What Is the Role of Biostatistics in Public Health? Posted August 25, 2021 in Public Health
By Dr. Bojana Beric-Stojsic
Biostatistics are applied statistics in biological and medical sciences for public health practice. Based on the data and models they provide, epidemiology — the study of the causation, spread and control of disease across time and space — gives us information about health status, morbidity and mortality in human populations. Together, biostats and epidemiology form the foundation of public health and guide all of our other decisions related to the prevention and control of diseases — both communicable and non-communicable — occurring in populations locally and globally.
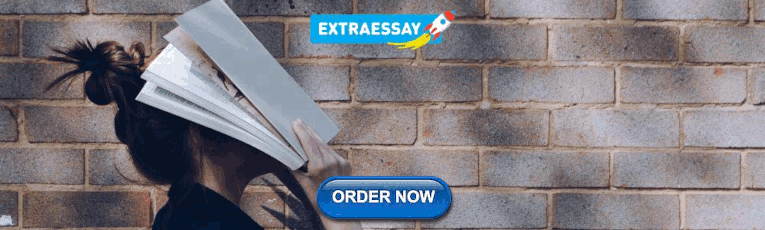
How Biostatistics In Public Health Inform the Guidance of Experts During Disease Outbreaks
In essence, the statistical method is used to explain and predict some of the health outcomes and the direction of epidemics and pandemics, and it definitely influences decision-makers in public health. Those who are working on, proposing and advising the mitigation strategies in response to contagions use biostatistics data and results to guide public health and other healthcare practitioners on how to go about controlling these diseases. Two good examples would be studies that were designed to determine the effectiveness of mask-wearing as a measure to prevent the spread of certain viruses, as well as clinical trials designed to test the effectiveness and efficacy of vaccines, including those developed for the prevention of SARS-CoV-2.
Data Collection and Interpretation
When we talk about biostatistics in public health or any other application, the most important thing to keep in mind is that they are good only if the study or the design for data collection is appropriate and carefully planned. Then, we can extrapolate results and further interpret them.
Most research studies are based on samples, which represent populations. Selecting that sample to be as representative as possible of the population and the condition that we intend to study is very important for accurate generalization of the results and for the design of appropriate interventions. On the other hand, epidemiologic measures, such as morbidity, mortality, or incidence and prevalence rates, are based on every case and are used to assess and inform us about the health status of a population. An example of a reliable and sustainable source of local data about health and its determinants is the County Health Rankings & Roadmaps program , developed through a collaboration between the Robert Wood Johnson Foundation and the University of Wisconsin Population Health Institute.
All research in public health and health sciences is and should be based on scientific methodology and planning. How do we plan and structure the data collection, data analysis, and interpretation of the findings, as well as the application of those findings? Biostats are truly valuable in emergencies, when connecting the reality of panic and uncertainty with the strength of the scientific method to provide solutions.
Become a Leader in Public Health
Learn to read, interpret and provide conclusions as solid evidence to support recommended changes in policy or new policies with FDU's Master of Public Health online program.
In the case of the COVID-19 pandemic, our initial public health preparedness strategy and response planning were not executed quickly and efficiently. A public health surveillance system with tracking of test-positive and disease cases, hospitalizations, and deaths should have been ready for implementation, based on the history of pandemics in the not-so-distant past. The Health and Human Services Pandemic Influenza Plan , developed in 2005 and revised in 2006, 2009, and, most recently, in 2017, should have been utilized without issues. Also, after the Anthrax attack in 2001, the emergency preparedness strategy with its system of communication was improved and expanded through the development of a functioning LINCS (Learning Information Network Communication System), which connected the state and local Departments of Health in New Jersey. This should have been expanded to other states years ago, which would have made us better prepared for COVID-19, and should have been utilized more effectively during the pandemic.
A coherent system was needed to allow for valuable and appropriate decisions and predictions; provide data for epidemiologists to investigate and learn about the modes of transmission, incubation period, severity of the disease and a variety of symptomatological and clinical manifestations; and inform public health and health care practitioners with evidence. But at that time, the data were unavailable, and the surveillance system was silent.
In the early stages, it was a real struggle, as a result of the uncoordinated public health system and surveillance. It took some time for coordination and agreement between federal, state and local governments. That said, there are some good examples of a quick “recovery” from the inevitable shortcomings, such as the collaboration between two local governmental agencies in New York City — the Department of Health & Mental Hygiene and the IT Department of the Office of Chief Medical Examiner — as they quickly established a surveillance system with data collection to provide the mortality rates and inform the practice.
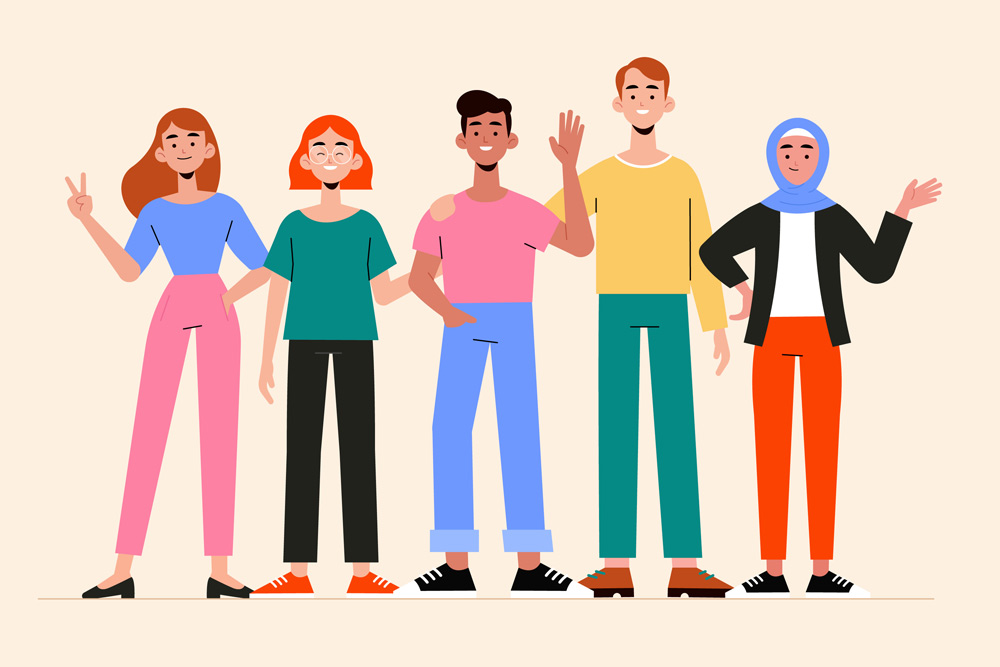
The National Healthcare Problem
The US public health and healthcare system, as we call it, is not functioning as one. A system consists of elements that are connected and working in synergy. The situation in this country at the beginning of the pandemic was not illustrative of that. It was so unfortunate that there was no effective connection between federal, state and local agencies, as that would have made this whole process of controlling the pandemic much smoother and produced a better outcome. A lot of responsibilities relative to public health were delegated to the states from the federal government without adequate support, creating a division between the national and state emergency responses, which became a huge problem in the initial weeks and months of this health crisis.
After the SARS pandemic, many of those in government had good intentions. A plan was developed in 2005, and the funding was provided to establish those links and that communication between the national, state and municipal governments; but sadly, the funding was eliminated without proper justification. When there’s no funding, usually there is no adequate provision of services, so the plan and strategies will not be implemented. There was and still is a disconnect, but the solution — as I see it, and as I teach my students in our Foundations and Issues of Public Health course — is to build those missing links and connect all levels of government, while establishing partnerships between public and private institutions, including civil society, non-profit and academic organizations.
One specific problem caused by this disconnect is that, at the beginning of the COVID-19 pandemic, private pharmaceutical companies started producing test kits, but they were not made available to governmental agencies to distribute to people. Developing strong public-private partnerships would solve this issue and make our system much more of a system for all people, which is something that government guarantees: care for all citizens.
A broader example is that we don’t often think about how much social and economic conditions, urban design and other “non-health” determinants are closely related to health outcomes, creating barriers for access to services in certain populations, especially those that are vulnerable and need them the most.
Biostatistical Lessons From the COVID-19 Pandemic
What we can learn from surveillance, data collection and vaccine development.
Biostatistics provide us with scientific, historical data and results; they give us direction for the future. If we examine certain diseases or trends in diseases, biostatistics should be guiding us on the right path. But the data have to be carefully collected and accurately interpreted to give biostatistics the power to support the symbiotic work of public health and healthcare professionals; otherwise, the biostats may be misleading, not useful and, in certain cases, even harmful.
To be effective, public health and healthcare systems need to work cooperatively, while cautiously recognizing that there may be some limitations when relying solely on surveillance data. The surveillance must accurately reflect the situation in a community in order to allow for appropriate comparisons between different communities, so that conclusions can be drawn and recommendations made. The reason for some lack of coordination in public health messaging at the national level last year could have been an uneven availability or utilization of testing in different localities, which may have caused inappropriate comparisons of data in those areas.
Another challenge that COVID-19 presented to scientists and practitioners was the wide variety of signs and symptoms that the SARS-CoV-2 virus causes in people. The timing of testing and onset of symptoms in patients is a very important factor in collecting accurate data to provide meaningful mitigation guidelines and care, which proved to be very challenging initially. As the pandemic progressed, this was better understood, and more accurate information was generated to guide public health guidelines and healthcare services.
Another positive note, which demonstrates the true value of biostatistics in this battle with COVID-19, is the success in developing new types of vaccines. This breakthrough is saving lives and providing us with facts about the effectiveness and efficacy of those vaccines in humans, while continuing to inform us about the progression of the virus in vaccinated vs. unvaccinated groups. It is encouraging information for public health and healthcare professionals and a source of comfort to the general public. It also gives us all a glimpse into potential future threats and our ability to quickly respond to them, both locally and globally.

What We Teach Our Students
Since the importance of biostatistics in public health cannot be overstated, it follows that it’s equally vital for aspiring professionals to learn how to read and use the data in the proper way before they graduate and enter the field. The purpose of biostats in our Master of Public Health program is to teach students to read peer-reviewed articles and newspaper articles and be able to properly and professionally interpret what is written, based on the knowledge they’ve gained in other courses, such as epidemiology and health policy. When you teach these subjects and work in these fields, you have to be able to read, interpret and provide conclusions as solid evidence to support recommended changes in policy or new policies.
We ensure our students understand that they need to be able to carefully design studies, propose sound ways of collecting data and select statistical analyses to interpret whatever they are studying, in order to offer credible direction for interventions. Then, our graduates have to be capable of translating this information clearly for their patients, their clients and the general public, most of whom might not have the same level of knowledge or health literacy as they do.
By allowing and guiding students to find the answers to their own questions using available resources that are accurate, valid and reliable, we show them how to make informed decisions, so that they are prepared to successfully complete all of the objectives above and start working effectively the day after graduation.
Notable Success Stories in Biostatistics and Epidemiology
I believe the greatest successes of biostatistics in public health have been the immunization and vaccination against smallpox, polio, influenza, SARS-CoV-2 and other viruses, along with related data collection. Even in pandemics, it’s important to remember that models are just models, but they have been useful in giving us an orientation in what to do and a good idea of how urgent certain outbreaks were. They have been used to predict the way that outbreaks would develop and progress. Additionally, in many cases, regression analysis has allowed us to see how several different variables all led or did not lead to a specific outcome.
Statistics explain and describe certain events that would not be clear or useful without them. The best examples are all of these infectious diseases and the efforts to control them, but even in smaller, local epidemics, such as salmonella, we always look to biostats for answers.

The Evolution and Future of Biostatistics in Public Health
Significant progress thus far.
Because of the great importance of biostatistics in healthcare, the field is continuously evolving and developing. We have basic mathematical relations and methods that we use, which have definitely been improved over the years and are now providing us with more information as well as more methods of looking at the same data. These advancements are enabling us to see trends, especially in epidemiology and other disease-related research. In addition to infectious diseases, biostatistics are teaching us more about cancer and chronic conditions like diabetes and cardiovascular disease, and have led to the development of better treatment plans to control them.
Healthcare and public health have been developing based on the ability of biostats to provide us with evidence, and that trend will continue in the future. This is the essence of any graduate program related to the health science fields.
The Influence of Technology in the Years Ahead
In the next five to 10 years, I hope that we will have more information about a greater number of diseases as well as the treatment and control of those conditions. The intersection of biostatistics and artificial intelligence is a big part of that. IBM’s programs, for instance, use biostatistics and mathematical models, which is exciting. Consider the capabilities of their Watson supercomputer: Instead of interpreting all of the labs themselves, healthcare professionals and scientists can provide Watson with necessary information about patients, and the computer will provide the diagnoses. It is programmed to consider everything at each moment and make determinations in an incredibly short period of time — something we humans cannot possibly do.
Nevertheless, we must remember that there is no substitute for the human brain and what we can do with the tools and technology available, especially when it comes to inquiring and critical thinking. I have questioned whether or not I, as a patient, would be able to trust a diagnosis from Watson; perhaps instead, I would prefer a good conversation with my physician, during which we could consider everything together and draw our own conclusions.
In multiple ways, this technological progress and innovation are very good and exciting for public health, epidemiology and humanity in general. This change that is available to us is a tool we didn’t have before. If we use it in the right way, we will find new preventative strategies and possibly cures for diseases, and people will get better or not get sick in the first place.
Biostatistics in Social Justice
Social justice is another crucial part of public health and healthcare, and should be underlying the ideas and motivation in all of our work. I work with the United Nations, representing two associations there in the matters of human rights and health issues in girls specifically.
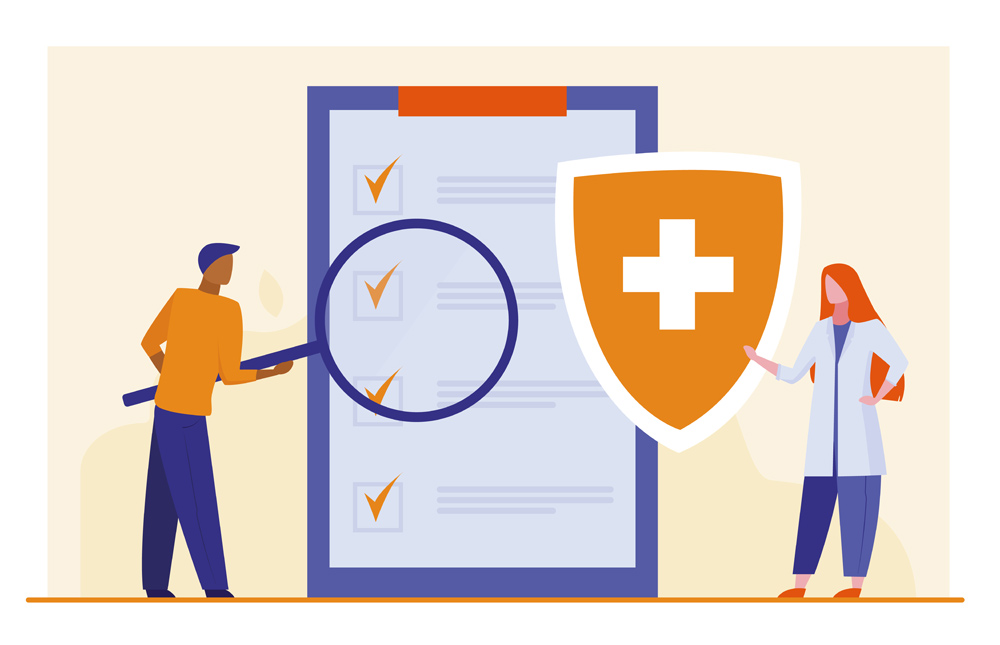
Well-documented Issues of Social Justice
One major problem in some parts of the world — especially sub-Saharan Africa and South Asia, but also East Asia, Latin America, the Middle East and elsewhere — is girls being forced into marriage at ages as young as nine or 10. Another is the fact that many schools in some very poor regions , including sub-Saharan Africa, do not have bathrooms for girls, so they can’t go to school on certain days of the month; there’s almost a structural, physical discrimination against girls in those places. In the countries of Chad and Senegal , for example, fewer than 10% of schools have toilets for female students; in the former, only 25% even have them for males. And in Malawi, neither boys nor girls have toilets or running water in schools. Similar issues exist in parts of the Middle East, such as Afghanistan, where only 4% of children have places to wash their hands.
There are parts of the globe where girls or children in general don’t even have access to elementary education. In Syria, girls are often prevented from going to school at all; with 33% of schools in the country being damaged or destroyed by war, boys’ educational opportunities are often greatly limited as well. Resources taken for granted in the developed world are still shockingly scarce in certain places: for instance, just 1% of schools in Myanmar and 2% in Niger have access to computers.
Some populations of the world — including people in sub-Saharan Africa as well as those in Afghanistan, Pakistan, Cambodia and other areas — are ravaged by diseases like cholera, typhoid, hepatitis and Dengue fever due to a lack of clean water . Many people in these least-developed regions also have extremely limited access to healthcare. And we know well that poverty is one of the essential reasons for all of the disparities and undesirable health outcomes. We can even find some of these great disparities in resources and access here in the United States, with certain communities living in terrible poverty.
Many people are aware of these issues, especially those who make decisions and develop policies, but until they see the numbers and compare different countries and regions, they don’t have the perspective to fully understand the scope and severity of the problems. Advocating for girls’ rights, children’s rights or human rights and social justice is always more effective when you have evidence: data and numbers from biostatistics and public health. The World Health Organization (WHO), for example, has plenty of data on their website, making it a good resource for such purposes. We illustrate all of our activities and support advocacy efforts using these numbers from biostatistical data collection.

Social Justice Issues We Need to Learn More About
Unfortunately, there are still some areas in which we don’t have enough data, including disparities and racial inequalities in health outcomes and access to healthcare.
Additional areas where available data is insufficient are social determinants of health, i.e., the environmental conditions that affect various health, functioning and quality-of-life risks and outcomes. These determinants exist in people’s birthplaces, hometowns, households, workplaces, schools, houses of worship and recreational areas, and are divided into five categories:
- Neighborhood and built environment , where risk factors can include high rates of violence, polluted or otherwise unsafe air or water, secondhand smoke and more.
- Social and community context , with risk factors such as discrimination, low income compared to expenses for essentials, anxiety and depression in caregivers of the disabled and a lack of support for children who have incarcerated parents or suffer mental trauma from bullying.
- Economic stability , which can be impacted by disabilities, illnesses and other conditions that make it hard to find and keep a job, as well as by low salaries that are not commensurate with the cost of living — leading to food insecurity, homelessness and other problems.
- Educational access and quality , which can be jeopardized or diminished by low income, disabilities, bullying and other factors that can increase stress and impair brain development in young kids.
- Healthcare access and quality , where negative effects can be caused by a lack of insurance and inability to afford medications, as well as an absence of needed healthcare services in close proximity to people’s homes.
The lack of data in these areas is not the fault of biostatistics; we have not yet explored these health issues in the appropriate way, and we need to do so to get more information and better understand them.
The Healthy People 2030 initiative is making progress in that endeavor. Subject matter experts from the federal government have been working in different groups and have identified developmental and research objectives to improve health and well-being. Both of these types of goals are related to public health issues that have an insufficient amount of reliable baseline data. Developmental objectives involve high-priority problems that have evidence-based interventions, while research objectives involve problems that are a heavy health or economic burden or have substantial disparities between population groups, and have yet to be linked to evidence-based interventions.
Over time, as interventions become associated with the research objectives, these goals can evolve into the developmental type. Then, when more credible data has been collected, those developmental objectives can further evolve into the core type, which are measurable goals with set targets for the decade.
About the Author
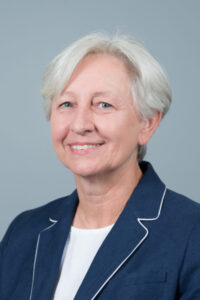
Bojana Beric-Stojsic, PhD is the Associate Professor of Public Health and Director of the Master of Public Health (MPH) Program in the School of Pharmacy and Health Sciences at Fairleigh Dickinson University. She and her team designed the fully online MPH program at FDU from scratch and launched it in the spring of 2020, providing an avenue for students to acquire solid public health leadership skills in health policy, health analytics, epidemiology and other public health areas, in order to prepare them for job placements ranging from nonprofit organizations to governmental organizations.
Previously, Dr. Beric-Stojsic held the position of Chair of the Department of Public Health at Long Island University in Brooklyn, NY. She has also been very active in supporting global social justice and children’s human rights, especially girls’ rights. Since 2010, she has served as an ambassador at the United Nations, representing the national professional association SOPHE (Society for Public Health Education) and the IUHPE-NARO (International Union for Health Promotion and Education – North America Regional Office) as Vice President. In her role as a UN representative, Dr. Beric-Stojsic has been very involved with The Working Group on Girls (WGG) , an organization that helps raise the voices of girls while advocating for and empowering them both here at home and around the world.
Dr. Beric-Stojsic is originally from Yugoslavia (now Serbia) and is passionate about traveling, having visited 40 US states and 23 countries thus far.

- Weill Cornell Medicine

Biostatistics
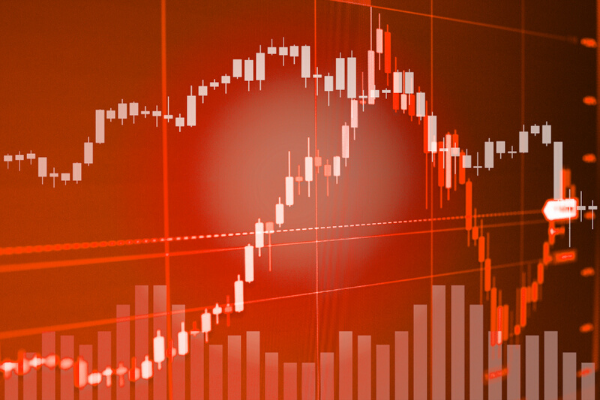
Biostatistics is the application of statistical techniques for scientific research in health-related fields, including medicine, biology and public health. It also encompasses development of novel methodologies that translate to better study design and analyses.
Biostatistics also contributes substantially to the area of Data Science through statistical learning techniques. Since the beginning of the 20th century, the field of biostatistics has become an indispensable tool in improving health and reducing illness. Epidemiology is concerned with the distribution, causation, and control of disease across time and space in human populations.
The Mission of the Division of Biostatistics is to:
- Provide quality statistical support in research design and analysis developed by the clinical and laboratory investigators.
- Train medical students, graduate students, postdoctoral fellows and other research staff in the use of statistical methods and software.
- Train the next generation of biostatisticians and data scientists.
- Develop innovative statistical research guided by and benefiting clinical and laboratory based research projects.
- Create and/or assist with epidemiologic studies in the fields of hypertension, women's health, perioperative outcomes and anesthesia.
The division was formed in October 2004, under the Medical College’s Strategic Plan for further development and retention of research scholars by fostering collaborations with biostatisticians and epidemiologists to further enhance and advance WCM research.
Internship Opportunity
Affiliated education program.
MS in Biostatistics & Data Science
Current Research Highlights
Dr. himel mallick named recipient of 2024 uab national alumni society young alumni rising star award.
July 30, 2024
Dr. Himel Mallick , assistant professor of population health sciences in the Division of Biostatistics at Weill Cornell Medicine, has been selected as a recipient of the 2024 UAB National Alumni Society...
Dr. Hua “Judy” Zhong Named Chief of the Division of Biostatistics at Weill Cornell Medicine
June 23, 2024
Dr. Hua "Judy" Zhong, an esteemed researcher and biostatistician, has been named chief of the Division of Biostatistics in the Department of Population Health Sciences at Weill Cornell Medicine, effective July 29.
Biostatistics is the application of statistical techniques for scientific research in health-related fields, including medicine, biology and public health. It also encompasses development of novel...
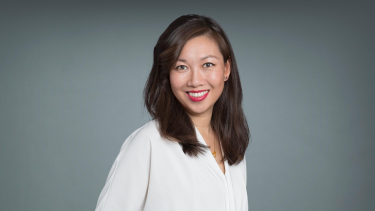
2024 Thomas R. Ten Have Symposium on Statistics in Mental Health
May 22, 2024
The 11th Annual Thomas R. Ten Have Symposium on Statistics in Mental Health will be held on June 28th, 2024, at Weill Cornell Medicine. This event continues the annual Columbia-Cornell-NYU-Penn-Yale Symposium and is jointly sponsored by the five universities. It will feature research presentations, posters, and social programs.
Click here...
Population Health Sciences 402 E. 67th St. New York, NY 10065 Phone: (646) 962-8001
Faculty & Staff
- Administrator, Grants and Finance

- Research Biostatistician III
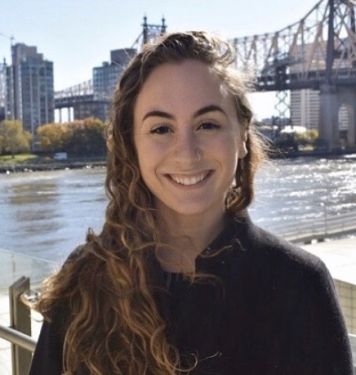
- Research Biostatistician II

- Program Director of Ph.D. in Population Health Sciences
- Associate Professor of Biostatistics in Population Health Sciences
- Associate Professor of Biostatistics in Psychiatry
- Professor of Biostatistics in Population Health Sciences
- Research Assistant

- Assistant Professor of Population Health Sciences

- Associate Professor of Research in Population Health Sciences
- Director of Biostatistics and Epidemiology Consulting Service
- Director of Research Design and Biostatistics Core of the Weill Cornell Clinical and Translational Science Center
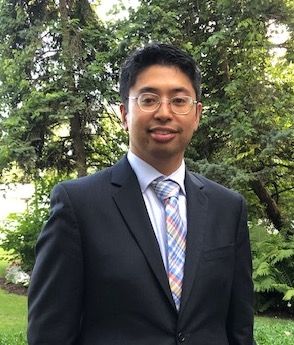
- Programmer Analyst II
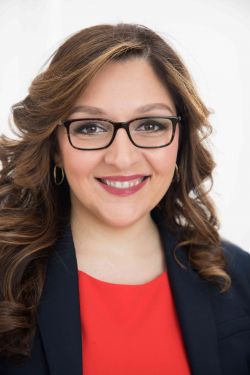
- Assistant Director, Biostatistics Services

- Professor of Population Health Sciences
- Professor of Epidemiology in Medicine

- Research Biostatistician I
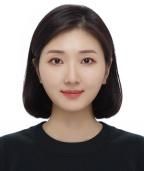
- Postdoctoral Associate
- Program Coordinator
- Clinical Professor of Biostatistics in Population Health Sciences
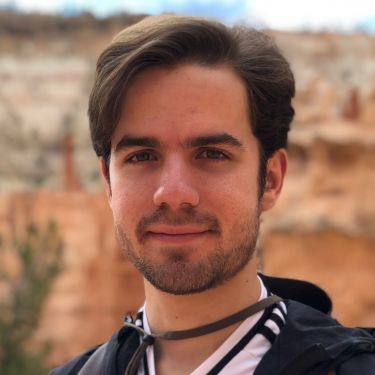
- Healthcare Data Specialist II

- Adjunct Assistant Professor of Population Health Sciences
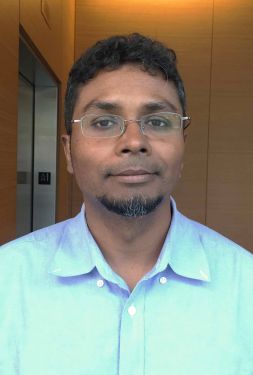
- Program Director, Certificate Program
- Associate Professor of Population Health Sciences
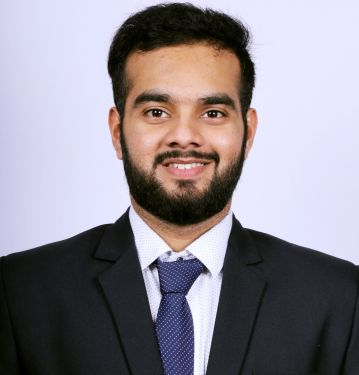
- Programmer Analyst I

- Adjunct Professor of Biostatistics in Population Health Sciences
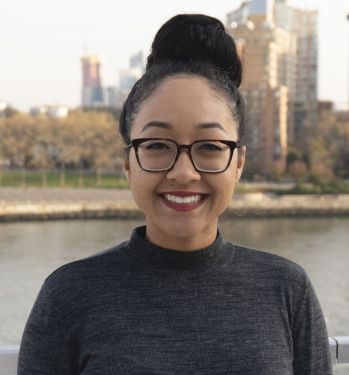
- Instructor in Population Health Sciences
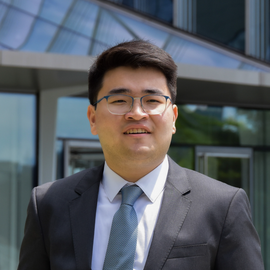
- Chief of the Division of Biostatistics
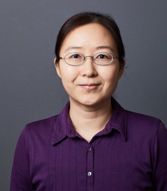
- Program Director of the M.S. in Biostatistics and Data Science
Recent Faculty Publications
Career opportunities.
What is Biostatistics?
bi·o·sta·tis·tics bīōstəˈtistiks / noun the branch of statistics that deals with data relating to living organisms.
Making sense of all the data. That’s one way of defining what a biostatistician does.
Or, as Professor and former Department Chair Patrick Heagerty likes to put it, “Turning data into knowledge.”
Biostatisticians use statistical methods and techniques to improve the health of people and communities. They help answer pressing research questions in medicine, biology and public health, such as whether a new drug works, what causes cancer and other diseases, and how long a person with a certain illness is likely to survive.

