Advertisement
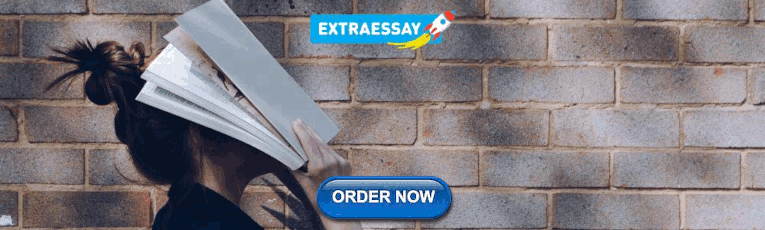
Assessing laptop use in higher education: The Laptop Use Scale
- Published: 30 December 2015
- Volume 28 , pages 18–44, ( 2016 )
Cite this article
- Robin Kay 1 &
- Sharon Lauricella 2
2431 Accesses
5 Citations
7 Altmetric
Explore all metrics
The laptop computer is considered one of the most used and important technological devices in higher education, yet limited systematic research has been conducted to develop a measure of laptop use in college and university. The purpose of the following study was to develop a research-based, theoretically grounded scale to assess student use of laptops inside and outside higher education classrooms. The Laptop Use Scale addressed four key areas: in-class academic use, in-class non-academic use, outside of class academic use, and outside of class non-academic use. Tested on 156 higher education students using laptops computers, the Laptop Use Scale showed acceptable internal reliability and good validity (face, content, construct, and convergent validity). It is argued that this scale can help assess and calibrate pedagogical strategies used to integrate laptops into higher education classrooms. Suggestions for future research on assessing student use of laptops are offered including a focus on multi-tasking behavior.
This is a preview of subscription content, log in via an institution to check access.
Access this article
Subscribe and save.
- Get 10 units per month
- Download Article/Chapter or eBook
- 1 Unit = 1 Article or 1 Chapter
- Cancel anytime
Price includes VAT (Russian Federation)
Instant access to the full article PDF.
Rent this article via DeepDyve
Institutional subscriptions
Similar content being viewed by others
Limiting Off-Task Behavior on Laptops in Classrooms Increases Student Engagement: Use It, or They Will Abuse It
The impact of technology exposure on student perceptions of a 1:1 program.
Transforming Teaching and Learning: Implications of a One-to-One Laptop Initiative for Professional Learning and Teacher Practices
Explore related subjects.
- Digital Education and Educational Technology
Aguilar-Roca, N. M., Williams, A. E., & O’Dowd, D. K. (2012). The impact of laptop-free zones on student performance and attitudes in large lectures. Computers and Education, 59 (4), 1300–1308. doi: 10.1016/j.compedu.2012.05.002 .
Article Google Scholar
Annan-Coultas, D. L. (2012). Laptops as instructional tools: Student perceptions. TechTrends, 56 (5), 34–41.
Awwad, F., & Ayesh, A. (2013). Effectiveness of laptop usage in UAS university undergraduate teaching. The Turkish Online Journal of Educational Technology, 12 (2), 77–88.
Google Scholar
Barak, M., Lipson, A., & Lerman, S. (2006). Wireless laptops as means for promoting active learning in large lecture halls. Journal of Research on Technology in Education, 38 (3), 245–263.
Barkhuus, L. (2005). Bring your own laptop unless you want to follow the lecture: Alternative communication in the classroom. In Proceedings of the 2005 International ACM SIGGROUP Conference on Supporting Group Work (pp. 140–143). New York, ACM. doi: 10.1145/1099203.1099230 .
Bruner, J. (1960). The process of education . Cambridge, MA: Harvard University Press.
Carfio, J., & Perla, R. (2008). Resolving the 50-year debate around using and misusing Likert scales. Medical Education, 42 (12), 1150–1152. doi: 10.1111/j.1365-2923.2008.03172.x .
Dahlstrom, E., Walker, J. D., & Dziuban, C, M. (2013). The ECAR study of undergraduate students and information technology (Research Report) . Louiseville, CO: EDUCAUSE Center for Analysis and Research. Retrieved from https://net.educause.edu/ir/library/pdf/ERS1302/ERS1302.pdf
Dalsgaard, C., & Godsk, M. (2007). Transforming traditional lectures into problem-based blended learning: Challenges and experiences. Open Learning: The Journal of Open, Distance and e-Learning, 22 (1), 29–42. doi: 10.1080/02680510601100143 .
Debevec, K., Shih, M., & Kashyap, V. (2006). Learning strategies and performance in a technology integrated classroom. Journal of Research on Technology in Education, 38 (3), 293–307.
DiGangi, S., Kilic, Z., Yu, C. H., Jannasch-Pennell, A., Long, L., Kim, C., et al. (2007). One to one computing in higher education: A survey of technology practices and needs. AACE Journal, 15 (4), 367–387.
Enfield, J. (2014). Looking at the impact of the flipped classroom model of instruction on undergraduate multimedia students at CSUN. TechTrends, 57 (6), 14–27. doi: 10.1007/s11528-013-0698-1 .
Field, A. (2005). Discovering statistics using SPSS (2nd ed.). Thousand Oaks, CA: Sage Publications.
Fried, C. B. (2008). In-class laptop use and its effects on student learning. Computers and Education, 50 (3), 906–914.
Gagné, R. (1985). The conditions of learning . New York: Holt, Rinehart and Winston.
Gaudreau, P., Miranda, D., & Gareau, A. (2014). Canadian university students in wireless classrooms: What do they do on their laptops and does it really matter? Computers and Education, 70 (3), 245–255. doi: 10.1016/j.compedu.2013.08.019 .
Hyden, P. (2005). Teaching statistics by taking advantage of the laptop’s ubiquity. New Directions for Teaching and Learning, 101 , 37–42.
Jamieson, S. (2004). Likert scales: how to (ab)use them. Medical Education, 38 (12), 1217–1218. doi: 10.1111/j.1365-2929.2004.02012.x .
Kay, R. H., & Lauricella, S. (2011). Exploring the benefits and challenges of using laptop computers in higher education classrooms: A formative analysis. Canadian Journal of Learning and Technology , 37 (1). Retrieved from http://www.cjlt.ca/index.php/cjlt/article/view/565/299
Kay, R. H. & Lauricella, S. (2014). Investigating the benefits and challenges of using laptop computers in higher education. Canadian Journal of Learning and Technology, 40 (2), 1–25. Retrieved from http://www.cjlt.ca/index.php/cjlt/article/view/831/393
Kline, P. (1999). The handbook of psychological testing (2nd ed.). London: Routledge.
Kolb, A. Y., & Kolb, D. A. (2005). Learning styles and learning spaces: Enhancing experiential learning in higher education. Academy of Management Learning and Education, 4 (2), 193–212. doi: 10.5465/AMLE.2005.17268566 .
Kraushaar, J. M., & Novak, D. C. (2010). Examining the effects of student multitasking with laptops during the lecture. Journal of Information Systems Education, 21 (2), 241–251.
Kuzon, W. M., Urbanchek, M. G., & McCabe, S. (1996). The seven deadly sins of statistical analysis. Annals of Plastic Surgery, 37 , 265–272.
Lasry, N., Mazur, E., & Watkins, J. (2008). Peer instruction: From Harvard to the two-year college. American Association of Physics Teachers , 76 (11), 1066–1069. Retrieved from http://mazur.harvard.edu/sentFiles/Mazur_61464.pdf
Lauricella, S. & Kay, R. H. (2010). Assessing laptop use in higher education classrooms: The laptop effectiveness scale (LES). Australian Journal of Educational Technology , 26 (2), 151–163. Retrieved from http://ajet.org.au/index.php/AJET/article/view/1087/342
Laurillard, D. (2002). Rethinking university teaching. A conversational framework for the effective use of learning technologies . London: Routledge.
Book Google Scholar
Lave, J., & Wenger, E. (1991). Situated learning: Legitimate peripheral participation . Cambridge: Cambridge University Press.
Lewis, S. E., & Lewis, J. E. (2005). Departing from lectures: An evaluation of a peer-led guided inquiry alternative. Journal of Chemical Education, 82 (1), 135. doi: 10.1021/ed082p135 .
Lindroth, T., & Bergquist, M. (2010). Laptopers in an educational practice: Promoting the personal learning situation. Computers and Education, 54 (2), 311–320. doi: 10.1016/j.compedu.2009.07.014 .
Mayes, T., & de Freitas, S. (2007). Technology-enhanced learning: The role of theory. In H. Beetham & R. Sharpe (Eds.), Rethinking pedagogy for a digital age (pp. 17–30). New York: Routledge.
