultimate choice depends on their research question, resources, goals, and constraints. In some fields of study, researchers refer to experimental ...
Armin Falk1 and James J. Heckman2. Laboratory experiments are a widely used methodology for advancing causal knowledge in the physical and life sciences. With the exception of psychology, the adoption of laboratory experiments has been much slower in the social sciences, although during the past two decades the use of lab experiments has ...
Experiments might be categorized according to a number of dimensions, depending upon professional norms and standards in different fields of study. In some disciplines (e.g., psychology or political science), a 'true experiment' is a method of social research in which there are two kinds of variables.
Using field experiments, scholars can identify causal effects via randomization while studying people and groups in their naturally occurring contexts. In light of renewed interest in field experimental methods, this review covers a wide range of field experiments from across the social sciences, with an eye to those that adopt virtuous practices, including unobtrusive measurement ...
Reactivity, or the phenomenon by which subjects tend to modify their behavior in virtue of their being studied upon, is often cited as one of the most important difficulties involved in social scientific experiments, and yet, there is to date a persistent conceptual muddle when dealing with the many dimensions of reactivity. This paper offers a conceptual framework for reactivity that draws on ...
Within social science the experiment has an ambiguous place. With the possible exception of social psychology, there are few examples of strictly experimental studies. The classic study still often cited is the Hawthorne experiments, which began in 1927, and is used mainly to illustrate what became known as the 'Hawthorne Effect', that is ...
An experiment is a procedure designed to test a hypothesis as part of the scientific method. The two key variables in any experiment are the independent and dependent variables. The independent variable is controlled or changed to test its effects on the dependent variable. Three key types of experiments are controlled experiments, field ...
Donald P. Green (Ph.D., University of California at Berkeley) is Professor of Political Science at Columbia University. The author of four books and more than one hundred essays, Green's research interests span a wide array of topics: voting behavior, partisanship, campaign finance, hate crime, and research methods.
Experiment: In experimental research, scientists use a specific experimental design (which includes a control and experimental group) to test hypotheses. This is not always possible or desirable in the social sciences, so social scientists tend to rely on a broader array of methods to collect data that can help them test their hypotheses about ...