“What, for example, does the data say about the association between an environmental exposure and a health outcome?” asks Heagerty, whose expertise is longitudinal studies, or data collected over time.
“Biostatistics is central to all of science, because science needs that gathering of evidence and the evaluation of that evidence to make a judgment.”
Biostatisticians use their quantitative skills to team with experts in other fields, from biologists and cancer specialists to surgeons and geneticists. But they are not mere number-crunchers. They play pivotal roles in designing studies to ensure enough data and the right kind of information are collected. Then they analyze, evaluate and interpret the results – accounting for variables, biases and missing data along the way.
“It’s a field that merges passion and skill with biomedical science and mathematics and statistics,” Heagerty says. “It’s got to have the bio in it somewhere.”
Adds Associate Professor Daniela Witten, “What we bring to the table is an understanding of not only the key statistical issues, but also the underlying biological and medical context.”
Some examples of ongoing and recent biostatistics work at the UW and its potential impact:
- Professor and former Department Chair Thomas Fleming was senior author of a study that showed antiretroviral therapy reduced the risk of heterosexual transmission of HIV by 96 percent – a discovery that could save countless lives and illnesses. Science magazine dubbed it the 2011 “Breakthrough of the Year.”
- Heagerty’s work on back pain with other UW colleagues showed that epidural injections for a common type of back pain made virtually no difference for patients – a finding that could potentially save costs and unnecessary medical procedures.
- Finding links between genetic variants and certain diseases. Now that genome sequencing is relatively cheap, scientists “should be able to identify the genetic underpinnings of a lot of human diseases and obtain a much better understanding of the science than was ever possible before,” according to Witten. That’s what precision medicine and targeted therapy is all about.
- Working with UW Biology Professor Samuel Wasser to use DNA from elephant tusks and dung to pinpoint where poaching occurs in Africa, giving law enforcement and conservation authorities the tools they need to crack down on the illegal trade.
At the UW, the Department of Biostatistics does two main things, according to Heagerty. It prepares students to practice biostatistics on a wide range of scientific teams and it develops ground-breaking thinkers through its PhD program.
“We’re training the next generation of innovators in the methodology,” he says.
That’s important in an era of emerging data sets, from genome sequencing to electronic medical records. New statistical tools and software are often needed to interpret the massive amounts of data and to detect correlations and causations.
The department has a long history of developing innovative methodology.
One of the classic investigative techniques in epidemiology is the case control study. This is research that starts with the outcome, or disease, first, then goes back to find risk factors that could have caused the disease. “It’s sort of a backward study design,” Heagerty says. “But it’s a really efficient study design. Those ideas were developed here by Ross Prentice (professor of Biostatistics).”
Professor Emeritus Norm Breslow, a former Chair of the department, was a leader in the development of survival methods used to study the time until an event such as death occurs. “These are huge contributions to medicine,” Heagerty says.
Then there are clinical trials, where researchers study the impact of a drug versus a placebo.
“Our department became prominent because of its work on how clinical trials are conducted and how the results are interpreted,” says Professor and former Chair Bruce Weir, a pioneer of statistical genetics. “Tom Fleming, Scott Emerson, Norm Breslow and Patrick Heagerty have developed new statistical methods to design clinical trials to interpret the results.”
UW biostatisticians also use their expertise to serve on data safety monitoring committees, overseeing numerous trials to see if they should be stopped early to prevent harm to participants or because a therapy or drug proves immediately effective.
The job market for biostatisticians is hot, from high-tech and pharmaceutical companies to research institutions. Some of the UW’s graduates have gone on to head academic departments elsewhere, giving the department a major role in shaping the careers and educations of biostatisticians across the country.
“The demand for people with our training is enormous,” Heagerty says. “This is a data-rich world and people who can gather the evidence and evaluate it are incredibly valuable in every domain – research, business or health care systems.”
Why Biostats?
Biostatisticians play a unique role in safeguarding public health and improving lives through quantitative research..
By combining across quantitative disciplines, biostatisticians are able to collaborate with other biomedical researchers to identify and solve problems that pose threats to health and to quality of life.
From assessing the health consequences of air quality to designing and evaluating new cancer studies, biostatisticians develop new methods to ensure that policies are based on evidence of benefit—whether targeted to populations or to individuals in need of care.
Biostatistics Ranked #1
Transformative research.
Our students work alongside faculty who are leaders in both statistical theory and its application to health research, in collaboration with laboratory and clinical scientists around the globe.

Examples of Faculty Research Projects
Secrets of Sound Health
Professor Francesca Dominici is a renowned expert in analyzing huge data sets to ferret out hidden environmental causes of disease. Her latest study is the first to analyze noise exposure near airports and its impact on cardiovascular disease.
Cell Phones & Mood Disorders
Associate Professor JP Onnela is developing methods for analyzing and modeling social and biological networks. His group uses cell phone communication and sensor data to investigate social and behavioral functioning of individuals with mood disorders.
Human Microbiome Project
Associate Professor Curtis Huttenhower received a Presidential Early Career Award from the White House in part for his work on the Human Microbiome Project – analyzing role that microbes play in maintaining health and immune function, as well as in disease.
Digging for Research Gold in EMRs
Professor Tianxi Cai has worked on the development of an algorithm that enabled her team to scan Electronic Medical Records (EMRs) – they are working to create a framework to help researchers use large datasets to better understand the genetic basis of complex diseases.
Birth Defects Related to Antiretrovirals
Director of Graduate Studies Paige Williams is working on a study about the safety of antiretroviral (ARV) medications use during pregnancy.
The Next Step
Our graduates are thriving in a wide range of careers in academia, industry, the government, and beyond. See where a degree in Biostatistics has taken them.
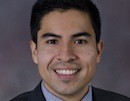
News from the School

Red meat and diabetes

How for-profit medicine is harming health care

A tradition of mentoring

Promising HIV treatment
- Biochemistry and Molecular Biology
Biostatistics
- Environmental Health and Engineering
- Epidemiology
- Health Policy and Management
- Health, Behavior and Society
- International Health
- Mental Health
- Molecular Microbiology and Immunology
- Population, Family and Reproductive Health
- Program Finder
- Admissions Services
- Course Directory
- Academic Calendar
- Hybrid Campus
- Lecture Series
- Convocation
- Strategy and Development
- Implementation and Impact
- Integrity and Oversight
- In the School
- In the Field
- In Baltimore
- Resources for Practitioners
- Articles & News Releases
- In The News
- Statements & Announcements
- At a Glance
- Student Life
- Strategic Priorities
- Inclusion, Diversity, Anti-Racism, and Equity (IDARE)
- What is Public Health?
We create and apply methods for quantitative research in the health sciences, and we provide innovative biostatistics education, making discoveries to improve health.
FIND OUT WHAT'S NEW MEET OUR STUDENTS ALUMNI SPOTLIGHTS SEMINARS
- Key Data Science Classes
- Our Unique Perspective to Biostatistics
- Anti-Racism Resource Directory
- Code of Conduct
- Alan Gittelsohn
- Allyn Kimball
- David Duncan
- Charles Rohde
- Dr. Helen Abbey
- Jerome Cornfield
- Lowell Reed
- Margaret Merrell
- Raymond Pearl
- William Cochran
- Dr. Alan Ross Through the Years
- Message from the Chair
- Health and Wellness
- Biostatistics Student Organization
- Journal/Computing Club
- Departmental Student Awards
- Major Awards in the Field
- Past Honors and Awards Winners
- Schoolwide Awards
- Job Openings
- Application Fee Waiver
- Epidemiology and Biostatistics of Aging Training Grant (NIA-T32)
- Guide to Introductory Biostatistics Course Sequences
- Primary Faculty Within Biostatistics
- Postdoctoral Fellows
- MHS Student Profiles
- PhD Student Profiles
- ScM Student Profiles
- Alumni Listing
- Featured Alumni
- 2016-2017 Noon Seminar Series
- 2018-2019 Noon Seminar Series
- 2019-2020 Noon Seminar Series
- 2020-2021 Noon Seminar Series
- 2022-2023 Seminar Series
- 2023-2024 Seminar Series
- E-Newsletters
- PhD Student Defenses
- Make a Gift
- Professional Society Awards
- Student Support Faculty
Biostatistics Headlines
Alumni spotlight: christopher lo, scm '23.
Christopher Lo, ScM ’23, is a data science trainer in the Data Science Lab at the Fred Hutch Cancer Center where he teaches biomedical data science to the Fred Hutch Cancer Center community.
Noted Biostatistician and Epidemiologist Jim Tonascia Retires
Jim Tonascia, whose public health career in biostatistics and epidemiology spanned more than five decades, retired from the Bloomberg School of Public Health this August.
Student Spotlight: Alyssa Columbus
Alyssa Columbus is a second-year PhD student in the Department of Biostatistics with an interest in public health informatics and data science, including educational interventions, ethical considerations, and policy implications.
What We Do in the Department of Biostatistics
The Bloomberg School's Department of Biostatistics is the oldest department of its kind in the world and has long been considered one of the best. Our faculty conduct research across the spectrum of statistical science, from foundations of inference to the discovery of new methodologies for health applications.
Our designs and analytic methods enable health scientists and professionals across industries to efficiently acquire knowledge and draw valid conclusions from ever-expanding sources of information.
Biostatistics Highlights
First in u.s..
First freestanding statistics department in the U.S.
Data science driving health and empowering opportunity
Foundational discoveries for inference and modeling
Creative, close-knit community
Biostatistics Programs
The Department of Biostatistics offers three graduate programs to applicants with a bachelor's degree (or higher) interested in professional or academic careers at the interface of the statistical and health sciences.
We also have funded training programs in the Epidemiology and Biostatistics of Aging for PhD students who are U.S. citizens or permanent residents.
Master of Health Science (MHS)
Our one-year MHS program provides study in biostatistical theory & methods. It is also open to students concurrently enrolled in a JHU doctoral program.
Master of Science (ScM)
Our ScM targets individuals who have demonstrated prior excellence in quantitative or biological sciences and desire a career as a professional statistician.
Doctor of Philosophy (PhD)
Our PhD graduates lead research in the foundations of statistical reasoning, data science, and their application making discoveries to improve health.
Nilanjan Chatterjee, PhD
Bloomberg Distinguished Professor Nilanjan Chatterjee, PhD, MS, models disease risk associated with genetics, lifestyle, biomarkers, and other factors, with the goal of improving disease prevention. Chatterjee recently received a GKII-KCDH Breakthrough Research Grant on Digital Health. His winning research proposal with Saket Choudhary will involve development of the first risk prediction model and clinical tool for the Indian population.
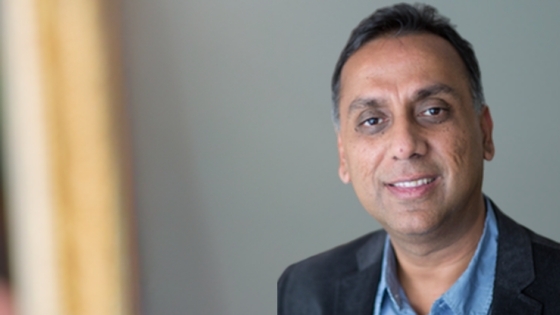
Biostatistics Consulting Center
The Johns Hopkins Biostatistics Center is the practice arm of our Department, providing the latest in biostatistical and information science expertise to a wide range of clients both within and outside Johns Hopkins.
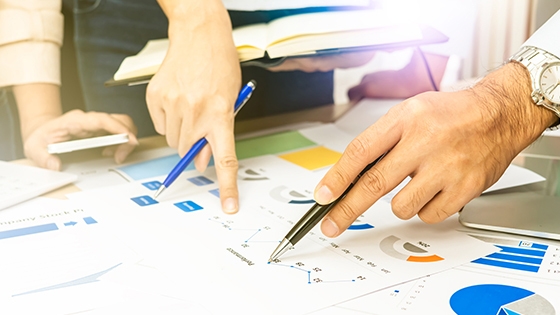
Alyssa Columbus, Second-Year PhD Student
Alyssa Columbus is a second-year PhD student with an interest in public health informatics and data science, including educational interventions, ethical considerations (e.g., privacy and security) , and policy implications.
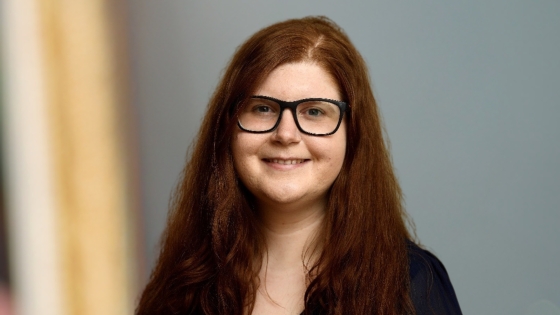
Follow our Department and Stay Up-To-Date!
Biostatistics dept seminar: opportunities for incorporating intersectionality into biomedical informatics, support our department.
A gift to our department can help to provide student scholarships and internships, attract and retain faculty, and support innovation.
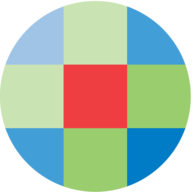
- Subscribe to journal Subscribe
- Get new issue alerts Get alerts
Secondary Logo
Journal logo.
Colleague's E-mail is Invalid
Your message has been successfully sent to your colleague.
Save my selection
Essentials of Biostatistics in Public Health, 3rd Edition
Description.
This book describes the fundamental concepts used in public health research, including epidemiology of diseases, study designs, and statistical methods. The author is a renowned biostatistician with a strong background in teaching biostatistics and mines her experience with the Framingham study to provide examples and illustrations.
The purpose of this book is to provide an overview of biostatistical methods used in public health. The book uses step-by-step guidance to understanding, applying, and interpreting biostatistical methods. It will help users master widely used biostatistical methods through reading and practice and develop critical thinking in applying these methods.
Beginner-level students in biostatistics, including graduate students, clinician scientists, and public health researchers, are the intended audience.
BOOK CONTENT/FEATURES
The 12 chapters include a beginner-level introduction to biostatistics and study designs and explanations of key concepts in data collection, presentation, and data analysis. One chapter demonstrates data presentation in the form of tables and figures. Each chapter begins with delineation of key questions in the form of “when and why” and relevant learning objectives, followed by concise descriptions of the concepts and methods, and finishing with a chapter summary. The end of each chapter affords students an opportunity to apply the knowledge they’ve gained by practicing on real-life data using Excel spreadsheets. Throughout the book, the author uses examples from the Framingham study. Tables and graphs are simple yet meaningful. The appendix contains useful statistical tables students can use to quickly obtain critical values. The glossary provides a nice recap of statistical terms and concepts presented in the book.
WEBSITE CONTENT/FEATURES
This book includes access to Navigate 2, an online companion that includes an e-book version and practice materials.
This will be an excellent resource for students, clinicians, and researchers who are looking for a clear and concise book on basic and advanced statistical methods. The use of real-life data for illustration and practice makes this an interesting and ideal book for those who want to improve biostatistical skills through hands-on practice. This book is strongly recommended for graduate-level students as well as clinicians interested in pursuing a career in public health research.
Rating: ★★★
Reviewed by: Furqan Khattak, MD ( East Tennessee State University Quillen College of Medicine )
- + Favorites
- View in Gallery
- Our Leadership
- Faculty Directory
- Administrative Staff
- SPH Committees
- SPH Councils
- Social Justice
- Accreditation
- Research News
- Faculty Publications
- Student Opportunities
- Center For Infectious Disease Studies
- EASA Center For Excellence
- Gun Violence Prevention Research
- Undergraduate Programs
- Graduate Certificates
- Master of Public Health
- Master of Science
- Dual Degree Programs
- Doctoral Programs
- Tuition and Fees
- Careers in Public Health
- Undergraduate Students
- Graduate Students
- Newly Admitted Students
- Student Groups & Organizations
- Student Success Center
- Milestone Program
- Graduation & Ceremonies
- Career Resources
- This Is Public Health
- Alumni and Professionals
- SPH Job Board
- Public Health Portland Style
- Public Health Practice Team
- Public Health News
- SPH Communications
Biostatistics Research in Public Health
What is biostatistics.
Researchers in biostatistics at the OHSU-PSU School of Public Health help drive and clarify the school’s research by applying and developing statistics methods to better understand public health.
Advanced biostatistics is essential to analyze complex and big data – or huge amounts of data in healthcare and other fields – and reveal and quantify the possible effects of health interventions on individuals, groups and populations. A sound analysis by biostatisticians has become even more important as the public and policymakers try to understand the effects of health reform initiatives, and as public health and health care systems increasingly use electronic health records, population-based health surveys and large scale community-based interventions.
Our biostatistics faculty are experts in Bayesian analysis, survival analysis, categorical data analysis, complex sampling/clinical trial design, statistical genetics, and machine learning and big data, among other areas. They collaborate with other scientists at OHSU in highly diverse projects such as clinical and community-based intervention studies, laboratory experiments and observational studies. We collaborate with School of Public Health investigators to select the most appropriate study design and analysis methods to align with the research questions they’re trying to answer.
Biostatistics faculty members contribute to groundbreaking research at the OHSU Knight Cancer Institute, the Oregon Clinical and Translational Research Institute, the Center for Health Research at Kaiser Permanente and epidemiology and health services research groups within the School of Public Health.
The OHSU-PSU School of Public Health is home to the Biostatistics & Design Program (BDP) , an OHSU Research Core dedicated to providing high quality biostatistics collaboration, with particular expertise in population science. Several of our Biostatistics faculty are involved in the BDP, as are a number of masters and PhD level staff biostatisticians. Internships for students enrolled in the Biostatistics programs (MS, MPH and Graduate Certificate) are possible through BDP.
- Rankings, Awards, and Stats
- Department Awards
- Undergraduate
- Information for New Statistics Freshmen
- Statistics Major Advising and Registration FAQs
- Statistics Major
- Statistics Minor
- Academic and Research Opportunities
- Clubs and Organizations
- Ph.D. Programs
- Master’s Programs
- Online Programs
- Application Process
- Student Life
- Research Areas
- Administration
- Graduate Students
- Faculty Resources
- Staff Resources
- Student Resources
- IT Resources
What Is Biostatistics?
Almost daily, the popular media report new research findings related to human health.
- A new treatment for HIV disease works better than current therapies
- High blood pressure is demonstrated to be associated with heart disease
- A study suggests that a certain pollutant may be harmful to humans
- Hormone replacement therapy is determined to carry increased risk of certain types of cancer (and the evidence is so compelling that the study is stopped earlier than planned)
Such results are the work of multidisciplinary teams of researchers, including physicians, public and environmental health specialists, and BIOSTATISTICIANS . Biostatisticians play essential roles in designing the studies, analyzing the data, and creating new methods for addressing these problems.
There is a critical shortage of biostatisticians with graduate training, and their skills are in great demand.
So What Is Biostatistics?
Statistics is the science that:
- develops methods for asking the right questions
- designs studies for collecting data relevant to answering the questions
- summarizes analyses and draws conclusions from the data
Statistics combines mathematical theory with knowledge of the specific challenges arising in different areas of science, making it a rewarding field of study for students who like math and quantitative problems and want to contribute to the advance of broader scientific understanding. Biostatistics is the exciting field of development and application of statistical methods to research in health-related fields, including medicine, public health and biology.
Since early in the 20th century, biostatistics has become an indispensable tool for understanding the cause, natural history and treatment of disease in order to improve human health. Biostatisticians work with scientists to identify and implement the correct statistical methods for designing studies and analyzing and interpreting the results. And as science progresses and new ways to measure and collect information become possible, new statistical techniques must be developed. With the breathtaking pace of science today, the skills of biostatisticians are especially in demand because of:
- new advances in bioinformatics and computational biology, genetics, neuroimaging, environmental science and many other areas
- the ability to collect, store and manipulate vast amounts of data, including electronic health records
These new challenges are giving rise to novel problems needing new statistical solutions. Biostatisticians are the experts who can make this happen!
Opportunities for Biostatisticians
Biostatisticians with graduate training are needed who can:
- collaborate with scientists on conception, design, analysis and interpretation of novel studies
- address new challenges arising from advances in biomedical science
- train the next generation of biostatisticians
- Doctoral-level biostatisticians are needed in academia as faculty to teach graduate courses, direct student research and develop new methods
- Master’s and doctoral-level biostatisticians are sought to collaborate with investigators in institutions such as cancer research centers and medical schools on the design, analysis, and interpretation of studies
- Federal agencies such as the National Institutes of Health, the Environmental Protection Agency, and the Food and Drug Administration hire biostatisticians with graduate training to carry out research and to collaborate on setting of policy and approval of drugs
- The pharmaceutical industry is a major employer of biostatisticians, who work in every aspect of drug development
Starting salaries are competitive, and the work is very rewarding!
Training to Be a Biostatistician
Graduate-level training in biostatistics is available in Departments of Statistics and Biostatistics in universities nationwide. Students come from a broad variety of undergraduate majors including statistics, mathematics, computer science, engineering, biology, physics, economics, psychology and education. A background in calculus and linear algebra and computing experience is very helpful. An introductory statistics course covering the basics of statistical thinking is also good preparation.
Upon enrolling in a graduate program, students take courses in statistical theory and methods. Theory courses provide the mathematical foundation underlying statistical methods. Methods courses focus on the appropriate use of statistical techniques for different types of problems. Further courses build on this background, covering specialized topics such as the analysis of survival data, longitudinal data analysis and advanced statistical modeling.
An excellent way to find out if biostatistics is the right career path for you is to enroll in the Summer Institute in Biostatistics!
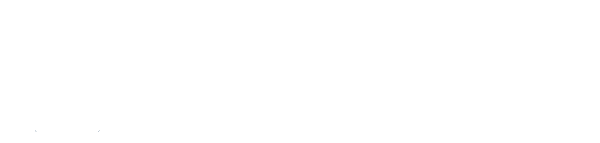
Information for:
- Current Students
- Faculty & Staff
- Degrees & Programs
- Campus Maps
- Jobs & Careers
- Campus Shuttles
- Student Life
- Giving
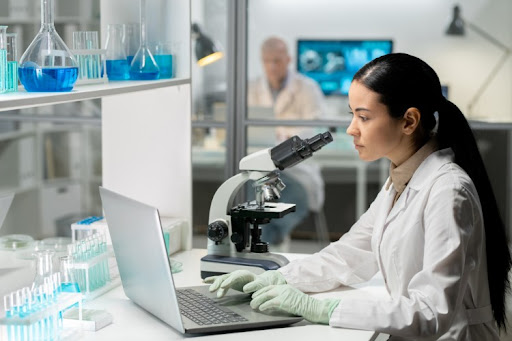
- Augusta University
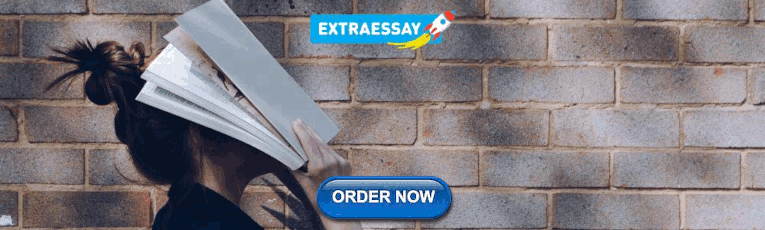
Biostatistics vs. Epidemiology: Key Topics in Public Health
In public health, knowledge is power. The work of public health professionals such as biostatisticians and epidemiologists can have a tremendous impact on individuals and communities. When their efforts are successful, they can help improve the quality of life in a community, provide children with better opportunities to thrive, reduce health issues and save community members money.
The backbone of effective public health strategy is research and analysis. By gathering, analyzing and monitoring data and information, public health officials can identify health issues and develop solutions that potentially lessen their burden on communities. These efforts can range from tracking diseases to being an advocate for laws that keep people safe.
Individuals interested in pursuing a Master of Public Health degree should understand the differences between biostatistics and epidemiology and how these differences shape careers in each field.
What Is Biostatistics?
Biostatistics is a subfield of biology that focuses on gathering, analyzing, interpreting and presenting biologically based scientific data. This information can be used to develop programs, initiatives and other strategies that can lead to programs that encourage healthier living.
The data behind biostatistics can be obtained through health-related information, such as medical records, or vital statistics, such as birth and death dates. Biostatisticians may gather data through sources that provide specific patient information, such as insurance claims; by researching peer-reviewed journals; or by conducting simple surveys.
The Role of Biostatistics in Public Health
In public health, biostatistics can uncover information on a specific health issue, its current effects and its potential impact if it’s not acted on. This information can be used to help build healthier communities.
For instance, biostatistics can do the following:
- Identify areas or populations that are more susceptible to specific diseases.
- Pinpoint vulnerabilities in a health care system, such as factors that may prevent people from receiving care.
- Track the effectiveness of community programs, such as school lunch programs.
Biostatisticians analyze a population segment and apply their findings to a broader population, which makes developing solutions more efficient.
What Is Epidemiology?
Epidemiology focuses on diseases and injuries in populations. Specifically, it is the study and analysis of a disease’s mechanics, causes, risks, frequency and transmission. The goal of epidemiology is to identify patterns in a disease’s cause and spread, and to help come up with strategies that mitigate its impact.
Epidemiology typically involves identifying a disease’s origin, tracking its outbreak, studying its characteristics and then developing mitigation strategies to slow or stop its spread. The process is methodical and takes time, particularly when the disease being studied is unknown. The process can also include the development of short-term strategies to mitigate the disease’s impact pending a long-term solution.
The Role of Epidemiology in Public Health
Epidemiology is often linked to public health and is associated with government agencies and universities. While the primary goal of epidemiology is to keep the public informed and educated about diseases and injuries, the field can be broken down into specific areas of public health.
These areas include:
- Infectious diseases
- Chronic diseases
- Public health preparedness
- Environmental health
- Veterinary epidemiology
Biostatistics vs. Epidemiology: Careers and Salaries
While professionals in both fields play a crucial role in public health, individuals considering biostatistics vs. epidemiology careers should closely examine what these professionals do.
What Does a Biostatistician Do?
Biostatisticians design, develop and execute analytical studies on various public health issues or concerns. These studies can take any of a number of forms, such as clinical trials, public health interventions like vaccination rollouts or studies on various health determinants.
While their focus depends on the precise subject being studied, their methodologies typically include:
- Data gathering
- Interpretation
The work of biostatisticians can lead to the design and implementation of programs intended to improve public health. In this aspect of the job, biostatisticians can work with health systems management professionals to create programs and policies within specific budgetary parameters.
Employers require biostatisticians to have an advanced degree such as a Master of Public Health, with a terminal degree like a PhD making candidates even more competitive. Biostatisticians must have strong analytical, technological and critical thinking skills to be able to analyze and interpret data. They must also have well-developed communication skills to accurately share their findings with others.
Biostatistician Salary and Job Growth
The U.S. Bureau of Labor Statistics (BLS) classifies biostatisticians under the mathematicians and statisticians category. The BLS notes that the 2021 median annual salary for those in mathematical science fields was $98,680. Factors that can affect an individual’s salary include their education level, years of experience and job location.
The BLS projects 31 percent job growth in the mathematicians and statisticians field between 2021 and 2031. This percentage is substantially higher than the average 5 percent growth the BLS predicts for all professions.
What Does an Epidemiologist Do?
Epidemiologists coordinate studies on certain diseases, injuries and ailments. Through the collection and analysis of various forms of data, they aim to detect patterns that could provide insight into a health issue’s origins and mechanics, such as how it may be spread. This data can come from sources that range from patient surveys and interviews to samples of body fluids.
Epidemiologists also work with other public health officials to educate them on how to mitigate the effects of diseases. They can write grant proposals to fund research projects as well.
The minimum education requirement for epidemiologists is an advanced degree such as a master’s in public health, although some in the field hold a doctoral degree. An effective epidemiologist needs strong mathematics and statistics skills to recognize patterns in data. They need solid leadership skills, as they may direct staff working with them on projects. They also need to have strong critical thinking skills, be detail oriented and be excellent communicators.
Epidemiologist Salary and Job Growth
The BLS reports that the 2021 median annual salary for epidemiologists was $78,830. Factors like their education level, years of experience and job location can dictate the exact salary an individual epidemiologist may receive.
The BLS predicts 26 percent job growth for epidemiologists between 2021 and 2031. This is significantly more than the average 5 percent growth the BLS projects for all professions.
Make a Key Difference in Public Health
When comparing the two fields, biostatistics vs. epidemiology, it’s clear they both play essential roles in equipping individuals with the knowledge they need to make important decisions about their health. Both biostatisticians and epidemiologists can supply crucial information on an unexpected pandemic or a known concern like cancer or heart disease, and they both can encourage behaviors that can lead to people living healthier, happier lives.
Augusta University Online’s Master of Public Health program can prepare you to help people make these empowering decisions. Our program is designed to help you grow the knowledge and skills you need to recognize health issues and develop programs that allow communities to thrive. Learn how we can help you make a positive impact in an important field.
Recommended Readings Fact vs Fiction: Augusta University Expert Says Students Need More Education About Social Media Use MPH Requirements, Curriculum and Career Opportunities 3 Public Health Topics for Research
Sources: American Public Health Association, What Is Public Health? Britannica, Epidemiology Centers for Disease Control and Prevention, About COVID-19 Epidemiology Indeed, Biostatistics: Definition, Importance and Applications Indeed, What Does a Biostatistician Do? (Plus Requirements) U.S. Bureau of Labor Statistics, Epidemiologists U.S. Bureau of Labor Statistics, Mathematicians and Statisticians
Want to hear more about Augusta University Online’s programs?
Fill out the form below, and an admissions representative will reach out to you via email or phone with more information. After you’ve completed the form, you’ll automatically be redirected to learn more about Augusta University Online and your chosen program.

