(E-cadherin)
16q22.1 | tumor suppressor gene | Inactivation of CDH1 in 85% of lobular breast carcinomas | | FHIT | 3p14.2 | putative tumor suppressor gene | The rate of FHIT hypermethylation in breast cancer was 8.4-fold higher than that in normal breast tissues | |
Maspin | 18q21.33 | tumor suppressor gene | Expression of Maspin in 20-80% invasive breast cancer | , |
PIK3CA | 3q26.3 | oncogene | Mutations in 37% of the HR /HER2 metastatic and 40% of early breast cancer | |
CCND1 (Cyclin D1) | 11q13 | oncogene | Overexpression in 50% of breast tumors | |
Notes: SNP-Single Nucleotide Polymorphisms; HR-Hazard ratio
Acknowledgments
We would like to thank Dr. Yan-Ling Wu for her advice on this paper. This work is supported by Zhejiang Provincial Natural Science Foundation of China under Grant No. LQ15C040001 and LY18H190003, Social Development Project of Public Welfare Technology Research in Zhejiang Province (LGF18H100002), and Zhejiang Provincial Foundation for Scientific Research in Medicine and Health (2015RCB009).
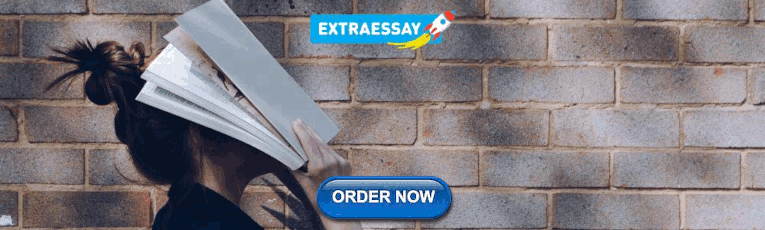
IMAGES
COMMENTS
Breast cancer (BC) is a highly prevalent malignancy worldwide, with complex pathogenesis and treatment challenges. Research reveals that methyltransferase-like 3 (METTL3) is widely involved in the pathogenesis... Dongqiong Xiao, Mingfu Zhang, Yi Qu and Xiaojuan Su. Breast Cancer Research 2024 26 :110.
Breast Cancer Research is an international, peer-reviewed online journal, publishing original research, reviews, editorials and reports. Open access research articles of exceptional interest are published in all areas of biology and medicine relevant to breast cancer, including normal mammary gland biology, with special emphasis on the genetic, biochemical, and cellular basis of breast cancer.
Breast cancer is currently one of the most prevalently diagnosed cancers and the 5th cause of cancer-related deaths with an estimated ... Colombet M., Mery L., Piñeros M., Znaor A., Soerjomataram I., Bray F. International Agency for Research on Cancer; Lyon, France: 2020. [(accessed on 9 July 2021)]. Global Cancer Observatory: Cancer Tomorrow. ...
Read the latest Research articles in Breast cancer from Nature. ... An analysis of 780 breast cancer genomes shows that focal amplifications are frequently preceded by dicentric chromosome ...
1. Introduction. Breast cancer has a very long history as it was first reported by the ancient Egyptians more than 3500 years ago in about 1500 B.C [].Today, breast cancer is the second most prevalent type of cancer and is a leading cause of most cancer-related deaths in women in the United States [].Around 281,550 women are projected to be diagnosed with breast cancer in 2021, and 43,600 ...
Go to: 1. Introduction. Breast cancer (BC) is the most frequent cancer and the second cause of death by cancer in women worldwide. According to Cancer Statistics 2020, BC represents 30% of female cancers with 276,480 estimated new cases and more than 42,000 estimated deaths in 2020 [ 1 ]. Invasive BC can be divided into four principal molecular ...
The estimated new breast cancer cases reached 2.3 million in 2020, accounting for 11.7% of all new cancers, and 684,996 cases died of it . In China, breast cancer was the most common malignancy among women, with an estimated number of 306,000 new cases occurring in 2016 . The incidence of breast cancer has increased since the widespread uptake ...