McCreary, J. R. (2009). The laptop-free zone. Valparaiso University Law Review, 43 , 1–87.
Miles, M. B., & Hubrman, M. (1994). Qualitative data analysis (2nd ed.). Thousand Oaks, CA: Sage Publication.
Murray, J. (2013). Likert data: What to use, parametric or non-parametric? International Journal of Business and Social Science, 4 (11), 258–264.
Newell, A. (1980). One final word. In D. T. Tuma & F. Reif (Eds.), Problem solving and education: Issues in teaching and research (pp. 175–189). Hillsdale, New Jersey: Lawrence Erlbaum Associates.
Norman, G. (2010). Likert scales, levels of measurement and the ‘‘laws’’ of statistics. Advances in Health Sciences Education—Theory and Practice, 15 (5), 625–632. doi: 10.1007/s10459-010-9222-y .
Nunnally, J. C. (1978). Psychometric theory . New York: McGraw-Hill.
Pew Research Center. (2013). Social media update 2013 . Washington: DC. Retrieved from http://www.pewinternet.org/files/2013/12/PIP_Social-Networking-2013.pdf
Piaget, J. (1970). Science of education and the psychology of the child . New York: Orion Press.
Prensky, M. (2010). Teaching digital natives . Thousand Oaks, CA: Corwin.
Sana, F., Weston, T., & Cepeda, N. J. (2013). Laptop multitasking hinders classroom learning for both users and nearby peers. Computers and Education, 62 (1), 24–31. doi: 10.1016/j.compedu.2012.10.003 .
Skolnik, R., & Puzo, M. (2008). Utilization of laptop computers in the school of business classroom. Academy of Educational Leadership Journal, 12 (2), 1–10.
Small, G., & Vorgan, G. (2009). iBrain: Surviving the technological alteration of the modern mind . New York: Harper.
Tapscott, D. (2009). Grown up digital: How the Net generation is changing your world . New York: McGraw-Hill.
Vygotsky, L. S. (1978). Mind and society . Cambridge, MA: Harvard University Press.
Wilson, B. G., & Myers, K. M. (2000). Situated cognition is theoretical and practical context. In D. H. Jonassen & S. M. Land (Eds.), Theoretical foundations of learning environments (pp. 57–86). New Jersey: Lawrence Erlbaum.
Wurst, C., Smarkola, C., & Gaffney, M. A. (2008). Ubiquitous laptop usage in higher education: Effects on student achievement, student satisfaction, and constructivist measures in honors and traditional classrooms. Computers and Education, 51 (4), 1766–1783. doi: 10.1016/j.compedu.2008.05.006 .
Download references
Author information
Authors and affiliations.
Faculty of Education, University of Ontario Institute of Technology, 11 Simcoe St N, PO Box 385, Oshawa, ON, L1H 7L7, Canada
Faculty of Social Science and Humanities, University of Ontario Institute of Technology, 55 Bond Street East, Bordessa Hall, Oshawa, ON, L1H 7L7, Canada
Sharon Lauricella
You can also search for this author in PubMed Google Scholar
Corresponding author
Correspondence to Robin Kay .
Appendix: Laptop Use Scale
Background information.
What is your gender? (Male, Female)
What year of university are you in? (1, 2, 3 or 4)
What was your average grade in all your courses last year? (<50, 50–59, 60–69, 70–70, 80–89, 90+)
What course are you taking? _____________
What is your average in the course right now? (<50, 50–59, 60–69, 70–70, 80–89, 90+)
How comfortable are you with using computer technology? (Not at all Comfortable, Somewhat Comfortable, Comfortable, Very Comfortable)
About how many hours per day do you spend using your laptop computer? _____
Academic use DURING class
How often did you do the following activities DURING class in this course?
Overall what (if any) do you see are the biggest benefits to having a laptop IN class for this course? Why?
Non-academic use DURING class
Overall what (if any) do you see are the biggest distractions to having a laptop IN class for this course? Why?
Academic OUTSIDE if class
How often did you do the following activities OUTSIDE of class in this course?
Overall what (if any) do you see are the biggest benefits to having a laptop OUTSIDE class? Why?
Overall what (if any) do you see are the biggest distractions to having a laptop OUTSIDE class? Why?
Rights and permissions
Reprints and permissions
About this article
Kay, R., Lauricella, S. Assessing laptop use in higher education: The Laptop Use Scale. J Comput High Educ 28 , 18–44 (2016). https://doi.org/10.1007/s12528-015-9106-5
Download citation
Published : 30 December 2015
Issue Date : April 2016
DOI : https://doi.org/10.1007/s12528-015-9106-5
Share this article
Anyone you share the following link with will be able to read this content:
Sorry, a shareable link is not currently available for this article.
Provided by the Springer Nature SharedIt content-sharing initiative
- Higher education
- Find a journal
- Publish with us
- Track your research
- Open access
- Published: 01 November 2021
Health-related consequences of the type and utilization rates of electronic devices by college students
- Mark Benden 1 , 2 , 3 ,
- Ranjana Mehta 1 , 2 , 3 ,
- Adam Pickens 1 , 3 ,
- Brett Harp 1 , 3 ,
- Matthew Lee Smith 3 , 4 ,
- Samuel D. Towne Jr 3 , 4 , 5 , 6 , 7 &
- S. Camille Peres 1 , 2 , 3
BMC Public Health volume 21 , Article number: 1970 ( 2021 ) Cite this article
5828 Accesses
6 Citations
69 Altmetric
Metrics details
College students are leading an evolution of device use both in the type of device and the frequency of use. They have transitioned from desktop stations to laptops, tablets, and especially smartphones and use them throughout the day and into the night.
Using a 35-min online survey, we sought to understand how technology daily usage patterns, device types, and postures affect pain and discomfort to understand how knowledge of that pain might help students avoid it. Data were analyzed from 515 students (69.5% male) who completed an internet-delivered survey (81.3% response rate).
Participants ranked smartphones as their most frequently used technology (64.0%), followed by laptops and tablets (both 53.2%), and desktop computers (46.4%). Time spent using smartphones averaged over 4.4 h per day. When using their devices, students were more likely to adopt non-traditional workplace postures as they used these devices primarily on the couch or at a chair with no desk.
Recent trends in wireless academic access points along with the portability of small handheld devices, have made smartphones the most common link to educational materials despite having the least favorable control and display scenario from an ergonomic perspective. Further, the potential impact of transitions in work environments due to COVID-19 may further exacerbate ergonomic issues among millions highlighting the need for such work to be carried out.
Peer Review reports
Introduction
College students, many of whom are future office workers, have higher levels of screen time and utilize multiple devices at higher rates compared to previous generations [ 1 ]. These have resulted in the increases in computer-related musculoskeletal disorders in this specific age group [ 2 ]. Studies have shown laptops to be ubiquitous with the college experience [ 3 ] and multiple studies have found elevated musculoskeletal discomfort associated with awkward postures for laptop use among laptop-using college students [ 4 ]. In some studies—where the prevalence of poor postures was measured across different devices such as tablets, cell phones—laptops and tablets were found to be the worst in terms posture and comfort [ 5 ]. Several studies have also explored the use of laptops and tablets as it pertains to discomfort. In those studies, discomfort from laptop and tablet use were clearly connected to poor posture and musculoskeletal symptoms [ 6 , 7 ]. Studies have reported adoption of poor or awkward postures in conjunction with electronic devices, such as a preference to sit without support when using mobile devices or bending head/neck to view small-screen devices, have led to increased reports of musculoskeletal pain [ 8 ]. However, there are currently some important gaps in this literature particularly given the trends showing movement in the direction of increased smartphone use [ 9 ]. Further, smartphone ownership among Americans reached 85% versus 77% for devices such as laptops or desktops and 53% for tablet computers according to a recent Pew Research Report [ 10 ]. Further, it is necessary to understand those trends from an ergonomic risk perspective. Specifically it is important to understand for college student device use: a) any connection of the rising use of smartphones with respect to posture and self-reported musculoskeletal pain; and b) contextualize smartphone posture and pain relative to laptops, tablets, and desktops.
Broad implications for public health
While identifying all relevant public health implications associated with poor ergonomic behaviors is outside the scope of this study, given wide variation across settings, populations, and possible health outcomes, we provide some brief examples here. For example, one study investigating musculoskeletal-related problems among students in medical science found high rates of lab-related musculoskeletal issues with the lower back reported as the most common site of issues in the past year [ 11 ]. While much broader in terms of work settings and geography, one example investigating lower back pain found the estimated global burden associated with lower back pain (occupationally related) was 21.7 million Disability-Adjusted Life Years (DALYs) [ 12 ] in 2010. While this is provided as an example of the potential global burden associated with certain ergonomic-related risk factors, these risk factors and the associated burden can vary as do potential solutions.