An official website of the United States government
The .gov means it’s official. Federal government websites often end in .gov or .mil. Before sharing sensitive information, make sure you’re on a federal government site.
The site is secure. The https:// ensures that you are connecting to the official website and that any information you provide is encrypted and transmitted securely.
- Publications
- Account settings
- My Bibliography
- Collections
- Citation manager
Save citation to file
Email citation, add to collections.
- Create a new collection
- Add to an existing collection
Add to My Bibliography
Your saved search, create a file for external citation management software, your rss feed.
- Search in PubMed
- Search in NLM Catalog
- Add to Search
Guidance for biostatisticians on their essential contributions to clinical and translational research protocol review
Affiliations.
- 1 Department of Preventive Medicine, Division of Biostatistics, Feinberg School of Medicine, Northwestern University, Chicago, IL, USA.
- 2 Department of Biostatistics, University of Michigan, Washington Heights, Ann Arbor, MI, USA.
- 3 Department of Biostatistics and Data Science, Division of Public Health Sciences, Wake Forest School of Medicine, Winston-Salem, NC, USA.
- 4 Michigan Institute for Clinical & Health Research (MICHR), University of Michigan, Ann Arbor, MI, USA.
- 5 Department of Public Health, Division of Biostatistics, University of Miami, Miami, FL, USA.
- 6 School of Public Health, Oregon Health & Sciences University, Portland, OR, USA.
- 7 Department of Public Health Sciences, Medical University of South Carolina, Charleston, SC, USA.
- 8 Department of Medicine, Division of Preventive Medicine, University of Alabama at Birmingham, Birmingham, AL, UK.
- 9 Department of Biostatistics, Indiana University, Indianapolis, IN, USA.
- 10 Department of Public Health Sciences, UC Davis School of Medicine, Davis, CA, USA.
- 11 Duke Biostatistics, Epidemiology and Research Design (BERD) Methods Core, Duke University, Durham, NC, USA.
- 12 Tufts Clinical and Translational Science Institute, Tufts University, Boston, MA, USA.
- 13 Institute of Clinical Research and Health Policy Studies, Tufts Medical Center, Boston, MA, USA.
- 14 Department of Medicine, Division of Allergy, Pulmonary, and Critical Care Medicine, Medical Director, Vanderbilt Human Research Protections Program, Vice-President for Clinical Trials Innovation and Operations, Nashville, TN, USA.
- 15 Department of Biomedical Data Science, Division of Biostatistics, Geisel School of Medicine at Dartmouth, Hanover, NH, USA.
- 16 Department of Biostatistics, Vanderbilt University Medical Center, Nashville, TN, USA.
- 17 Department of Preventive Medicine and Population Health, University of Texas Medical Branch, Galveston, TX, USA.
- PMID: 34527300
- PMCID: PMC8427547
- DOI: 10.1017/cts.2021.814
Rigorous scientific review of research protocols is critical to making funding decisions, and to the protection of both human and non-human research participants. Given the increasing complexity of research designs and data analysis methods, quantitative experts, such as biostatisticians, play an essential role in evaluating the rigor and reproducibility of proposed methods. However, there is a common misconception that a statistician's input is relevant only to sample size/power and statistical analysis sections of a protocol. The comprehensive nature of a biostatistical review coupled with limited guidance on key components of protocol review motived this work. Members of the Biostatistics, Epidemiology, and Research Design Special Interest Group of the Association for Clinical and Translational Science used a consensus approach to identify the elements of research protocols that a biostatistician should consider in a review, and provide specific guidance on how each element should be reviewed. We present the resulting review framework as an educational tool and guideline for biostatisticians navigating review boards and panels. We briefly describe the approach to developing the framework, and we provide a comprehensive checklist and guidance on review of each protocol element. We posit that the biostatistical reviewer, through their breadth of engagement across multiple disciplines and experience with a range of research designs, can and should contribute significantly beyond review of the statistical analysis plan and sample size justification. Through careful scientific review, we hope to prevent excess resource expenditure and risk to humans and animals on poorly planned studies.
Keywords: Biostatistician; Protocol; Review; Scientific rigor; Translational research.
© The Association for Clinical and Translational Science 2021.
PubMed Disclaimer
Conflict of interest statement
The authors have no conflicts of interest to declare.
Illustration of varying degrees of…
Illustration of varying degrees of relevance for protocol items across common study types.…
Similar articles
- Folic acid supplementation and malaria susceptibility and severity among people taking antifolate antimalarial drugs in endemic areas. Crider K, Williams J, Qi YP, Gutman J, Yeung L, Mai C, Finkelstain J, Mehta S, Pons-Duran C, Menéndez C, Moraleda C, Rogers L, Daniels K, Green P. Crider K, et al. Cochrane Database Syst Rev. 2022 Feb 1;2(2022):CD014217. doi: 10.1002/14651858.CD014217. Cochrane Database Syst Rev. 2022. PMID: 36321557 Free PMC article.
- The future of Cochrane Neonatal. Soll RF, Ovelman C, McGuire W. Soll RF, et al. Early Hum Dev. 2020 Nov;150:105191. doi: 10.1016/j.earlhumdev.2020.105191. Epub 2020 Sep 12. Early Hum Dev. 2020. PMID: 33036834
- [The role of biostatistics in institutional review boards]. Schlattmann P, Scherag A, Rauch G, Mansmann U. Schlattmann P, et al. Bundesgesundheitsblatt Gesundheitsforschung Gesundheitsschutz. 2019 Jun;62(6):751-757. doi: 10.1007/s00103-019-02951-9. Bundesgesundheitsblatt Gesundheitsforschung Gesundheitsschutz. 2019. PMID: 31065736 Review. German.
- Bioethical Issues in Biostatistical Consulting Study: Additional Findings and Concerns. Wang MQ, Fan AY, Katz RV. Wang MQ, et al. JDR Clin Trans Res. 2019 Jul;4(3):271-275. doi: 10.1177/2380084419837294. Epub 2019 Apr 22. JDR Clin Trans Res. 2019. PMID: 31009581
- Translational Metabolomics of Head Injury: Exploring Dysfunctional Cerebral Metabolism with Ex Vivo NMR Spectroscopy-Based Metabolite Quantification. Wolahan SM, Hirt D, Glenn TC. Wolahan SM, et al. In: Kobeissy FH, editor. Brain Neurotrauma: Molecular, Neuropsychological, and Rehabilitation Aspects. Boca Raton (FL): CRC Press/Taylor & Francis; 2015. Chapter 25. In: Kobeissy FH, editor. Brain Neurotrauma: Molecular, Neuropsychological, and Rehabilitation Aspects. Boca Raton (FL): CRC Press/Taylor & Francis; 2015. Chapter 25. PMID: 26269925 Free Books & Documents. Review.
- Self-management including exercise, education and activity modification compared to usual care for adolescents with Osgood-Schlatter (the SOGOOD trial): protocol of a randomized controlled superiority trial. Krommes K, Thorborg K, Clausen MB, Rathleff MS, Olesen JL, Kallemose T, Hölmich P. Krommes K, et al. BMC Sports Sci Med Rehabil. 2024 Apr 20;16(1):89. doi: 10.1186/s13102-024-00870-0. BMC Sports Sci Med Rehabil. 2024. PMID: 38643184 Free PMC article.
- A comprehensive survey of collaborative biostatistics units in academic health centers. Hanlon AL, Lozano AJ, Prakash S, Bezar EB, Ambrosius WT, Brock G, Desai M, Pollock BH, Sammel MD, Spratt H, Welty LJ, Pomann GM. Hanlon AL, et al. Stat (Int Stat Inst). 2022 Dec;11(1):e521. doi: 10.1002/sta4.521. Epub 2022 Dec 28. Stat (Int Stat Inst). 2022. PMID: 37502567 Free PMC article.
- A template for the authoring of statistical analysis plans. Stevens G, Dolley S, Mogg R, Connor JT. Stevens G, et al. Contemp Clin Trials Commun. 2023 Jun 9;34:101100. doi: 10.1016/j.conctc.2023.101100. eCollection 2023 Aug. Contemp Clin Trials Commun. 2023. PMID: 37388218 Free PMC article.
- A multicentric, 2 × 2 factorial, randomised, open-label trial to evaluate the clinical effectiveness of structured physical activity training and cognitive behavioural therapy versus usual care in heart failure patients: a protocol for the PACT-HF trial. Jeemon P, Reethu S, Ganapathi S, Lakshmi Kanth LR, Punnoose E, Abdullakutty J, Mattumal S, Joseph J, Joseph S, Venkateswaran C, Sunder P, Babu AS, Padickaparambil S, Neenumol KR, Chacko S, Shajahan S, Krishnankutty K, Devis S, Joseph R, Shemija B, John SA, Harikrishnan S. Jeemon P, et al. Wellcome Open Res. 2022 Aug 12;7:210. doi: 10.12688/wellcomeopenres.18047.1. eCollection 2022. Wellcome Open Res. 2022. PMID: 36105556 Free PMC article.
- How to write statistical analysis section in medical research. Dwivedi AK. Dwivedi AK. J Investig Med. 2022 Dec;70(8):1759-1770. doi: 10.1136/jim-2022-002479. Epub 2022 Jun 16. J Investig Med. 2022. PMID: 35710142 Free PMC article.
- NIH. NIH Peer Review: Grants and Cooperative Agreements [Internet], 2015. ( https://grants.nih.gov/grants/PeerReview22713webv2.pdf )
- Mayden KD.Peer review: publication’s gold standard. Journal of the Advanced Practitioner in Oncology 2012; 3(2): 117. - PMC - PubMed
- Selker HP, Welch LC, Patchen-Fowler E, et al.Scientific Review Committees as part of institutional review of human participant research: initial implementation at institutions with Clinical and Translational Science Awards. Journal of Clinical and Translational Science 2020; 4(2): 115–124. - PMC - PubMed
- Emanuel EJ, Wendler D, Grady C.What makes clinical research ethical? Journal of the American Medical Association 2000; 283(20): 2701–2711. - PubMed
- Eblen MK, Wagner RM, Roy Chowdhury D, Patel KC, Pearson K.How criterion scores predict the overall impact score and funding outcomes for National Institutes of Health peer-reviewed applications. PLoS One 2016; 11(6): e0155060. - PMC - PubMed
Publication types
- Search in MeSH
Related information
Grants and funding.
- UL1 TR003096/TR/NCATS NIH HHS/United States
- P30 DK123704/DK/NIDDK NIH HHS/United States
- P30 CA023108/CA/NCI NIH HHS/United States
- P30 AR072582/AR/NIAMS NIH HHS/United States
- UL1 TR001420/TR/NCATS NIH HHS/United States
- UL1 TR001439/TR/NCATS NIH HHS/United States
- UL1 TR002243/TR/NCATS NIH HHS/United States
- UL1 TR001450/TR/NCATS NIH HHS/United States
- UL1 TR002544/TR/NCATS NIH HHS/United States
- UL1 TR002529/TR/NCATS NIH HHS/United States
- UL1 TR002736/TR/NCATS NIH HHS/United States
- UL1 TR001086/TR/NCATS NIH HHS/United States
- UL1 TR001422/TR/NCATS NIH HHS/United States
- U54 GM104941/GM/NIGMS NIH HHS/United States
- UL1 TR002553/TR/NCATS NIH HHS/United States
- UL1 TR000002/TR/NCATS NIH HHS/United States
- UL1 TR002240/TR/NCATS NIH HHS/United States
LinkOut - more resources
Full text sources.
- Europe PubMed Central
- PubMed Central
- Citation Manager
NCBI Literature Resources
MeSH PMC Bookshelf Disclaimer
The PubMed wordmark and PubMed logo are registered trademarks of the U.S. Department of Health and Human Services (HHS). Unauthorized use of these marks is strictly prohibited.
- Open access
- Published: 05 February 2020
Why do you need a biostatistician?
- Antonia Zapf ORCID: orcid.org/0000-0001-5339-2472 1 ,
- Geraldine Rauch 2 &
- Meinhard Kieser 3
BMC Medical Research Methodology volume 20 , Article number: 23 ( 2020 ) Cite this article
26k Accesses
16 Citations
24 Altmetric
Metrics details
The quality of medical research importantly depends, among other aspects, on a valid statistical planning of the study, analysis of the data, and reporting of the results, which is usually guaranteed by a biostatistician. However, there are several related professions next to the biostatistician, for example epidemiologists, medical informaticians and bioinformaticians. For medical experts, it is often not clear what the differences between these professions are and how the specific role of a biostatistician can be described. For physicians involved in medical research, this is problematic because false expectations often lead to frustration on both sides. Therefore, the aim of this article is to outline the tasks and responsibilities of biostatisticians in clinical trials as well as in other fields of application in medical research.
Peer Review reports
What is a biostatistician, what does he or she actually do and what distinguishes him or her from, for example, an epidemiologist? If we would ask this our main cooperation partners like physicians or biologists, they probably could not give a satisfying answer. This is problematic because false expectations often lead to frustration on both sides. Therefore, in this article we want to clarify the tasks and responsibilities of biostatisticians.
There are some expressions which are often used interchangeably to the term ‘biostatistician’. In here, we will use the expression ‘(medical) biostatistics’ as a synonym for ‘medical biometry’ and ‘medical statistics’, and analogously we will do for the term ‘biostatistician’.
In contrast to the clearly defined educational and professional career steps of a physician, there is no unique way of becoming a biostatistician. Only very few universities do indeed offer studies in biometry, which is why most people working as biostatisticians studied something related, subjects such as mathematics or statistics, or application subjects such as medicine, psychology, or biology. So a biostatistician cannot be defined by his or her education, but must be defined by his or her expertise and competencies [ 1 ]. This corresponds to our definition of a biostatistician in this article. The International Biometric Society (IBS) provides a definition of biometrics as a ‘field of development of statistical and mathematical methods applicable in the biological sciences’ [ 2 ]. In here, we will focus on (human) medicine as area of application, but the results can be easily transferred to the other biological sciences like, for example, agriculture or ecology. As mentioned above, there are some professions neighbouring biostatistics, and for many cooperation partners, the differences between biostatisticians, medical informaticians, bioinformaticians, and epidemiologists are not clear. According to the current representatives of these four disciplines within the German Association for Medical Informatics, Biometry and Epidemiology (GMDS) e. V.:
‘Medical biostatistics develops, implements, and uses statistical and mathematical methods to allow for a gain of knowledge from medical data.’ ‘Results are made accessible for the individual medical disciplines and for the public by statistically valid interpretations and suitable presentations’ (authors’ translation from [ 3 ]).
‘Medical informatics is the science of the systematic development, management, storage, processing, and provision of data, information and knowledge in medicine and healthcare’ (authors’ translation from [ 4 ]).
Bioinformatics is a science for ‘the research, development and application of computer-based methods used to answer biomolecular and biomedical research questions. Bioinformatics mainly focusses on models and algorithms for data on the molecular and cell-biological level’ [ 5 ].
‘Epidemiology deals with the spread and the course of diseases and the underlying factors in the public. Apart from conducting research into the causes of disease, epidemiology also investigates options of prevention’ (authors’ translation from [ 6 ]).
Another discipline is data science, which is a relatively new expression used in a multitude of different contexts. Often it is meant as a global summarizing term covering all of the above mentioned fields. As there is no common agreement on what data science is and as this term does not correspond to a uniquely defined profession, this expression will not be discussed in more detail.
The self-descriptions as stated above are rather general and not necessarily complete. Therefore, we will in the following describe the specific tasks and responsibilities of biostatisticians in different important application fields in more detail. This allows us to specify what cooperation partners may (or may not) expect from a biostatistician. Furthermore, clarification of the roles of all involved parties and their successful implementation in practice will overall lead to more efficient collaborations and higher quality.
Tasks and responsibilities of biostatisticians
There are many medical areas where biostatisticians can contribute to the general research progress. These fields of application and the related biostatistical methods are not strictly separated, but there are many overlaps and a classification of the related methodology can be done in various ways. We consider in the following the important application fields of clinical trials, systematic reviews and meta-analysis, observational and complex interventional studies, and statistical genetics to highlight the tasks and responsibilities of biostatisticians working in these areas.
Biostatisticians working in the area of clinical trials
The tasks of biostatisticians in clinical trials are not limited to the analysis of the data, but there are many more responsibilities. It is a quite misguided view that biostatisticians are only required after the data has been collected. According to Lewis et al. (1996), statistical considerations are not only relevant for the analysis of data but also for the design of the trial [ 7 ]. This is not a personal view, but general consensus. It is demanded by the ethics committee and confirmed by the principle investigator and / or the sponsor when stating that the clinical trial will be conducted according to Good Clinical Practice (GCP). The corresponding guideline E6 from the International Council for Harmonisation of Technical Requirements for Pharmaceuticals for Human Use (ICH) explicitly states that statistical expertise should be utilized throughout all stages [ 8 ]. In there, it is stated in Section 5.4.1: ‘The sponsor should utilize qualified individuals (e.g. biostatisticians, clinical pharmacologists, and physicians) as appropriate, throughout all stages of the trial process, from designing the protocol and CRFs [case report forms, AZ] and planning the analyses to analyzing and preparing interim and final clinical trial reports.’ Mansmann et al. [ 9 ] provided a more specific guidance about good biometrical practice in medical research and the responsibilities of a biostatistician. In there, the responsibility of a biostatistician is described as a person participating in the planning and the execution of a study, in the dissemination of the results and in statistical refereeing. These are very general descriptions of the tasks and responsibilities of biostatisticians. In the following, we will explain the biostatistician’s mission in more detail based on the guidance on good biometrical practice [ 9 ] and on the E9 guideline from the ICH about Statistical Principles for Clinical Trials [ 10 ].
In the initial phase of a medical research project, a biostatistician should actively participate in the assessment of the relevance and the feasibility of the study. During the planning phase, the biostatistician should already be involved in the discussion of general study aspects as outlined in more detail below. It is evident that the physician must provide the framework for this. However, the biostatistician can and should point out important biostatistical issues which will have important influence on the whole construct of the study. Therefore, an important part of the biostatistician’s work is to be done long before a study can start. For example, the appropriate study population (special subgroups or healthy subjects in early phases versus large representative samples of the targeted patient population in confirmatory trials) and reasonable primary and secondary endpoints (e.g. suitable to the study aim, objectively measurable, clearly and uniquely defined) need to be identified. He also should make the physician aware of the potential problems with multiple or composite primary endpoints and with surrogate or categorised (especially dichotomized) variables. Another very important topic related to the general study design is blinding and randomisation as techniques to avoid bias. Moreover, the comparators or treatment arms must be specified and it has to be defined how they are embedded in the general study design (for example parallel or crossover). It also has to be specified the aim in whether is to show superiority or non-inferiority of the new treatment and whether interim analyses are reasonable (group sequential designs). Moreover the procedures for data capture and processing have to be discussed at this point. Only after fixing all these planning aspects, the biostatistician can provide an elaborated sample size calculation.
During the ongoing study, main tasks and responsibilities consist of biostatistical monitoring (for example as part of a data safety monitoring board) and performing interim analyses (if planned). If any modifications of the study design are urgently required during the ongoing trial (for example changes within an adaptive designs, or early stopping after an interim analysis), the biostatistician has to be involved in the discussions and decisions as otherwise the integrity of the study can be damaged.
The main data analysis is performed after all patients were recruited and fully observed. However, the statistical methods applied within the data analysis must already be specified during the planning phase within the study protocol. The study protocol should already be as detailed as possible in particular with regard to the analysis of the primary endpoint(s). In addition, the statistical analysis plan (SAP), which must be finalized before start for the data analysis, provides a document which describes all details on the primary, secondary and safety analyses. It also covers possible data transformations, applied point and interval estimators, statistical tests, subgroup analyses, and the consideration of interactions and covariates. Furthermore, the used data sets (for example intention to treat or per protocol), the handling of missing values, and a possible adjustment for multiplicity should be described and discussed. Another important issue is how the integrity of the data and the validity of the statistical software can be guaranteed.
In a last step, after the finalization of the data analysis according to the SAP, the biostatistician contributes to reporting the results in the study report as well as in the related publications submitted to medical journals. He or she is responsible for the appropriate presentation and the correct interpretation of the results.
To sum up, in clinical studies, the tasks and responsibilities of biostatisticians thus extend from the planning phase, through the execution of the study to data analysis and publication of the results. In particular, a careful study planning, in which the contribution of a biostatistician is indispensable, is essential to obtain valid study results.
Biostatisticians working in the area of systematic reviews and meta-analysis
To judge the level of evidence of medical research, different systems of evidence grading were suggested. The recent grading system from the Oxford Centre for Evidence-Based Medicine (OCEBM) defines ten evidence levels. The highest level is a systematic review of high quality studies for the therapeutic as well as for the diagnostic and prognostic context [ 11 ]. The need for such reviews results from the huge amount of articles in the medical literature, which has to be aggregated appropriately [ 12 ]. As Gopalakrishnan and Ganeshkumar describe, the aim of a systematic review is to ‘systematically search, critically appraise, and synthesize on a specific issue’ [ 13 ]. A meta-analysis, which additionally provides a quantitative summary, can be part of a systematic review, if a reasonable number of individual studies are available. The task and responsibilities of biostatisticians in this field are described in the following. As in clinical trials, the biostatistician should already be involved during the planning phase of a systematic review/meta-analysis to discuss the design aspects and the feasibility. Beside the literature search and the collection of the study data (most often not available on an individual patient level), the assessment of the study quality and the risk of bias are important topics. There are different tools for the assessment, like the GRADE approach (Grading of Recommendations, Assessment, Development and Evaluation) [ 14 ] or the QUADAS-2 tool (Quality Assessment of Diagnostic Accuracy Studies) for diagnostic meta-analyses [ 15 ]. A general description of these approaches can be found in the Cochrane Handbook [ 16 ]. The main task of biostatisticians in the field of systematic reviews is then to perform the meta-analysis itself including the calculation of weighted summary measures, creation of graphs, and performing subgroup and sensitivity analyses. As a last step, the biostatistician should again support the physicians in interpreting und publishing the results.
In summary, the tasks and responsibilities of biostatisticians in the field of systematic reviews and meta-analyses relate to the proper planning, the evaluation of the quality of the individual studies, the meta-analysis itself and the publication of the results.
Biostatisticians working in the area of observational and complex interventional studies
In observational studies, where confounding plays a major role, statistical modelling aims at incorporating, investigating, and exploiting relationships between variables using mathematical equations. Other important examples for application of the related techniques are longitudinal data measured repeatedly in time for the same subject or data with an inherent hierarchical structure, for example data of patients observed in different departments within various clinics. Valid conclusions from the analysis are only obtained if the functional relationship between the variables is correctly taken into account [ 17 ]. Another prominent task of statistical modelling is prediction, for example to forecast a future outcome of patients. Frequently, the relationship between the involved variables is complex. For example, patients may undergo several states between start of observation and outcome and the transitions between these states as well as potential competing risks have to be adequately considered (see, for example, Hansen et al. [ 18 ]). Extrapolation is another field of growing interest where techniques of statistical modelling are indispensable. This process can be defined as ‘extending information and conclusions available from studies in one or more subgroups of the patient population (source population), or in related conditions or with related medicinal products, to make inferences for another subgroup of the population (target population), or condition or product’ [ 19 ]. For example, clinical trial data for adults may be used to assist the development of treatments for children [ 20 ]. Last but not least, statistical modelling may be of help in situations where data of different origin shall be synthesized to increase evidence, for example, from randomized clinical trials, observational studies, and registries. These examples are by far not exhaustive and illustrate the wide spectrum of potential data sources and applications. It is obvious that there are direct connections to the two working areas of biostatisticians described in the preceding subsections, and consequently there are substantial overlaps in the related tasks and responsibilities. As in the other working areas considered, the biostatistician is responsible for choosing a correct and efficient analysis method that includes all relevant information. Due to the complexity of statistical models, this point is especially challenging here. Furthermore, it is the task of biostatisticians to decide whether the mandatory data required to adequately map the underlying relationships are included in the available data set, whether data quality and completeness is sufficiently high to justify a reliable analysis, and to define appropriate methods dealing with missing values. It is highly recommended to prepare an SAP not only for clinical trials (see Biostatisticians working in the area of clinical trials section) but also for analyses using methods of statistical modelling.
Again, the biostatistician is responsible not only for a proper planning and conducting of the analyses but also for appropriate interpretation and presentation of the results. The particular challenge for biostatisticians in this area is to choose appropriate statistical models for the analysis of data with a complex structure.
Biostatisticians working in the area of statistical genetics
Biostatisticians working in the fields of genetics and genomics are often the responsible persons for the final integration of multidisciplinary expertise in mathematics, statistics, genetics, epidemiology, and bioinformatics to only cite some common ingredients. Planning tasks include the design of research studies, which may pursue exploratory and/or confirmatory objectives. There exist a broad range of possible study designs which make use of well-differentiated modelling techniques. Generated data are often pre-processed by bioinformaticians before it reaches the biostatistician. Pre-processing of sequencing data, for instance, usually comprises quality control of sequenced reads, alignment to the human reference genome and markup of duplicates previously to the identification of somatic mutations and indels. Good knowledge of the limitations of applied pre-processing techniques by the statistician is often very helpful. A strong background and a deep understanding of genetics and genomics as well as an interdisciplinary thinking are a must for biostatisticians working in this area. These competences will be even more important in future. For example, emerging fields of research like Mendelian randomization where genetic variants are used as instruments to predict causality will require an even stronger interaction between statistics and genetics.
In the field of statistical genetics, tasks and responsibilities relate in particular to study planning, critical review of pre-processing, and data analysis using appropriate statistical models.
Biostatistics mainly addresses the development, implementation, and application of statistical methods in the field of medical research [ 3 ]. Therefore, an understanding of the medical background and the clinical context of the research problem they are working on is essential for biostatisticians [ 21 ]. Furthermore, a specific professional expertise is inevitable, and also soft skill competencies are very important. Regarding the professional expertise, the ICH E9 guideline states that a trial statistician should be qualified and experienced [ 10 ]. Qualification, which means biostatistical expertise, covers methodological background (mathematics, statistics, and biostatistics), biostatistical application, medical background, medical documentation, and statistical programming. The experience relates to consulting, planning, conducting and analysing medical studies. Jaki et al. [ 22 ] gave a review of training provided by existing medical statistics programmes and made recommendations for a curriculum for biostatisticians working in drug development. Regarding the soft skills of a biostatistician, some literature exists (for example [ 23 ] or [ 24 ]). Furthermore, Zapf et al. [ 1 ] summarize the professional expertise and the needed soft skills of a biostatistician according to the CanMEDS framework [ 25 ], which was developed to describe the required abilities of physician (the original abbreviation ‘Canadian Medical Education Directions for Specialists’ is no longer in use).
In this article, we did not explicitly consider the recently upcoming field of biomedical data science which is applied in many different areas of medical research such as, for example, individualized medicine, omics research, big data analysis. The tasks and responsibilities of biostatisticians working in this domain are not different from those reported above but in fact include all mentioned aspects [ 26 ].
There is evidently an overlap between the tasks and responsibilities of medical biostatisticians and neighbouring professions. However, all disciplines have different focuses. Important application fields of biostatistics are clinical studies, systematic reviews / meta-analysis, observational and complex interventional studies, and statistical genetics.
In all fields of biostatistical activities, the working environment is diverse and multi-disciplinary. Therefore, it is essential for fruitful, efficient, and high-quality collaborations to clearly define the tasks and responsibilities of the cooperating partners. In summary, the tasks and responsibilities of a biostatistician across all application areas cover active participation in a proper planning, consultation during the entire study duration, data analysis using appropriate statistical methods as well as interpretation and suitable presentation of the results in reports and publications. These tasks are similarly formulated by the ICH E6 guideline concerning good clinical practice [ 8 ].
Availability of data and materials
Not applicable.
Abbreviations
Canadian Medical Education Directions for Specialists
Case report form
Good Clinical Practice
German Association for Medical Informatics, Biometry and Epidemiology
Grading of Recommendations, Assessment, Development and Evaluation
International Biometric Society
International Council for Harmonisation of Technical Requirements for Pharmaceuticals for Human Use
Oxford Centre for Evidence-Based Medicine
Quality Assessment of Diagnostic Accuracy Studies
Statistical analysis plan
Zapf A, Hübner M, Rauch G, Kieser M. What makes a biostatistician? Stat Med. 2018;38(4):695–701.
Article Google Scholar
Homepage of the International Biometric Society. http://www.biometricsociety.org/about/definition-of-biometrics/ . Accessed 11 Nov 2019.
Homepage of the German Society of Medical Informatics, Biometry and Epidemiology (GMDS), Section Medical Biometry. http://www.gmds.de/fachbereiche/biometrie/index.php . Accessed 11 Nov 2019.
Homepage of the German Society of Medical Informatics, Biometry and Epidemiology (GMDS), Section Medical Informatics. https://gmds.de/aktivitaeten/medizinische-informatik/ . Accessed 11 Nov 2019.
Homepage from the professional group bioinformatics (FaBI). https://www.bioinformatik.de/en/bioinformatics.html . Accessed 11 Nov 2019.
Homepage of the German Society of Medical Informatics, Biometry and Epidemiology (GMDS), Section Epidemiology. https://gmds.de/aktivitaeten/epidemiologie/ . Access 11 Nov 2019.
Lewis JA. Editorial: statistics and statisticians in the regulation of medicines. J R Stat Soc Ser A. 1996;159(3):359–62.
International Conference on Harmonisation of Technical Requirements for Registration of Pharmaceuticals for Human Use (1996). Guideline for good clinical practice E6 (R2). https://www.ema.europa.eu/en/documents/scientific-guideline/ich-e-6-r2-guideline-good-clinical-practice-step-5_en.pdf . Access 11 Nov 2019.
Google Scholar
Mansmann U, Jensen K, Dirschedl P. Good biometrical practice in medical research - guidelines and recommendations. Informatik, Biometrie und Epidemiologie in Medizin und Biologie. 2004;35:63–71.
International Conference on Harmonisation of Technical Requirements for Registration of Pharmaceuticals for Human Use (1998). Statistical principles for clinical trials E9. https://www.ema.europa.eu/en/documents/scientific-guideline/ich-e-9-statistical-principles-clinical-trials-step-5_en.pdf . Accessed 11 Nov 2019.
OCEBM. The Oxford 2011 levels of evidence: Oxford Centre for Evidence-Based Medicine; 2011. http://www.cebm.net/oxford-centre-evidence-based-medicine-levels-evidence-march-2009/ . Accessed 11 Nov 2019
Mulrow CD. Systematic reviews: rationale for systematic reviews. BMJ. 1994;309:597–9.
Article CAS Google Scholar
Gopalakrishnan S, Ganeshkumar P. Systematic reviews and meta-analysis: understanding the best evidence in primary healthcare. J Fam Med Prim Care. 2013;2(1):9–14.
Schünemann H, Brożek J, Guyatt G, Oxman A, editors. GRADE handbook for grading quality of evidence and strength of recommendations. Updated October 2013: The GRADE Working Group; 2013. Available from https://gdt.gradepro.org/app/handbook/handbook.html . Accessed 11 Nov 2019
Whiting PF, Rutjes AWS, Westwood ME, Mallett S, Deeks JJ, Reitsma JB, Leeflang MMG, Sterne JAC, Bossuyt PMM, the QUADAS-2 Group. QUADAS-2: a revised tool for the quality assessment of diagnostic accuracy studies. Ann Intern Med. 2011;155:529–36.
Higgins JPT, Green S, editors. Cochrane handbook for systematic reviews of interventions version 5.1.0 [updated March 2011]: The Cochrane Collaboration; 2011. Available from http://handbook.cochrane.org . Accessed 11 Nov 2019
Snijders AB, Bosker RJ. Multilevel analysis - an introduction to basic and advanced multilevel modeling. London: SAGE Publications; 1999.
Hansen BE, Thorogood J, Hermans J, Ploeg RJ, van Bockel JH, van Houwelingen JC. Multistate modelling of liver transplantation data. Stat Med. 1994;13:2517–29.
European Medicines Agency (2012). Concept paper on extrapolation of efficacy and safety in medicine development - EMA/129698/2012. http://www.ema.europa.eu/docs/en_GB/document_library/Scientific_guideline/2013/04/WC500142358.pdf . Accessed 11 Nov 2019.
Wadsworth I, Hampson LV, Jaki T. Extrapolation of efficacy and other data to support the development of new medicines for children: a systematic review of methods. Stat Meth Med Res. 2018;27(2):398–413.
Simon R. Challenges for biometry in 21st century oncology. In: Matsui S, Crowley J, editors. Frontiers of biostatistical methods and applications in clinical oncology. Singapore: Springer; 2017. Available from https://link.springer.com/chapter/10.1007%2F978-981-10-0126-0_1 . Accessed 25 Nov 2019.
Jaki T, Gordon A, Forster P, Bijnens L, Bornkamp B, Brannath W, Fontana R, Gasparini M, Hampson LV, Jacobs T, Jones B, Paoletti X, Posch M, Titman A, Vonk R, Koenig F. A proposal for a new PhD level curriculum on quantitative methods for drug development. Pharm Stat. 2018;17:593–606.
CAS PubMed PubMed Central Google Scholar
Lewis T. Statisticians in the pharmaceutical industry. In: Stonier PD, editor. Discovering new medicines. Chichester: Wiley; 1994. p. 153–63.
Chuang-Stein C, Bain R, Branson M, Burton C, Hoseyni C, Rockhold FW, Ruberg SJ, Zhang J. Statisticians in the pharmaceutical industry: the 21st century. Stat Biopharm Res. 2010;2(2):145–52.
Royal College of Physicians and Surgeons of Canada. CanMEDS: better standards, better physician, better care. http://www.royalcollege.ca/rcsite/canmeds/canmeds-framework-e . Accessed 11 Nov 2019.
Alarcón-Soto Y, Espasandín-Domínguez J, Guler I, Conde-Amboage M, Gude-Sampedro F, Langohr K, Cadarso-Suárez C, Gómez-Melis G (2019) Data Science in Biomedicine. arXiv:1909.04486v1. Available from https://arxiv.org/abs/1909.04486v1 . Accessed 25 Nov 2019.
Download references
Acknowledgements
There was no funding for this project.
Author information
Authors and affiliations.
Department of Medical Biometry and Epidemiology, University Medical Center Hamburg-Eppendorf, Martinistr. 52, 20246, Hamburg, Germany
Antonia Zapf
Charité - Universitätsmedizin Berlin, corporate member of Freie Universität Berlin, Humboldt-Universität zu Berlin, and Berlin Institute of Health, Institute of Biometry and Clinical Epidemiology, Charitéplatz 1, 10117, Berlin, Germany
Geraldine Rauch
Institute of Medical Biometry and Informatics, Heidelberg University Hospital, Im Neuenheimer Feld 130.3, 69120, Heidelberg, Germany
Meinhard Kieser
You can also search for this author in PubMed Google Scholar
Contributions
AZ drafted the work and all authors substantively revised it. All authors approved the final version and agreed both to be personally accountable for the author’s own contributions and to ensure that questions related to the accuracy or integrity of any part of the work are appropriately investigated, resolved, and the resolution documented in the literature.
Corresponding author
Correspondence to Antonia Zapf .
Ethics declarations
Ethics approval and consent to participate, consent for publication, competing interests.
The authors declare that they have no competing interests.
Additional information
Publisher’s note.
Springer Nature remains neutral with regard to jurisdictional claims in published maps and institutional affiliations.
Rights and permissions
Open Access This article is distributed under the terms of the Creative Commons Attribution 4.0 International License ( http://creativecommons.org/licenses/by/4.0/ ), which permits unrestricted use, distribution, and reproduction in any medium, provided you give appropriate credit to the original author(s) and the source, provide a link to the Creative Commons license, and indicate if changes were made. The Creative Commons Public Domain Dedication waiver ( http://creativecommons.org/publicdomain/zero/1.0/ ) applies to the data made available in this article, unless otherwise stated.
Reprints and permissions
About this article
Cite this article.
Zapf, A., Rauch, G. & Kieser, M. Why do you need a biostatistician?. BMC Med Res Methodol 20 , 23 (2020). https://doi.org/10.1186/s12874-020-0916-4
Download citation
Received : 21 March 2019
Accepted : 28 January 2020
Published : 05 February 2020
DOI : https://doi.org/10.1186/s12874-020-0916-4
Share this article
Anyone you share the following link with will be able to read this content:
Sorry, a shareable link is not currently available for this article.
Provided by the Springer Nature SharedIt content-sharing initiative
- Medical research
- Biostatistician
- Responsibilities
BMC Medical Research Methodology
ISSN: 1471-2288
- General enquiries: [email protected]
Information
- Author Services
Initiatives
You are accessing a machine-readable page. In order to be human-readable, please install an RSS reader.
All articles published by MDPI are made immediately available worldwide under an open access license. No special permission is required to reuse all or part of the article published by MDPI, including figures and tables. For articles published under an open access Creative Common CC BY license, any part of the article may be reused without permission provided that the original article is clearly cited. For more information, please refer to https://www.mdpi.com/openaccess .
Feature papers represent the most advanced research with significant potential for high impact in the field. A Feature Paper should be a substantial original Article that involves several techniques or approaches, provides an outlook for future research directions and describes possible research applications.
Feature papers are submitted upon individual invitation or recommendation by the scientific editors and must receive positive feedback from the reviewers.
Editor’s Choice articles are based on recommendations by the scientific editors of MDPI journals from around the world. Editors select a small number of articles recently published in the journal that they believe will be particularly interesting to readers, or important in the respective research area. The aim is to provide a snapshot of some of the most exciting work published in the various research areas of the journal.
Original Submission Date Received: .
- Active Journals
- Find a Journal
- Proceedings Series
- For Authors
- For Reviewers
- For Editors
- For Librarians
- For Publishers
- For Societies
- For Conference Organizers
- Open Access Policy
- Institutional Open Access Program
- Special Issues Guidelines
- Editorial Process
- Research and Publication Ethics
- Article Processing Charges
- Testimonials
- Preprints.org
- SciProfiles
- Encyclopedia
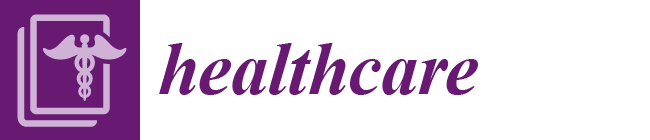
Journal Menu
- Healthcare Home
- Aims & Scope
- Editorial Board
- Reviewer Board
- Topical Advisory Panel
- Instructions for Authors
- Special Issues
- Sections & Collections
- Article Processing Charge
- Indexing & Archiving
- Editor’s Choice Articles
- Most Cited & Viewed
- Journal Statistics
- Journal History
- Journal Awards
- Society Collaborations
- Conferences
- Editorial Office
Journal Browser
- arrow_forward_ios Forthcoming issue arrow_forward_ios Current issue
- Vol. 12 (2024)
- Vol. 11 (2023)
- Vol. 10 (2022)
- Vol. 9 (2021)
- Vol. 8 (2020)
- Vol. 7 (2019)
- Vol. 6 (2018)
- Vol. 5 (2017)
- Vol. 4 (2016)
- Vol. 3 (2015)
- Vol. 2 (2014)
- Vol. 1 (2013)
Find support for a specific problem in the support section of our website.
Please let us know what you think of our products and services.
Visit our dedicated information section to learn more about MDPI.
The Role of Biostatistics in Public Health
- Print Special Issue Flyer
Special Issue Editors
Special issue information, benefits of publishing in a special issue, published papers.
A special issue of Healthcare (ISSN 2227-9032). This special issue belongs to the section " Health Informatics and Big Data ".
Deadline for manuscript submissions: closed (30 April 2024) | Viewed by 877
Share This Special Issue
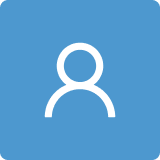
Dear Colleagues,
Biostatistics is an indispensable field within public health that utilizes statistical methods to investigate and understand health-related phenomena. Biostatistics in public health aims to provide quantitative tools and methods for studying and improving population health. It involves applying statistical techniques to collect, analyze, and interpret health-related data to derive meaningful conclusions and guide evidence-based decision making. Biostatistics plays a vital role in several domains of public health, including disease surveillance, epidemiology, clinical trials, health services research, environmental health, and policy evaluation. Its scope of application ranges from analyzing disease patterns and identifying risk factors to evaluating the effectiveness of interventions and assessing healthcare outcomes. Biostatistics enables public health professionals to quantify health disparities, monitor population health indicators, assess the impact of public health programs, and inform policy development. By utilizing biostatistical methods, public health practitioners can effectively address public health challenges, allocate resources efficiently, and promote the well-being of communities. We aim to understand the recent developments in biostatics and how they could be relevantly utilized for improvements in public health interventions, initiatives, and policy changes. We cordially invite insightful publications on the topic.
Dr. Venkataraghavan Ramamoorthy Dr. Mayur Doke Dr. Muni Rubens Guest Editors
Manuscripts should be submitted online at www.mdpi.com by registering and logging in to this website . Once you are registered, click here to go to the submission form . Manuscripts can be submitted until the deadline. All submissions that pass pre-check are peer-reviewed. Accepted papers will be published continuously in the journal (as soon as accepted) and will be listed together on the special issue website. Research articles, review articles as well as short communications are invited. For planned papers, a title and short abstract (about 100 words) can be sent to the Editorial Office for announcement on this website.
Submitted manuscripts should not have been published previously, nor be under consideration for publication elsewhere (except conference proceedings papers). All manuscripts are thoroughly refereed through a single-blind peer-review process. A guide for authors and other relevant information for submission of manuscripts is available on the Instructions for Authors page. Healthcare is an international peer-reviewed open access semimonthly journal published by MDPI.
Please visit the Instructions for Authors page before submitting a manuscript. The Article Processing Charge (APC) for publication in this open access journal is 2700 CHF (Swiss Francs). Submitted papers should be well formatted and use good English. Authors may use MDPI's English editing service prior to publication or during author revisions.
- public health
- biostatistics
- population health
- environmental health
- social determinants of health
- advanced statistical modeling
- Ease of navigation: Grouping papers by topic helps scholars navigate broad scope journals more efficiently.
- Greater discoverability: Special Issues support the reach and impact of scientific research. Articles in Special Issues are more discoverable and cited more frequently.
- Expansion of research network: Special Issues facilitate connections among authors, fostering scientific collaborations.
- External promotion: Articles in Special Issues are often promoted through the journal's social media, increasing their visibility.
- e-Book format: Special Issues with more than 10 articles can be published as dedicated e-books, ensuring wide and rapid dissemination.
Further information on MDPI's Special Issue polices can be found here .
Further Information
Mdpi initiatives, follow mdpi.
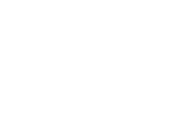
Subscribe to receive issue release notifications and newsletters from MDPI journals

