Power Electronics Talks
Power Electronics, Power Factor Correction, Ferrites, Power Converters, Power Electronics Components, Power Electronics Applications, Standards and Testing, Product Review, Tools and Calculators
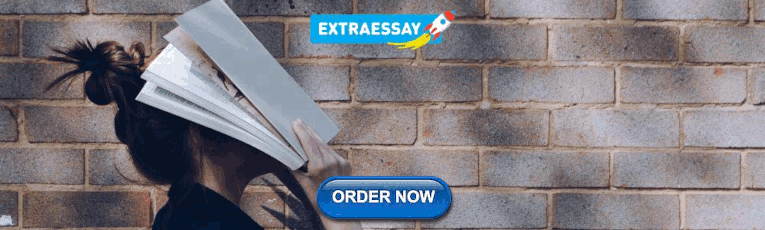
Hysteresis loop or B-H curve and Hysteresis loss
What is hysteresis loop or bh curve and hysteresis loss, hysteresis loop.
Hysteresis loop or B-H curve |
- The loop is produced by measuring the magnetic flux (B) of a ferromagnetic material when the applied magnetizing force is changed (H).
- A ferromagnetic material which has been never before magnetized or demagnetized ferromagnetic material will trail the dashed line (see the figure) as magnetizing force (H) is increased.
- The dashed line shows that, the larger the quantity of current applied (H+), the stronger the magnetic field in the component (B+).
- At "a" point nearly all of the magnetic domains are aligned and an extra increase in the magnetizing force will generate very little increase in magnetic flux.
- The magnetic saturation point has been reached for the material.
- When magnetizing force (H) is decreased to zero, the curve will move or change from "a" point to "b" point.
- At this point, we can notice that some magnetic flux leftovers in the material even though the magnetizing force (H) is zero. This is called as the point of retentivity on the graph and shows the remanence or level of remaining magnetism in the material. Some of the magnetic domains stay aligned, but some magnetic domains lose their alignment.
- With the application of magnetizing force in reverse direction, the curve moves to "c" point, where the flux has been decreased to zero. This point is called as coercivity point on the curve or loop. The reversed magnetizing force has reversed plenty of the domains, so that the remaining flux within the material is zero.
- To remove the residual magnetism from the material a force has to be apply, this required force is called as the coercive force or coercivity of the material.
- In the negative direction when magnetizing force is increased, the material will become again magnetically saturated or material under goes in saturation, but in the opposite or reverse direction i.e. towards "d" point.
- Decreasing magnetizing force (H) to zero brings the curve to "e" point. The available level of remaining magnetism is equal to that achieved in the other direction.
- Increasing magnetizing force (H) back in the positive direction will return or bring back the magnetic flux (B) to zero.
- We can notice that, the curve did not return back to the beginning or origin of the graph because some magnetizing force is needed to remove the remaining or residual magnetism.
- Now the curve in the graph will take a diverse or different path from “f” point back to the saturation point, here at this point it with complete the loop.
B-H curve measurement on Oscilloscope |
Advantages of Hysteresis loop or bh curve
- The magnetisation process of a ferromagnetic core.
- The part of the curve the ferromagnetic core is magnetised decides flux density because this depends on the circuits previous history which gives the core a form of “memory”.
- Ferromagnetic materials have memory because they stay magnetised after the external magnetic field has been taken out.
- Relays, solenoids and transformers can be easily magnetised and demagnetised because, they are made up of Soft ferromagnetic materials such as silicon steel or iron, which have very narrow magnetic hysteresis loops resulting in very small amounts of residual magnetism.
- Residual magnetism can be overcome by a coercive force; energy which is in use is dissipated as heat in the magnetic material. This heat is known as hysteresis loss, the material’s value of coercive force decides the amount of loss.
- A very small coercive force can be made that have a very narrow hysteresis loop by adding additive’s to the iron metal such as silicon. Magnetisation and demagnetisation of soft magnetic materials with narrow hysteresis loops are easy.
Hysteresis loop for Soft and Hard Material |
Applications of Hysteresis
What is hysteresis loss.
Please do not share any SPAM LINKS in the comment box.
Popular Posts

- Privacy Policy
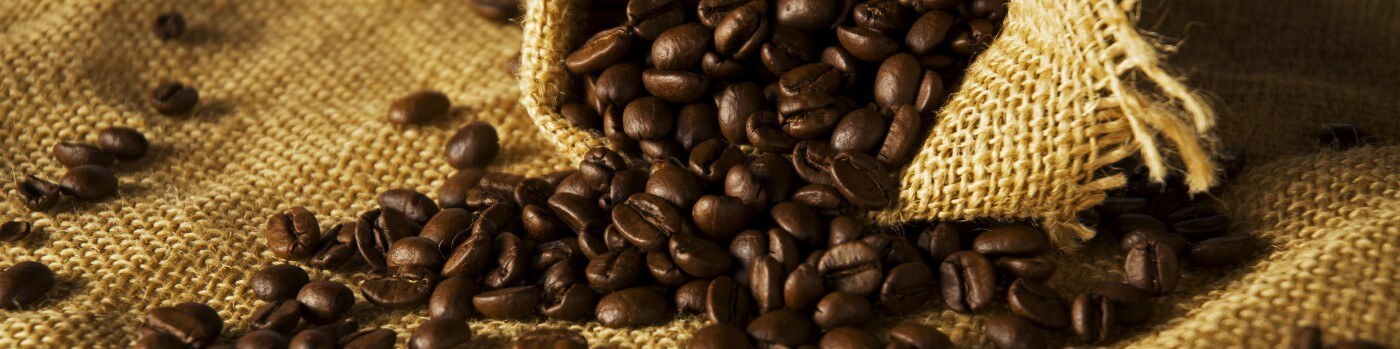
Electrical Engineering Professional
Electrical engineering lessons worth reading
The B-H Curve
The B-H curve (or a magnetization curve, excitation curve, magnetic hysteresis or saturation function) is the way to describe relation between a strength of a magnetic field H and a corresponding magnetic flux density B for a certain material. To have the understanding and ability to interpret the B-H curve, understanding both B and H is required. Therefore, the short introduction of both is following.