Some potential solutions
In terms of potential solutions to certain ergonomic-related risks, one study investigating ways to reduce the musculoskeletal burden of workers found that ergonomic intervention implementation was associated with reductions in both severe and frequent pain [ 13 ]. Further, one somewhat recent review found limited evidence of effective ergonomic interventions from 15 randomized controlled trials, though their inclusion criteria may not represent all settings, interventions, target populations, or location of musculoskeletal disorders (e.g., focused on upper limb and neck) [ 14 ]. The authors of this review indicated further need of high-quality research into potential interventions in office settings, among other findings. Furthermore, more general considerations, though not necessarily exhaustive, are worth mentioning.
In general terms, utilization of 1) an optimized control device for data entry, 2) a visual display of that data, and 3) having the display located at an optimal viewing distance, angle, height, and luminance has become the ergonomic standard for most professional work. Translated to what is commercially available today we would describe that optimum control/display product as an ergonomic keyboard and separate mouse along with a well-positioned and high-quality monitor. Monitor size and multiple monitors has begun to erode that theoretical optimum but generally, these combinations are considered more beneficial to user health and safety than the next major step down in ergonomics, a laptop with integrated mouse. Since in this device the displays and controls are connected, one must compromise position of one over the other. Additionally, size of both and design limitations make these combinations more of an occasional use and or portable/travel use device. Finally, handheld tablets and then smartphones are considered the lowest level of ergonomics since once again, the control and display are integrated but, in this case, they are on the same plane and in the truest sense, the display is the control. For each part of portability and weight positives users gain through this progression, they give up on ergonomics, comfort, etc. In the final level of smartphones, they even provide the physical support for the device during use. In classic human factors or industrial engineering terms, this wasteful use of muscle energy is unproductive work that leads to fatigue and then altered and later poor postures with increased use. To further complicate the challenge of providing ideal ergonomic environments is the disruption linked to the dramatic shift of both university education and previously office-based work environments of major industries transitioning, in some cases for many months, to remote working conditions. This may force individuals to rely on their homes to serve as office-like environments, which may not be properly resourced.
In addition to traditional ergonomic risk factors, such as awkward posture and repetitive behavior, non-physical factors, such as cognitive overload or stress, have shown to impact biomechanical and behavioral outcomes, across a variety of activities, ranging from typing [ 15 ] to walking [ 16 ]. For example, the Ecological Model of MSD [ 17 ] proposes that combined physical and psychological strain may affect the detection, labeling, and attribution of sensations arising as the result of biomechanical strain as well as the behavioral response to symptoms. Additional mental demand and/or mental stress have been shown to adversely influence upper extremity muscle activity [ 18 , 19 , 20 ], which over a prolonged period of time, can lead to the development of MSDs [ 21 ]. It is thus likely that the risk of prolonged usage of electronic devices, which imposes significant muscle activity due to sustained and awkward postures, may be compounded under stress originating from a variety of sources.
This study seeks to build on knowledge of college student device use and to further connect it to the rising use of smartphones with respect to posture and musculoskeletal self-reports of pain and to put those smartphone sources in context relative to laptops, tablets, and desktops. Our intention with this study was to first understand this backdrop of ergonomics as it relates to devices and then to quantify how much time college students with all three types of devices spend on each user scenario and how they felt it impacted their body.
Participants
Participants were recruited from a large southwestern tier-1 land-grant university’s geosciences department during the Fall semester as part of a baseline assessment before an ergonomics training program. Upon receipt of administrative information for the semester from the department, all participants were requested to complete a survey online (created in Qualtrics®). Of the 631 students in the program (495 undergraduate and 136 graduate), 515 fully completed the survey (81.3% response rate). The study was approved by the University Institutional Review Board and all methods were performed in accordance with the relevant guidelines and regulations.
The survey distributed to participants was developed by the investigators and contained questions regarding several topic areas on ergonomics knowledge, technology use, and health. This paper, and the items covered herein, will focus on the: a) technology participants used regularly and how much they used that technology; b) postures participants adopted with that technology; and c) amount of pain or discomfort participants were currently experiencing. As mentioned previously, the survey was distributed to participants during the beginning of the Fall 2014 semester before students had participated in ergonomic training programs. The survey was conducted online and took approximately 35 min to complete. No incentives were provided to complete the survey.
Device usage
Participants responded to several sets of questions created for this study to determine the types of interactive technology (including a control and a display) they used on a regular basis and the level to which they used this technology. The question lines were as follows: “What types of interactive technology devices do you use on a regular basis (e.g., most days of the week?) Check all that apply. ( Tech use )”; “What types of interactive technology devices do you use most often? Click & Drag to arrange in order. ( Tech most )” ; “What % of the time are you interacting with your device: (Note: 0-100%. Please ensure this total to 100% combined device time). ( Tech for )” ; “On average, on any given day, how many minutes do you spend interacting with the following device(s) continuously without a break? (Tech no break )”; “On average, how many hours do you spend interacting with the following device(s) each day? (There were clarifying instructions regarding the term “interacting”.) ( Hours tech )”. It should be noted that for those who indicated they were gamers, they were asked to indicate how many hours they spent per day gaming as well. Students self-identified their “gamer” status as this term was identified by student researchers as commonplace among college students. For the study’s purposes, the term generally refereed to those spending multiple hours per week in competitive computer games. Each of the questions required the participants to respond based on five different categories: Tablet, Desktop, Laptop, Smartphone, and Other (participants could indicate what the Other category represented). Finally, participants were asked to indicate the percent of time they used any technology ( Tech for) for the following activity: School, Work, Recreation, or Other.
Participants responded to 18 items asking them to indicate how frequently they were in the position displayed for that item. Their frequency responses were based on a 9-point scale with response categories ranging from “Never” to “More than 12 hours per day”. The images for the 18 items are described in Table 3 of the Results sections and the measure had a Chrobach’s alpha of 0.779. The images were adopted from several sources with six keyboard related images purchased from an online source [ 22 ]; four images showing postures with handheld devices came from a published Steelcase posture study [ 23 ]; and the remainder were purchased from another online image source [ 24 ].
Participants were asked to indicate the amount of pain or discomfort using a body map questionnaire with 16 different body regions [ 25 ]. The body regions were presented in a diagram format on the Qualtrics® survey and participants clicked on a body part to indicate the level of pain they were currently experiencing in that part. The body regions are listed in Table 5 of the Results section and participants responded on a 5-point scale: 1 - (None) No Pain/Discomfort; 2 - (Just Noticeable) Pain/Discomfort Does Not Restrict Activity; 3 - (Some Pain/Discomfort) Restricts Some Activity; 4 - (Moderate Pain/Discomfort) Restricts Most Activity); and 5 - (Intolerable Pain/Discomfort) Restricts All Activity. This questionnaire has been used widely in ergonomic research as it provides a clear reference to the body region the participant is to rate and can be done quickly and easily [ 26 , 27 , 28 ]. There was an overall Chrobach’s alpha of 0.894 for this measure.
Activity and stress
There were four questions created for this study that asked participants to indicate the number of days per week they engaged in moderate and vigorous exercise per week as well as the number of minutes per day they engaged in that experience. These were free response items. Participants also responded to the following item regarding stress: “Please choose the number (0-10) that best describes how much stress you have been experiencing in the last 30 days? 10 = Extreme Stress, 0 = No Stress.”
To identify differences in how long and where participants used their devices, repeated measure analysis of variance (RMANOVA) were conducted. These analyses were corrected for Sphericity (when necessary) using the Greenhouse-Geisser correction.
Data reduction analyses were conducted with the posture and pain responses using Principal Component Analysis (PCA), with Varimax orthogonal rotation using Kaiser Normalization. These analyses were conducted on the data obtained from all participants ( n = 515) to determine the underlying structure of the items on the survey. Pairwise exclusion was used to adjust for those with missing data. All other analyses were performed on the participants with complete data (Posture: n = 512 and Pain: n = 506).
To identify predictors of participants’ pain, separate stepwise multiple regressions (SMR) were conducted for each of the components identified in the PCA for pain. The SMR included the predictor variables: Tech most, Tech use (for each device), Tech for (for each device), Tech no break (for each device), Hours tech (for each device), Sex, BMI, Positions (components from PCA). Due to the low number of participants in the gaming and “other” categories, these variables were not included in the SMR.