Initiatives
Our community.
- Education & Admissions
Division of Biostatistics and Population Health
Pioneering cutting-edge research and employing advanced statistical methodologies to drive innovation and profoundly influence healthcare and public health outcomes.
The Biostatistics and Population Health Section of the Biomedical Informatics Department is committed to harnessing the power of data to profoundly impact healthcare and public health. Through rigorous research and collaboration, our team explores the intricate realms of biostatistics and population health to drive innovation, expand knowledge, and enhance outcomes.
Aspire to be a leading Biostatistics and Population Health program, driving innovation, advancing knowledge, and profoundly impacting healthcare and public health outcomes through advanced statistical methodologies and interdisciplinary collaboration.
Our Mission
- Research Excellence: Advance multidisciplinary biostatistics and population health research using state-of-the-art quantitative methods.
- Pioneering Progress: Lead groundbreaking advancements in biomedical and population health research.
- Cultivating Leaders: Shape the future leaders in biostatistics and population health science, ensuring a legacy of expertise and dedication.
Our Faculty
Joe McElroy
John Bridges
Mohamed Elsaid
Stacey Culp
Xiaoli Zhang
Fode Tounkara
Hyoshin Kim
Naleef Fareed
Dongjun Chung
Saurabh Rahurkar
From the Front Row: Using biostatistics and P-value in public health research
Published on March 20, 2023
Joe Cavanaugh , professor and head of the University of Iowa Department of Biostatistics is this week’s guest. He chats with Amy and Anya about the central role that biostatistics plays in public health and medical research and explains the concept of P-value and its use in biostatistics.
Find our previous episodes on Spotify , Apple Podcasts , and SoundCloud .
Hello, everyone, and welcome back to From the Front Row. Behind a lot of public health evidence is numbers, and biostatisticians are the ones behind the scene, interpreting those numbers every day.
One staple of many biostatistical tests is a number called the p-value. Most of us are taught that if the p-value is smaller than 0.05, you found something statistically significant. And if it’s larger than 0.05, your numbers were probably due to random chance. Because the p-value is used so widely in statistics, this concept has a huge impact on evidence-based decisions and research impacted. But it turns out there is a lot of controversy behind the p-value. We have Dr. Cavanaugh, one of our biostatistics professors, on the show today to talk with us about just that.
Dr. Cavanaugh has published more than 160 peer-reviewed papers, and his research contribution span a wide range of fields, from cardiology to health services utilization to sports medicine to infectious disease, just to name a few. Outside of that, he’s an elected fellow of the American Statistical Association, an elected member of the International Statistical Institute, and has received several awards for teaching and mentoring. We’re glad to have him at the college and we’re very excited to have him on the show today to break down p-values for our audience.
I’m Amy Wu, joined today by Anya Morozov. If it’s your first time with us, welcome. We’re a student-run podcast that talks about major issues in public health and how they are relevant to anyone, both in and outside the field of public health. Welcome to the show, Dr. Cavanaugh.
Joe Cavanaugh:
It’s great to be here. Thank you for inviting me.
Of course. Before we get into the topic of today’s episode, can you tell us a little bit about your background and what folks can do with a biostatistics degree?
Yeah, absolutely. Most biostatisticians, they followed a rather non-linear path to the discipline. I went to a small STEM college as an undergraduate, Montana Tech in my hometown of Butte, Montana, and I received bachelor’s degrees in computer science and mathematics. And I found that I enjoyed computing, but I really wanted to program my own ideas.
I had a couple of programming jobs, one at a utility company and one at a national lab, but I was programming the ideas of engineers and physicists. And to me, the creative aspect of computing is the development of the algorithms. And as far as my mathematics degree goes, I really liked my math courses, but I realized I liked the more applied side of math.
So one of my undergraduate mentors suggested that I consider work in statistics, and I followed his advice and received my PhD in statistics from the University of California Davis. I spent the first 10 years of my academic career in a department of statistics at the University of Missouri. And then in 2003, I slightly changed course from stat to biostat, and moved here to the University of Iowa. So this is my 20th year as a Hawkeye.
What I liked about biostatistics is that it allows you to use your quantitative skills to help solve important practical problems. And the field has always been one where demand greatly exceeds supply, so the job market is excellent. And as far as the types of jobs that our graduates pursue, it’s pretty wide-ranging. We have some that work at pharmaceutical companies, biomedical research facilities, such as the Mayo Clinic, Fred Hutchinson Cancer Center, Memorial Sloan Kettering Cancer Center, high tech companies like Facebook and Google, and government agencies like the FDA, the NIH, and the CDC.
Awesome. Thanks for sharing. As a biostatistics student, I’m very excited to hear that there are a lot of job prospects out there.
Absolutely.
And I do enjoy the breadth of work that I have the potential of going into.
Anya Morozov:
Yeah, so we mentioned a little bit in the introduction about p-values. If they’re smaller than 0.05, generally you’ve found something statistically significant, and if they’re larger than 0.05, your findings might be due to random chance. But could you explain a little more and remind us what a p-value is for non-statisticians?
Yeah, absolutely. So in many statistical applications, you’re going to build a statistical model to investigate the possible association between an explanatory variable and an outcome of interest. So an example might be to investigate the association between influenza and flu vaccinations to determine the extent to which your risk is reduced if you’re vaccinated.
So to test this association, you formulate two hypotheses. There’s a null hypothesis, which assumes that the association or the so-called effect doesn’t exist. And then there’s an alternative hypothesis, which assumes that the association or effect does exist and is potentially important. So the p-value, it’s computed by assuming hypothetically that the null hypothesis is true, and then finding the probability of obtaining data similar to the data observed in your study under that assumption. So if this probability then is small, this might cause you to doubt the veracity of the null hypothesis and to view the alternative hypothesis as more credible.
So in more succinct terms, you can view the p-value perhaps as a type of conditional probability. It’s trying to address the question just what is the probability of the data, given that the null hypothesis is true. And to some extent, the p-value is founded on the notion of a proof by contradiction, because you’re assuming that the null hypothesis is true, and then you’re trying to determine whether or not the data discredits that assumption by getting a low probability.
Yeah, so just also for the non-statisticians out there, a lower p-value would basically make you favor the alternative hypothesis over the null?
That’s correct. Yeah. And as you alluded to earlier, Amy, a common practice, which I’ll comment about in a bit, is to compare the p-value to a level of significance that is set at 0.05. 0.05 probability. So if the p-value is less than that, often you declare the result as being statistically significant. And if it’s greater than that, then you say that you don’t have the burden of proof met in order to reject the null in favor of the alternative. But that practice is problematic, so I think we’re going to get to that issue in just a bit.
Yeah, of course. So on that subject, could you give us a brief history on the controversy surrounding p-values? Or example, last semester in your distinguished faculty lecture, you mentioned that the Basic and Applied Social Psychology journal decided to ban all p-values in 2015. So for non-statisticians, can you explain why they would do this, what are some of the pitfalls of p-values, et cetera?
Yeah, certainly. There’s a few different questions there that I’ll try to address, Amy. To begin a bit of history about the p-value, it’s been around for about a century. It’s often credited to Karl Pearson, who introduced the concept in 1925. It was introduced in the context of hypothesis testing, which is a paradigm designed for very specific types of studies, namely randomized experiments. So in biostatistics, clinical trials would be an example of a randomized experiment.
But as it turns out, since then, p-values have become much more pervasively used and often, I would argue, in context for which they were not designed. And they’re often misapplied and misinterpreted and used to justify conclusions that really are not warranted.
So over the last decade or two, scientists have become more concerned with reproducibility. There’s been a lot of backlash against p-values, and some scientists have suggested that the best way of dealing with the problems caused by the p-value is to just banish it altogether. But from my perspective, that is neither a practical nor an ideal solution.
Having said that, there are many problems with the p-value. One of the most significant issues is based on the practice that you alluded to earlier, comparing the p-value to the 0.05 level of significance in deciding whether to reject the null hypothesis and declare the existence of an effect if the p-value is less than 0.05. Now, the reason this is a problem is that the p-value can assume any value between zero and one. So it’s a continuous measure that should be evaluated on a spectrum of evidence.
To illustrate that idea a bit further, there’s very little practical difference between a p-value of 0.04 and 0.06. So making completely different decisions based on these two p-values is not rational. To say that if you have a p-value of 0.04, that the effect is significant, that you should pay attention to it. But if you have a p-value of 0.06, you haven’t met the burden of proof, and therefore, you shouldn’t doubt the null hypothesis.
Now, one of the problems that has resulted from this binary decision-making, it’s a practice known as p-hacking. That’s the practice of repeatedly analyzing data using different analytic techniques to obtain a p-value that is less than 0.05. So you might come up with a variety of different models, and the first time you get a p-value for the effect of interest of .14, you’re not happy with that. You reformulate the model, you get a p-value of 0.08, and you’re still not happy. Reformulate it again, you get a p-value of 0.04, and then you say, “Okay, I finally achieved the burden of proof, that level of significance.” P-hacking is one of the reasons that many studies are not reproducible.
So another problem with the p-value is that it allows you to assess statistical significance, but not clinical or practical significance. To explain the difference between those two ideas, suppose that we have a treatment for hypertension that’s designed to reduce systolic blood pressure, so you conduct a clinical trial to try to assess the efficacy of the treatment. Now, the result is statistically significant if you can establish that the mean change in blood pressure is non-zero, but the result is clinically significant if the mean change is substantial enough to impact a person’s health. You could argue that that’s a higher bar to attain than statistical significance.
So as it turns out, one can obtain a small p-value that leads to statistical significance if a small change is estimated with a high degree of accuracy. In that setting, you’re quite sure that the change is non-zero, but you’re also quite sure that the change is minor. And a small p-value can arise when an effect is accurately estimated, but it’s estimated to be small. Small enough that it probably is not clinically important or practically meaningful.
So how can you get around that problem? Well, confidence intervals or Bayesian credible intervals, they’re more informative because they provide a range of plausible values for the effect of interest. And the center of the interval, it represents what you could think of as the most likely value for the effect. It’s often the point estimate, the so-called point estimate of the effect. And in the width of the interval reflects the accuracy of the effect estimate. And both the point estimate and its measure of accuracy are very important in coming up with an overall assessment of the effect of interest.
So the problem with the p-value is it’s taking these two important pieces of information, the point estimate and the measure of accuracy, often called the standard error, and conflating these two quantities by combining them into one number. And once you’ve collapsed those two quantities into one number, there’s no way of separating them out and determining what the two quantities are individually.
Yeah, so I had one follow-up question. If you could briefly explain what reproducibility is in the context of p-values and p-hacking.
Yeah, absolutely. A study is reproducible if you can conduct a very similar study with the same outcome of interest, the same explanatory factor of interest, and get a similar result. And because all studies are inherently flawed to various degrees, reproducibility is very important because it’s the aggregation of evidence over a variety of different studies that starts giving us a definitive understanding of a particular phenomenon.
So for instance, we now widely accept the fact that there are a lot of bad health conditions that are a result of smoking cigarettes. But 50 years ago, that was not widely known. And all cigarette smoking studies are observational. You can’t do a randomized experiment where you break subjects into two groups and say, “You’re going to smoke, and you’re not going to smoke.” But because we have found over time that the ill effects of smoking are reproducible in different observational studies, that preponderance of evidence over a variety of different studies has led us to the conclusion that you shouldn’t smoke.
So the problem that has resulted with reproducibility in some studies is you’ll have a paper, say, that’s published, and it declares an effect as being statistically significant, and then the authors will say, “This is a conclusive result.” And other authors may say, “Well, I think that this phenomenon is important enough to investigate in a separate study with a different database, different population of interest,” and they may find no evidence at all of the same effect. And you could imagine that one of the reasons why that could happen is if you have authors that are repeatedly analyzing the data in order to get a p-value that’s less than 0.05 and then they publish the result once they find the right analysis that will give them that result, then it’s going to lead to a study that is not reproducible. So that has become a major issue recently in science where you have a study that is investigating a very important phenomenon. Other investigators want to see if they can replicate the result, and they’re unable to do so.
Yeah. Well, thanks for extending on reproducibility. I also just wanted to retouch on clinical significance versus statistical significance. So what I’m hearing is that results can be statistically significant but not clinically relevant or important, say, to medical professionals, for example, or they could be both. So you’re kind of saying that p-values kind of can only speak to the former, where they’re only statistically significant and not-
That’s exactly correct, Amy. So you could imagine a situation where you have, say, a small effect where any physician would take a look at the effect and say, “Not worth taking that drug, because if it’s going to have such a minor impact on your health, that it’s just not worth it.” And you could imagine another study where you have a large effect, say in the hypertension example, where you have a drug that could potentially reduce your systolic blood pressure by as much as 20 to 30 points, which would be a major game changer.
Now, in either of those settings, if you have a highly accurate estimate, you’ll get a very small p-value, and so you’ll be able to establish statistical significance. In both of those settings, you can say fairly conclusively that the effect is non-zero. But in one case, it’s non-zero, but it’s very small and very close to zero. And in the other case, it’s non-zero and it’s substantial in magnitude. In the latter setting, you would have clinical or practical significance, whereas in the former setting, you would not. But in both of those settings, you would have statistical significance.
So it’s almost like the p-value is creating this incentive for folks in research to really strive for statistical significance, and the clinical or public health significance can become secondary to that if you’re focused so much on whether or not you’re hitting that 0.05 or whatever p-value you’ve set as statistically significant.
Yeah, that’s exactly right, Anya. And I will say that part of the problem is you’re incentivized, through the publication process, to have statistically significant results. So another problem that results in a lack of reproducibility is called publication bias. So there’s the feeling among editors of journals and reviewers of articles that if you don’t establish an effect that is statistically significant, then we haven’t learned anything. And yet a null result, a null finding can often be as informative or even more informative than a result that is statistically significant.
But if the journals are going to practice this tendency where they’re going to favor results that report statistically significant findings and ignore results that don’t report such findings, then basically you’re getting a very biased representation of a particular phenomenon. So you might have, for instance, a subtle effect. And in some studies, it’s showing up as statistically significant. In other studies, it’s not. And yet the only studies that are being published are those where you have statistical significance. So if you search the literature, you think, “Well, this effect is showing up consistently in a large variety of different studies,” because you haven’t seen all of the studies where it hasn’t shown up as being significant, due to the fact that those studies are not published.
So we just talked a little bit about the potential pitfalls of p-values. In your aforementioned lecture, you mentioned that the p-value is not going away. So are p-values still relevant?
Yeah, I definitely think that they are, Amy. They still have relevance in the settings for which they were designed, hypothesis testing in the context of randomized experiments such as a clinical trial that’s performed to assess the efficacy of a new drug by comparison to a placebo. But there’s a saying that you might have heard that goes along the following lines, when all you have is a hammer, every problem looks like a nail. And unfortunately, researchers often treat the p-value like a hammer and use it as a tool rather indiscriminately for problems where it is contextually inappropriate.
So from my perspective, p-values still have a place in statistics, a very prominent place, but they should probably be used much less pervasively. And in settings where a p-value is inappropriate, there are other inferential tools that are available. They’re not perhaps as widely known, but as statisticians, we should promote the use of those tools rather than always producing p-values because we think that is what is expected.
Yeah, so along those lines, p-values can be useful in some settings, but not all. You have done some work on one alternative to the p-value, called the discrepancy comparison probability, or DCP. Can you tell us a little bit about that alternative?
Yeah, yeah, I’d be happy to. To provide a little bit of background, the p-value is often used to test for the existence of an effect in the context of a statistical model. That’s how we typically see p-values used in research, especially observational studies.
Now, the model under the alternative hypothesis, it contains the effect of interest, and the model under the null hypothesis does not. And the model often contains other variables of interest as well. So as an example, think of a prognostic model that is formulated to predict the onset of heart disease for middle-aged individuals. Now, the effect of interest might be a measure of physical activity, because we know that if you’re physically active, that that should reduce your risk of future heart disease. But you’ll probably want to include other variables in the model that could impact this relationship, such as age, BMI, cholesterol level, blood pressure, sex, ethnicity.
Now, if the p-value is small, we reject the null model in favor of the alternative model, and we claim that there is an effect. But the problem in this context is that the p-value can only be defined and interpreted under the assumption that one or the other model represents truth, because that’s the hypothesis testing paradigm. You have a null hypothesis, in this case, a null model, an alternative hypothesis, in this case, an alternative model, and both represent incompatible states of nature. One represents the truth, and one does not. That’s the hypothesis testing paradigm, and it’s up to you to try to use the data in order to try to decide which of those two competing states of nature is the most credible.
So where do you run into problems when you’re comparing two models in a hypothesis testing setting? Well, models, they’re only approximations to reality. They don’t represent reality. So the entire paradigm of hypothesis testing and p-values is really misaligned with statistical modeling. There’s a quote that is a favorite among statisticians that is attributed to George Box, who was a very famous statistician. It goes like this, “All models are wrong, some are useful.” So to unpack that quote, all models are wrong because all models are approximations to reality. They don’t represent reality. Some are useful because some are sufficiently accurate approximations for the inferential purpose at hand.
So the discrepancy comparison probability, or the DCP, it represents the probability that the null model is closer to the truth, to reality, than the alternative model, or that the null model is less discrepant from the truth or reality than the alternative model. And importantly, the DCP, it doesn’t assume that either model represents the truth. So basically assessing the probability that the null model is a better approximation to the truth than the alternative model.
Now, like the p-value, the estimated DCP is going to be close to zero if the alternative model is markedly better than the null model. But unlike the p-value, the estimated DCP will be close to one if the null model is markedly better than the alternative model. So it tells you something if it’s small, if it’s close to zero. And it tells you something if it’s close to one, if it’s large.
So that actually points out another flaw with the p-value, and that is that a small p-value, it represents evidence against the null hypothesis, in favor of the alternative hypothesis. But as it turns out, a large p-value really doesn’t tell you anything, and that it represents an absence of evidence rather than evidence in favor of the null hypothesis. And you’ve heard that adage, absence of evidence is not evidence of absence. But often when researchers will come up with a large p-value, 0.5, they’ll say, “Well, this provides evidence that the null hypothesis is credible,” and that’s not the case.
But based on the way that the DCP is set up, it will lean towards one if the null model is a better approximation to reality than the alternative model, and it will lean towards zero if the alternative is a better approximation to reality. So it does provide evidence in support of either model, but again, only thinking of how well the models approximate the truth, not by trying to think that either model represents the truth.
So along the lines of the holistic philosophy behind the DCP, with these non-binary or interpretations along the spectrum or continuum, could you talk about the role of biostatisticians in interpreting these complex and nuanced medical or public health type problems?
Yeah, absolutely. One point that I’d like to make is that statistical methods require advanced training. And part of the problem with the misuse of p-values is that sophisticated analyses are often conducted by researchers without the appropriate training. So Amy, you’re working on a graduate degree in biostatistics, and Anya, you’re working on a graduate degree in epidemiology, where you have to learn a lot of biostatistics.
So epidemiologists who are well trained in modern statistical methods, and biostatisticians, they’re more aware of what a p-value can tell you and what it cannot tell you. Also, they’re likely more aware of alternative measures of statistical evidence that have been introduced during the past few decades. So if you’re working on a particular study and you’re convinced that a p-value is not the best measure of statistical evidence, that you might be aware, if you have training in more advanced methods, of some of these alternatives.
Now, having said this, scientific paradigms are hard to change. And because p-value are so predominant in biomedical and public health research, it will take time to change the culture so that p-values are used more sparingly and in context where they’re more appropriate. But from my perspective, biostatisticians and statisticians, they really need to be willing to push the envelope and use some of these more modern and sophisticated inferential tools, such as say, Bayesian posterior probabilities, Bayes factors, likelihood ratio, statistics, information criteria, such as the Akaike information criterion, the Bayesian information criterion.
Now, these phrases probably sound unfamiliar to students who’ve had an introductory course in statistics but haven’t gone beyond that course. And in that introductory course, they’re likely to remember two constructs, the p-value and the confidence interval. But if you’ve had more advanced training in biostatistics, you’re likely more aware of some of these tools, and there may be settings that arise in your research where you feel like you should advocate in favor of using something other than the p-value in order to address the inferential question of interest. And if we, as statisticians, always default to the p-value because we think that’s what editors of journals and referees of articles are going to expect, then the culture is never going to change.
Well, I’m glad you’re here at the college and just generally kind of advocating for more nuance in how we interpret results of studies. I also think it kind of shows the importance of communication in any field, even biostatistics. I feel like that’s one where traditionally, I think maybe communication isn’t as important to being able to do biostatistics, but you have to be able to communicate. If you are proposing that change and not using the p-value to a lab full of people who maybe aren’t biostatisticians, you have to be able to talk about these other methods and why there may be a better fit.
That’s very well said, Anya. In fact, I will often say to our students that there is this perception when you’re in graduate school that being really good at math or being really good at computing, that those are the most important skills for a biostatistician. And they are, without question, important skills, and those are the types of skills that will often allow you to get good grades in your coursework.
But the most important skill, from my perspective, is to have very good oral and written communication skills for exactly the reason that you mentioned, because everything that we do is collaborative, and you don’t want to talk to your collaborators as though they have the same background that you do. A physician is not going to talk to a patient the same way that they would talk to another physician with expertise in that area.
So it’s a real art for an epidemiologist or a biostatistician to be able to distill the essence of an inferential result and communicate what it can tell you and what it can’t tell you in such a way that your collaborators understand what you’ve done with their data and what conclusions are warranted, what conclusions are not warranted. And it’s not easy to do. It takes a lifetime of practice. So I completely agree with you. And I think perhaps if we all communicated better as biostatisticians, then perhaps that would be a step in the right direction as far as using more appropriate inferential tools. Because when a setting does arise where you feel that p-value isn’t appropriate, you can articulate the reasons why.
Very well said. Now we’ll move on to our last question on the show. This is one that we ask to all of our guests. It can be related to p-values, biostatistics, or just everyday life. But what is one thing you thought you knew but were later wrong about?
Yeah. Well, this was a fun question to think about, Anya, and so I wanted to provide an academic answer and a completely non-academic answer. I’ll start with the academic answer. And this does tie in with our discussion about p-values. I think that learning about the politics and the messiness of publishing scientific research was a real eye-opener for me. When I was young, I believed that good research was published, and bad research was not. Now I realized that most research is imperfect, and that the evaluation of research is highly subjective. Also, to publish research, you often need to sacrifice idealism for pragmatism. But I would claim that once you understand the rules of the game, and that includes the problems that are endemic to the publication process, you realize that you can still conduct and publish good work and do so with ethics and integrity, but you’ll often need to battle to defend your principles.
So that’s one thing that is academic, related to research, that I thought I knew, had sort of an idealistic oversimplified view, and then later found out that I was misguided.
So here’s my non-academic answer, and this just occurred to me this morning. I’m a big fan of football, both college and the NFL. My favorite college team is, of course, the Hawkeyes, and my favorite NFL team is the Buffalo Bills. The Buffalo Bills were really bad for a long time, and in 2018, they drafted a new quarterback, Josh Allen, and I thought they’d made a horrible mistake, that they’d wasted this high first round draft pick on someone who would be a complete bust. And now, as it turns out, Josh Allen is one of the best quarterbacks in the NFL, and the Bills are actually a good team. So I’ve never been so happy to be so wrong. That’s my fun answer.
All right. Well, thanks, Dr. Cavanaugh, for joining us for this episode. It was very helpful to hear you explain p-values, their history, their pitfalls, their future, and also novel biostatistical methods, like the DCP, which you have worked on. And we’re very lucky that you’ve been able to explain it from a biostatistician’s perspective to our non-statistician audience. So yeah, thank you.
Thank you for having me, Amy and Anya. It’s been a pleasure.
That’s it for our episode this week. Big thanks to Dr. Joe Cavanaugh for joining us today. This episode was hosted and written by Amy Wu and Anya Morozov, and edited and produced by Anya Morozov. You can learn more about the University of Iowa College of Public Health on Facebook. And our podcast is available on Spotify, Apple Podcasts, and SoundCloud.
If you enjoyed this episode and would like to help support the podcast, please share it with your colleagues, friends, or anyone interested in public health.
Have a suggestion for our team? You can reach us at [email protected].
This episode was brought to you by the University of Iowa College of Public Health. Until next week, stay healthy, stay curious, and take care.