The strength of a magnetic field H
The strength a magnetic field H (or known as magneto-motive force) is a vector field quantity which means that it possesses a value and a direction for each point of space in which it exists. For the sake of the example, we are going to analyse the the coil with N turns through which current I is flowing. According to the Ampere’s law, the value of strength of a magnetic field is following:
where C is the closed integrating curve and dl is the infinitesimal element of that curve. The factor NI is more commonly known by a descriptive name of an ampere-turn. The same value of ampere-turn can be achieved by different combinations of numbers of turns and current since it is a product. From the Ampere’s law we can conclude that strength of a magnetic field is related to the ampere-turns because ampere-turns are the cause of the magnetic field. Finally, the unit of H is ampere-turn per meter, or A/m . The reason is that there is no unit for number of turns so the unit of ampere is alone. Where does the meter come from? Remember the line integral? Its unit is in meters. To completely understand why the meter exists in the H unit, we can consider two cases which include a coil with 10 turns and 1 ampere which results in 10 ampere-turns. In the first case, the coil is wound up tightly as possible. In the second case, the coil is very loosely wound so it has a lot of empty space between turns. The first coil will generate more concentrated magnetic field than the second one. To assess the spatial distribution of a magnetic field final unit ends up with meter in the denominator.
The density of a magnetic flux B
where A is the sample surface for which we are calculating the density. The unit of B is in teslas T. The value of B is easily measured with magnetic field meters. Knowing the magnetic flux is impossible with direct measurement. But knowing the definition for B , the flux is calculate-able. From last equation, the magnetic flux for a area A is following:
The relation between H and B
The experiments have shown that reluctivity is proportional to length of a path for magnetic flux l and reverse proportional to the affected area A . Therefore:
By replacing the values that we derived for the magnetic field strength and the magnetic flux density we end with next relation:
or more commonly:
To conclude why the relation between B and H is non-linear in nature is simple real world constrictions. When a magnetic material lies in the magnetic field the internal domains will orient in parallel to the existing magnetic field providing denser magnetic flux, according to the magnetic domain theory. After the (almost) linear part of hysteresis, the permeability of material decreases and the material gets saturated. That means that much more magneto-motive force is needed to increase flux in the material. Which, as a result, creates many headaches for power system operators when handling transformers and motors particularly which will be analysed in future posts.
Share the post!
4 thoughts on “ the b-h curve ”.
- Pingback: Transformer inrush current – Electrical Engineering Professional
- Pingback: Motor Protection Functions – Electrical Engineering Professional
- Pingback: Motor Protection Functions | Electrical Engineering Professional
- Pingback: Transformer inrush current | Electrical Engineering Professional
Leave a comment Cancel reply

- Already have a WordPress.com account? Log in now.
- Subscribe Subscribed
- Copy shortlink
- Report this content
- View post in Reader
- Manage subscriptions
- Collapse this bar
All Lab Experiments
Draw B-H curve using CRO
- B.Tech. Support Sem - II
- Physics Practical Files
Find the B-H curve lab manual in pdf format
The viva questions of this can be studied through this video link – https://www.youtube.com/watch?v=TeLHOEOQmWE&t=222s
Leave a Reply Cancel reply
Your email address will not be published. Required fields are marked *
Save my name, email, and website in this browser for the next time I comment.
Related Posts
To determine the hall coefficient of a semiconductor sample and determine its carrier concentration.
This Link provides you the handwritten practical file of the hall coefficient of a semiconductor experiment (with readings) ...
Material Science & Engineering – Notes
This post contains the notes for Material Science and engineering course, IGDTUW, Sem-III. Notes are in pdf format. ...
To Study the Output and Transfer Characteristics of a Junction Field Effect Transistor (jFET)
This Link provides the handwritten practical file of the above mentioned experiment (with readings) in the readable pdf format. ...
- Trending Categories

- Selected Reading
- UPSC IAS Exams Notes
- Developer's Best Practices
- Questions and Answers
- Effective Resume Writing
- HR Interview Questions
- Computer Glossary
Magnetic Hysteresis Including B-H Curve – Importance of Hysteresis Loop
B-h curve or magnetisation curve.
The B-H curve or magnetisation curve is the graph plotted between magnetic flux density (B) and magnetising force (H). The B-H curve indicates the manner in which the magnetic flux density varies with the change in magnetising force.
The following figure shows the general shape of B-H curve of a magnetic material. The nonlinearity of the curve shows that the relative permeability μ r of a magnetic material is not constant but varies depending upon the magnetic flux density.

Magnetic Hysteresis
The phenomenon of lagging of magnetic flux density (B) behind the magnetising force (H) in a magnetic material subjected to the cycle of magnetisation (i.e., it is magnetised first in one direction and then in the other) is called as magnetic hysteresis .