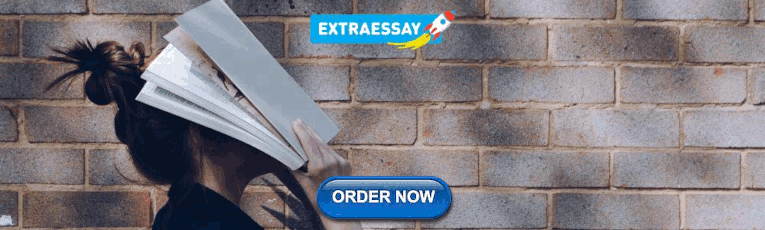
Demographics
The demographics (academic level, ethnicity, race, sex, history of technology use, and gamer status) of the participants are as follows; Freshman (24.9%), Sophomore (21.2%), Junior 18.4%), Senior (21%), Senior+ (12.9%); Hispanic (18.3%), Non-Hispanic (80%); White (83.1%), Black (3.7%), Asian (10.7%), Hawaiian (1.4%), American Indian (3.9%); Male (66.8%), Female (30.5%), Other (.2%), Missing (2.5%); Historic User Yes (91.8%); Gamer Yes (25.4%). The average body mass index (BMI) of the participants was 24 (SD 4.2).
Technology use
When asked to indicate the type of technology they used regularly ( Tech Use ), the highest number of participants reported use of Smartphone ( n = 500) followed by Laptop ( n = 494). The number of participants indicating they used Desktops and Tablets were 277 and 182, respectively. Eighteen participants indicated they used another type of technology, which included gaming consoles ( n = 7), iPod® ( n = 3), and a FitBit® ( n = 1), with seven participants giving no response of the type of other technology they used. The Smartphone had the highest percent (64.0%) of participants indicating that this was the type of technology they used the most frequently ( Tech most ). This was followed by the Laptop (53.2%), Tablet (53.2%), and Desktop (46.4%).
Time on technology
Table 1 provides descriptions of how participants used their technology along with daily activity levels. Specifically, the percent of time they used the technology for different activities, how many hours per day they used the technology, how long they worked on the technology without a break, and their current stress levels. For those variables where participants gave a specific value (e.g., Hours per day on the Smartphone), outlying (or impossible, e.g., 25 h per day) values were (i.e., identified through descriptive analyses [ 29 ]; Ludbrook, 2008) were considered missing values in the analyses and the descriptive statistics are presented below.
The percent of time they used technology for different activities ( Tech for ) was School (M = 49.4%), followed by Recreation (M = 32.9%), Work (M = 9.2%), and Other (M = 1.4%). An RMANOVA found that there was a significant effect for percent of time with different activities, F (2.2, 1087.9) = 619.29, p < 0.001, \( {\upeta}_{\mathrm{p}}^2 \) = 0.55, and pairwise comparisons indicated that all of means were significantly different from each other (all p’s < 0.001).
Also seen in Table 1 is that participants’ average hours of technology use per day ( Hours tech ) were highest for the Smartphone (M = 4.1, SEM = 0.1) and Laptop (M = 3.8, SEM = 0.1). The average use hours were lower for Desktop (M = 1.1, SEM = 0.1), Tablet (M = 0.49, SEM = 0.1), and other technologies (M = 0.74, SEM = 0.3). An RMANOVA was done with the four most frequently used technologies (i.e., Smartphone, Laptop, Desktop, and Tablet) and these results indicated there were significant differences in participants hourly uses of the technology, F (2.3, 971.6) = 285.8, p < 0.001, \( {\upeta}_{\mathrm{p}}^2 \) = 0.48. Pairwise comparisons indicated that the means of all four technologies were significantly different from each of the other devices (all p’s < 0.035).
The number of minutes participants reported using their technology without a break ( Tech no break ) is presented in Table 1 . On average, the highest use without a break was highest for Laptop (M = 78.6, SEM = 3.0), followed by Desktop (M = 32.5 SEM = 2.3), Smartphone (M = 29.4, SEM = 2.0 respectively), and Other (M = 20.8, SEM = 8.5), and Tablet (M = 14.0, SEM = 1.3). RMANOVA indicates an effect of type of device on minutes without a break, F (2.6, 897.3) = 88.39, p < 0.001, \( {\upeta}_{\mathrm{p}}^2 \) = 0.21, and pairwise comparison found that all means were different at p < 0.001 except for Smartphone and Desktop ( p = 0.66).
Given the low percentage of time the participants reported using technology for “Other” and the low number of the participants responding to the “Other” categories, these variables will not be included in the regression models.
Table 2 provides the descriptive statistics for the participants’ reported activity levels (both the levels and amount of exercise), the percent of their days spent in different positions, and the amount of stress they have experienced recently. The participants reported doing a moderate number of exercise and average of 5 days a week for 74 min per day. Regarding vigorous exercise, they reported doing it 2.5 days per week for 50.6 min per day. The participants spent most of their day sitting (51.9%), followed by Walking (20.8%), Standing (12.8%), and Labor (7.3%). Finally, participants reported a mean stress level of 6.0 (with 1 being low and 10 being high).
Posture with technology
Table 3 provides participants responses regarding their posture when interacting with devices. The table provides a description of the posture, the image the participants saw, and descriptive statistics from the 9-point scale (treated continuously). Of the different postures, working on a laptop situated on a table in bent seated posture was found to be the most dominant (frequency and time in posture), followed by working on tablet situated on a table. The use of typical workstation, i.e., chair and table, were found to be the most common, followed by chair alone. Habitual postures, i.e., sitting/sleeping on a bed/sofa, were reported to be the least commonly adopted postures.
Table 4 reports findings from the PCA analysis of the 18 positions, the rotated component matrix, and the percent variance explained for each component (bolded component coefficients denote component loadings). The PCA resulted in 17 items loading onto six components. The item Desk Laptop loaded equally onto three components and was thus removed from the analysis. Further, the item Keyboard-Wrist Flexion loaded onto two items and was place in the component that it loaded onto were it most logically fit (with Keyboard Hands-No Ext).
The first component explained 22.74% of the variance, had four items, and all showed someone reclining in some way (Recline)—Bed laptop-back, Bed laptop-front, Chair ottoman-laptop, and Floor/bed recline tablet. The second component contained four items—Elbow desk laptop, Elbow desk tablet, Desk phone, tablet, and Chair/table phone—all showing someone either leaning or slouching and explained 9.7% of the variance (Lean/Slouch). The third component (9.19% of the variance) was comprised of two associated with ulnar deviation of the wrists either with the mouse or keyboard (Mouse-Ulnar deviation and Keyboard-Ulnar deviation). The fourth component explained 8.05% of the variability and contained three items that show someone sitting or standing at a desk (Desk Sit, Desk Stand, and Chair Sit). The fifth and six components both contained items associated with hand posture at the keyboard: one component (explaining 6.54% variance) showed the hands at the keyboard from above—one with no deviation and the other with radial deviation. The other component shows hands at the keyboard from the side—again one with no deviation and the other with extension (explaining 5.54% of the variance). These have been named Wrists – Radial deviation and Wrist – Flexion respectively.
Reported pain
The first section of Table 5 shows the descriptive statistics of participants’ responses to the items asking them to indicate their level of pain or discomfort on a 5-point scale (treated continuously). The second section of the Table 5 provides PCA analysis of the sixteen body parts for the items in three different components (see rotated component matrix). The first explained 40.6% of the variance and contained parts of the upper body not including the arms and hands (Upper Body)—Neck, Upper Back, Lower Back, Shoulders, Middle Back, and Eyes. The second component explained 10.3% of the variance and contained parts of the lower body (Lower Extremities)—Lower Legs, Thighs, Feet, Knees, Elbow, and Buttocks. The third component explained 7.5% of the variance and contained body parts in the distal upper extremities (Distal Upper Extremities)—Hands, Wrists, Lower Arms, and Upper Arms.
Predicting pain
Three stepwise multiple regressions SMR were conducted—one with each pain components as a criterion—to identify the variables that contributed to participants’ reported pain. The predictors used in the regression models were: Hours they used each technology (Tablet, Desktop, Laptop, Smartphone), Minutes they went without a break for each technology (Tablet, Desktop, Laptop, Smartphone), average score for each posture factor (Reclined, Lean/ Slouch, Wrists - Ulnar deviation, Desk posture, Wrists - Radial deviation, Wrists - Flexion), the percent of time they used any technology for different activities (Work, School, Recreation), Sex, BMI, the amount of stress they experienced, information about their exercise activities (Days per Week Moderate, Minutes per Day Moderate, Days per Week Vigorous, Minutes per Day Vigorous), and finally the percent of the day they spent Sitting, Standing, Walking, and in Labor.