An official website of the United States government
The .gov means it’s official. Federal government websites often end in .gov or .mil. Before sharing sensitive information, make sure you’re on a federal government site.
The site is secure. The https:// ensures that you are connecting to the official website and that any information you provide is encrypted and transmitted securely.
- Publications
- Account settings
Preview improvements coming to the PMC website in October 2024. Learn More or Try it out now .
- Advanced Search
- Journal List
- Elsevier - PMC COVID-19 Collection

Epidemiology and Biostatistics
Epidemiology and biostatistics are the cornerstone of public health and preventive medicine. These practices use mathematical, scientific, and social methods to monitor disease trends and provide intervention to prevent future disease. [147 questions.]
3.1. Epidemiology and Biostatistics Questions
- A. Cross-sectional
- B. Prospective cohort
- C. Retrospective cohort
- D. Randomized control trials
- E. Meta-analysis
- A. Randomized control trial
- C. Case-control
- D. Ecological
- E. Survival analysis
- C. Marriage
- A. Federal Bureau of Investigation
- B. Federal Health Statistics Center
- C. National Center for Health Statistics
- D. National Library of Medicine
- E. There is no centralized organization that records these statistics
Which of the following parties is included in the triple-blinded study, but not the double-blinded study?
- A. Institutional Review Board
- B. Shareholders
- C. Statisticians that analyze the data
- D. Study participants
- E. Study investigators
- A. Case-control
- D. Randomized controlled
- E. Cross-sectional
- A. Length bias
- B. Lead-time bias
- C. Incidence bias
- D. Mortality bias
- E. Morbidity bias
- A. Only selecting men to participate in a study about marital happiness of spouses
- B. Performing a survey of hospital experience of congestive heart failure (CHF) patients one week after admission for CHF, in the patients that have not yet been discharged
- C. An investigator is trying to prove that cabbage consumption in pregnancy causes autism, so he interrogates mothers of autistic children about cabbage consumption, but not mothers of children without autism.
- D. A scale that records all of the patients 2500 kg more than they actually weigh
- E. Randomly assigning 1000 study participants to separate arms in a study
The FDA approval panel responds that this test has what type of bias?
- A. Confounding
- B. Hawthorne effect
- C. Misclassification
- D. Lead-time
When the study results are tabulated, it is found that there is no difference between iatrogenic infections between the new soap and old soap. However, it is found that there were more iatrogenic infections on the second floor than the first.
Which answer best explains why there were more infections in patients on the second floor?
- A. Regression towards the mean
- B. Healthy worker effect
- C. Placebo effect
- D. Hawthorne effect
- E. Random error
What is the best explanation for the participants no longer meeting study criteria?
- A. Hawthorne effect
- B. Information bias
- C. Neyman bias
- D. Recall bias
- E. Regression towards the mean
- A. Randomization
- B. Restriction
- C. Matching
- D. Stratification
- E. Hypothesis testing
Which factor is a cofounder?
- B. Lung cancer
- D. Bias between those that took the survey and refused the survey
- E. There are no cofounders
- A. Sensitivity, specificity, and negative predictive value
- B. Specificity, false-positive rate, and incidence
- C. Sensitivity, specificity, and prevalence
- D. NPV, incidence, and prevalence
- E. NPV, false-positive rate, and sensitivity
- A. Geographic region
- B. Person-time
- C. Population at risk
- D. Prevalence
- E. None of the above

What is the incidence density of Influenza after receiving the experimental influenza vaccine?
- A. 0.13 cases/person-year
- B. 0.25 cases/person-year
Which of the following hypotheses states that the hospital chain may assume their data approaches normal distribution?
- B. Central limit theorem
- C. Inferential statistics
- D. Binomial distribution
- E. Kaplan–Meier function
What is his IQ?
What is the Z-score for an IQ of 105?
- A. −0.66
Which of the measures of central tendency is heavily influenced by the outlier?
- D. Geometric mean
- E. All are appropriate to accurately depict this dataset
Of the last 10 claims, what percentage of the workers can be classified as obese?
- A. Mean = median = mode
- B. Mean = median < mode
- C. Mean < median < mode
- D. Mean > median > mode
The physician counts are as follows:

Which physician represents the median number of the patients seen?
- A. The number needed to treat increases
- B. The confidence interval becomes smaller
- C. The power of the study decreases
- D. Clinical relevance increases
- E. Positive predictive value decreases
Using the information available, what does this mean?
- A. If the drug is tested on 1000 similar sample populations, 950 of these sample populations would likely lose a mean between 1 and 39 lbs.
- B. If the drug is tested on 1000 similar patients, 95% of these patients would likely lose between 1 and 39 lbs.
- C. The findings are not clinically significant
- D. The findings are not statistically significant
- E. The risk ratio shows a mild statistical benefit
- A. Both p- values and confidence intervals are without units of measurement
- B. Confidence intervals that include 1 are always statistically significant
- C. Larger samples produce smaller confidence intervals
- D. Numbers within confidence intervals all have equal clinical importance
- E. p- Values are not comparable to confidence intervals
The scores were normally distributed. The average test score for the class was 50%. The standard deviation was 10% and the highest score was 90%. Approximately what percentage of students passed the exam?
What is the probability that her next patient will be recovering from either alcohol or drug abuse?
- A. Henry’s law
- B. Interquartile range
- C. Stratification
- D. Direct adjustment
- E. Indirect adjustment
Which of the following statements represents his null hypothesis?
- A. Reading the book would increase his test score
- B. Reading the book is not associated with his test score
- C. Reading the book would decrease his test score
- D. The null hypothesis may not be developed until the p -value is available
- A. Accept the alternative hypothesis when there is a high p- value
- B. Accept the null hypothesis when there is a high p- value
- C. Accept the alternative hypothesis when there is a low p- value
- D. Accept the null hypothesis when there is a low p- value
- E. There is no relationship between the null hypothesis, alternative hypothesis and p- value
- A. False-positive
- B. False null is rejected
- C. True null is accepted
- D. True null is rejected
- E. More than one of the above
- A. This is a type I error because the result is likely positive when the disease is not present
- B. This is a type I error because the result is likely negative when the disease is present
- C. This is a type II error because the result is likely positive when the disease is not present
- D. This is a type II error because the result is likely negative when the disease is present
- E. There is no error, as the test will correctly identify when the disease is present
- A. Increases
- B. Decreases
- C. Remains the same
- D. No relationship
- E. Need more information
- A. Decrease the number of test-takers included in the study
- B. Decrease the alpha level of the study
- C. Increase the beta of the study
- D. Increasing the null from 65th to 75th percentile
- A. Degree of precision desired
- B. Expected attrition rates
- C. Size of the population under investigation
- D. Type of study being conducted
- E. All of the above are considerations when calculating sample size
y = a + b 1 x 1 + b 2 x 2 + e
- A. Adjustment coefficient
- B. Dependent variable
- D. Independent variable
- E. Regression constant
- A. There is a negative correlation between sunset and traffic
- B. There is no correlation between sunset and traffic
- C. There is a positive correlation between sunset and traffic
- D. Either sunset or traffic is causative of the other

According to this scatterplot, what is the relationship between systolic blood pressure and heart rate?
- A. Negative exponential
- B. Negative linear
- C. No correlation
- D. Positive exponential
- E. Positive linear
Which answer best describes the relation of this teenager’s number of hours worked and severity of acne?
- A. Strongly negative
- B. Mildly negative
- C. No relation
- D. Mildly positive
- E. Strongly positive
Which statistical test is most appropriate?
- A. Chi-square
- C. Multiple regression
- E. Wilcoxon signed rank test
- B. Small sample size
- C. Studying survival rates in fixed periods
- D. There is high loss to follow-up
- E. The two methods are not comparable
- B. Cross-sectional
- C. Ecological
- D. Meta-analysis
- E. Randomized controlled trial
- A. Consistency of association
- B. Strength of association
- C. Evidence of association
- D. Analogy of other similar associations
- E. All of the above are considerations
- A. p- value, mean
- B. Standard deviation, number of samples
- C. Incidence, prevalence
- D. Expected outcome, observed outcome
- E. Alpha, beta
Without more information, which of the following can be stated as true?
- A. The results of this study are not internally valid
- B. The results of this study are externally valid
- C. The physician’s conclusion applies to all patients taking this drug
- D. The results are not clinically significant to the 60 patients screened
- E. None of the above are true
Favorite ice cream | ||||
---|---|---|---|---|
Chocolate | Strawberry | Vanilla | ||
Male | 30 | 30 | 60 | |
Female | 70 | 20 | 50 | |
In looking at the favorite ice cream by gender, the professor feels that there are distinct differences between men and women in his class. He decides to conduct a chi-squared ( χ 2 ) analysis to test his hypothesis. How many degrees of freedom ( df ) are there in this χ 2 analysis?
The results are as follows:
Exclusive use of outdoor exercise equipment by gender | |||
---|---|---|---|
Aerobic | Anaerobic | ||
Men | 75 | 50 | |
Women | 25 | 125 | |
After looking at the data, the health department employee hypothesizes that different genders prefer to use different types of gym equipment. He decided to use chi-squared ( χ 2 ) analysis to determine if this difference is due to chance alone. What is the approximate test statistic that the employee will use to compare to the critical value to accept or reject the null hypothesis?
What is the Kappa ratio?
- A. Student’s t-test
- B. Paired t-test
- C. Chi-squared
- D. Pearson’s correlation
- E. Kaplan–Meier analysis
- A. Paired t-test
- B. Wilcoxon signed rank test
- D. χ 2
- E. Regression analysis
Which test is best for the truck driver to test his hypothesis that all the brands taste the same?
- B. Chi-squared
- C. Mann–Whitney U test
- D. Multiple regression
- E. Odds ratio
What percent of his point production is tied to his nightly sleep?
Which test would be best to test whether or not one insecticide reduces mosquito-borne illness more than others, while controlling for confounding from demographics and differing risk of exposure (using outdoor attractions as a surrogate)?
- 1. Cohort study
- 2. Epidemic curve
- 3. Longitudinal data
- A. Each demonstrates secular patterns
- B. Each is a type of time-series analysis
- C. Each is analyzed through multiple regression
- D. Each will always show causation of correlation
- C. Interval
- E. Combination of two or more of the above
When stratified into groups according to BMI classification (underweight, obese, etc.), what type of variable is represented?
- A. Interval
- E. Numerical
- A. Lower n (number of observations) has no effect on a binomial distribution
- B. Lower p (success) rate skews the distribution to the right
- C. Normal distribution is never a viable substitute for binomial distribution
- D. There is one universal binomial distribution curve to be used in every scenario
- E. Variables in the binomial distribution are continuous
- B. Chi-square
- C. Likelihood ratio
- D. Paired t-test
- E. Wilcoxon test
- A. Continuous
- B. Dichotomous
The test scores are as follows:
66 , 62 , 73 , 70 , 68 , 59 , 74 , 76 , 65 , 65 , 71 , 62 , 67 , 69 , 70 , 62 , 71 , 72 , 64
What is the interquartile range?
She decides to plot these last nine exam scores into the following table.
Comparative Monday and Friday classroom test performance during the semester | |||||||||
---|---|---|---|---|---|---|---|---|---|
Week 1 | Week 2 | Week 3 | Week 4 | Week 5 | Week 6 | Week 7 | Week 8 | Week 9 | |
Monday | Better | Worse | Better | Better | Better | Better | Worse | Better | Better |
Friday | Worse | Better | Worse | Worse | Worse | Worse | Better | Worse | Worse |
Assuming that the two classes are identical, outside of the date they go to class and take tests, which test would be most appropriate to analyze this data?
- A. Chi-squared test
- C. Student’s t-test
- D. Sign test
- E. Pearson correlation
- B. Chi-square (χ 2 )
- E. Spearman rank correlation
- A. Kruskal–Wallis one-way test
- B. Mann–Whitney U test
- C. Spearman rank correlation coefficient
- D. Wilcoxon test
What is the 3 year survival rate?
- D. >2
- E. All of the above
- A. Analysis of covariance
- C. Linear regression
- D. Multivariate analysis of variance
- E. Spearman rank correlation coefficient
- A. Recall bias
- B. Observer bias
- C. Response bias
- D. Attrition bias
- E. Publication bias
- A. 20–24 weeks gestation
- B. 20–26 weeks gestation
- C. 20–28 weeks gestation
- D. 20–30 weeks gestation
- E. 20–32 weeks gestation
- A. Number of pregnancies
- B. Number of live births
- C. Number of women aged 15–44
- D. Number of pregnant women that die due to complications of pregnancy
- E. Number of women having a high risk pregnancy
- A. Differences between birth and death rates
- B. Differences of income
- C. Differences between immigration and emigration
- D. Differences of life expectancy
- E. Differences of income
- A. Black Hispanic men
- B. Black non-Hispanic women
- C. White Hispanic women
- D. White non-Hispanic men
- E. White non-Hispanic women
- A. Healthcare facilities reporting influenza like illness (ILI)
- B. Public health laboratory reporting positive chlamydia case
- C. A clinician reporting a bite from a strange and aggressive dog
- D. A news station calling a local health department about a salmonella outbreak
- E. A health department epidemiologist calling the local hospital to ask about confirmed HIV cases
- A. Agency for Healthcare Research and Quality
- B. Centers for Disease Control and Prevention
- C. Council of State and Territorial Epidemiologists
- D. National Academy of Medicine
- E. National Association of County and City Health Officials
- C. Congressional Health Committee
- D. Council of State and Territorial Epidemiologists
- A. When the condition is designated as “notifiable” by the state
- B. When the condition is designated as “notifiable” by the Surgeon General
- C. When the condition has a high case fatality rate
- D. When the condition is highly infectious
- E. When the CDC determines that the disease is beyond the scope of a local health department
- A. Specificity
- B. Sensitivity
- C. Positive predictive value
- D. Negative predictive value
- E. Prevalence
- A. Ethical consequences of performing test
- B. Predictive value of results
- C. Stress related to testing
- D. Validity of test results
- E. All of the above are considerations to make when creating screening recommendations
- A. Outbreak
- B. Norovirus Outbreak
- C. Norovirus Outbreak in Tulsa, Oklahoma
- D. Cases of Norovirus in Tulsa, Oklahoma, July, 2014
- E. Cases of Norovirus in Tulsa by Date of Onset, Tulsa, Oklahoma, July, 2014
- A. The beginning
- B. The middle
- D. Depends on disease
- E. The graph should always start in January
- A. Number of cases and time
- B. Number of ill and number of dead
- C. Sensitivity and specificity
- D. Suspected cause of illness 1 and suspected cause of illness 2
- E. Type of outbreak and time
- A. Common source
- C. Continuous common source
- D. Propagated
- E. All of the above outbreak patterns are more than one incubation period
Ate | Did not eat | |||
---|---|---|---|---|
Food | Ill | Well | Ill | Well |
A. Chicken | 20 | 18 | 22 | 10 |
B. Burger | 31 | 11 | 6 | 22 |
C. Hotdog | 12 | 15 | 15 | 28 |
D. Oranges | 25 | 30 | 3 | 12 |
E. Egg roll | 1 | 5 | 30 | 34 |
Which food is most likely the cause of the diarrheal illness?
- A. Chicken tender
- D. Watermelon
- E. Egg roll
- A. Fly back home to the United States
- B. Isolation
- C. Quarantine
- D. Antibiotic therapy
- E. Nothing, she is asymptomatic
- C. United States
- D. Nobody has the authority to detain this passenger
- E. World Health Organization
- A. Epidemic curve
- B. Gantt chart
- C. Geographic information systems
- D. Ishikawa diagram
What is the PPV of this test?
- A. It stays the same
- B. It increases
- C. It decreases
- D. There is no effect
- E. Depends on other variables
- D. Depends on other variables
Specificity + __________ = 100%
- A. Sensitivity
- B. True-positive
- C. True-negative
- D. False-positive
- E. False-negative
Sensitivity + ________ = 1
- A. False-negative
- B. False-positive
- C. Specificity
- D. True-negative
- E. True-positive
- A. False-negative rate
- B. Negative predictive value
- D. Sensitivity
- E. Specificity
- B. Positive predictive value
- C. Negative predictive value
- D. False-positive error rate
- E. False-negative error rate
- A. Federal Department of Vital Statistics
- B. Federation of State Medical Boards
- C. Health Resources and Services Administration
- D. National Vital Statistics System
- E. There is no national collaboration; individual states maintain their own vital statistics
Which of the following answers does not meet criteria for influenza-like illness (ILI)?
- A. Temperature greater than 100°F (37.8°C)
- C. Sore throat
- D. Myalgias
- E. Positive influenza type B
- A. Home interview
- B. Phone interview
- C. Physical in-person exam
- D. Options A and C
- E. Options B and C
- A. Access data from his state’s Department of Vital Statistics
- B. Access a pregnancy medication exposure registry
- C. Conduct case-control study
- D. Conduct ecological study
- E. Conduct randomized control trial
- A. Department of Health and Human Services (DHHS)
- B. Health Resources and Services Administration (HRSA)
- C. International Classification of Diseases Executive Committee (ICDEC)
- D. International Medical Billers Coalition (IMBC)
- E. World Health Organization (WHO)
- A. American-Indian and Alaska Native
- B. Asian and Pacific islander
- D. 6 months
- A. <3 per 100,000
- B. 3–5 per 100,000
- C. 3–5 per 1000
- D. 5–7 per 100,000
- E. 5–7 per 1000
- A. Every year
- B. Every 2 years
- C. Every 3 years
- D. Every 5 years
- E. Every 10 years
- A. Those 15 years of age and below
- B. Parents of those 18 years of age and below
- C. Students in school
- D. Random telephone survey
- E. Both A and B
- A. In person interview
- B. Physical examination
- C. Telephone interview
- D. Internet survey
- E. Combination of the above choices
- A. Evaluating the number of malaria cases in a community during summer and winter
- B. Following the number of newly hired coal workers that develop pneumoconiosis
- C. Asking mothers of children with neural tube defects about their use of folic acid
- D. Administering a new type of drug to compare it to the safety of the old drug
- E. Comparing the number of deaths observed deaths in a population to the number of expected deaths
- A. The alpha level
- B. The beta level
- C. The exposure
- D. The outcome
- E. The hypothesis
Which of the following is true?
- A. Drug A has a higher risk of death
- B. Drug B has a higher risk of death
- C. Drug A has a higher rate of death
- D. Drug B has a higher rate of death
- E. The death rate and risk of both drugs are identical
- A. Number of bicycle injuries in Florida per year
- B. Number of bicycle injuries in Florida per the state population in a year
- C. Number of bicycle injuries, aged 24–35, in Florida per the state population in a year
- D. Number of bicycle injuries, with black hair aged 24–35, in Florida per the state population in a year
- E. Cannot be determined from this information
- A. Life expectancy is higher at birth
- B. Life expectancy is higher at age 60
- C. There is no relationship between the two
- D. It is the same
- E. Impossible to tell with the data given
- B. Homicide
- C. Unintentional injuries
- E. Heart disease
- B. Heart disease
- C. Homicide
- D. Motor vehicle accident
- A. Contaminated equipment at a nursing home leading to deaths of 10 patients, all aged older than 75
- B. Drowning of a 10-year-old
- C. Homicide of a 50-year-old man and his 48-year-old wife
- D. Intentional drug overdose of a 35-year-old and his 55-year-old companion
- E. Motor vehicle accident killing four people aged 68
People killed in gas leak in underground subway | |||||||||
---|---|---|---|---|---|---|---|---|---|
Age group | <1 | 1–14 | 15–24 | 25–34 | 35–44 | 45–54 | 55–64 | 65–74 | >75 |
Number of deaths | 5 | 5 | 5 | 5 | 5 | 5 | 5 | 5 | 10 |
Assuming an endpoint of 75 years of age, what is the approximate overall number of years of potential life lost (YPLL)?

- C. Declining
- D. No specific pattern
- E. Impossible to tell
- A. −0.1
- B. −0.13
- C. −0.2
- D. −0.23
- A. Attributable risk
- B. Attributable risk percent
- C. Population attributable risk
- D. Population attributable risk percent
- E. Risk ratio
What is the relative risk ( RR ) of diagnosis of brain cancer in those exposed to Chemical X, compared to those unexposed to Chemical X?
- A. Attack rate
- B. Cox regression analysis
- C. Odds ratio
- A. Federal Obstetrics Monitoring Program (FOMP)
- B. Obstetrics Surveillance Network (OSN)
- C. Pregnancy Risk Assessment Monitoring System (PRAMS)
- D. Survey of Maternal Care (SMC)
- E. Women and Infant Survey (WIS)
- A. A case-control study is used to yield the odds ratio
- B. If the prevalence is >10%
- C. The total number of subjects is >100
- D. The outcome is rare
- E. When causation has been established
The data can be filled into the following 2 × 2 table:

Which value goes in box X?
- A. Necessary and sufficient
- B. Necessary and not sufficient
- C. Not necessary and not sufficient
- D. Not necessary and sufficient
Which of the following options best answers this question?
- A. Efficient
- B. Sufficient
- C. Necessary
- D. Sufficient and necessary
- A. A case-control study where exposed subjects are misclassified as unexposed and a similar number of unexposed are classified as exposed
- B. A case-control study where exposed subjects are misclassified as unexposed, but no unexposed subjects are misclassified
- C. Interviewing mothers of children with birth defects about chemical exposures in pregnancy
- D. Study participants receiving the experimental drug dropping out of a study due to adverse effects, while subjects in the placebo group remain in the study
- A. The drug has poor clinical significance
- B. The trial lacks internal validity
- C. The trial lacks external validity
- D. The trial is not statistically significant
- E. There is no need for new cholesterol medications
- A. Antivaccination public sentiment
- B. Limited access to vaccinations
- C. Improper storage of a vaccine
- D. Prohibitive cost of the vaccine
Prior to initiating the study, the researcher wishes to see how accurate the formula is if the expected parameters were to vary. What is the name of the process used to accomplish this?
- B. Data organization
- C. Sensitivity analysis
- D. Standard deviation
- A. Infectivity
- B. Immunogenicity
- C. Pathogenicity
- D. Secondary attack rate
- E. Virulence
After speaking with a representative at the CDC, it appears that the influenza virus has mutated to a form that is not covered by the annual vaccination. Furthermore, it has turned into a strain that only those older than 45 years old demonstrate any immunity.
What is the best explanation for this influenza epidemic?
- A. Antigenic drift
- B. Antigenic shift
- C. Malaria coinfection
- E. Resistance to neuraminidase inhibitor
How is this outbreak in chickens best categorized?
- B. Enzootic
- C. Epidemic
- D. Epizootic
- E. Pandemic
- A. Characterize the epidemic (time, place)
- B. Develop a hypothesis
- C. Establish a case definition
- D. Establish a diagnosis
- E. Determine whether or not an epidemic is occurring
- A. Depends on the type of drug
- B. The drug is widely considered to be safe
- C. The drug has little margin for safety
- D. The LD 50 is likely close to the ED 50 and TD 50
- A. American Inoculation Safety Network
- B. Immunization Complication Monitoring Organization
- C. Phase 4 Study Observation Program
- D. US Shot Surveillance Alignment
- E. Vaccine Adverse Event Reporting System
- A. To recommend physicians for privileges at a hospital
- B. Analyze results of utilization review
- C. Review the ethics of a research study
- D. Review cases of clinician misconduct for the state licensing board
- E. Determine accreditation status for educational institutions.
- A. IRB approval
- B. Legal decision making capacity
- C. Presumption of competence
- D. Understanding of risks and benefits
- E. Voluntary decision making, without coercion
What is a potential concern of the IRB?
- A. The test may significantly alter the way that clinicians screen for this disease
- B. The company that manufactures the treatment may suffer financial loss
- C. It would be unethical to not screen patients for a curable disease
- D. Harms exceeding benefits is not a legitimate concern to investigate
- E. Disproving the benefit profile of the screening test would alter the test’s indications
- A. Adults in apartments
- B. College students in dormitories
- C. Homeless people in shelters
- D. Inmates in prison
- E. All of the above are included
- A. Behavioral Risk Factor Surveillance System
- C. Department of Justice
- D. Office of National Drug Control Policy
- E. Substance Abuse and Mental Health Services Administration
- A. Congenital malformations
- B. Low birth weight
- C. Maternal complications
- D. Sudden infant death syndrome
- E. Respiratory distress of newborn
3.2. Epidemiology and Biostatistics Answers
Cross-sectional studies look at a snapshot of the population being studied. Extrapolating the population findings to an individual level may lead to ecological fallacy, in which an association at the population level is not necessarily true at the individual level. This is especially true when there is a larger population (constituting a cross-sectional ecological study). For example, a cross-sectional ecological study showing that City B has a higher rate of mesothelioma than City C may falsely lead someone to believe that all residents of City B are more likely than residents of City C to get mesothelioma, regardless of asbestos exposure.
A RCT allows the investigator control over the exposure. Although an RCT would yield the most robust results, it would also be considered unethical to withhold a screening tool that has been shown by multiple previous studies to be effective.
A cohort study would involve enrolling study participants based off their exposure, in this case whether or not he/she had a screening colonoscopy. Information gained from a cohort study can be used to compare case fatality ratios and determine how effective an intervention is.
A case-control study categorizes study participants based off of their disease status rather than their exposure status and is thus less appropriate for this example.
The vital statistics sector of each state’s department of health records birth, death, marriage, and divorce. Cancer is typically reported through registries recorded at health facilities. Hospital cancer registries send their records to the central cancer registry in its state. The state cancer registry will then submit it to the CDC’s National Cancer Institute’s Surveillance, Epidemiology, and End Results (SEER) Program.
The NCHS compiles statistical information in numerous categories from numerous sources (states, municipalities, private organizations, etc.). These statistics are used to guide public health decision making and create goals, such as the Healthy People program. In addition to storing health statistics, NCHS also collects health data. The NHANES is hosted within NCHS.
When a study has subjective outcomes, such as wrinkle reduction, blinding the parties is used to eliminate bias. Single-blinded studies blind the study participants. Double-blinded studies blind the study participants and the study investigators. Triple-blinded studies blind the study participants, study investigators, and the statisticians. Blinding is less common in objective studies, such as those recording lab results, but it still may be beneficial.
The IRB is the group that approves studies, mainly based on how ethical and feasible they are. There is no need to blind this group. Shareholders should not have influence over the internal workings of the study, so there is no need to blind this group either.
The main difference between an observational study and a controlled study is that controlled studies will manipulate the risk factor. In a randomized controlled trial (RCT), exposure to the risk factor is determined by those conducting the study; thus, it is an experimental study and not an observational one.
Length bias occurs when a less aggressive disease appears to have a higher incidence. This is because slower moving diseases are more likely to be detected since the subject is alive for longer. To the contrary, diseases that cause mortality sooner are less likely to be detected.
Length bias is often confused with lead-time bias. Lead-time bias occurs when the diagnosis is made earlier and creates the illusion that the subject lived longer than if the diagnosis were made later. If a subject with a terminal disease is diagnosed 1 month earlier, he will still die at the same time. However, the records will indicate that he lived one month later with the earlier diagnosis.
Nondifferential error/bias is also called random error, or chance error. If a sample is has equal amounts of error on both sides of the true value, the error will cancel out and the overall value will closely approximate the true value. Differential error/bias produces deviation in one direction from the true value, either above or below.
Answers A, B, C, and D are all examples of differential bias. All of these answers target only one of the two populations that should be interrogated equally. In answer “A,” only the men and not the women are questioned about marital happiness. In answer “B,” the CHF patients that have been discharged will not be questioned regarding their experience. The patients that have been discharged sooner may have a different perception of their hospital stay. In answer “C,” the researcher contributes to recall bias by pressuring mothers of autistic children, but not the nonautistic. Mothers of children with mental or physical disabilities are more likely to reflect more heavily on their exposures and activities during pregnancy. In answer “D,” the scale produces a differential bias by pushing the observed value in one direction away from the true value.
Only answer E is an example of nondifferential bias. Participants are randomized equally, without bias.
Lead-time bias is the appearance that early diagnosis of a disease prolongs survival with that disease. In this case, the FDA panel should be concerned that the early diagnosis of pancreatic cancer is not actually increasing the length of time that the patients live.
Confounding is not a type of bias. Confounding occurs when there is a variable interacting with the independent variable (exposure) and dependent variable (outcome).
The Hawthorne effect is also known as observer bias. It is the theory that people (including study participants) will change their behavior if they believe that they are being observed.
Misclassification bias occurs where there are errors in recording disease or exposure.
Length bias occurs when the prevalence of a disease with a longer lasting disease appears higher than the prevalence of shorter lasting diseases. Consider “Disease X,” which lasts 1 month and “Disease Y,” which lasts for 6 months. There are 5 months of extra opportunity for “Disease Y” to be discovered, leading to the appearance of a higher prevalence compared to “Disease X.”
The Hawthorne effect states that individual behavior is changes when a person is aware they are being observed. In this case, the nurses are more likely to use the soap because they are being observed. However, the nurses on the first floor are even more likely to use soap because there is a higher risk of being discovered to not be in compliance with study parameters.
Regression towards the mean states that the further a value is from the mean, the more likely future recordings are closer to the mean. For example, if an otherwise healthy patient presents to your clinic and is found to have high blood pressure, future blood pressure readings are expected to be closer to the true blood pressure.
The healthy worker effect states that workers are typically healthier than the general population because they are different from the general population, as ill and disabled people are typically unemployed.
The placebo effect, occurs when a person believes they are healthier because they are receiving treatment, even if the treatment is not scientifically effective.
Random error is an accepted discrepancy in clinical studies. It may be controlled for in all phases of study design. There is no reason to believe that random error is the source of the findings in this vignette.
Regression towards the mean states that the further a value is from the mean, the more likely future recordings are closer to the mean. Systolic blood pressure is not a static measurement: It varies daily, and those recorded at outlier measures are more likely to be at the extreme for their norm. Over time, an individuals recorded blood pressures will average out to more accurately show their mean.
The Hawthorne effect is the observation that individual behavior changes once that individual is aware they are being observed.
Information bias is the use of erroneous study data and may result from imprecise and invalid study measures.
Neyman bias (also known as selective survival bias), occurs when cases in a study that survive have different exposures than those that die.
Recall bias occurs when those that suffer an adverse event recall their exposure history differently than those that did not suffer an event. A common example is that mothers of children with birth anomalies may recall their pregnancy differently than mothers of healthy children.
Stratification is the only technique listed that reduces confounding during the analysis stage. It involves breaking the data into strata that can be more descriptive. For example, stratification of elementary school students would reveal that 3 rd graders have higher understanding of math than 1st graders, but less than 5th graders. Randomization, restriction, and matching are all techniques to reduce confounding during the design stage of a study. Hypothesis testing is not a method used to control for confounding.
Confounding is a type of bias that occurs when a third variable influences (confounds with) the factor of interest and skews the observed result between exposure and disease. A confounding variable by definition must be associated with both the outcome and exposure. In this example, alcohol use is a confounder, as it is a risk factor for cardiovascular disease and is associated with cigarette smoking.
Confounding may be accounted for and controlled in studies. Controls to reduce confounding may be built into the design stage, or analysis stage of a study. Ways to control for confounding in the design stage include randomization, restriction, and matching. Ways to control for confounding in the analysis stage include standardization, stratification, and statistical modeling.

Bayes’ theorem is a mathematical tool for figuring out the probability of an event. It can be calculated every time new information is received that may alter the probability of the event.
Once a prior probability is known the next sequential event will yield a posterior probability. When more information is learned, the former posterior probability becomes the prior probability and a new posterior probability is calculated. Prevalence is equivalent to a prior probability. Bayes’ theorem can be considered an alternative method of calculating the PPV.
The following formula may be used in place of Bayes’ theorem:
Posterior Probability = ( Prevalence ) ( Sensitivity ) ( Prevalence ) ( Sensitivity ) + [ ( 1 − Prevalence ) ( 1 − Specificity ) ]
This formula may be difficult to remember, but the prevalence, specificity, and sensitivity can be used to fill out the cells of a 2 × 2 table, which will yield the PPV.
Incidence density is a tool used to describe the number of new cases of a disease (incidence) per summation of time that each person is at risk of disease in a specified time and place. It is useful for observing dynamic populations (including clinical trials), where people are entering the leaving the risk pool. Additionally, it allows for each subject to be counted in the numerator more than once. This is important in cases where a subject experiences the disease of interest more than once.
For example, a researcher may be interested in observing a daycare to see how frequently children develop conjunctivitis over a 3-year period. In this setting, not all children are in the daycare for the same amount of time. Moreover, some children contract conjunctivitis more than once. If one child attends the daycare daily for 36 months and another is only there for 6 months, the overall person-time would be 42 months.
The downside of person-years is that a few amount of subjects may substantially influence the incidence density. This happens when a small number of people are observed over a long period of time are calculated along with a large number of people observed for a short duration.
Refer to Answer #15 (directly above) for an explanation of incidence density.
The time period of observation in this vignette is measured in years. Altogether, the six patients combined for 16 years of observation. During that time period, there were four cases of influenza.
Incidence Density = # New Cases Summation of Person − Time = 4 16 Person − Year = 0.25 Cases Person − Year
The central limit theorem states that when there are a large amount of mutually independent random variables, the mean population will approach normal distribution. A general rule of thumb is that for the central limit theorem to hold true, N ≥ 30.
The Hawthorne effect is the phenomenon where subjects change their behavior because they are aware that they are being observed.
Inferential statistics methods allow one to make a statement about the general population by studying a smaller part of that population.
Binomial distribution describes data that has two discrete outcomes, typically success or failure.
Kaplan–Meier function is a tool used to examine survival analysis.
The IQ is a tool for gauging intellectual abilities. It is determined through standardized testing to calculate the mental age, which is then divided by the chronological age and multiplied by 100. IQ was designed to follow a normal distribution with the central mean at 100 and the standard deviation of 15. As always, 68% of people fall within one standard deviation of the mean (85–115), 95% fall within two standard deviations of the norm (70–130), and 99% fall within three standard deviations of the norm (55–155). Observations more than two standard deviations from the mean are typically considered to be abnormal.
Two standard deviations below 100 is 70. An IQ of 70 nearly meets criteria for intellectual disability from the International Classification of Diseases (ICD) and the Diagnostic and Statistical Manual of Mental Disorders (DSM) categorize intellectual disability as follows: Mild (50–69), moderate (35–49), severe (20–34), and profound (>20). Individuals with intellectual disability experience reduced intellect and impaired adaptive functioning.
The Z-score describes how many standard deviations are between an observed value and the mean. Observed values that are larger than the mean will have positive Z-scores , while observed values that are less than the mean will have negative Z-scores . It can be calculated through the following formula:
Z - score = x − μ σ
x is the observed value
μ is the mean of the distribution
σ is the standard deviation
To solve this problem, it is important to understand that the IQ scale was designed to follow a normal distribution with the central mean at 100 and the standard deviation of 15.
The Z-score for an IQ of 105 is calculated as follows:
Z - score IQ 105 = 105 − 100 15 = 5 15 = 0 . 334
In this set, 3000 is the outlier. This outlier would greatly skew the mean. With the full set, the mean is 281.8. Without the value of 3000 contributing to the mean, the overall mean drops to 10.
The geometric mean is typically used for extremely large numbers. It is most often used in logarithmic fashion. The geometric mean is found by multiplying all of the numbers ( n ) in the set and then taking the nth root of the product. Another method of calculating the geometric mean involves converting all of the numbers in a set into a logarithmic scale. The geometric mean cannot be used on negative numbers, or the number zero.
To answer this question, it is important to understand the definition of obesity. Obesity is determined based on the body mass index (BMI), which is calculated as a person’s weight in kilograms (kg) divided by the person’s height in meters squared (m 2 ).
Category | BMI (kg/m ) |
---|---|
Underweight | <18.5 |
Healthy | 18.5–24.9 |
Overweight | 25.0–29.9 |
Obese | ≥30.0 |
Five of the ten (50%) people filing claims are obese.

If a distribution is skewed to one side, there are fewer events on that side of the curve. This means that there are more events on the other side of the curve. An easy way to remember this is that skew rhymes with few.
In a skewed distribution, relative positions of the measures of central tendency is constant. From the tail (side with few) to the peak, the order is mean, median, and mode, as is shown in the diagram.
To solve this problem, you must first put the physicians in order of the number of patients they saw:
150 , 220 , 250 , 250 , 300 , 315 , 335 , 360 , 390 , 400 , 410
After placing the numbers in ascending order, you must then multiply the number of subjects by the percentage sought. This leads to 0.50 × 11 = 5.5. After rounding this number up, you get the 6th number. The 6th number in the ascending series is 315. Therefore, 315 represents the 50th percentile. The 50th percentile is also called the median.
The same method of calculation can be used for all percentiles, not just the 50th. As there is no universally correct consensus on how to measure percentiles and quantiles, some numbers may slightly vary by mathematician.
To calculate the interquartile range (the middle 50%), subtract the number found at the 25th percentile from the 75th percentile.
As the number of subjects increases, the confidence interval becomes smaller. This is because the confidence interval is calculated by the formula x ¯ ± Z - score ( S . D . n ) , where n represent the number of trials. S . D . n represents the standard error of the mean (S.E.M), which describes how far a population mean varies from the true mean. Since n is in the denominator, the S.E.M. will decrease as n increases. As the S.E.M. decreases, the confidence interval decreases.
The NNT is a function of the attributable risk and is unrelated to the number of overall subjects.
As the number of subjects increases, the power increases.
Clinical relevance of a study is unrelated to the number of subjects.
As the prevalence of a disease increases, the PPV increases. Thus, the PPV will only increase if the number of subjects with the disease of interest increases.
The true value of this weight loss medication may never be known. However, confidence intervals provide a range of values that are believed to include this value. Confidence intervals are often preferred to p- values because they convey more information. Using the information presented in the vignette, the reader is not only able to tell that the average weight loss is 20 lbs, the reader is also able to know that if this subject is repeated in a similar group 95% of the time, the average weight loss will fall between 1 and 39 lbs.
Confidence intervals are used to describe parameters of the study population, not the individual subjects. If the confidence interval characterizing a risk difference does not include 0, the findings are said to be statistically significant. This is because if 0 is not included in the confidence interval, less than 5% of similar studies would have a mean of 0. A risk difference of 0 means that there is no change. Similarly, if a confidence interval describing an odds ratio ( OR ) or risk ratio ( RR ) includes 1, the results are not statistically significant. This vignette describes the risk difference, not the OR or RR .
Clinical significance depends on the situation and the person interpreting the data. A 1 lb weight loss may not be considered clinically significant in a 250 lbs person, but a 39 lbs weight loss in a 250 lbs person is significant.
The true value of an intervention within a population may never be known. Confidence intervals provide a range of values that are believed to include this value. They are used to describe parameters of the study population, not the individual subjects. A confidence interval gives the likelihood of future studies to yield a range of results. A 95% confidence interval means that 95 out of 100 studies in similar groups will yield a population statistic that falls within the confidence interval.
Unlike p- values, confidence intervals are expressed in units. Confidence intervals are often preferred to p- values because they convey more information. Both confidence intervals and p- values express a degree of certainty, which may be converted from one approach to the other. For example, a 95% confidence interval is the same as a p- value of 0.05.
If the confidence interval characterizing a risk difference does not include 0, the findings are said to be statistically significant. This is because if 0 is not included in the confidence interval, less than 5% of similar studies would have a mean of 0. A risk difference of 0 means that there is no change. Similarly, if a confidence interval describing an odds ratio ( OR ) or risk ratio ( RR ) includes 1, the results are not statistically significant.
Confidence intervals measure precision around a point estimate. Larger studies are more precise and yield more narrow intervals. In homogenous confidence intervals, all values carry equal importance. However, in heterogeneous confidence intervals, certain values hold more significance than others.
97.5% of the medical students passed the exam. To better understand this question, one must understand standard deviation within the normal distribution. When the count is normally distributed, 68% will fall within one standard deviation. Half of these (34%) will be greater than the mean and the other half (34%) wall fall below the mean. Roughly 96% of the count will fall between two standard deviations. Half of the 96% (48%) will fall above the mean and half below. The remaining 4% falls outside of the two standard deviations, with 2% above and 2% below the cutoff.
The following illustration demonstrates the distribution of the test scores. All of the students that received a score greater than two standard deviations below the mean have been shaded in gray. Adding together all of the gray segments, one can find that 98% of the medical students passed their art history course. They are now able to continue their medical education and take a course in geography.

This question requires the understanding of probabilities. For any event, the highest possible probability is 1.0, while the lowest possible probability is 0.
If the number of alcohol (0.6) and opiate (0.5) abusers are added together, the number would be 1.10, which is not a possible percentage. This addition does not account for overlap in the group that abuse both alcohol and opiates. If alcohol and opiate abuse were mutually exclusive, adding these two numbers would yield the correct answer.
To find the probability that the next patient abuses either alcohol or opiates, the Rule of Addition must be used. This requires the addition of the events of interest (alcohol and opiate abuse), minus the common overlap between events. Therefore the answer to this question would be calculated by:
0 . 6 + 0 .5 − 0 . 3 = 0 .8
There is a 0.8 probability that the next patient will abuse either alcohol or opiates.
Standardized Mortality Ratio = Observed Number of Deaths Expected Number of Deaths × 100
Calculating the standardized mortality ratio (SMR) is a method dividing the total number of observed deaths in a population to the total number of expected deaths in a population. The number is usually multiplied by 100, leaving the standard population with a value of 100. If the observed number of deaths is greater than the expected number of deaths, the SMR will be >1.
Adjustment produces fictional numbers that can be used to compare populations with different variables. There are two forms of adjustment: Direct adjustment and indirect adjustment. Direct adjustment requires a second population from the original, which is used to extrapolate rates that create a less biased comparison. Meanwhile, indirect rates are performed when there is no comparison population, so a standard population must be used to accomplish the same goal.
The standardized mortality ratio (SMR) is a form of indirect adjustment used to evaluate the actual versus the expected ratio of deaths and compare this metric between populations.
An SMR value of 1 indicates that the number of observed deaths is what is expected. Meanwhile, an SMR greater than 1 indicates there have been more observed deaths than expected. Finally, an SMR less than 1 indicates that there have been less observed deaths than expected.
The SMR of each population can be compared to others, while holding the variable of concern constant, in order to determine if the outcome of interest (death) is different between populations.
None of the other options are directly related to the SMR.
Developing a hypothesis is the first step to answering a statistical question. For any given investigation, there is either an association between the variables, or there is not. A null hypothesis (H 0 ) assumes that there is no difference between the variables being tested. To the contrary, an alternative hypothesis (H A ) assumes that there is a difference between the variables. The alternative hypothesis may be considered the opposite of the null hypothesis.
The null hypothesis is assumed to be true unless stated otherwise. The purpose of a hypothesis test is to determine if the sample results of a study provide enough evidence against the null that it is likely the null would be false in the target population. Once the null is rejected, the alternate hypothesis is accepted as true. If the null cannot be rejected, it does not mean that the null is accepted as true. This is because data that is insufficient to show that a difference between variables is zero does not prove that the difference is zero.
In this case, the null hypothesis would assume that there is no association between reading the book and test score. Meanwhile, alternative hypothesis states that there is an association between reading the book and test score.
The answer builds upon the explanation of hypothesis testing from Answer #31 (directly above).
The strength of evidence to accept or reject the null hypothesis is calculated as the p- value. It estimates the probability of finding an association in the target population as large as the association found in the sample, while assuming that the null hypothesis is true. A small p- value means that the association found in the sample is unlikely to be due to chance. Furthermore, the null and alternative hypothesis are differentiated by an artificial cut point, known as the significance level. If the p- value is less than the significance level, the null hypothesis is rejected and the alternative hypothesis is accepted. Because it is considered to be a measure of strength of evidence against the null, the p- value should not be used to infer whether or not the null is true or false.
A type I error occurs when a null hypothesis is rejected when it is actually true. It is frequently called a false-positive. If the true null is rejected, that means the alternative hypothesis may falsely accepted when the association may be due to chance. The probability of making a type 1 error is represented by α.
A type II error occurs when a false null hypothesis is not rejected, while the alternative hypothesis is true. This is known as a false-negative. The probability of making this type of error is represented by β. The power (1 − β) of a study is the likelihood of committing a type II error.
This question requires understanding the concept of anergy. Anergy refers to an inadequate immune response and may be a result of several variables including: The age of the patient, the overall health of the patient, immunosuppression, and many other factors. In this problem, the poorly controlled HIV status implies that the patient is immunosuppressed and incapable of demonstrating a response to the PPD. Therefore, the PPD will be negative, despite the patient actually having tuberculosis.
This question is an example of a Type II error. Also known as false-negative error and beta error, type II error occurs when something is declared as false, when it is actually true. This type of error may occur in cases of anergy and testing within the window period, amongst many other examples.
A type I error occurs when something is declared as true, when it is actually false. This type of error is also known as false-positive error and alpha error. An example of a type I error would be a positive PPD due to nontuberculosis mycobacterium exposure. In this instance, a positive PPD would indicate that a patient has been exposed to tuberculosis, when they have not. Another example of a type I error would be a positive RPR while testing for syphilis in a patient that has Lyme disease, lupus, malaria, or is pregnant.
The confidence interval is derived by using the formula:
Confidence Interval ( Z - score ) = x ¯ + Z - score ( SD n )
- x ¯ is the mean
- SD n represents the standard error of the mean (SEM), the variation within a sample
- 95% of the scores fall within 1.96 standard deviations of the mean.
The equation is solved by plugging in the numbers as follows:
95 % = 80 ± 1.96 ( 15 225 ) = 80 ± 1.96 ( 1 ) = 78.04,81.96
As the Prevalence of the disease in the population increases, the PPV increases. Alternatively, when the prevalence increases, the NPV decreases.
The power of a test is the probability of correctly rejecting the null hypothesis. A study with insufficient power may not detect and accurately identify an important causative effect. Increasing the number of study participants always increases the power. Power can be represented by the equation: Power = 1 − beta, where beta represents the probability of rejecting the null when the null is actually true. Therefore, as beta increases, power decreases (answer C). Increasing the threshold of the null hypothesis (answer D) means that the null is more likely to be rejected, thus increasing power. As the difference between the alternative and mean hypothesis increases, the power will also increase.
A higher alpha level will result in rejecting the null more often, thus increasing power (answer B).
Determining minimum sample size for a study is an important stage in the planning process. Having a large enough sample size is important for obtaining power and detecting a clinically meaningful differences with statistical assurance. On the other end, having a large sample size is expensive and exhausts resources. A common goal of clinical researchers is to find the minimum number of study participants necessary to yield meaningful results that are valid, accurate, reliable, and have integrity.
Each study has special considerations when determining the sample size. The type of study and the hypothesis being tested are primary considerations. Other important variables include the degree of precision desired, expected attrition (dropout) rates, size of population under investigation, and the method of sampling adopted.
The larger the sample size, the greater the accuracy and precision. Specific types of studies may anticipate larger attrition. There should be enough study participants to counteract the expected attrition. Larger populations call for larger sample sizes to represent enough of the population to produce accurate study conclusions. Finally, sample size depends on the type of sampling being used. For example, randomly drawn studies will require a larger sample size than a stratified sampling plan.
Sample size calculation should be deliberated with consideration to precision analysis, power analysis, and probability assessment. A large part of calculations are based upon criteria for controlling type I and type II errors. Sample size calculation is typically performed in stages: Size estimation/determination, sample size justification, sample size adjustment, and sample size re-estimation. Each stage carried specific considerations. For example, the adjustment stage must consider factors such as expected attrition rate and covariates.
This equation is the key to performing multivariable analysis, which is used to understand the relationship that different independent variables interact upon a dependent variable. This type of analysis is useful for showing the change in a dependent variable when one or more independent variable changes.
In y = a + b 1 x 1 + b 2 x 2 + e ,
y = dependent variable
a = regression constant, the starting point where independent variables begin to act on the dependent variable
b = adjustment coefficient that weighs different independent variables according to importance
x = independent variable
An example of multivariate analysis would be the understanding of how grade point average (GPA), physics test scores, biology test scores, and chemistry test scores correlate with medical school entrance exam scores. If the entrance exam emphasizes biology most of all, the biology test scores will hold a higher adjustment coefficient.
The variable ( r ) describes the linear correlation between two quantitative variables. Potential values of r span from −1 to +1. If r < 0, the correlation is negative. If r = 0, there is no correlation. If r > 0, the correlation is positive. Answer “D” is not correct because correlation does not equal causation. In this example, it is unlikely that the sun setting causes more traffic, or that an increase in traffic causes the sun to set. It is more likely that rush hour occurs during the time the sun is setting.
A line of best fit can be used to help identify a linear relationship between variables. When inserted into the scatterplot from the vignette, it reveals a positive linear relationship.

This question is an example of correlation analysis. When the investigator has control over the independent variable, it is known as regression analysis.
Pearson correlation coefficient is a tool used to estimate the strength of a linear relationship between two normally distributed variables. It is represented by the r value, which varies from −1 to +1. Values closer to −1 have a negative association. That is, when one value decreases, the other increases. In contrast, values closer to +1 have a positive association, meaning both variables increase together. When r is 0, there is no association between the variables.
Multiple regression is a method for examining how a normally distributed dependent variable is influenced by two or more continuous independent variables. If performed correctly, it allows researchers to assess the impact of one variable while controlling others. Multiple regression may be viewed as an extension of simple linear regression.
Survival analysis is used to determine the outcome of dichotomous variables, including live/die and success/failure.
The actuarial method of survival analysis is used to determine the number of survivors in fixed time intervals, such as years and months. A new line of the table is created for every fixed time period. Because of the set time periods, this method as not as good as the Kaplan–Meier method for accounting for censorship and loss to follow-up. This method is useful medical research and the insurance industries. It may be easier to apply this method if the sample size is large.
The Kaplan–Meier method of survival analysis does not have fixed time intervals. A new line of the life table is calculated for every new death. During death free intervals, study participants may be removed from the denominator if these participants are censored or lost to follow-up. This allows for more accurate computation of survival rates. It is easier to apply this method if the sample size is small.
A funnel plot is a tool used to evaluate for publication bias. Publication bias occurs when the results of published studies are different than the results of unpublished studies. There is a tendency of studies demonstrating certain opinions to be published, while studies demonstrating the contrary opinion go unpublished. Consider an example of a study that demonstrates colon cancer screening actually increases the incidence of colon cancer. The researcher may more apprehensive to publish such a study that bucks conventional understanding and may provide a danger to the public. Funnel plots populate a “funnel” of expectations around the mean. Gaps in the funnel may suggest publication bias.
Meta-analysis studies are composed of numerous studies and have an inherent risk of publication bias.
This question is asking about the NNT, the number of people who would need to be treated to benefit one person. It is calculated by the following equation:
Number Needed to Treat ( NNT ) = 1 Absol ute Risk Reduction
Absolute Risk Reduction ( ARR ) = Risk ( Exposed ) – Risk ( Unexposed )
Ideally, the length of time that treatment is required to obtain a unit of benefit should be included in the calculation.
In this question, an ARR of 5% (0.05) is given. The NNT is calculated as follows:
NNT = 20 = 1 0.05
Meanwhile, the number needed to harm (NNH) is calculated as follows:
Number Needed to Harm ( NNH ) = 1 Absolute Risk Inc rease
The following nine considerations are widely utilized to distinguish causal from noncausal associations. Although none of the considerations can bring indisputable evidence for causality, together the considerations can have a strong predictive value. Besides temporality, there are no necessary or sufficient criterion to establish a relationship as causal.
- 1. Consistency of association
- 2. Strength of association
- 3. Specificity (only one factor is consistently implicated)
- 4. Temporal factors
- 5. Coherence of explanation—does it make logical sense?
- 6. Biological plausibility
- 7. Experimental evidence from a controlled trial supports causality
- 8. Dose-response relationship
- 9. Analogy (can be applied to similar associations)
The standard error ( SE ) is calculated as:
SE = SD √ N
Where SD = Standard deviation
N = Number of samples
While the SD shows the variability of individual observations, the SE shows the variability of means of samples.
This question is intended to test understanding of statistical inference and validity. Statistical inference is the practice of making general characterizations after analyzing a sample. Part of exercising statistical inference is the assessment of validity. Internal validity describes how well a study represents true associations present within the study. It is dependent on how well the design, data collection, and analysis is performed. Bias and random variation can reduce internal validity. There is nothing to suggest that the study in the vignette lacks internal validity. External validity describes how well results of one study are generalizable to a different population. Due to the small number of subjects, this study lacks external validity and should not be used to infer that patients worldwide will experience similar results.
A study may be statistically significant with or without biological or scientific significance. If this study is found to be internally valid, the weight loss medication not promoting weight loss would be considered clinically significant.
Degrees of freedom ( df ) is calculated by the following formula:
Degrees of Freedom = ( Rows − 1 ) ( Columns − 1 ) In this problem, there are two rows and three columns.
D egrees of Freedom = ( 2 − 1 ) ( 3 − 1 ) = 2
Chi-squared is the most commonly used nonparametric test and is best for hypothesis testing between categorical variables. When used appropriately, it tells an investigator whether observations are correlated, or if they are due to chance.
After rounding, the test statistic is 57. Because of rounding, other people calculating this question may reach a slightly different test statistic.
Chi-squared ( χ 2 ) is calculated by using the following formula:
∑ ( Observed Data − Expected Data ) 2 Expected Data
The observed data is the number in the original table that was plotted by the health department employee.
The expected data is calculated though the following formula for each box:
Expected = ( Rows ) ( Columns ) ( Total )
This formula is plugged into the original table to get the following:
Expected use of outdoor exercise equipment by gender | |||
---|---|---|---|
Aerobic | Anaerobic | ||
Men | 125 | ||
Women | 150 | ||
100 | 175 | 275 |
The observed and expected values may now be clearly expressed in the following table:
Observed and expected use of outdoor exercise equipment by gender | |||
---|---|---|---|
Aerobic | Anaerobic | ||
Men | 75 | 50 | |
45 | 80 | ||
Women | 25 | 125 | |
100 | 175 |
Finally, the chi-squared equation ( χ 2 = ∑ ( Observed Data − Expected Data ) 2 Expected Data ) may be used for each box and yield the following
Use of outdoor exercise equipment by gender | |||
---|---|---|---|
Aerobic | Anaerobic | ||
Men | |||
Women | |||
100 | 175 |
When these four numbers are added together (20 + 16.3 + 11.3 + 9.5), the test statistic is 57.1. The test statistic is then compared with the χ 2 critical value using the χ 2 table. To use the table, you must choose a significance level and select the degree of freedom ( df ):
Degrees of Freedom = ( Rows − 1 ) ( Columns − 1 )
In this example, df is 1. Following the table, using df 1 and α 0.05, the critical value (aka significance value) is found to be 3.84. Because the test statistic is higher than the critical value, the null hypothesis is rejected and the alternative hypothesis is accepted. This means that the worker’s observations show that men and women have different preferences between aerobic and anaerobic exercise machines.
The Kappa ratio is a measurement of the agreement between two parties when accounting for random chance. It is necessary to account for chance because random agreements will happen by chance. Kappa ratios are valued from −1 to +1. The further negative the ratio, the more disagreement, while the further positive, the further the agreement. A Kappa ratio of 0 indicates that the agreement is occurring due to chance alone.
The true or false answers represent a dichotomous variable that can be placed on a 2 × 2 table. The table is set up by the number of true and number of false responses by each resident. Boxes a and d represent agreement, while boxes b and c represent disagreement.
The table below has been filled out with the information extracted from the above vignette.