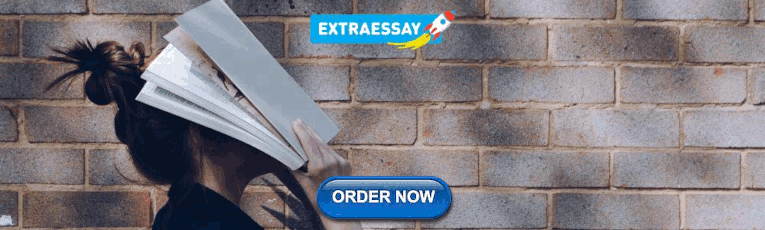
Hysteresis Loop
Consider a coil of N turns wound on an un-magnetised iron bar AB (see the figure). The magnetising force (H = NI/l) produced by the coil can be changed by varying the current through the coil. It can be seen that when the iron bar is subjected to one complete cycle of magnetisation, the resultant B-H curve traces a loop abcdefa called as hysteresis loop .

Explanation
When the current in the coil is zero, the H is zero and hence B in the iron bar is zero. When H is increased by increasing the coil current, the magnetic flux density also increases until the point of maximum magnetic flux density $\mathit{(+B_{max})}$ is reached. At this point, the material is saturated and beyond this point, the magnetic flux density will not increase regardless of any increase of magnetising force (H). For this, the B-H curve follows the path oa (see the hysteresis loop).
Now, if the H is gradually decreased by decreasing the coil current, it is found that the magnetic flux density does not decrease along the path oa but follows the path ab . At point b , the magnetising force is zero but magnetic flux density in the material has a finite value (equal to ob ) called residual flux density $\mathit{(+B_{r})}$. The ability of retaining residual magnetism by a magnetic material is called as retentivity of the material.
In order to demagnetise the iron bar i.e. to remove the residual magnetism (ob) , the magnetising force is reversed by reversing the coil current. When H is gradually increased in the reversed direction, the B-H curve follows the path bc so that when H = oc, the residual magnetism is zero. The values of H = oc required to completely remove the residual magnetism is known as coercive force (H c ) .
Now, if H is further increased in reverse direction, the material again saturates in the reverse direction (point d ). Reducing H to zero and then increasing it in the positive direction traces the curve defa. Therefore, when an iron bar is subjected to one complete cycle of magnetisation, the B-H curve traces a closed loop abcdefa called hysteresis loop .
Importance of Hysteresis Loop
The shape and size of the hysteresis loop depends upon the nature of the material. The choice of a magnetic material for a particular application depends upon the shape and size of the hysteresis loop.
Consider the following cases of hysteresis loop to understand it importance −
The smaller the area of the hysteresis loop of a magnetic material, the less is the hysteresis loss (e.g. silicon steel). Therefore, the silicon steel is widely used for making cores of transformer and rotating electric machines which are subjected to rapid reversals of magnetisation.

The larger the area of hysteresis loop of a magnetic material, the greater is the hysteresis loss (e.g. hard steel). Therefore, the hard steel is used for making permanent magnets because this material has high retentivity and coercivity.

The hysteresis loop for wrought iron has fairly good residual magnetism and coercivity. Therefore, it is used for making cores of electromagnets.

- Related Articles
- Hysteresis Loss and Eddy Current Loss
- Hysteresis Motor – Construction, Operation, Characteristics and Applications
- How can I plot hysteresis threshold in Matplotlib?
- What is hysteresis thresholding? How can it be achieved using scikit-learn in Python?
- Draw rough diagrams to illustrate the following:(a) Open curve (b) Closed curve
- Yield Curve and Inverted Yield Curve
- Features Of Indifference Curve
- The speed of a moving object is determined to be $0.06\ m/s$. This speed is equal to: (a) $2.16\ km/h$ (b) $1.08\ km/h$(c) $0.216\ km/h$(b) $0.0216\ km/h$
- Differences Between Beveridge Curve and Job Creation Curve
- Market Demand Curve is the Average Revenue Curve
- Supply Curve
- What is the definition of Curve?
- Importance of History
- Importance of Air
- Importance of Polysaccharides
Kickstart Your Career
Get certified by completing the course

- Electricity & Magnetism
- Physics Lab Instruments
- B-H Curve / Magnetic Hysteresis Curve Experiment

- Spectroscopy
- Interferometry
- Polarization
- Fiber Optics
- Lasers & Opto-Electronics
- Diffraction
- Millikan's Oil Drop Apparatus
- Apparatus for the study of Photo Electric Effect
- Franck - Hertz Experiment
- Apparatus for the Study of Biot - Savart's Law
- Hall Effect Apparatus
- Hall Effect Sample Holder for Electromagnet
- Magnetic Susceptibility - Quinke’s Method
- Magnetic Susceptibility - Gouy's method
- Holographic Lab
- Thin film Characterization
- Astro Physics
- Sources & Detectors

Get Quote
Can't read the image? click here to refresh.
Holmarc’s B-H curve experiment model no: HO-ED-EM-09 is used for the analysis of the magnetic hysteresis loop / B-H curve for soft iron & laminated iron cores used in two coils bound electromagnet with DC current supply. Magnetic field intensity H & flux density B is measured & hysteresis loop recorded using the dedicate software. Remenance & coercive field strength of soft & laminated iron cores evaluated with the help of software.