Table 6 reports findings from the SMR for each of the different pain component criterion. For the Upper Extremity pain component, the final model had an adjusted variance explained (a.k.a., R 2 ) of .13, and two variables contributed to this model. The Percent Time of Technology Use for School explained 8% unique variance and was negatively related to Upper Extremity pain. The Reclined Position component explained 5% unique variance and was positively related to Upper Extremity pain.
For the Upper Body component, the final model had an adjusted R 2 of .28 indicating it explained 28% of the variance. As seen in Table 5 , there were four variables that contributed to this model, with the Amount of Stress contributing the most unique variance (16% unique variance). Lean/Slouch Posture Component contributed 7% unique variance and the Reclined Posture Component and Wrists Ulnar Deviation Component both contributed 3% unique variance to Upper Body pain.
The third pain component, Lower Body, had three variables that uniquely and positively contributed to the total overall variability in this model (total variance explained was 17%). For this model, Reclined Posture explained 8% unique variance and Minutes on the Tablet with no break explained 5% unique variance. The third variable was Amount of Stress and this explained 4% unique variance.
This study explored technology use among college students and its relation to their reported pain with a particular interest on the use of smartphones. Specifically examined was devices type, device usage, and posture and any connection between these variables and reported pain level among this population. School (49.4%) and recreation (32.9%) were the activities they used technology for the most and they used their smartphone (4.10) and laptop (3.77) for the highest number of hours. However, it was the laptop that they would use the longest without a break (78.6 min). The focus of this study was particularly on identifying whether, and if so, how the rapid and ubiquitous adoption of handheld devices (e.g., smartphones and tablets) by college students impacted their experience of pain. To explore this, we conducted stepwise multiple regressions—if our results had corresponded with previous results, we would expect to find that variables associated the type of device (specifically the use of tablets and laptops) would contribute the most variability to the reported pain in the upper body and upper extremity pain components. If our expectation that the pervasive use of smartphones was a concern, we would expect variables associated the use of smartphones to contribute the most variability to reported pain. However, we found neither. Indeed, tablet use (i.e., minutes of tablet use with no break) was the only predictor that contributed to reported pain and this was for the lower body component. Further, although the minutes of tablet use with no break significantly contributed to the model, it was only 5% of the overall explained variance of 17% for this model.
Our results instead show that posture components and stress more consistently contributing to students’ reported pain. This is particularly seen in the model explaining the Upper Body reported pain. This model explained 28% of the variance in reported pain and Stress explained 16% of that variance. Further, three different posture components—Lean/Slouch, Reclined, and Wrist Ulnar Deviation—explained the additional 13% (7, 3%, & 3% respectively). Further, in this study, for all three body groups (Upper extremity, Upper body, and Lower body), the Reclined Posture Component contributed significantly to reported pain—with more time in these postures resulting in more pain (see Table 6 ). Students generally experienced more pain in the Upper Body regions (see Table 5 ) than the other two components.
There were interesting differences between the predictors of reported pain for three body groups. For instance, for the Distal Upper Extremities (hands, wrists, upper & lower arms), the Percent Time Use/School was negatively related to pain, indicating that the more they used any device for School, the less pain they experience in this body region. This result is difficult to interpret but could be due to generally lower pain ratings in this body region. It could also indicate that this measure is not sensitive for these body regions, particularly in this population (i.e., primarily young, and healthy participants) particularly given that previous studies using the Body Discomfort Diagrams have primarily focused on working adults [ 26 , 27 , 28 ].
Another interesting finding was the contribution of stress to the variability in reported pain for the Upper Body (Neck, Upper/Lower/Middle back, Shoulder, and Eyes). As seen in Table 6 , Stress accounted for over half of the variability explained by the model (16% out of 28%), while posture components (i.e., Lean/Slouch, Reclined, and Wrist Ulnar Deviation) accounted for the rest. That stress would be related to pain in the Upper Body is not surprising given that many tend to tighten their shoulders when stressed and is consistent with the literature on findings related to neck and shoulder pain prevalence among college students [ 30 , 31 , 32 , 33 , 34 ]. However, the fact that stress contributes to the Lower Body as well suggests that in this population, stress management maybe as important for mitigating pain as ergonomic interventions that impact posture and device use. Indeed, given that their average stress level was 6.1 on a scale with 0 = No Stress and 10 = Extreme Stress, the amount of stress these students are experiencing combined with less experience managing stress could be an area for future investigation.
Generally, college students still reported significant portions of their day in traditional desk and chair work positions with laptops and desktops. However, the use of alternate postures such as floor, bed and chair without desk were also very common along with the multitude of postures used with smartphones that are less constrained due to their weight, size, and heat output. The result of these postures and durations with respect to reported discomfort was concentrated in the neck, back, and eyes. Albeit low levels of relative discomfort as is often typical with a sample population of young & mostly healthy adults such as that reviewed by Towne et al. in a previous paper on this same college student cohort that included a look at sex, sleep and race/ethnicity [ 35 ]. For future research, “floor/bed phone” as an option should be considered as students pointed out this was missing and certainly a common activity for them.
Although it is surprising that the number of hours using a device did not contribute at all to the reported pain and that the number of minutes without a break only contributed to one component for one device (the tablet), these results are somewhat promising for ergonomic interventions with this population. The results essentially indicate that, regardless of device, ergonomic interventions that focus on improving posture and facilitating stress management may reduce the likelihood of pain. Although clearly the reported pain is not high on average for this group, it does exist and that is concerning in and of itself given that these are young and relatively healthy people. Further, as mentioned previously, their stress level is also somewhat concerning. Therefore, these results suggest that posture and stress management interventions may be effective regardless of device and thus, as new technologies are introduced and adopted, the intervention methodologies may not need to be remarkably different.
In general, individuals who spent more time in sedentary postures reported more pain and would therefore benefit from behavioral modifications that encourage different postures throughout the day. This is timely for college students, given major universities have transitioned, in many cases, to remote learning since COVID-19. This introduction of significant shifts in the students’ learning environment, including the students’ ability to access optimal ergonomic set ups (e.g., devices and related equipment), may lead to more lasting technology-use behaviors. For those already in office environments in major industries that have shifted to remote work for months, potentially longer, this is also an important consideration. For example, it is unlikely that all will have distinct and necessary office space in their home that might help facilitate a more ergonomic set up. Furthermore, having appropriate equipment (e.g., ergonomic keyboards, monitors) to utilize may also take additional resources unavailable for many. Thus, the availability of proper equipment and space for both college students, but also those already in industries previously relying on office workspaces prior to COVID-19 clearly presents a challenge. Anecdotally speaking, since COVID-19, we have seen an increase of both students and adults working from home-based workstations such as couches, beds, and kitchen counters. If this is to become more common than before COVID-19, additional work may need to be done with remote workers to evaluate risk levels and then if needed, provide evidence-based interventions. The potential cost of resources needed to facilitate an ergonomic-like environment within one’s home, potentially including the added cost of additional square footage (especially for already space-restricted apartment homes) and additional resource cost of purchasing equipment, may lead to disparities across income, a known social and structural determinant of health inequities [ 36 ]. By extension, the known health issues stemming from inadequate ergonomic set ups and device interactions may disproportionately affect those already at-risk of health inequities. Indeed, an algorithmic approach Pierson et al [ 37 ] attempted to explain the pain disparities in underserved populations and how they might apply to improved treatment approaches for clinicians. However, this variability has not been researched with respect to pain disparities associated with ergonomic issues or in college students. Therefore, studies exploring these potential disparities are recommended for future research. Furthermore, employers who either do not recognize the importance of ergonomics, during temporary remote working conditions, or those who cannot support the additional costs involved in supplying appropriate office-like environments during remote work under the pandemic may also contribute to these potential disparities.
With a tech-focused society and growing rates of technology use (smartphones and laptops), efforts are needed to ensure pain is deferred or delayed until later years if the productivity of this workforce is to be preserved. Our team believes that although technology induced inactivity has been part of the struggle for information age workers to move and remain healthy, it is also technology that is most likely to provide smart solutions derived from “precision ergonomics” that will supplement the population level solutions of one approach for all that have been most common up until now. This concept is discussed as “agile science” by Patrick et al. in their discussion of technology and health finally coming together for the good of people on a large scale [ 38 ].