From this, one can calculate the Kappa statistic.
Kappa = Observed Agreement − Agreement Due to Chance Total Number − Agreement Due to Chance
No matter how well a study is matched, it is not possible to find better comparisons than the subjects to match themselves. A paired t-test allows researchers to compare the significance of an intervention on a normally distributed group before and after they experience the intervention. The null hypothesis is that the intervention produces little to no difference from before to after. Meanwhile, the alternative hypothesis is true if the before and after measurements are further apart.
Analysis of variance (ANOVA) uses the f-test. This test compares the dispersion within individual variable groups to dispersion between variable groups. An f-test is used when one is comparing three or more variables. It may indicate that one group is statistically different from the others, but it does not exhibit which group is different.
While t-tests are used to directly compare two groups to each other, analysis of variance (ANOVA) is used to compare multiple groups simultaneously. The null hypothesis when using ANOVA is that there is no difference between groups. To the contrary, the alternative is that the groups are different. ANOVA does not tell how they are different, only that they are different. After a significant effect has been found through ANOVA, post-hoc analysis is used to tell how the variables differ. In this analysis, post-hoc analysis would determine which coffee is best (and worst).
While t-tests could be used to compare the data directly, it is not preferred. This is because it would require each combination of variables to be compared directly. In this case, each coffee would have to be compared against one another. If each t-test has 0.05 risk of error, the overall error rate compounds with each t-test.
ANOVA uses the f-test. This test compares the dispersion within individual variable groups to dispersion between variable groups.
The coefficient of determination describes the proportion of variation of a dependent variable that can be explained by an independent variable. It can be calculated by squaring the Pearson correlation coefficient. If the Pearson correlation coefficient (r) is 0.8, the coefficient of determination (r 2 ) is 0.64, or 64%. This means that hours of sleep (independent variable) is responsible for 64% of point production (dependent variable). Because only 64% of point production is attributed to sleep, 36% (100%–64%) is caused by other factors.
Multiple R squared (R 2 ) is analogous to r 2 , but is used in multiple regression.
The ideal test to calculate this problem should be able to compare the means of categorical independent variables (type of insecticide) with a continuous dependent variable (incidence of mosquito-borne illness). An alysis o f va riance (ANOVA), an alysis of cova riance (ANCOVA), and t-test are all capable of performing this calculation. Because there are more than 2 independent variables (3 insecticides), it is less preferable to use the t-test. This is because multiple t-tests would have to be performed, a test comparing each independent variable to one other. Not only does this route take longer, but it also leaves more room for error. ANOVA compares the means of ≥2 independent variables on one dependent variable to investigate if there is a significant difference between the independent variables. ANOVA can only tell that a difference exists, but it cannot tell where the difference is. To find where the difference exists, post-hoc tests should be performed. ANCOVA is similar to ANOVA, except that group means are adjusted by a covariate to adjust for confounding in ANCOVA. Confounding occurs when there is an association between the exposure and outcome that is distorted by another variable, such as age.
Cohort studies, epidemic curves, and longitudinal data collection are epidemiologic tools used in time-series analysis. A time-series is a sequence of measurements and observations made at successive points in time. Time-series analysis interprets this data by recognizing time as the independent variable. The effect is measured at various times, including before and after suspected cause, but is not necessarily used to demonstrate causation of correlation. Another example of time-series analysis is the multiple time-series study, in which a suspected risk factor is introduced to several groups at different times.
Degrees on the Fahrenheit or Celsius scales are examples of interval data. When an interval scale is used, the exact difference between each number is known. However, because there is no true zero, one number that is twice the other number does not have twice the difference. For example, 60°F is not twice as hot as 30°F.
To the contrary ratio numbers have a true zero and exact difference between numbers. For example, 60 meters is exactly twice as long as 30 meters.
A nominal scale does not rank the variables, it merely categorizes them, such as the hair color of people a room.
An ordinal scale categorizes variables by the order they are placed in, even if there is no constant value between the variables, such as the satisfaction scale of patients in a hospital.
The possible grouping of variables in this question is: Underweight (BMI < 18.5%), ideal weight (BMI between 18.5 and 25), overweight (BMI > 25%), and obese (BMI > 30%). This grouping of variables are considered ordinal. If the question asked for the type of variable according to each individual weight, the correct answer would be ratio.
Ordinal variables have an order, but not necessarily equal values between the variables. Consider the pain scale in a hospital, where the difference between one and two may not be the same as six and seven. Similarly, with regards to BMI classification: Obesity > overweight > ideal weight > underweight.
Interval variables are ordered according to value. The difference between ordinal variables and interval variables is that interval variables have set values between the variables. The difference between 67° and 68° is the same as between 97° and 98°.
Nominal variables do not have an order and are grouped in name only. An example of nominal variables are colors: Red, blue, green, etc.
Ratio variables have a true zero, where zero value actually means there is zero of the variable. In ratio variables, two times of a specific value is actually twice as high. For example, 20K is twice as warm as 10K. To the contrary, 20°C is not twice as warm as 10°C
There is no such thing as numerical variables.
The Kelvin temperature scale is an example of a ratio variable. Other examples include measurements in height (meters, feet) and weight (pounds).
For further explanation of nominal, ordinal, interval, and ratio variables, refer to Answers #59 and #60 (directly above).
The binomial distribution curve graphs probabilities of dichotomous, binary variables (not continuous). There is a different binomial distribution for every combination of numbers ( n ) and probability of success ( p ). The larger the number of observations ( n ) and the closer the probability of success ( p ) is to 0.5, the closer the binomial curve appears to the normal curve. If n ≥ 30, many statisticians feel comfortable using the normal distribution in place of the binomial distribution.
When the smaller the p , the further the distribution is skewed to the right. Likewise, the larger the p , the further the distribution is skewed to the left. Even with extreme p , a larger n will approximate the normal distribution.
When the binomial distribution approaches the normal distribution, the following tendency measures apply:
Mean = ( n ) ( p )
Variance = ( n ) ( p ) ( q )
Standard Deviation = ( n ) ( p ) ( q )
n = number of observations
p = probability of success
q = probability of failure
Chi-square uses a hypergeometric probability distribution, where larger numbers accurately follow the distribution. For this reason, chi-square only provides approximate p- values. If a sample size is sufficiently small, the sample size will not follow a chi-square distribution.
Fisher’s exact test shows exact p- values. When larger chi-square numbers are used, the two tests approximate each other. If less than 20% of the cells in a chi-square table have an expected count of <5, or any one cell has an expected count of <1, it is recommended to use Fisher’s exact test.
McNemar’s test may be viewed as a special type of chi-squared, in which the variables are not completely independent (variables in chi-square are independent). McNemar’s test is used to analyze matched pairs or calculate before and after changes in the same variable. While the t-test analyzes continuous variables, McNemar’s test checks for an association between binary/dichotomous variables
The Mann–Whitney U test is a nonparametric test comparable to the two sample t-test. It is used to test the median between two groups. The null hypothesis is that both groups are similar. The alternative hypothesis is that the two populations are different.
The interquartile range is the difference between the 25th and 75th percentiles of the observations.
To calculate the interquartile range, the observations should be placed in ascending order.
1 59, 2 62, 3 62, 4 62, 5 64, 6 65, 7 65, 8 66, 9 66, 10 67, 11 68, 12 69, 13 70, 14 70, 15 71, 16 71, 17 72, 18 73, 19 74
The 25th percentile is calculated by using the formula:
25 th Percentile = ( Number of Observations + 1 ) 4 = 20 4 = 5 th Number = 64
The 75th percentile is calculated by using the formula:
25 th Percentile = 3 ( Number of Observations + 1 ) 4 = 60 4 = 15 th Number = 71
The 25th percentile is then subtracted from the 75th percentile to get the interquartile range: 71 − 64 = 7.
If the interquartile range were to fall between two numbers, an average of these numbers may be chosen to represent a quartile.
As you can see, the interquartile range provides insight to the spread of data, but it ignores a large amount of data.
Due to the small sample population available, normal distribution may not be assumed and a nonparametric test must be used. This immediately eliminates the paired t-test and student’s t-test, which are parametric studies. By comparing two otherwise identical populations, a t-test would have been appropriate if there were the assumption of normalcy. Another important observation of the available data is that there are no specific numbers available. The data only denotes that there is a difference between the two groups, but does not describe how much the difference is. Both Wilcoxon signed rank test and chi-squared test (as do both t-tests) require specific number to perform. The sign test is a nonparametric test that compares dichotomous differences (better/worse or +/−) in data from matched otherwise identical pairs and ignores the magnitude of difference. Related to the Wilcoxon signed rank test, the sign test is an analog to the paired t-test. The null hypothesis in a sign test is that the difference between two groups is zero. In this question, the null hypothesis is that each group tested better than the other group five times.
Please refer to the following table to depict the relationship between parametric and nonparametric tests
Parametric test | Nonparametric test (alternative to parametric test) |
---|---|
Student’s t-test | Mann–Whitney U Test (also known as the Wilcoxon Rank-Sum Test) |
Paired t-test | Wilcoxon signed rank test, Sign test |
ANOVA | Kruskal–Wallis test |
Pearson Correlation | Spearman correlation |
Chi-square (χ 2 ) is a nonparametric test.
Spearman rank correlation coefficient ( r s ) is the nonparametric alternative test to the Pearson correlation coefficient (used to measure linear strength of association between two variables). It works by ranking the X and Y variables according to value and inserting these rankings into the formula used for the Pearson correlation coefficient. In addition to being used for nonnormal continuous data, it can also be used for ordinal data.
Analysis of variance (ANOVA) is used to compare multiple groups simultaneously. The null hypothesis when using ANOVA is that there is no difference between groups. To the contrary, the alternative is that the groups are different. ANOVA does not tell how they are different, only that they are different. After a significant effect has been found through ANOVA, post-hoc analysis is used to tell how the variables differ. In this analysis, post-hoc analysis would determine which coffee is best (and worst).
The sign test is a nonparametric test that compares dichotomous differences (better/worse or +/−) in data from matched otherwise identical pairs and ignores the magnitude of difference. Related to the Wilcoxon signed rank test, the sign test is an analog to the paired t-test. The null hypothesis in a sign test is that the difference between two groups is zero.
Refer to the table in Question #68 (directly above) to see nonparametric alternatives to parametric tests.
Refer to the table in Question #68 (two questions above) to see nonparametric alternatives to parametric tests.
The survival table for this question is shown in the following graph:

To solve this problem, the survival rate from the end of the first three time periods (years) of interest should be multiplied by each other.
Logistic regression is used to find the likelihood of an outcome when the outcome is dichotomous. Dichotomous variables are commonly represented in the form of success/failure, improved/unimproved, or alive/dead.
This question asks to find the overall effect that one independent variable (new medical office) has on two dependent variables (physician satisfaction and patient satisfaction). When there is more than one dependent variable, the situation is said to be multivariate. Furthermore, multivariate tests involve more than one dependent variable. M ultivariate an alysis o f va riance (MANOVA) is a tool used to evaluate multivariate tests and determine significance between groups. MANOVA is an extension of an alysis o f va riance (ANOVA).
When there are multiple dependent variables, they are often times related to one another. In this case, the contentment of each party in the physician–patient relationship likely depends on the contentment of the other party. If one were to perform separate t-tests or ANOVA tests to solve for each dependent variable, this relationship is not properly addressed. MANOVA considers the correlation between dependent variables, reducing distortion from relationships amongst them.
Publication bias refers to the tendency of investigators to publish results that have the desired outcome. Studies that achieve desired outcome are more likely to be published. If an investigator performing a meta-analysis only includes studies that have achieved the investigators’ preconceived notions, the meta-analysis will have a differential error.
Fetal death prior to 20 weeks gestation is defined as an early fetal death, commonly called a miscarriage.
Fetal death between 20 and 28 weeks gestation is defined as intermediate fetal death.
Fetal death after 28 weeks gestation is defined as a late fetal death, commonly referred to as stillbirth.
Maternal Mortality Rate = Number of Pregnancy Related Deaths Number of Live Births × 100 , 000
Although the denominator of this equation should technically be the number of pregnancies, that statistic is not as readily available. For ease of calculation, the number of live births is used. This figure includes pregnancies with more than one child.
The demographic gap is the difference between birth and death rates.
Life expectancy may be calculated at birth or any age afterwards. The overall life expectancy in the United States has been steadily increasing due to public health and medical advancements. Differences of life expectancy between sexes, ethnic groups, and races have been narrowing. Women are expected to live roughly 81.2 years, while men succumb roughly 5 years earlier, at age 76.4. Of course these numbers fluctuate with a plethora of variables accrued over a lifespan.
In the United States overall, the non-Hispanic White population has a higher life expectancy than the non-Hispanic Black population. However, the Hispanic White population has a higher life expectancy than the non-Hispanic White population and likewise for the Hispanic Black population. The difference with Hispanic ethnicity adds on over two years of extended life expectancy to both White and Black populations.
The exact reason for this Hispanic epidemiological paradox is under debate. Although extended life expectancy is typically tied to wealth and education, Hispanics buck this trend. The healthy migrant effect reasons that Hispanic immigrants are generally healthier than those that do not immigrate. Other arguments state that unhealthy Hispanic immigrants to the United States may return to their country of origin prior to death. Other theories suggest that cultural effects may confer a protective risk factor.
The Asian-American population enjoys the longest life-expectancy in the United States.
Active surveillance occurs when the health department takes action to seek out cases of illness. A health department calling healthcare providers to inquire about cases of illness is an example of active surveillance. Passive surveillance is where a health facility or laboratory notifies the health department of a reportable disease.
The NNDSS is a collaboration between local, state, and federal public health agencies to combat notifiable diseases through surveillance, data collection, data analysis, and sharing of public health data. It does this through numerous media, including the maintenance of the National Electronic Disease Surveillance Syndrome (NEDSS).
NNDSS is supported through the Center of Disease Control and Prevention’s Division of Health Informatics and Surveillance (DHIS).
The CSTE is an organization composed of epidemiologists, representing epidemiologists from all of the states and territories of the United States. Together, these epidemiologists collaborate and provide assistance to each other and other public health agencies, such as the CDC.
CTSE maintains a list of notifiable diseases that the states modify and adopt into law. States submit this data to the CDC to help track local trends in infectious diseases.
The CSTE is an organization that represents public health epidemiologists from states and territories. It maintains a list of notifiable diseases that the states modify and adopt into law. In addition to practitioners and laboratories being mandated to report notifiable diseases to the state in a set time period, the state is also asked to submit their notifications to the CDC in a set time period of either 4 hours, 24 hours, or 7 days depending on the type of disease.
The appropriate screening test to identify those at risk of the fatal adverse event would have a high sensitivity [true-positive/(true-positive + false-negative)]. Sensitivity shows the proportion of those that have a disease that are accurately identified as those really having it. In a 2 × 2 tablet, sensitivity is calculated by A A + C . A highly sensitive test helps to rule out a disease because it indicates that a negative test is likely not to have the disease. This can be remembered by the acronym snout ( s e n sitivity + rule out ).
To the contrary, specificity is defined by true-negative/(true-negative + false-positive) and calculated on the 2 × 2 table from B B + D . It represents the proportion of those without a disease that are accurately identified as not having it. A highly specific test helps rule in a disease because it indicates that a positive test is less likely to be a false-positive. Specificity can be remembered by the acronym spin ( sp ecificity + rule in ).
The PPV identifies the probability of those who test positive for the disease to those that actually have the disease.
The NPV identifies the probability of those that test negative for the disease to those that do not have the disease.
Prevalence is the proportion of those that have the disease in the population.
Implementation of a properly conducted screening test is a complex effort. Prior to initiating a screening test, the test itself must be fully scrutinized. Factors that should be evaluated include ethical consequences, psychological consequences, stigmatization, predictive value of results, test validity (and reliability once validity is established), treatment options, economics, and the risk of false-positives or false-negatives. It is also important to figure out how to properly notify the public about the availability of the screening test so that only those in the target population come forward for screening. After results are available, it must be determined how to appropriately disseminate the results.
Ethical and psychological considerations when considering a screening test come in a variety of forms, depending on the test being deliberated. Consider for example, a test that has no available treatment. What type of mental anguish would it cause for someone to know that they have an ailment that cannot be reversed? On the other hand, what if one sibling tests positive for a genetic trait that leads to cancer in everyone that has that trait. Is it ethical to perform a genetic test on one sibling, while the other would rather not have the test performed? Often times there is no correct answer in bioethics. However, it is important to consider the main bioethics principles of autonomy, beneficence, nonmaleficence, and justice.
There are always benefits and drawbacks in medicine. One common drawback is stress and angst related to undergoing the screening process. Those found to be at risk of disease suffer from psychological stress and identify themselves as weak and vulnerable. Meanwhile, self-perceived health is a predictor of future health status.
Another common drawback is false-positive results. The rate of false-positives depends on the test being conducted. A test yielding a large amount of false-positives may be considered acceptable if the benefit of discovering a serious disease is present. This is currently up for debate with prostate cancer, where it is estimated that for every man whose life is saved from prostate cancer by PSA screening methods, 47 men are over-diagnosed and treated, even though they do not need the treatment.
When creating any type of graph in epidemiology, it is important to be as descriptive as possible. It is ideal if a reader can understand the context of the graph by looking at the graph alone. The title should include the type of illness, place of outbreak, and when the outbreak occurred. In addition, the table should be labeled with the dates of incidence on the x-axis and the number of cases on the y-axis.
When charting the annual incidence of a disease, the incidence pattern is the primary focus. This is best displayed by the epidemiologic year, which spans from the month of lowest incidence from one year to another. By placing the lowest incidence at both sides of the graph, it allows the person viewing the graph to appreciate the time leading up to and after the highest incidence. If the highest incidence is plotted in the beginning or end of the curve, the peak incidence is likely to be broken up between the beginning and end of the curve.
An epidemic curve is an investigative tool that describes the patterns of an outbreak. These patterns help to identify the source of the outbreak and potentially how to address it. To compose an epidemic curve, the number of new cases should be plotted against a unit of time, most often days.
Common source—A group of people become ill after being exposed to a point source contaminant. All affected persons become ill within one incubation period. There are no secondary waves, in which people fall ill outside of the first incubation period. Example: Radiation toxicity after a nuclear power plant radiation leak.
Continuous common source—A common source continuously affects those with contact. Example: Soft serve ice cream machine contaminated with Listeria.
Propagated—Infection is transmitted from one person to another. May be direct or indirect contact. Often include waves of secondary or tertiary spread outside of the first incubation period. Rate of propagation depends on herd immunity, opportunities for exposure, and secondary attack rate. Example: Influenza outbreak.
Mixed—Occurs when a common source outbreak is complicated by person-to-person spread. Example: A bacterial conjunctivitis outbreak from a telescope that spreads amongst children at daycare.
Different patterns of disease outbreaks were explained in Answers #87 and #88 (directly above).
To solve this question, it is necessary to build upon the numbers presented in the table. Variables of interest include the attack rate % (number of ill/total number), the attack rate difference (attack rate in exposed − attack rate in unexposed), and the attack rate ratio (attack rate in exposed/attack rate in unexposed).
Burgers have the highest attack rate %, attack rate difference and attack rate ratio, making them the most likely source of the diarrheal illness.
Ate | Did not eat | Comparison | ||||||
---|---|---|---|---|---|---|---|---|
Food | Ill | Well | Attack rate % | Ill | Well | Attack rate % | Attack rate difference | Attack rate ratio |
A. Chicken | 20 | 18 | 52.6 | 22 | 10 | 68.7 | −16.1 | 0.77 |
B. Burger | 32 | 8 | 80.0 | 6 | 22 | 21.4 | 58.6 | 3.74 |
C. Hotdog | 12 | 15 | 44.4 | 15 | 28 | 34.9 | 9.5 | 1.27 |
D. Oranges | 25 | 30 | 45.5 | 6 | 9 | 40.0 | 5.5 | 1.14 |
E. Egg roll | 1 | 5 | 16.7 | 20 | 44 | 31.3 | −14.6 | 0.53 |
Attack Rate = Ill Ill + Well × 100
Attack Rate Difference = Attack Rate of Food Eaten − Attack Rate of Food Not Eaten
Attack Rate Ratio = Attack Rate of Food Eaten Attack Rate of Food Not Eaten
Smallpox is a viral infection that can be transmitted by respiratory droplets and fomites, such as blankets. Although there is no antiviral agent that has been proven to be effective against smallpox, there is a very effective vaccination. This vaccination has been used to eradicate the smallpox virus worldwide. The last naturally occurring case of smallpox was in 1977. Because of this, worldwide immunization ceased in 1980. The vaccination is not used to prevent acute infection.
The key to answering this question correctly is understanding the difference between isolation and quarantine. Isolation insulates people with an infectious illness from those without the illness. Meanwhile, quarantine insulates people who have been exposed to a contagious illness from those without the illness. There are several different types of personal and property quarantine measures. In the United States, government entities have the ability to enforce quarantine and isolation measures.
The incubation period for smallpox is typically 10–14 days. Quarantine should last this long at a minimum. If possible, use of a negative pressure room would be advised.
Although individuals have rights, their liberties may be trumped by the rights of society as a whole to be protected from health threats. For this reason, governments may impose isolation for people showing signs of contagious illness and quarantine for those exposed (and asymptomatic) to the illness. These public health practices protect the public by reducing exposure to infectious disease.
In the United States, legal authority to isolate and quarantine is divided between the states and federal government. If a communicable disease is suspected or present in someone entering the United States, the CDC may issue a federal order to isolate or quarantine. Furthermore, the CDC may issue orders to isolate or quarantine in order to limit spread of disease from one state to another. Each state has their own isolation and quarantine statutes. States may isolate, quarantine, and trace persons with infections disease within their borders. This is commonly performed for tuberculosis.
Public health officials have the legal authority to react swiftly to infectious disease threats. An order to quarantine or isolate does not need advance approval from courts and violation of these orders may result in arrest. Detainees may legally challenge public health orders, but these orders take time and judges have limited jurisdiction and typically defer to medical experts.
For the sake of the rights of society to be protected from health threats, public health officers have the authority to reveal a patient’s condition to those exposed. Similarly, hospitals are obligated to inform health departments of names and contacts of those with specific contagious disease.
The WHO governs disease globally and maintains the International Health Regulations (IHR). These voluntary regulations attempt to limit spread of contagious disease by addressing by influencing political, diplomatic, and trade relationships amongst all WHO member states. IHR is not directly enforceable, but insubordinate nations may face economic and social disruptions from other participating nations.
Nearly every event on Earth can be spatially referenced into geographic data. The GIS are interactive computer-based applications that map geographic data. It describes the way we study the environment and produces spatial data that is used in a variety of industries, including healthcare and public health. Many aspects of health and well-being have spatial dimensions, including health disorders, disease risk factors, health interventions, and health outcomes.
GIS is an important tool in epidemiology, health administration, and health marketing. It may be used to map out any combination of factors to reveal potential correlations between health events. For example, one may use it to look at a cluster of increased disease incidence and compare it to individual demographic characteristics. Equally interesting, it could identify the exposed and unexposed groups to known risk factors for disease. With this information, GIS may help find appropriate places to institute a health intervention.
Epidemic curves are charts that plot the number of people with an infection versus the time at which they get the infection. It is used to identify the origin of an infection and the speed at which it travels through the population.
Gantt charts are an administrative tool to help create a project schedule. On a Gantt chart, each member is assigned a task and a time period to complete that task.
Ishikawa diagrams are also commonly known as cause-and-effect diagrams and fishbone diagrams. An Ishikawa diagram reads from right to left. At the far right of the diagram is the problem to be addressed. Moving to the left, the diagram identifies root causes of the problem(s). These root causes are further broken into sub-causes. Once the diagram has been drawn out, it takes the shape of fish bones.

To calculate the answer, it is easiest to use a 2 × 2 table to determine the unknown values from the known values:

Prevalence is the percentage of a population that has a condition. It is calculated by a + c.
Sensitivity represents the proportion of those that have a disease that are accurately identified as those really having it. In a 2 × 2 tablet, sensitivity is calculated by A A + C .
Specificity represents the proportion of those without a disease that are accurately identified as not having it. In a 2 × 2 tablet, specificity is calculated by D B + D .

The PPV represents the proportion of those who test positive for the disease to those that actually have the disease. In a 2 × 2 tablet, sensitivity is calculated by A A + B .
Using the numbers available in the 2 × 2 table, the PPV is A A + B = 153 153 + 83 = 0.648

In a 2 × 2 table, when prevalence A + C A + B + C + D increases, the PPV A ( A + B ) is increased and the NPV C C + D is decreased.
The sensitivity A ( A + C ) and specificity D ( B + D ) are not affected by changes in prevalence.

In a 2 × 2 table, when prevalence A + C A + B + C + D increases, the sensitivity A ( A + C ) and specificity D ( B + D ) remain unchanged.
However, when prevalence increases, the PPV A ( A + B ) increases and the NPV C C + D decreases.
Specificity is represented by the formula D ( B + D ) . Meanwhile, false-positive is represented as B ( B + D ) . When added together, the specificity and false-positive error rate is equal to 1 or 100%. The false-positive rate is often calculated as (1 – specificity).
Sensitivity is represented by the formula A ( A + C ) . Meanwhile, false-negative error rate is represented as C ( A + C ) .
When added together, the sensitivity and the false-negative error rate is 1.
Sensitivity ( A ( A + C ) ) is the proportion of those with a disease that test positive. As stated in the vignette, nine out of every ten people with the disease test positive. Meanwhile, specificity ( D ( B + D ) ) is the proportion of those without a disease that test negative for it. These two metrics are not to be confused with the PPV and NPV. The PPV ( A ( A + B ) ) is the proportion of those with a positive test result that actually have the disease. Finally, the NPV ( C C + D ) is the proportion of those with a negative result that do not have the disease.
The receiver operating characteristic (ROC) curve can be considered a graph of positive likelihood ratios. The y-axis is the sensitivity, while the x-axis is 1 − specificity, which is the proportion of false-positive results.
The closer the cutoff point is to the upper left corner of an ROC Curve, the higher is the sensitivity and the lower is the false-positive error rate.
When measuring a continuous variable, such as the amount of potassium in a serum metabolic panel, setting the cutoff point between abnormal and normal limits can be a challenge. If the cutoff is too high, there are a lot of false-negative results. If the cutoff is too low, there will be more false-positive results. The ROC curve is a tool used to determine the best cutoff point for a continuous variable. It is a graph composed of the sensitivity along the y-axis and the false-positive error rate along the x-axis.
The false-positive error rate is equal to (1 − specificity). The sensitivity divided by the false-positive error rate is the likelihood ratio + (LR+). Therefore, the ROC curve graphs the LR+.
Recording vital statistics (birth, marriage, divorce, and death) is a responsibility of the states, two select cities (Washington D.C. and New York City) and United States territories. These entities share their vital statistics with the National Vital Statistics System (within the NCHS).
The US Outpatient Influenza-like Illness Surveillance Network (ILINet) receives information regarding the number of patients seen overall and the number of Influenza-Like Illness (ILI) seen from roughly 2000 outpatient healthcare providers weekly. This information is stratified by age group and evaluated for changes in trends.
ILINet case definition includes the following: Fever > 100°F (37.8°C), cough, and/or sore throat. If a patient has these signs/symptoms and is found to have a noninfluenza illness, it is not reported to be ILI. If a flu test is positive, it will be reported as influenza-like illness.
The NHANES is a program designed to assess the health and nutritional status of residents of the United States. It is a survey conducted by the NCHS, which is a part of the CDC. The data gathered from NHANES helps determine risk factors and prevalence of disease seen in the United States. This input has been used to influence health policy affecting individuals and the general public. Examples of changes influenced by NHANES include removing lead from gasoline, establishing baseline estimates for serum cholesterol and use of height/weight percentiles.
Information gathered for NHANES is exclusively through home interviews and standardized physical exams in mobile exam centers. Physical exams vary depending on age, gender, and medical history.
NHANES participants are chosen at random, based on their community, which is further divided into neighborhoods. After being screened for eligibility, there are nearly 5000 people surveyed each year. This means each participant represents approximately 50,000 US residents.
The BRFSS is performed exclusively through a telephone survey.
The best option listed for this student is to access a pregnancy medication exposure registry. With restricted resources, the student will be limited in conducting his own study. Furthermore, conducting an RCT to find medication adverse events is controversial in pregnant women. Pregnant women are usually excluded from pharmaceutical clinical trials, so information on medication adverse events is limited. Therefore, the Food and Drug Administration supports pregnancy exposure registries for reporting of adverse events discovered after taking specific drugs.
Departments of vital statistics are often times mandated to only cover a combination of statistics related to birth, death, fetal deaths, marriages, and separations. This data would not be adequate to evaluate the risk of adverse events related to pharmaceuticals taken during pregnancy.
The International Classification of Diseases (ICD) is published by the WHO. This classification outlines an international catalog of diseases, disorders, and injuries into a universal common language. It is used by epidemiologist, health administrators, and clinicians in over 100 countries around the world to study population-wide disease patterns and healthcare outcomes. This data is used to adjust the way healthcare is provisioned and practiced. Health-related variables recorded include vital records, reason for physician encounters, morbidity, and mortality. ICD is also widely used as a basis for resource allocation, including reimbursements in the United States.
In the United States, Black individuals experience the highest IMR, while the lowest IMR is experienced by Asians and Pacific Islanders.
Obstetrical-related data are important metrics used to compare health status among different populations. The most widely compared obstetric metric is the IMR, which widely serves as a surrogate marker for the overall status of a health system.
The neonatal mortality rate takes into consideration deaths that occur within the first 28 days of life. This is an important metric, as the majority of infant deaths typically occur shortly after birth.
A list of obstetric oriented rates has been listed below:
- • Fetal Death Rate = T o t a l N u m b e r o f F e t a l D e a t h s ( I n t e r m e d i a t e & L a t e ) i n a g i v e n t i m e p e r i o d T o t a l N u m b e r o f L i v e B i r t h s D u r i n g t h e S a m e P e r i o d o f T i m e × 1000
- • Infant Mortality Rate = T o t a l N u m b e r o f D e a t h s o f I n f a n t s ( < 1 - y e a r O l d ) i n a G i v e n T i m e P e r i o d T o t a l N u m b e r o f L i v e B i r t h s D u r i n g t h e S a m e P e r i o d o f T i m e × 1000
- • Maternal Mortality Rate = D e a t h s D u e t o P r e g n a n c y − R e l a t e d I l l n e s s i n a G i v e n T i m e P e r i o d T o t a l N u m b e r o f L i v e B i r t h s D u r i n g t h e S a m e P e r i o d o f T i m e × 100 , 000
- • Neonatal Mortality Rate = T o t a l N u m b e r o f D e a t h s o f N e o n a t e s ( < 28 D a y s O l d ) i n a G i v e n T i m e P e r i o d T o t a l N u m b e r o f L i v e B i r t h s D u r i n g t h e S a m e P e r i o d o f T i m e × 1000
- • Perinatal Mortality Rate = N e o n a t a l D e a t h s + F e t a l D e a t h s ( I n t e r m e d i a t e & L a t e ) i n a G i v e n T i m e P e r i o d T o t a l N u m b e r o f L i v e B i r t h s a n d F e t a l D e a t h s D u r i n g t h e S a m e P e r i o d o f T i m e × 1000
The IMR is an important health statistic internationally. Although it includes many variables, it is used as a key measure to compare health systems between countries, regions, and even cities. IMR is recorded as the number of deaths occurring per thousand live births that occur before the first birthday. The IMR in the United States typically hovers around 6.0. This number has been declining over the decades.
The WHO estimates that the global IMR is roughly 32 deaths per 1000 live births. This number is a vast improvement from 30 years ago, when the IMR was double today’s numbers.
Worldwide, nearly 75% of all deaths under five years of age occur within the first year of life.
The US Census Bureau is a government organization mandated by the constitution with the mission to serve as the leading source of quality data about the country and economy. The Census Bureau conducts a population and housing census once every ten years. It also conducts an economic and government census every five years. In addition, it conducts a community survey annually. The information is used to distribute resources adequately to fund programs for public health, education, neighborhood improvements, etc.
The Youth Risk Behavior Surveillance System (YRBSS) is a nationwide high and middle school-based survey conducted by the CDC in conjunction with all other levels of government. It monitors behaviors that contribute to morbidity and mortality in America’s youth. Classifications of behavior surveyed include actions leading to violence and injury, sexual decision making, alcohol use, tobacco use, drug use, diet, physical activity, and prevalence of asthma.
The Behavior Risk Factor Surveillance System (BRFSS) is the largest telephone survey in the world, with over 400,000 surveys annually. It conducts telephone surveys monthly in all 50 states, the District of Columbia, the US Virgin Islands, Guam, American Samoa, and Puerto Rico. Amongst many individual state and federal uses for monitoring trends, BRFSS data monitors progress towards Healthy People objectives. Individual surveys consist of core questions, rotating core questions (asking every other year), and additional standardized modules on specific topics determined by the state.
Each of these answers describes a different type of study. Only answer D is an example of an experimental study. An experimental study is one in which the investigator has control over the exposure of the intervention being studied. The other four options are all examples of observational studies, in which the investigator observes, without intervening.
The difference between experimental studies and nonexperimental studies is that investigators have control over the exposure in experimental studies. For this reason, experimental studies are considered to be the gold standard, secondary to meta-analysis studies, which analyze data from numerous peer-reviewed studies.
It is not always possible to control the exposure. Quasi-experimental studies are utilized when an investigator only has partial control over the study. For example, an investigator may wish to investigate a hypothesis by creating an exposure to a cohort that experienced of a nonreproducible natural disaster and compare it to a control that was not in the disaster.
To answer this question, it is important to understand the definitions of both risk and rate. Risk is defined as the number of subject achieving the qualifying event (death in this example) during the defined time period. Assuming there is no attrition, the denominator (subjects at risk of qualifying event) does not change. To the contrary, the rate is the number of qualifying events that occurring during the defined time period divided by the average number of subjects at risk. The average subjects at risk used in this equation is typically the number of subjects at risk during the halfway mark of the time period.
In this question, the rats exposed to Drug A died early in the study. Therefore, there were fewer rats susceptible to death at the halfway point (because they had already died.) When more deaths take place in the early period, the number of subjects at risk during the halfway point is lower. When the denominator is lower, the rate will increase, and vice versa.