Magnetic hysteresis loop recorded for soft iron core and laminated core electromagnet
Find the coercive force, remenance, Residual magnetism, BH curve saturation from graph
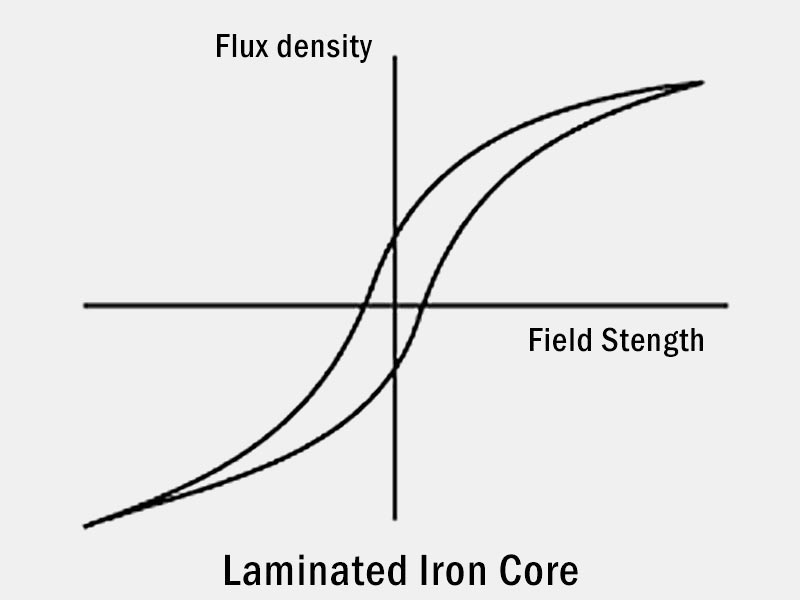
When we decrease the magnetic field flux density retain in the core shows residual magnetism Br. This time Magnetic field H is zero. Holmarc B-H software as per theory increase the magnetic field H in the reverse direction by increasing the current (i) for reducing flux density to zero & find Coercive force / field Hc. Further increment of H saturates the B in reverse direction. Decrease the H in to zero again, while the residual flux present in the reverse direction. Software increases the H value to make flux density zero & continues increment of H makes the flux density B saturates again. So this track / loop is known as magnetic hysteresis or B-H curve plot.
Either using soft/hard iron core materials in the electromagnet the size of hysteresis loop becomes different. In application by notice the hysteresis loop one can identify the heat loss/energy loss in transformers. Larger the hysteresis size greater the loss. Low hysteresis core materials are used in making electromagnets, solenoids, relays etc. Hysteresis in hard core material is useful for making hard disk drives, magnetic floppy disc etc.

Scope of Supply
Quantity : 1 no.
Dust Protective cover Instruction Manual
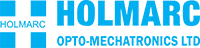
Established in 1993, Holmarc Opto-Mechatronics Ltd manufactures variety of scientific and engineering instruments for research, industry and education.
ISO 9001:2015 Made in INDIA
Privacy Policy
Ours is an organisation dedicated for the design, development and manufacture of optics, opto-mechanics, educational products and positioning devices.
We have in-house infrastructure capabilities for all the processes involved in the manufacture of our products. This helps us to have total control of quality in our products and services.
Our core competency is in prototype development and low volume production of opto-mechanical devices and systems where skilled labour is the major input. Our location in INDIA enables us to be the most cost effective in this field of activity compared to any other company in the world.
The products described in this website constitute only the standard items we manufacture for research and industrial market. One of our major activity is in OEM market where we develop and supply low volume opto-mechanical devices & positioning as per custom design. We also undertake contract manufacturing of opto-mechanical products in standard and custom specifications.
We try improving the quality of our products for every consignment we dispatch. Our customers can expect to receive better products each time a purchase order is placed with us.
Our strength is our satisfied customers worldwide. We request your continued support in our endeavor to serve you better.
We value the feedback on our products and services. Please mail us to [email protected]
We thank you for taking time to visit our website and await for an opportunity to be at your service in near future.
With best wishes and regards,
Staff & Management, Holmarc Opto-Mechatronics Ltd.
B.7., H.M.T. Industrial Estate, H.M.T. P.O Kalamassery, Kochi - 683 503, Kerala, India. Ph. No: +91 81369 44434
Terms & Conditions
Purchase Orders: Written, faxed and e-mail purchase orders are accepted. All orders require immediate written confirmation.
Product warranty: Our warranty for all our mechanical components and systems are ONE year from the date of shipment. Repair or replacement will be made free of charge for products with defects in material and workmanship during this period. For our optical components, our warranty is 30 days from the date of shipment. Defective items will be replaced free of cost. The customer must notify any defect in optical components within 48 hours of receipt. For all warranty replacement, a written statement with an authorized signature indicating the reason for rejection attaching test reports are requested to accompany the returned parts. All defective items must be returned in their original shipping container within 10 days of the date of shipment.
Quotations: All quotations are valid for 90 days from the date of issue.
Payment: 100% Advance payment prior to dispatch. Customers paying by international wire transfer must prepay all bank charges including all charges imposed by correspondent or intermediary banks.
Shipping & Delivery: The shipping costs, Custom Duty and any (if) landing taxes etc will be extra. The date of delivery is only an estimate and we will have no liability for late or partial deliveries. we may refuse any order for any reason. Catalog items are normally shipped immediately from inventory. In the event that the items is out of stock, an estimated delivery date is given at the time of order. Most items can be shipped within 45 days.
Installation: Onsite installation of our equipment's can be provided on buyer's account.