Limitations
One of the major limitations of this study is the use of surveys to assess the constructs of interest. Self-report data may not be as reliable as observational data and at the same time, they may allow for evaluation of constructs with a larger sample. The accuracy of self-reports is inherently challenged due to recall bias and social desirability. For example, either of these could result in some respondents reporting higher or lower levels of screen time or time in specific postures. That said, given the confidential nature of survey responses in this online survey it may be that respondents were more likely to report more accurate estimates than one might otherwise. For example, if it had been an in-person survey where one’s instructors or advisors were aware of their responses tied to their names, this could increase the potential for bias associated with responses tied to social desirability. Thus, the confidential nature of the survey was a major strength in this regard. Another limitation is associated with the sample. Given that the data were taken from a single, albeit large, STEM-focused department in one of the largest universities in the US, it is not likely to be generalizable to the entire student body. However, this was not an expectation, given variation in demographic composition within and between university settings. Even with these limitations, the findings have important implications regarding the importance of understanding ergonomic risk factors in this population.
As we seek to further enhance capabilities of hand-held devices (primarily smartphones), we need to understand the increase in exposure to device use and the transition to unhealthy postures that they permit via macro design features. Further, the recent addition of streaming services has added to the overall viewing time by handheld device users. If the same device is used for pleasure viewing like binge watching television shows and completing homework and other assignments, the human interface for control and display will continue to grow in importance to our health outcomes. Given that the goal is to prevent musculoskeletal injuries and illness among students and adults using these devices, our findings suggest we many need to reconsider our approach. As noted above our team believes, as others have tested, that the use of these same devices to prompt, encourage, and nudge proper behaviors, including breaks from their use and proper ergonomics, are both feasible and the most likely path toward better health outcomes for students [ 39 , 40 ]. The understanding we provide here should help device designers and programmers better plan for user exposure to their software, apps, accessories, tools, cases and devices within the heavy use crowd of college age students.
Availability of data and materials
The data that support the findings of this study are protected in accordance with the Texas A&M University Institutional Review Board for the current study, and so are not publicly available.
Bröhl C, Rasche P, Jablonski J, Theis S, Wille M, Mertens A. Desktop PC, tablet PC, or smartphone? An analysis of use preferences in daily activities for different technology generations of a worldwide sample. In: Paper presented at: International Conference on Human Aspects of IT for the Aged Population; 2018.
Google Scholar
Katz JN, Amick BC, Carroll BB, Hollis C, Fossel AH, Coley CM. Prevalence of upper extremity musculoskeletal disorders in college students. Am J Med. 2000;109(7):586–8. https://doi.org/10.1016/S0002-9343(00)00538-6 .
Article CAS PubMed Google Scholar
Bowman PJ, Braswell KD, Cohen JR, Funke JL, Landon HL, Martinez PI, et al. Benefits of laptop computer ergonomics education to graduate students. Open J Therapy Rehabil. 2014;2014(01):25–32. https://doi.org/10.4236/ojtr.2014.21006 .
Article Google Scholar
Bubric K, Hedge A. Differential patterns of laptop use and associated musculoskeletal discomfort in male and female college students. Work. 2016;55(3):663–71. https://doi.org/10.3233/WOR-162419 .
Article PubMed Google Scholar
Szucs KA, Cicuto K, Rakow M. A comparison of upper body and limb postures across technology and handheld device use in college students. J Phys Ther Sci. 2018;30(10):1293–300. https://doi.org/10.1589/jpts.30.1293 .
Article PubMed PubMed Central Google Scholar
Dockrell S, Bennett K, Culleton-Quinn E. Computer use and musculoskeletal symptoms among undergraduate university students. Comput Educ. 2015;85:102–9. https://doi.org/10.1016/j.compedu.2015.02.001 .
Werth A, Babski-Reeves K. Effects of portable computing devices on posture, muscle activation levels and efficiency. Appl Ergon. 2014;45(6):1603–9. https://doi.org/10.1016/j.apergo.2014.05.008 .
Woo EH, White P, Lai CW. Musculoskeletal impact of the use of various types of electronic devices on university students in Hong Kong: an evaluation by means of self-reported questionnaire. Man Ther. 2016;26:47–53. https://doi.org/10.1016/j.math.2016.07.004 .
Kim H-J, Min J-Y, Kim H-J, Min K-B. Accident risk associated with smartphone addiction: a study on university students in Korea. J Behav Addict. 2017;6(4):699–707. https://doi.org/10.1556/2006.6.2017.070 .
Pew Research Center. Mobile Fact Sheet. 2021. Available at: https://www.pewresearch.org/internet/fact-sheet/mobile/ . Accessed May 2021.
Penkala S, El-Debal H, Coxon K. Work-related musculoskeletal problems related to laboratory training in university medical science students: a cross sectional survey. BMC Public Health. 2018;18(1):1–9. https://doi.org/10.1186/s12889-018-6125-y .
Driscoll T, Jacklyn G, Orchard J, Passmore E, Vos T, Freedman G, et al. The global burden of occupationally related low back pain: estimates from the global burden of disease 2010 study. Ann Rheum Dis. 2014;73(6):975–81. https://doi.org/10.1136/annrheumdis-2013-204631 .
Cole DC, Hogg-Johnson S, Manno M, Ibrahim S, Wells RP, Ferrier SE. Reducing musculoskeletal burden through ergonomic program implementation in a large newspaper. Int Arch Occup Environ Health. 2006;80(2):98–108. https://doi.org/10.1007/s00420-006-0107-6 .
Hoe VC, Urquhart DM, Kelsall HL, Zamri EN, Sim MR. Ergonomic interventions for preventing work-related musculoskeletal disorders of the upper limb and neck among office workers. Cochrane Database Syst Rev. 2018;10(10). https://doi.org/10.1002/14651858.CD008570.pub3 .
Hughes LE, Babski-Reeves K, Smith-Jackson T. Effects of psychosocial and individual factors on physiological risk factors for upper extremity musculoskeletal disorders while typing. Ergonomics. 2007;50(2):261–74. https://doi.org/10.1080/00140130601049378 .
Osofundiya O, Benden ME, Dowdy D, Mehta RK. Obesity-specific neural cost of maintaining gait performance under complex conditions in community-dwelling older adults. Clin Biomech. 2016;35:42–8. https://doi.org/10.1016/j.clinbiomech.2016.03.011 .
Sauter SL, Swanson NG. An ecological model of musculoskeletal disorders in office work. Beyond Biomechanics. 1996:3–21. https://doi.org/10.1201/9781482272680 .
Mehta RK, Nussbaum MA, Agnew MJ. Muscle-and task-dependent responses to concurrent physical and mental workload during intermittent static work. Ergonomics. 2012;55(10):1166–79. https://doi.org/10.1080/00140139.2012.703695 .
Mehta RK, Agnew MJ. Exertion-dependent effects of physical and mental workload on physiological outcomes and task performance. IIE Transactions Occup Ergonomics Hum Factors. 2013;1(1):3–15. https://doi.org/10.1080/21577323.2011.632488 .
Mehta RK, Agnew MJ. Influence of mental workload on muscle endurance, fatigue, and recovery during intermittent static work. Eur J Appl Physiol. 2012;112(8):2891–902. https://doi.org/10.1007/s00421-011-2264-x .
Lundberg U. Psychophysiology of work: stress, gender, endocrine response, and work-related upper extremity disorders. Am J Ind Med. 2002;41(5):383–92. https://doi.org/10.1002/ajim.10038 .
LINE.17QQ.COM. Available at: https://line.17qq.com/articles/wgwqnmmsy.html . Accessed May 2021.
“Posture Support in a Changing Workplace.” Steelcase. Steelcase, November 19, 2018. https://www.steelcase.com/research/articles/topics/wellbeing/posture-support-changing-workplace/ .
Iconfinder. Available at: https://www.iconfinder.com/icons/2043807/body_computer_incorrect_postures_sitting_working_wrong_icon . Accessed May 2021.
Cameron JA. Assessing work-related body-part discomfort: current strategies and a behaviorally oriented assessment tool. Int J Ind Ergon. 1996;18(5–6):389–98. https://doi.org/10.1016/0169-8141(95)00101-8 .
Åhsberg E. Dimensions of fatigue in different working populations. Scand J Psychol. 2000;41(3):231–41. https://doi.org/10.1111/1467-9450.00192 .
Kee D, Karwowski W. The boundaries for joint angles of isocomfort for sitting and standing males based on perceived comfort of static joint postures. Ergonomics. 2001;44(6):614–48. https://doi.org/10.1080/00140130110038044 .
Li KW. Ergonomic design and evaluation of wire-tying hand tools. Int J Ind Ergon. 2002;30(3):149–61. https://doi.org/10.1016/S0169-8141(02)00097-5 .
Ludbrook J. Outlying observations and missing values: how should they be handled? Clin Exp Pharmacol Physiol. 2008;35(5–6):670–8. https://doi.org/10.1111/j.1440-1681.2007.04860.x .