A rate must have a numerator and a denominator. The numerator typically displays the event of interest, while the denominator will establish the population at risk. Because there is no denominator in answer A, this statistic is not considered to be a rate.
Rates are either crude or specific. Crude rates use the entire number of events without breaking it down into subgroups. Meanwhile, specific rates are created by using subgroups such as hair color and age.
Life expectancy can be described as the average number of years of life one can expect to live based on mortality rates. It can be calculated from any stage of life. The life expectancy at birth is considerably lower than life expectancy of the same person at a later age. The reason for this is that those still living at the later age will have avoided mortality at a younger age, while others in the same cohort will have died. The life expectancy at birth takes account of all of those that will suffer premature death.
Between the ages 1 and 44, unintentional injuries are the leading cause of death. Within this group, motor vehicle accidents are the most common specific cause. Heart disease is the leading cause of death in the United States, but typically affects the elderly.
Years of potential life lost (YPLL) is a measure of premature mortality. It statistic emphasizes deaths that occur at an earlier age and deemphasizes deaths that occur later in life.
The YPLL is calculated as follows:
When there are less than 20 deaths, it is easiest to subtract the age of death from the endpoint. In the United States, the endpoint is usually determined to be 75 years of age. The difference between age of death and the endpoint represents the YPLL from one person. YPLLs from all individuals in consideration are then added together to get the aggregate YPLL. If a 17-year-old and 20-year-old die, the total YPLL would be 113. This is calculated as follows:
75 − 17 = 58 YPLL ( 1 ) 75 − 20 = 55 YPLL ( 2 ) YPLL ( 1 ) + YPLL ( 2 ) = 113
When there are more than 20 people in consideration, it is easier to calculate YPLL through frequency tables. It is calculated by dividing ages into the following groups: Under 1 year, 1–14 years, 15–24 years, 25–34 years, 45–54 years, 55–64 years, and 65–74 years. Each age group is then identified by the midpoint. That midpoint is then subtracted from the endpoint (usually 75). The difference between the midpoint and endpoint is then multiplied by each person that falls within the group. For example, if a 17-year-old and 20-year-old were to die in a motor vehicle accident, this would tally into the 15–24 group. The midpoint of this group is 19.5, which is subtracted from 75 to get 55.5. Finally, this number is multiplied by two, because there are two people in this age group being calculated. Therefore, the YPLL from this group could be calculated as 110.
The leading causes of YPLLs in descending order are cancer, unintentional injuries, heart disease, and respiratory infections. Note that although heart disease is the leading overall cause of death, most deaths caused by heart disease are older and do not contribute on an individual basis as greatly to YPLL.
Years of potential life lost (YPLL) is explained in Question #118 (directly above).
YPLLs for the five options are listed below:
Option A: | Because all 10 patients are older than 75, there are no YPLLs. |
Option B: | 75 − 10 = 65 YPLL (Total) |
Option C: | 75 − 50 = 25 YPLL (1) |
75 − 48 = 27 YPLL (2) | |
YPLL (1) + YPLL (2) = 52 YPLL (Total) | |
Option D: | 75 − 35 = 40 YPLL (1) |
75 − 55 = 20 YPLL (2) | |
YPLL (1) + YPLL (2) = 60 YPLL (Total) | |
Option E: | 75 − 68 = 7 YPLL (1) |
75 − 68 = 7 YPLL (2) | |
75 − 68 = 7 YPLL (3) | |
75 − 68 = 7 YPLL (4) | |
YPLL (1) + YPLL (2) + YPLL (3) + YPLL (4) = 28 YPLL (Total) |
When calculating years of potential life lost (YPLL) for more than 20 people in consideration, it is easier to calculate YPLL through frequency tables. It is calculated by dividing ages into the following groups: Under 1 year, 1–14 years, 15–24 years, 25–34 years, 45–54 years, 55–64 years, and 65–74 years. Each age group is then identified by the midpoint. That midpoint is then subtracted from the endpoint (usually 75). The difference between the midpoint and endpoint is then multiplied by each person that falls within the group.
The following table calculates the YPLLs of the 50 gas leak victims.
People killed in gas leak in underground subway | ||||||||||
---|---|---|---|---|---|---|---|---|---|---|
(1) Age group | <1 | 1–14 | 15–24 | 25–34 | 35–44 | 45–54 | 55–64 | 65–74 | >75 | Sum |
(2) Midpoint of group | 0.5 | 7.5 | 19.5 | 29.5 | 39.5 | 49.5 | 59.5 | 69.5 | ||
(3) Difference of midpoint to endpoint | 74.5 | 67.5 | 55.5 | 45.5 | 35.5 | 25.5 | 15.5 | 5.5 | ||
(4) Number of deaths | 5 | 5 | 5 | 5 | 5 | 5 | 5 | 5 | 10 | 50 |
(5) Difference x deaths (lines 3 × 4) | 372.5 | 337.5 | 277.5 | 227.5 | 177.5 | 127.5 | 77.5 | 27.5 | 1625 |
The population growth of the population pyramid pictured in the question represents a declining population. As is often seen in developed nations, there is a larger population of older individuals than younger ones. A smaller group of younger individuals signifies that the birth rate has slowed down. When the birthrate equals the death rate, the population pyramid exhibits parallel vertical lines on both sides. When a population is growing, as seen in developing nations, the pyramid appears as a triangle with the base at the bottom wider than the peak at the top.
Sick building syndrome (SBS) is a constellation of symptoms that occurs in workers continuously exposed to indoor environments. Symptoms include dry skin/mucous membranes, pruritus, mental fatigue, headache, and airway infections that are more pronounced during exposure to the indoor workplace. These symptoms lead to a large economic impact due to absenteeism, presenteeism, litigation, and workers’ compensation claims. There are numerous risk factors contributing to SBS, including overly populated buildings, presence of carpet within the building, presence of mold/dust, and psychiatric stress. Due to this varied etiology and lack of specific biological markers, the prevalence of SBS is hard to determine.
This question asks to find the risk difference (RD):
Risk Difference = Risk in exposed − Risk in unexposed
RD may be performed with point prevalence, cumulative incidence, or incidence rates.
Risk difference is positive when the risk in the exposed population is greater than the risk in the unexposed. It is negative when the risk in the exposed group is less than the risk in the unexposed group. In this problem, the new AC system is considered to be the exposure.
To calculate the RD for this equation, you must first calculate the risk in the exposed and unexposed groups. Knowing that there are 1000 employees, there are 225 employees in the unexposed group. This means that the point prevalence rate of SBS is 225 1000 = 0.225 . After the installation of the new AC unit, the prevalence dropped to 125 1000 = 0.125 .
These numbers are the plugged into the risk difference equation:
The risk difference is negative when the exposed population is less than those without the exposure.
To solve this problem, one must use the following equation:
Plugging in the available data:
Vaccine efficacy can also be found through the formula:
Vaccine Efficacy % = 1 − RR × 100
In this case, the data can be summarized into the following table:
Develop mononucleosis | Do not develop mononucleosis | ||
---|---|---|---|
Mononucleosis vaccine | 80 | 120 | |
Placebo | 80 | 20 | |
Using this 2 × 2 table, the risk ratio may be calculated;
Risk Ratio ( RR ) = a a + b c c + d = 80 200 80 100 = 0.5 = 50 %
Vaccine efficacy is a useful calculations for ideal conditions, while Vaccine effectiveness better demonstrates how vaccinations work in the real world. Vaccine effectiveness accounts for variables such as vaccine storage, vaccine administration, access to care, and cultural barriers to vaccination.
The Attributable risk (AR) is also known as the risk difference. It is the risk in the exposed, minus the risk in the unexposed. It does not give the percentage, as asked for in the question. Attributable risk percentage (AR%) is able to give the percentage of risk attributed to a specific risk factor, in those that are exposed to the risk factor, as asked for in the question. Meanwhile, the population attributable risk percentage (PAR%) is able to give the percentage of risk attributed to a specific risk factor in the general population, not just the group exposed to the risk factor. Note that in the second PAR% equation below (fourth equation), the variable “effective proportion” is added to the second AR% equation to specify the population at risk, in this case red meat consumers.
The attributable risk percent is represented by the following two equations;
The population attributable risk percent is represented by the following two equations;
The relative risk (also known as the risk ratio) represents the risk amongst those exposed to a risk factor, compared to those unexposed. The vignette explains that 15% of those exposed had brain cancer, while 10% of the unexposed had brain cancer. The RR for this problem could be solved as follows:
Relative Risk ( RR ) = Risk Among Exposed Risk Among Unexposed = 0.15 0.1 = 1.5
The relative risk may also be completed by competition of a 2 × 2 table:

Filling in the information from the vignette:

Solving for the missing information:

The relative risk (risk ratio) shows that those exposed to Chemical X are 1.5 times more likely to be diagnosed with brain cancer than those not exposed to Chemical X. Because the RR is >1, there is said to be a positive association between Chemical X and brain cancer. When RR is equal to 1, there is no relationship between the two variables. Finally, when RR <1, there is a negative association.
When possible, the risk ratio ( RR ) is the preferred method of analysis of risk. Cohort studies are best calculated with RR . However, the risk ratio cannot be calculated directly from a case-control study. The odds ratio ( OR ) is the best tool for risk analysis of case-control studies. It is important to remember that as the OR is only a reliable estimator of the RR if the prevalence of disease is less than 5%.
The OR is also used in other methods of analysis, including calculating the logistic regression and Cox regression analysis.
The PRAMS is operated by the CDC in conjunction with state health departments in order to identify healthcare trends in pregnant women and women that have just given birth. It provides state-specific, population-based data on maternal attitudes and experiences prior-to, during, and after pregnancy. This data is used by health professionals to monitor and track healthcare goals in addition to formulate opportunities for improvements in care.

The odds of an event considers the chances that one event will occur compared to another. The odds of square A to square B would be compared as A:B. This is opposed to the risk, which includes the denominator and would be written as a a + b . When the prevalence (A+C) is small, the Odds Ratio ( A × D B × C ) is similar to the Risk Ratio ( a a + b c c + d ) . As prevalence grows, the denominator of the risk ratio also grows (while odds remain the same) and the odds ratio will no longer approximate the risk ratio.
In summary, the odds ratio is a good estimate of the risk ratio when the prevalence of a disease is low. Some investigators are willing to accept a 10% prevalence, while others feel that the odds ratio does not approximate the risk ratio if the prevalence is greater than 5%.
To answer this question correctly, the table must be completed, as shown below. To better depict all that is truly occurring in a 2 × 2 table, the borders have been added.

The standard 2 × 2 table is then completed based off of information available in the vignette:

Once the incomplete squares are calculated, the final table may be completed:

In case-control studies, the risk is calculated through the use of the odds ratio
Odds Ratio = ad bc
When solved for this problem,
Odds Ratio = ( 7 ) ( 100 ) ( 20 ) ( 52 ) = 0.67
The people using the supplement are 0.67 as likely to experience constipation as those not taking the supplement. Because this number is below 1, the supplement is considered to be a protective factor of constipation.
In a sufficient cause, the disease will always occur if the cause is present. In a necessary Cause, the cause must be present for the disease to occur.
Cirrhosis is not always a result of alcohol and not everyone that consumes alcohol will develop cirrhosis. Therefore, alcohol is not sufficient or necessary for cirrhosis.
Healthcare is necessary, but not sufficient for improved healthcare. This was the conclusion of “Health care: Necessary but not sufficient,” a brief from the Center of Society and Health in conjunction with the Robert Wood Johnson Foundation.
Necessary and sufficient are terms used to describe correlation between two variables, once causality has been established. For something to be necessary, it must be present to produce change. The change does not occur unless the necessary factor is present. For something to be sufficient, the presence of that factor will always bring change. Consider Mycobacterium tuberculosis: It is both necessary and sufficient for miliary tuberculosis.
Differential and nondifferential error are classified as the two types of misclassification bias.
Nondifferential misclassification occurs when the frequency of errors are the same in both populations being compared. This coincides with option A, where both the exposed and unexposed subjects are misclassified. Nondifferential error typically reduces the effect of the association and brings the measured association back to the null. An example of nondifferential bias would be analysis of incomplete medical records. More specifically, if a dichotomous variable such as cigarette smoking were left blank, the bias would be nondifferential.
Meanwhile, differential misclassification occurs when the misclassification occurs more in one of the groups being compared than the other. Differential misclassification may influence the association either towards or away from the null. Going back to the previous example of incomplete medical records, if each patient’s chart was indiscriminately checked off for being a nonsmoker to save time, this would be an example of differential bias.
Option C is an example of recall bias. People that have had a memorable event (such as mothers of children with birth defects) will typically think harder to recall that event than those that did not experience the event. Because the ill and nonill groups may provide different recollection of their experience recall bias is a type of differential misclassification.
The sample group in this study represents a small spectrum of the population. In a drug that is potentially marketed to men and women of age that places them at increased risk of cardiovascular disease resulting from dyslipidemia, this study only evaluated men between the ages 57 and 60. External validity (also known as generalizability) occurs when results of an observation hold true in different situation. The sample population in clinical studies should represent the target population that is to be treated. Due to the limited variation in this study sample, the trial lacks external validity.
With the information provided in the vignette, there is every reason to believe that the conclusion of the study accurately represents the population that was studied. When the conclusions accurately represent the population being studied, the study is said to be internally valid. Internal validity depends on the study design, data collection, and analysis of data.
There is great clinical significance in controlling dyslipidemia and reducing cardiovascular events.
Statistical significance is demonstrated via the alpha value.
Vaccine efficacy is obtained through studies, while vaccine effectiveness is how vaccinations perform in the real world. All of the above options are real-life variables that work to decrease the efficacy of vaccines.
Sensitivity analysis is the process of examining how expected outcomes change when they are placed under different assumptions.
Attributable risk is used to determine what effect an exposure to a risk factor has on the effect of the population.
Data organization is a general term that is not specific to sensitivity analysis.
Standard deviation is a measure of dispersion, which biostatisticians use to see how far spread out the numbers in a population are.
- • Immunogenicity—The ability to produce an immune response and protection from reinfection of a pathogen.
- • Infectivity—Ability to cause an infection. Measured by the number of infectious particles required to cause infection.
- • Pathogenicity—Ability of microbial agent to induce disease.
- • Secondary attack rate—The proportion of susceptible people that contract a disease after exposure from an infected person. It is a measure of the infectivity.
- • Virulence—Severity of the infection after the disease occurs. Measured by case fatality or severe morbidity.
Influenza is an RNA virus in the Orthomyxoviridae family. It is composed of hemagglutinin and neuraminidase proteins that are directly involved in the infectivity of the influenza virus. The two component proteins are continuously undergoing changes. When these changes are abrupt the genetic changes generate a new set of amino acids, leading to different proteins and a new influenza strain that may differ greatly from immunity provided from vaccination or previous exposure. Dramatic changes to the influenza virus are known as antigenic shift and are more often responsible for particularly severe influenza seasons. Less dramatic changes are called antigenic drift. In this case, the population typically carries a greater immunity from vaccinations and prior exposure of influenza strains that were similar to the currently circulating one.
The current outbreak of influenza in chickens can best be described as epizootic. An epizootic is an increase in the usual prevalence of a disease in an animal population, an animal disease outbreak.
An endemic is when the prevalence level of a disease in a human population is regular and constant.
An enzootic is when the prevalence level of a disease in an animal population is regular and constant.
An epidemic is an unusual increase in the occurrence of a disease. Even one case of a disease may be considered epidemic, such as would be the case if there was a confirmed polio diagnosis in the United States.
A pandemic is when a disease affects more people than usual and affects many regions and nations.
Animal and human disorders may interact. Sometimes enzootic trends can trigger epidemics in humans.
The steps in an Epidemiologic Outbreak Investigation are as follows;
- 1. Confirm that an outbreak is occurring
- 2. Establish a diagnosis
- 3. Establish a case definition
- 4. Investigate the number of cases
- 5. Analyze the data and characterize the epidemic
- 6. Develop a hypothesis
- 7. Test the hypothesis
- 8. Implement action for control
- 9. Monitor prevention measures
The ED 50 represents the smallest dose that is effective in 50% of the test population. Meanwhile, the LD 50 represents the dose that is lethal in 50% of the test population. If the ED 50 and the LD 50 are close together, the same dose (volume of drug) that produces a beneficial effect in half of the population will also cause death in half of the population. Contrarily, if the ED 50 and LD 50 are far apart, the drug has more room for error.
The LD 50 is a poor indicator of health effects because death is one of the most undesirable outcomes of toxicity. Depending on the situation, a more preferable measurement is the TD 50 , the dose at which the drug is toxic to 50% of the population. As with the LD 50 , comparing the TD 50 to the ED 50 can yield important safety information.
Other important acronyms to know are NOAEL and LOAEL. NOAEL stands for “no observed adverse effect level,” while LOAEL stands for “lowest observed adverse effect level.”
The National Childhood Vaccine Injury Act requires clinical professionals and vaccine manufacturers to report vaccine associated events to the US DHHS. To accomplish this, the VAERS was developed by the CDC and FDA.
The objectives of VAERS are as follows:
- • Detect new vaccine adverse events
- • Monitor trends in vaccine adverse events
- • Identify risk factors for vaccine adverse events
- • Identify vaccinations with high adverse event rate that were manufactured in the same batch
- • Assess and monitor the safety of newly licensed vaccines
Organizations that conduct research on human subjects are required to create an IRB to evaluate the potential risks and benefits of human experimental research. In order to approve human research experiments, the IRB must evaluate the full proposed study. The IRB members must have an understanding of the science behind the research and the legislation regarding human research. The IRBs also assure that human subjects receive appropriate informed consent and fully understand their involvement in the research process.
Informed consent is an educational process by which a person makes an educated decision to participate or not participate in a procedure. To adequately obtain informed consent, several factors must be in place.
The individual must be legally allowed to make a decision. For example, minors are typically unable to make a decisions that lead to informed consent.
Informed consent requires presumption of competence. This presumption implies that a person can comprehend information, understand risks and benefits, exercise judgement, and make a decision based off the information.
Informed consent requires voluntary decision making that is free of coercion. If there are external factors influencing the decision making process, the decision may not be independent and cannot be considered appropriate for informed consent.
Finally, informed consent requires the full disclosure of all relevant information. An informed decision cannot be made if there is missing information.
It is considered unethical not to use a screening test that has been shown to save lives. Consider mammography as an analogous example to the screening test in this question. Because it is known to be effective at detecting breast cancer, many groups would consider it unethical to not use screening mammography to screen breast cancer for research purposes. On the other hand, mammography often leads to harms in the form of stress to the patient and unnecessary surgical procedures.
The NSDUH is housed within the Substance Abuse and Mental Health Administration (SAMHSA). It is the nation’s primary source of information on patterns, prevalence, and consequence of drug use and mental disorders in the noninstitutionalized population, age 12 and older. NSDUH questions include alcohol, marijuana, tobacco, and all other illicit drugs. The study gathers data through face-to-face interviews at the place of residence and does not include incarcerated prisoners, homeless people not living in shelters, or military personnel on active duty.
The NSDUH is a key source of information to provide complimentary information to the BRFSS.
SAMHSA is the operating division under the DHHS that aims to reduce the public health impact of mental illness and substance abuse in the United States. The DAWN records hospital emergency room information in order to provide surveillance of trends in drug use. Meanwhile, SAMHSA’s National Study on Drug Use and Health (NSDUH) tracks patterns and consequences of alcohol, tobacco, illicit drugs, and mental illness in the United States through random interviews.
The BRFSS is conducted by the CDC to monitor health-related risk behaviors, use of preventive health services and status of chronic health conditions. Like SAMHSA, CDC is one of the operating divisions under the DHHS.
The DOJ contains the Drug Enforcement Agency, which originally founded DAWN. However, DAWN has been fully transferred to SAMHSA. The DOJ used to maintain the National Drug Intelligence Center (NDIC), which predicted future drug use trends via a national drug threat assessment. The NDIC is no longer in operation.
The ONDCP is a component of the Executive Office of the President of the United States. ONDCP advises the president on drug issues and coordinates activities to control illicit drug use. Additionally, the ONDCP composes the annual National Drug Control Strategy, which describes efforts to reduce drug use, drug distribution, drug-related violence, and health problems related to illicit drugs.
The IMR in the United States hovers around six deaths per 1000 live births. Of these deaths, the largest percentage is due to congenital malformations. Low birth weight is the second leading contributor to IMR in the United States. After these top two causes of infant morbidity, there is a significant drop in infant specific causes of death. Other common contributors to IMR include maternal complications, SIDS, unintentional injuries, placental complications, sepsis, and respiratory distress.
Bibliography
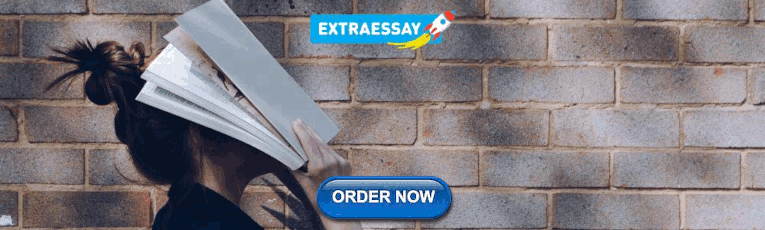
COMMENTS
The value of our health and medical research investment is at risk unless we foster the discipline of biostatistics. Every year, Australia's National Health and Medical Research Council (NHMRC) spends around $800 million on medical and public health research,1 much of which depends critically on the correct analysis and interpretation of data. We argue here that the value of our health ...
Biostatistics are applied statistics in biological and medical sciences for public health practice. Based on the data and models they provide, epidemiology — the study of the causation, spread and control of disease across time and space — gives us information about health status, morbidity and mortality in human populations.
Biostatistics is the application of statistical techniques for scientific research in health-related fields, including medicine, biology and public health. It also encompasses development of novel methodologies that translate to better study design and analyses. ... Associate Professor of Biostatistics in Population Health Sciences; kaz2004@med ...
bīōstəˈtistiks/. noun. the branch of statistics that deals with data relating to living organisms. Making sense of all the data. That's one way of defining what a biostatistician does. Or, as Professor and former Department Chair Patrick Heagerty likes to put it, "Turning data into knowledge.". Biostatisticians use statistical methods ...
Biostatistics can help identify the best way to deploy resources to treat populations. To control an epidemic, the goal is not only finding the best way to treat an infected person, but also to control spread in the population. In both infectious diseases and behavioral research, interventions provided to individuals may well impact others in ...
Abstract. Collecting, analyzing, and interpreting data are essential components of biomedical research and require biostatistics. Doing various statistical tests has been made easy by sophisticated computer software. It is important for the investigator and the interpreting clinician to understand the basics of biostatistics for two reasons.
Why Biostats? Biostatisticians play a unique role in safeguarding public health and improving lives through quantitative research. By combining across quantitative disciplines, biostatisticians are able to collaborate with other biomedical researchers to identify and solve problems that pose threats to health and to quality of life.
Associated Data. Data Availability Statement. The key role of statistical modeling in epidemiology and public health is unquestionable. The methods and tools of biostatistics are extensively used to understand disease development, uncover the etiology, and evaluate the development of new strategies of prevention and control of the disease.
What We Do in the Department of Biostatistics. The Bloomberg School's Department of Biostatistics is the oldest department of its kind in the world and has long been considered one of the best. Our faculty conduct research across the spectrum of statistical science, from foundations of inference to the discovery of new methodologies for health ...
DESCRIPTION. This book describes the fundamental concepts used in public health research, including epidemiology of diseases, study designs, and statistical methods. The author is a renowned biostatistician with a strong background in teaching biostatistics and mines her experience with the Framingham study to provide examples and illustrations.
The OHSU-PSU School of Public Health is home to the Biostatistics & Design Program (BDP), an OHSU Research Core dedicated to providing high quality biostatistics collaboration, with particular expertise in population science. Several of our Biostatistics faculty are involved in the BDP, as are a number of masters and PhD level staff ...
Biostatistics is the exciting field of development and application of statistical methods to research in health-related fields, including medicine, public health and biology. Since early in the 20th century, biostatistics has become an indispensable tool for understanding the cause, natural history and treatment of disease in order to improve ...
The data behind biostatistics can be obtained through health-related information, such as medical records, or vital statistics, such as birth and death dates. Biostatisticians may gather data through sources that provide specific patient information, such as insurance claims; by researching peer-reviewed journals; or by conducting simple surveys.
Basics of Biostatistics. Application of statistical methods in biomedical research began more than 150 years ago. One of the early pioneers, Florence Nightingale, the icon of nursing, worked during the Crimean war of the 1850s to improve the methods of constructing mortality tables. The conclusions from her tables helped to change the practices ...
Measurements of Accuracy in Biostatistics. Haiying Wang, ... Huiru Zheng, in Encyclopedia of Bioinformatics and Computational Biology, 2019. Abstract. Due to the nature of biological and medical data, biostatistics has been playing an increasing role in a wide range of applications in biology and medicine. The aim of this article is to provide insights on some basic concepts and measurement ...
17 Department of Preventive Medicine and Population Health, University of Texas Medical Branch, Galveston, TX, USA ... Members of the Biostatistics, Epidemiology, and Research Design Special Interest Group of the Association for Clinical and Translational Science used a consensus approach to identify the elements of research protocols that a ...
Biostatistics mainly addresses the development, implementation, and application of statistical methods in the field of medical research [].Therefore, an understanding of the medical background and the clinical context of the research problem they are working on is essential for biostatisticians [].Furthermore, a specific professional expertise is inevitable, and also soft skill competencies ...
Biostatistics plays a vital role in several domains of public health, including disease surveillance, epidemiology, clinical trials, health services research, environmental health, and policy evaluation. Its scope of application ranges from analyzing disease patterns and identifying risk factors to evaluating the effectiveness of interventions ...
A basic understanding of statistical concepts is necessary to effectively evaluate existing literature. Statistical results do not, however, allow one to determine the clinical applicability of published findings. Statistical results can be used to make inferences about the probability of an event among a given population. Careful interpretation by the clinician is required to determine the ...
The Biostatistics and Population Health Section of the Biomedical Informatics Department is committed to harnessing the power of data to profoundly impact healthcare and public health. Through rigorous research and collaboration, our team explores the intricate realms of biostatistics and population health to drive innovation, expand knowledge ...
Members of the Biostatistics, Epidemiology, and Research Design Special Interest Group of the Association for Clinical and Translational Science used a consensus approach to identify the elements of research protocols that a biostatistician should consider in a review, and provide specific guidance on how each element should be reviewed.
We have Dr. Cavanaugh, one of our biostatistics professors, on the show today to talk with us about just that. Dr. Cavanaugh has published more than 160 peer-reviewed papers, and his research contribution span a wide range of fields, from cardiology to health services utilization to sports medicine to infectious disease, just to name a few.
Epidemiology and biostatistics are the cornerstone of public health and preventive medicine. These practices use mathematical, scientific, and social methods to monitor disease trends and provide intervention to prevent future disease. [147 questions.] Keywords: Epidemiology, biostatistics, statistics, odds ratio, risk ratio, rate, analysis ...