Specifications: HOLMARC reserves the right to modify, change or otherwise improve all items in the catalog specifications without prior notice.
Taxes: We collect general sales tax on shipments, unless the purchaser supplies us a signed official sales tax exemption certificate with the order. Purchase made outside our region are subject to state or local sales/ use tax, if any and other taxes payable by reason of this transaction.
Repairs: If your unit is out of warranty but needs repair, please contact us with details regarding the damage.
Information
- Author Services
Initiatives
You are accessing a machine-readable page. In order to be human-readable, please install an RSS reader.
All articles published by MDPI are made immediately available worldwide under an open access license. No special permission is required to reuse all or part of the article published by MDPI, including figures and tables. For articles published under an open access Creative Common CC BY license, any part of the article may be reused without permission provided that the original article is clearly cited. For more information, please refer to https://www.mdpi.com/openaccess .
Feature papers represent the most advanced research with significant potential for high impact in the field. A Feature Paper should be a substantial original Article that involves several techniques or approaches, provides an outlook for future research directions and describes possible research applications.
Feature papers are submitted upon individual invitation or recommendation by the scientific editors and must receive positive feedback from the reviewers.
Editor’s Choice articles are based on recommendations by the scientific editors of MDPI journals from around the world. Editors select a small number of articles recently published in the journal that they believe will be particularly interesting to readers, or important in the respective research area. The aim is to provide a snapshot of some of the most exciting work published in the various research areas of the journal.
Original Submission Date Received: .
- Active Journals
- Find a Journal
- Proceedings Series
- For Authors
- For Reviewers
- For Editors
- For Librarians
- For Publishers
- For Societies
- For Conference Organizers
- Open Access Policy
- Institutional Open Access Program
- Special Issues Guidelines
- Editorial Process
- Research and Publication Ethics
- Article Processing Charges
- Testimonials
- Preprints.org
- SciProfiles
- Encyclopedia
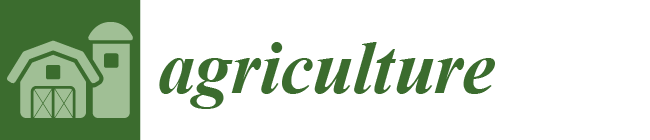
Article Menu
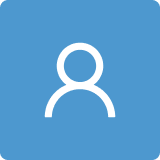
- Subscribe SciFeed
- Recommended Articles
- Google Scholar
- on Google Scholar
- Table of Contents
Find support for a specific problem in the support section of our website.
Please let us know what you think of our products and services.
Visit our dedicated information section to learn more about MDPI.
JSmol Viewer
Non-destructive detection method of apple watercore: optimization using optical property parameter inversion and mobilenetv3.
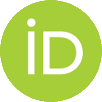
1. Introduction
2. materials and methods, 2.1. data acquisition, 2.2. simulation data collection, 2.2.1. three-layer model of apples.
- Determine whether a photon crossed the boundary. If the boundary was not crossed, the photon was absorbed and scattered, and the weight was updated. If the boundary was crossed, it was determined whether the photon escaped from the apple tissue surface;
- Determine whether a photon overflows. If it does not overflow, it is necessary to determine whether it is refracted or reflected and update the weights accordingly. If it overflows;
- Whether this is the last photon is determined. If this is the last photon, the operation is terminated.
2.2.2. Intersection Point Calculation
: Junction calculation |
Input: photon Output: Photon out of bounds position 1: current_pos = photon.current.position 2: previous_pos = photon.current-1.position 3: current_layer = current_pos.current.layer 4: previous_layer = previous_pos.current.layer 5: abs (current_layer − previous_layer) == 1: 6: True: 7: midpoint = (current_pos + previous_pos)/2 8: midpoint_layer = midpoint.current.layer 9: current_layer == midpoint_layer: 10: current_pos = midpoint 11: 12: previous_pos = midpoint 13: midpoint_layer == current_layer or midpoint_layer == previous_layer: 14: 15: 16: midpoint 17: |
2.2.3. Photon Cross-Layer Constraint Algorithm
: Photon transboundary confinement |
Input: photon Output: Ensure the photon crosses the layer boundary correctly 1: current_pos = photon.current.position 2: previous_pos = photon.current-1.position 3: current_layer = current_pos.current.layer 4: previous_layer = previous_pos.current.layer 5: abs(current_layer − previous_layer) == 1 6: midpoint = (current_pos + previous_pos)/2 7: midpoint = Junction calculation(photon) 8: current_layer = midpoint.current.layer 9: current_pos = midpoint 10: |
2.2.4. Absorption and Scattering of Photons
2.3. data augmentation.
- For the training set, the images are randomly cropped and the cropped images are resized to 224 × 224 pixels. The diversity of the images is enhanced by flipping the images randomly horizontally with 50% probability and mirroring the flipped images with 50% probability.
- For the test set, the longer side of the image was resized to 256 pixels, keeping the aspect ratio constant. The center region was cropped from the adjusted image with a cropping size of 224 × 224 pixels. It ensures that the data processing of the validation set is consistent with the training set and keeps the image features of the validation set unchanged, so as to accurately evaluate the performance of the model.
- The mean and standard deviation values obtained from the ImageNet dataset are used to normalise the pixel values of an image on both the test and training sets. This allows the data to have a distribution that is more suitable for training before feeding into the neural network.
2.4. Experimental Method
2.4.1. mobilenetv3 model and dilated convolution, 2.4.2. transfer learning, 2.4.3. comparison algorithm.