Posted: January 20, 2023. Many young women who are diagnosed with early-stage breast cancer want to become pregnant in the future. New research suggests that these women may be able to pause their hormone therapy for up to 2 years as they try to get pregnant without raising the risk of a recurrence in the short term.
The latest research evidence 1 shown that 44.4% of global cancer deaths and 42.0% of global cancer disability-adjusted life years can be attributed to GBD 2019 estimated risk factors. Our study ...
Breast Cancer Research and Treatment is a comprehensive forum dedicated to all aspects of breast cancer research. The journal's focus spans across various disciplines including surgery, radiotherapy, medical oncology, endocrinology, epidemiology, immunology and cell biology. Provides an international platform for the discussion and resolution ...
Breast Cancer: Basic and Clinical Research is an international, peer-reviewed, open access journal that covers all aspects of research and treatment of breast cancer. The journal aims to promote understanding of breast cancer biology and pathogenesis, clinical interventions, and epidemiology and population genetics. ... Submit your paper to one ...
Assuming that the underlying burden of clinically meaningful breast cancer was unchanged, these data suggest that 30 cases of cancer per 100,000 women were destined to become large but were ...
Breast cancer is caused by the development of malignant cells in the breast. The malignant cells originate in the lining of the milk glands or ducts of the breast (ductal epithelium). Breast ...
PDF | Breast cancer is the most frequent malignancy in women worldwide and is curable in ~70-80% of patients with early-stage, non-metastatic disease.... | Find, read and cite all the research you ...
Advances in Breast Cancer Research. A polyploid giant cancer cell (PGCC) from triple-negative breast cancer. NCI-funded researchers are working to advance our understanding of how to prevent, detect, and treat breast cancer. They are also looking at how to address disparities and improve quality of life for survivors of the disease.
Breast cancer is still the most common cancer worldwide. But the way breast cancer is viewed has changed drastically since its molecular hallmarks were extensively characterised, now including immunohistochemical markers (eg, ER, PR, HER2 [ERBB2], and proliferation marker protein Ki-67 [MKI67]), genomic markers (eg, BRCA1, BRCA2, and PIK3CA), and immunomarkers (eg, tumour-infiltrating ...
In this review, we will highlight the different diagnosis techniques and treatment strategies for breast cancer management and explore recent advances in breast cancer theranostics. Our main focus will be to summarize recent trends and technologies in breast cancer diagnosis and treatment as reported in recent research papers and patents and ...
The relationship between hormonal contraceptive use and breast cancer risk has been demonstrated in two important papers—a reanalysis of 54 epidemiological studies by the ... fish, legumes, oils, and vegetable oils reduces the risk of breast cancer . Research suggests that nutrition affects the prognosis of breast cancer. ...
1. Set learning rate to 10 −3 and train the last layer for 3 epochs. 2. Set learning rate to 10 −4, unfreeze the top layers and train for 10 epochs, where the top layer number is set to 46 for ...
Deep learning analysis of radiological images has the potential to improve diagnostic accuracy of breast cancer, ultimately leading to better patient outcomes. This paper systematically reviewed the current literature on deep learning detection of breast cancer based on magnetic resonance imaging (MRI). The literature search was performed from 2015 to Dec 31, 2022, using Pubmed.
The object of this study was to identify and analyze the qualities of the top 100 cited papers in breast cancer-related studies. Materials and Methods. ... Breast cancer research has progressed historically and gone through milestones in various areas in this process. These areas include breast cancer gene associations, breast cancer treatment ...
Introduction Breast cancer remains a significant scientific, clinical and societal challenge. This gap analysis has reviewed and critically assessed enduring issues and new challenges emerging from recent research, and proposes strategies for translating solutions into practice. Methods More than 100 internationally recognised specialist breast cancer scientists, clinicians and healthcare ...
Introduction. Breast cancer is one of the most common cancers in women worldwide, accounting for approximately 570,000 deaths in 2015. Over 1.5 million women (25% of all women with cancer) are diagnosed with breast cancer every year throughout the world 1, 2.In America, it is estimated that 30% of all new cancer cases (252,710) among women are breast cancer in 2017 3.