Haroon H, Mehmood S, Imtiaz F, Ali SA, Sarfraz M. Musculoskeletal pain and its associated risk factors among medical students of a public sector University in Karachi, Pakistan. J Pak Med Assoc. 2018;68(4):682–8.
PubMed Google Scholar
Bruls VE, Bastiaenen CH, de Bie RA. Non-traumatic arm, neck and shoulder complaints: prevalence, course and prognosis in a Dutch university population. BMC Musculoskelet Disord. 2013;14(1):8. https://doi.org/10.1186/1471-2474-14-8 .
Jenkins M, Menéndez CC, Amick Iii BC, Tullar J, Hupert N, Robertson MM, et al. Undergraduate college students' upper extremity symptoms and functional limitations related to computer use: a replication study. Work. 2007;28(3):231–8.
Schlossberg EB, Morrow S, Llosa AE, Mamary E, Dietrich P, Rempel DM. Upper extremity pain and computer use among engineering graduate students. Am J Ind Med. 2004;46(3):297–303. https://doi.org/10.1002/ajim.20071 .
Obembe AO, Johnson OE, Tanimowo TO, Onigbinde AT, Emechete AA. Musculoskeletal pain among undergraduate laptop users in a Nigerian University. J Back Musculoskelet Rehabil. 2013;26(4):389–95. https://doi.org/10.3233/BMR-130397 .
Towne SD, Ory MG, Smith ML, Peres SC, Pickens AW, Mehta RK, et al. Accessing physical activity among young adults attending a university: the role of sex, race/ethnicity, technology use, and sleep. BMC Public Health. 2017;17(1):721. https://doi.org/10.1186/s12889-017-4757-y .
Solar O, Irwin A. A conceptual framework for action on the social determinants of health. 2007.
Pierson E, Cutler DM, Leskovec J, Mullainathan S, Obermeyer Z. An algorithmic approach to reducing unexplained pain disparities in underserved populations. Nat Med. 2021;27(1):136–40. https://doi.org/10.1038/s41591-020-01192-7 .
Patrick K, Hekler EB, Estrin D, Mohr DC, Riper H, Crane D, et al. The pace of technologic change: implications for digital health behavior intervention research. Elsevier; 2016.
Aust LA, Bockman SA, Hermansen-Kobulnicky CJ. One click away: pilot study of the perceived academic impact of screen time among pharmacy students. Curr Pharm Teach Learn. 2019;11(6):565–70. https://doi.org/10.1016/j.cptl.2019.02.019 .
Cain J. Effectiveness of Issuing Well-being Challenges to Nudge Pharmacy Students to Adopt Well-being Protective Behaviors. Am J Pharm Educ. 2020;84(8):1150–5.
Download references
Acknowledgements
This research received no external funding.
Author information
Authors and affiliations.
ErgoCenter, Department of Environmental and Occupational Health, School of Public Health, Texas A&M University, College Station, TX, USA
Mark Benden, Ranjana Mehta, Adam Pickens, Brett Harp & S. Camille Peres
Wm Michael Barnes ‘64 Department of Industrial and Systems Engineering, Texas A&M University, College Station, TX, USA
Mark Benden, Ranjana Mehta & S. Camille Peres
Department of Environmental and Occupational Health, School of Public Health, Texas A&M University, College Station, TX, 77843, USA
Mark Benden, Ranjana Mehta, Adam Pickens, Brett Harp, Matthew Lee Smith, Samuel D. Towne Jr & S. Camille Peres
Center for Population Health and Aging, Texas A&M University, College Station, TX, USA
Matthew Lee Smith & Samuel D. Towne Jr
School of Global Health Management and Informatics, University of Central Florida, 4364 Scorpious Street, HPA II, Orlando, FL, 32816-2205, USA
Samuel D. Towne Jr
Disability, Aging, and Technology Cluster, University of Central Florida, Orlando, Florida, 32816-2205, USA
Southwest Rural Health Research Center, Texas A&M University, College Station, TX, 77843, USA
You can also search for this author in PubMed Google Scholar
Contributions
All authors together conceived and developed the study under the lead of MB. SCP, AP, RM, MS & SDT developed the questions, survey methods and participated in the analysis and writing. MB led the first draft with BH and the entire team discussed results and contributed to the final manuscript. All authors read and approved the final draft of the manuscript.
Corresponding author
Correspondence to Samuel D. Towne Jr .
Ethics declarations
Ethics approval and consent to participate.
The study was approved by the Texas A&M University Institutional Review Board and all methods were performed in accordance with the relevant guidelines and regulations. Electronic informed consent was gained from all participants in accordance with the IRB [ 35 ]. Written informed consent was not applicable, given responses were gained via electronic survey and the survey was completed as part of administrative procedures within the unit [ 35 ].
Consent for publication
Competing interests.
The authors declare that the research was conducted in the absence of any commercial or financial relationships that could be construed as a potential conflict of interest.
Additional information
Publisher’s note.
Springer Nature remains neutral with regard to jurisdictional claims in published maps and institutional affiliations.
Rights and permissions
Open Access This article is licensed under a Creative Commons Attribution 4.0 International License, which permits use, sharing, adaptation, distribution and reproduction in any medium or format, as long as you give appropriate credit to the original author(s) and the source, provide a link to the Creative Commons licence, and indicate if changes were made. The images or other third party material in this article are included in the article's Creative Commons licence, unless indicated otherwise in a credit line to the material. If material is not included in the article's Creative Commons licence and your intended use is not permitted by statutory regulation or exceeds the permitted use, you will need to obtain permission directly from the copyright holder. To view a copy of this licence, visit http://creativecommons.org/licenses/by/4.0/ . The Creative Commons Public Domain Dedication waiver ( http://creativecommons.org/publicdomain/zero/1.0/ ) applies to the data made available in this article, unless otherwise stated in a credit line to the data.
Reprints and permissions
About this article
Cite this article.
Benden, M., Mehta, R., Pickens, A. et al. Health-related consequences of the type and utilization rates of electronic devices by college students. BMC Public Health 21 , 1970 (2021). https://doi.org/10.1186/s12889-021-11975-3
Download citation
Received : 13 November 2020
Accepted : 06 October 2021
Published : 01 November 2021
DOI : https://doi.org/10.1186/s12889-021-11975-3
Share this article
Anyone you share the following link with will be able to read this content:
Sorry, a shareable link is not currently available for this article.
Provided by the Springer Nature SharedIt content-sharing initiative
BMC Public Health
ISSN: 1471-2458
- General enquiries: [email protected]
Academia.edu no longer supports Internet Explorer.
To browse Academia.edu and the wider internet faster and more securely, please take a few seconds to upgrade your browser .
Enter the email address you signed up with and we'll email you a reset link.
- We're Hiring!
- Help Center
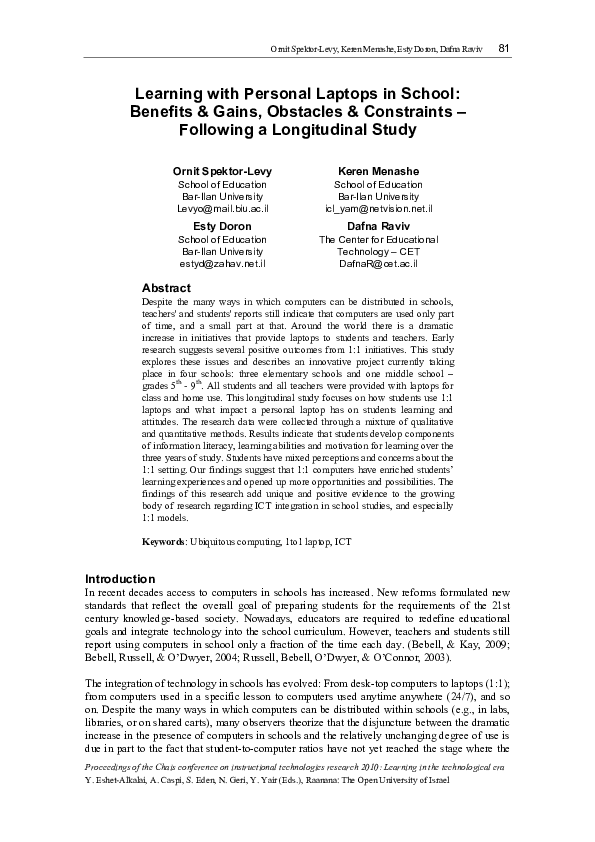
Download Free PDF
Learning with Personal Laptops in School: Benefits & Gains, Obstacles & Constraints–Following a Longitudinal Study

telem-pub.openu.ac.il
Related papers
Journal of Computer …, 2007
ONE-TO-ONE LAPTOPS IN A PUBLIC SECONDARY SCHOOL: STUDENTS' USAGE AND THE IMPACT ON ACHIEVEMENT Computer technology has become ubiquitous in the lives of today's learners. Schools and districts are responding to the rise of technology with a push to expand access to computers for all students in the form of one-to-one laptop initiatives. While such initiatives have been shown to help students develop computer and technology skills, their impact on achievement has been more difficult to ascertain. Differences in implementation levels between different schools, teachers, and students, have made the relationship between laptop use and achievement difficult to measure. This study was designed to provide more information regarding the relationship between different types and frequencies of laptop usage and student reading achievement, as well as the barriers and opportunities which limit or promote the use of the laptops by students and teachers. This study used a mixed methods de...