- SVM [ 50 ] is a classical machine learning method that excels at handling small samples and high-dimensional data. It is used to compare the improvement of deep learning models in feature extraction and classification tasks.
- ResNet50 [ 51 ] is a deep residual network that addresses the vanishing gradient problem in deep neural networks by introducing residual connections. It serves as a good reference for evaluating the performance of new models in complex tasks.
- VGG16 [ 52 ] increases the depth of the network by stacking small convolutional kernels to enhance feature extraction capabilities. It performs well in large-scale image classification tasks and is a classic benchmark in comparative experiments.
- ShuffleNetV2 is a lightweight neural network whose efficiency and low computational requirements make it ideal for comparison with MobileNetV3-small, especially in resource-constrained environments.
- InceptionNetV3 [ 53 ] utilises the Inception module, whose complex architecture and multi-scale feature extraction capabilities provide a contrast with our model in terms of feature diversity and complexity.
- MobileNetV3_large is another version of MobileNetV3, and comparing its performance with our model helps us understand the trade-off between model complexity and performance.
2.4.4. Evaluation Index
2.5. experimental environment and parameter setting, 3. results and discussion, 3.1. data augmentation effect and comparative analysis, 3.2. comparison of the model pre-training algorithms, 3.3. comparison experiments of transfer learning methods, 3.4. ablation experiment, 3.5. confusion matrix, 4. conclusions, author contributions, institutional review board statement, data availability statement, acknowledgments, conflicts of interest, abbreviations.
- Hong, J.; Zhang, T.; Shen, X.; Zhai, Y.; Bai, Y.; Hong, J. Water, energy, and carbon integrated footprint analysis from the environmental-economic perspective for apple production in China. J. Clean. Prod. 2022 , 368 , 133184. [ Google Scholar ] [ CrossRef ]
- Moriya, S.; Kunihisa, M.; Okada, K.; Iwanami, H.; Iwata, H.; Minamikawa, M.; Katayose, Y.; Matsumoto, T.; Mori, S.; Sasaki, H. Identification of QTLs for flesh mealiness in apple ( Malus× domestica Borkh.). Hortic. J. 2017 , 86 , 159–170. [ Google Scholar ] [ CrossRef ]
- Liu, Z.; Du, M.; Liu, H.; Zhang, K.; Xu, X.; Liu, K.; Tu, J.; Liu, Q. Chitosan films incorporating litchi peel extract and titanium dioxide nanoparticles and their application as coatings on watercored apples. Prog. Org. Coat. 2021 , 151 , 106103. [ Google Scholar ] [ CrossRef ]
- Li, W.; Liu, Z.; Wang, H.; Zheng, Y.; Zhou, Q.; Duan, L.; Tang, Y.; Jiang, Y.; Li, X.; Jiang, Y. Harvest maturity stage affects watercore dissipation and postharvest quality deterioration of watercore’Fuji’apples. Postharvest Biol. Technol. 2024 , 210 , 112736. [ Google Scholar ] [ CrossRef ]
- Itai, A. Watercore in fruits. In Abiotic Stress Biology in Horticultural Plants ; Springer: Berlin/Heidelberg, Germany, 2015; pp. 127–145. [ Google Scholar ]
- Zupan, A.; Mikulic-Petkovsek, M.; Stampar, F.; Veberic, R. Sugar and phenol content in apple with or without watercore. J. Sci. Food Agric. 2016 , 96 , 2845–2850. [ Google Scholar ] [ CrossRef ] [ PubMed ]
- Arnold, M.; Gramza-Michałowska, A. Enzymatic browning in apple products and its inhibition treatments: A comprehensive review. Compr. Rev. Food Sci. Food Saf. 2022 , 21 , 5038–5076. [ Google Scholar ] [ CrossRef ] [ PubMed ]
- Herremans, E.; Melado-Herreros, A.; Defraeye, T.; Verlinden, B.; Hertog, M.; Verboven, P.; Val, J.; Fernández-Valle, M.E.; Bongaers, E.; Estrade, P. Comparison of X-ray CT and MRI of watercore disorder of different apple cultivars. Postharvest Biol. Technol. 2014 , 87 , 42–50. [ Google Scholar ] [ CrossRef ]
- Rittiron, R.; Narongwongwattana, S.; Boonprakob, U.; Seehalak, W. Rapid and nondestructive detection of watercore and sugar content in Asian pear by near infrared spectroscopy for commercial trade. J. Innov. Opt. Health Sci. 2014 , 7 , 1350073. [ Google Scholar ] [ CrossRef ]
- Prananto, J.A.; Minasny, B.; Weaver, T. Near infrared (NIR) spectroscopy as a rapid and cost-effective method for nutrient analysis of plant leaf tissues. Adv. Agron. 2020 , 164 , 1–49. [ Google Scholar ]
- Fatihoglu, E.; Aydin, S.; Gokharman, F.D.; Ece, B.; Kosar, P.N. X-ray use in chest imaging in emergency department on the basis of cost and effectiveness. Acad. Radiol. 2016 , 23 , 1239–1245. [ Google Scholar ] [ CrossRef ]