Journal of Educational Computing Research, 2004
This study compares teaching and learning activities in 4th and 5th grade classrooms that were permanently equipped with one laptop for each student and classrooms that share a cart of laptops that create a 1:1 laptop environment on a temporary basis. The study originated from a question posed to us by Andover Public Schools (MA): “How does teaching and learning differ when upper elementary students (4th and 5th graders) are provided with their own laptop computers?” In response to this question, we undertook an intensive two month study that employed a mixed methodology that included student surveys, student drawings, teacher interviews, and 56 structured classroom observations. The findings summarized in this article provide evidence of several differences in teaching and learning activities between the two settings. Classrooms that were fully equipped with 1:1 laptops showed more technology use across the curriculum, more use of technology at home for academic purposes, less larg...
Interdisciplinary Journal of e-Skills and Lifelong Learning
Concepts, Methodologies, Tools, and Applications
This chapter describes some of the findings from a QUAL + QUAN concurrent mixed method study that examined the first-year implementation of a one-to-one (1:1) laptop initiative in a suburban, independent, co-educational middle and high school in the United States. Overall, the 1:1 laptop implementation was viewed as a positive learning experience for students, teachers, staff, and the school administration. Nevertheless, several problems developed over the course of implementation. These were: technical problems, issues with student distraction and off-task behavior, inappropriate uses of technology, as well as challenges to pedagogical and classroom management, and inclusion of teachers’ voices in implementation decisions. They are introduced in addition to several possible solutions. The chapter closes with suggestions for future research, such as the need to research 2:1 and 3:1 mobile technology initiatives, which are sure to become a part of the 21st century teaching and learni...
Educational Technology Research and Development, 2003
Medieval Encounters, 2024
This article explores unions between elite Muslim men and elite non-Muslim women from the conquered populations during the seventh to ninth centuries CE. It considers cases from a range of geographic settings, including the Iberian Peninsula, North Africa, the Fertile Crescent, and Iran. It examines these unions in their immediate historical contexts as well as literary artifacts of much later periods. With respect to the former, it argues Muslim conquerors often used elite non-Muslim women to cement their alliances with indigenous elites and as instruments to humiliate and abase these elites. With respect to the latter, it argues that stories of aristocratic non-Muslim women constitute a neglected but important feature of conquest narratives and they show how elite non-Muslim lineage remained prized among Muslims long after the conquests were over. Finally, as the article argues, the phenomenon demonstrates that many in early Muslim society considered maternal lineage to be very important, even if social standing was technically based mainly on the father.
Islamic Thought and the Art of Translation. Texts and Studies in Honor of William C. Chittick and Sachiko Murata (Islamic History and Civilization, 202. Ed. Mohammed Rustom), 2023
In his "Kitāb al-Isfār ʿa natāʾij al-asfār", Muḥammad and Adam are presented by Ibn ʿArabī as “respectively father and son to one another”. These unusual ascriptions contrast with the classical qualifications of Adam as the father of mankind and Muḥammad as the seal of the prophets, and clearly indicate an intricate correlation between the two figures that exceeds their chronological succession. As I have already shown elsewhere, the notions of “relation” (nisba) and “correlation” (munāsaba) are absolutely central to the ontology of Ibn ʿArabī. In this study, we will see through the example of the relation between Adam and Muḥammad, that this correlative approach seems also crucial to understand the intricacies of Ibn ʿArabī’s prophetology. Rather than a comprehensive survey and a thorough analysis of the question, this paper will propose some introductory notes to a study that would deserve a much longer and methodological research.
TEFL Journal, 2023
This study provided an overview of research that has investigated the role of (direct vs. indirect) written corrective feedback (WCF) in enhancing writing accuracy of Intermediate learners of Navid English Institute in Shiraz. This study is quantitative and experimental survey and then accuracy in the used two functions of English article system (referential indefinite ‘a’, ‘an’ and referential definite ‘the’) was measured during eight weeks by means of pre-test and post-test sixty students that include (20 control group,20 experimental group,20 experimental group) participated in the study which sought to investigated whether what kind of teacher written corrective feedback will be the most effective in writing accuracy then participants received teacher WCF on ten essay under three treatment conditions: Group (1)control group did not receive WCF on specific grammar errors; group (2) received direct WCF; group (3) received indirect WCF. Then at the end of the term, it investigated which type of written corrective feedback will be the most effective in writing accuracy of definite and indefinite article and does accuracy of two functions of the English article vary during eight weeks as results of WCF or not.
Music and Institutions in Fascist Italy, 2024
Gallia, 2024
Prizren Social Science Journal, 2019
Jüdische Geschichte & Kultur. Magazin des Dubnow-Instituts, 2023
Perspectivas do discurso jurídico: argumentação, hermenêutica e cultura, 2015
Proceedings of Indian History Congress, 2009
Disputation Literature in the Near East and Beyond, 2020
Mataram Grafika, 2016
npj Primary Care Respiratory Medicine, 2022
Jurnal Material Kedokteran Gigi, 2020
Revista Brasileira de Pós-Graduação, 2014
Educational Research for Policy and Practice, 2018
The European respiratory journal, 2015
American Journal of Human Biology, 2015
Primus , 2022
Environmental science and pollution research international, 2018
Related topics
- We're Hiring!
- Help Center
- Find new research papers in:
- Health Sciences
- Earth Sciences
- Cognitive Science
- Mathematics
- Computer Science
- Academia ©2024
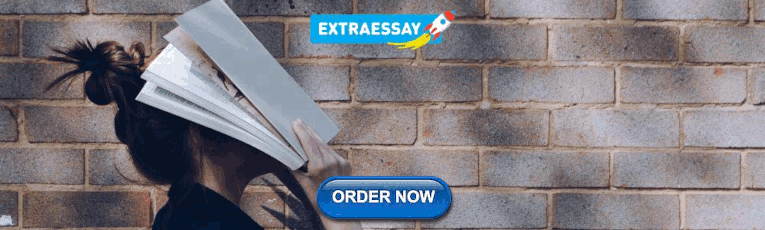
IMAGES
VIDEO
COMMENTS
laptops and/or smartphones into a lecture theatre, there was no significant difference in academic performance over the years of studies. The findings have linked student multitasking processes in
The purpose of the following study was to develop a research-based, theoretically grounded scale to assess student use of laptops inside and outside higher education classrooms. The Laptop Use Scale addressed four key areas: in-class academic use, in-class non-academic use, outside of class academic use, and outside of class non-academic use.
This study proposes a theoretical model on the influence of several computer factors on the academic achievement of high school students. The results, in general, empirically support the literature in similar findings. The proposed conceptual model explains 31.1% of academic achievement.
This study provides an in-depth description of adult learners’ perceptions and experiences using laptop computers in higher education institutions. The study also examines the influence on human beings’ cognitive activity by using laptops.
The introduction of new technology-assisted learning tools such as mobile devices, smartboards, MOOCs, tablets, laptops, simulations, dynamic visualisations, and virtual laboratories have altered education in schools and institutions.
Participants ranked smartphones as their most frequently used technology (64.0%), followed by laptops and tablets (both 53.2%), and desktop computers (46.4%). Time spent using smartphones averaged over 4.4 h per day.
This review paper discusses various research studies that focus on how laptop computers affect student learning, how laptops affect instruction, teachers' perceptions about using laptop computers, and specific school districts that have implemented laptop computers into their curriculum.
Laptop learning: A comparison of teaching and learning in upper elementary classrooms equipped with shared carts of laptops and permanent 1:1 laptops. Journal of Educational Computing Research , 30(4), 313–330.
In this Occasional Paper, we present the results of a CRLT research study that examined student perceptions of how laptops affect attentiveness, engagement, and learning, and we suggest guidelines for using laptops and other mobile devices effectively in the classroom.
This chapter describes some of the findings from a QUAL + QUAN concurrent mixed method study that examined the first-year implementation of a one-to-one (1:1) laptop initiative in a suburban, independent, co-educational middle and high school in the United States.