- Sun, X.-L.; Zhou, T.-T.; Sun, Z.-Z.; Li, Z.-M.; Hu, D. Research progress into optical property-based nondestructive fruit and vegetable quality assessment. Food Res. Dev. 2022 , 43 , 208–209. [ Google Scholar ]
- Wang, S.; Huang, X.; Lyu, R.; Pan, S. Research progress of nondestructive detection methods in fruit quality. Food Ferment. Ind. 2018 , 44 , 319–324. [ Google Scholar ]
- Pan, L.; Wei, K.; Cao, N.; Sun, K.; Liu, Q.; Tu, K.; Zhu, Q. Measurement of optical parameters of fruits and vegetables and its application in quality detection. J. Nanjing Agric. Univ. 2018 , 41 , 26–37. [ Google Scholar ]
- Mondal, A.; Mandal, A. Stratified random sampling for dependent inputs in Monte Carlo simulations from computer experiments. J. Stat. Plan. Inference 2020 , 205 , 269–282. [ Google Scholar ] [ CrossRef ]
- Guan, T.; Zhao, H.; Wang, Z.; Yu, D. Optical properties reconstruction of layered tissue and experimental demonstration. In Proceedings of the Complex Dynamics and Fluctuations in Biomedical Photonics IV, San Jose, CA, USA, 20–25 January 2007; SPIE: Paris, France, 2007; pp. 227–234. [ Google Scholar ]
- Zhang, M.; Li, C.; Yang, F. Optical properties of blueberry flesh and skin and Monte Carlo multi-layered simulation of light interaction with fruit tissues. Postharvest Biol. Technol. 2019 , 150 , 28–41. [ Google Scholar ] [ CrossRef ]
- Mahesh, B. Machine learning algorithms-a review. Int. J. Sci. Res. IJSR 2020 , 9 , 381–386. [ Google Scholar ] [ CrossRef ]
- Shruthi, U.; Nagaveni, V.; Raghavendra, B. A review on machine learning classification techniques for plant disease detection. In Proceedings of the 2019 5th International Conference on Advanced Computing & Communication Systems (ICACCS), Coimbatore, India, 15–16 March 2019; IEEE: Piscataway, NJ, USA, 2019; pp. 281–284. [ Google Scholar ]
- Guan, Z.; Tang, J.; Yang, B.; Zhou, Y.; Fan, D.; Yao, Q. Study on recognition method of rice disease based on image. Chin. J. Rice Sci. 2010 , 24 , 497. [ Google Scholar ]
- Kattenborn, T.; Leitloff, J.; Schiefer, F.; Hinz, S. Review on Convolutional Neural Networks (CNN) in vegetation remote sensing. ISPRS J. Photogramm. Remote Sens. 2021 , 173 , 24–49. [ Google Scholar ] [ CrossRef ]
- Anagnostis, A.; Asiminari, G.; Papageorgiou, E.; Bochtis, D. A convolutional neural networks based method for anthracnose infected walnut tree leaves identification. Appl. Sci. 2020 , 10 , 469. [ Google Scholar ] [ CrossRef ]
- Rachmad, A.; Fuad, M.; Rochman, E.M.S. Convolutional Neural Network-Based Classification Model of Corn Leaf Disease. Math. Model. Eng. Probl. 2023 , 10 , 530–536. [ Google Scholar ] [ CrossRef ]
- Weiss, K.; Khoshgoftaar, T.M.; Wang, D. A survey of transfer learning. J. Big Data 2016 , 3 , 1345–1459. [ Google Scholar ] [ CrossRef ]
- Deng, L.; Li, J.; Han, Z. Online defect detection and automatic grading of carrots using computer vision combined with deep learning methods. LWT 2021 , 149 , 111832. [ Google Scholar ] [ CrossRef ]
- Mansheng, L.; Chunjuan, O.; Huan, L.; Qing, F. Image recognition of Camellia oleifera diseases based on convolutional neural network & transfer learning. Trans. Chin. Soc. Agric. Eng. Trans. CSAE 2018 , 34 , 194–201. [ Google Scholar ]
- Elharrouss, O.; Akbari, Y.; Almaadeed, N.; Al-Maadeed, S. Backbones-review: Feature extraction networks for deep learning and deep reinforcement learning approaches. arXiv 2022 , arXiv:2206.08016. [ Google Scholar ] [ CrossRef ]
- Zhang, X.; Zhou, X.; Lin, M.; Sun, J. Shufflenet: An extremely efficient convolutional neural network for mobile devices. In Proceedings of the IEEE Conference on Computer Vision and Pattern Recognition, Salt Lake City, UT, USA, 18–23 June 2018; pp. 6848–6856. [ Google Scholar ]
- Türkmen, S.; Heikkilä, J. An efficient solution for semantic segmentation: ShuffleNet V2 with atrous separable convolutions. In Proceedings of the Scandinavian Conference on Image Analysis, Norrköping, Sweden, 11–13 June 2019; Springer: Cham, Switzerland, 2019; pp. 41–53. [ Google Scholar ]
- Howard, A.G.; Zhu, M.; Chen, B.; Kalenichenko, D.; Wang, W.; Weyand, T.; Andreetto, M.; Adam, H. Mobilenets: Efficient convolutional neural networks for mobile vision applications. arXiv 2017 , arXiv:1704.04861. [ Google Scholar ]
- Sandler, M.; Howard, A.; Zhu, M.; Zhmoginov, A.; Chen, L.-C. Mobilenetv2: Inverted residuals and linear bottlenecks. In Proceedings of the IEEE Conference on Computer Vision and Pattern Recognition, Salt Lake City, UT, USA, 18–23 June 2018; pp. 4510–4520. [ Google Scholar ]
- Howard, A.; Sandler, M.; Chu, G.; Chen, L.-C.; Chen, B.; Tan, M.; Wang, W.; Zhu, Y.; Pang, R.; Vasudevan, V. Searching for mobilenetv3. In Proceedings of the IEEE/CVF International Conference on Computer Vision, Seoul, Republic of Korea, 27 October–2 November 2019; pp. 1314–1324. [ Google Scholar ]
- Yang, L.; Quan, F.; Shuzhi, W. Plant disease identification method and mobile application based on lightweight CNN. Trans. Chin. Soc. Agric. Eng. 2019 , 35 , 194–204. [ Google Scholar ]
- Si, H.; Wang, Y.; Zhao, W.; Wang, M.; Song, J.; Wan, L.; Song, Z.; Li, Y.; Fernando, B.; Sun, C. Apple Surface Defect Detection Method Based on Weight Comparison Transfer Learning with MobileNetV3. Agriculture 2023 , 13 , 824. [ Google Scholar ] [ CrossRef ]
- Peng, Z.; Cai, C. An effective segmentation algorithm of apple watercore disease region using fully convolutional neural networks. In Proceedings of the 2017 Asia-Pacific Signal and Information Processing Association Annual Summit and Conference (APSIPA ASC), Kuala Lumpur, Malaysia, 12–15 December 2017; IEEE: Piscataway, NJ, USA, 2017; pp. 1292–1299. [ Google Scholar ]
- Pan, L.-Q.; Fang, L.; Hou, B.-J.; Zhang, B.; Peng, J.; Tu, K. System and principle of optical properties measurement and advances on quality detection of fruits and vegetables. J. Nanjing Agric. Univ. 2021 , 44 , 401–411. [ Google Scholar ]
- Xu, Z.; Wang, Z.; Huang, L.; Liu, Z.; Hou, R.; Wang, C. Double-integrating-sphere system for measuring optical properties of farm products and its application. Trans. CSAE 2006 , 22 , 244–249. [ Google Scholar ]
- Xu, H.; Sun, Y.; Cao, X.; Ji, C.; Chen, L.; Wang, H. Apple quality detection based on photon transmission simulation and convolutional neural network. Trans. Chin. Soc. Agric. Mach. 2021 , 52 , 338–345. [ Google Scholar ]
- Li, J.; Xue, J.; Li, J.; Zhao, L. Study of the Changes in Optical Parameters of Diseased Apple Pulps Based on the Integrating Sphere Technique. Spectroscopy 2020 , 35 , 32–38. [ Google Scholar ]
- Solanki, C.; Thapliyal, P.; Tomar, K. Role of bisection method. Int. J. Comput. Appl. Technol. Res. 2014 , 3 , 535. [ Google Scholar ] [ CrossRef ]
- Toublanc, D. Henyey–Greenstein and Mie phase functions in Monte Carlo radiative transfer computations. Appl. Opt. 1996 , 35 , 3270–3274. [ Google Scholar ] [ CrossRef ] [ PubMed ]
- Maharana, K.; Mondal, S.; Nemade, B. A review: Data pre-processing and data augmentation techniques. Glob. Transit. Proc. 2022 , 3 , 91–99. [ Google Scholar ] [ CrossRef ]
- Hu, J.; Shen, L.; Sun, G. Squeeze-and-excitation networks. In Proceedings of the IEEE Conference on Computer Vision and Pattern Recognition, Salt Lake City, UT, USA, 18–23 June 2018; pp. 7132–7141. [ Google Scholar ]
- Ma, L.; Liu, X.; Li, H.; Duan, K.; Niu, B. Neural network lightweight method with dilated convolution. Comput. Eng. Appl. 2022 , 58 , 85–93. [ Google Scholar ] [ CrossRef ]
- Niu, S.; Liu, Y.; Wang, J.; Song, H. A decade survey of transfer learning (2010–2020). IEEE Trans. Artif. Intell. 2020 , 1 , 151–166. [ Google Scholar ] [ CrossRef ]
- Mormont, R.; Geurts, P.; Marée, R. Comparison of deep transfer learning strategies for digital pathology. In Proceedings of the IEEE Conference on Computer Vision and Pattern Recognition Workshops, Salt Lake City, UT, USA, 18–23 June 2018; pp. 2262–2271. [ Google Scholar ]
- Soekhoe, D.; Van Der Putten, P.; Plaat, A. On the impact of data set size in transfer learning using deep neural networks. In Proceedings of the Advances in Intelligent Data Analysis XV: 15th International Symposium, IDA 2016, Stockholm, Sweden, 13–15 October 2016; Proceedings 15. Springer: Berlin/Heidelberg, Germany, 2016; pp. 50–60. [ Google Scholar ]
- Kruithof, M.C.; Bouma, H.; Fischer, N.M.; Schutte, K. Object recognition using deep convolutional neural networks with complete transfer and partial frozen layers. In Proceedings of the Optics and Photonics for Counterterrorism, Crime Fighting, and Defence XII, Edinburgh, UK, 26–27 September 2016; SPIE: Cergy, France, 2016; pp. 159–165. [ Google Scholar ]
- Noor, A.; Zhao, Y.; Koubâa, A.; Wu, L.; Khan, R.; Abdalla, F.Y. Automated sheep facial expression classification using deep transfer learning. Comput. Electron. Agric. 2020 , 175 , 105528. [ Google Scholar ] [ CrossRef ]
- Li, Z.; Niu, B.; Peng, F.; Li, G.; Yang, Z.; Wu, J. Classification of peanut images based on multi-features and SVM. IFAC-Pap. 2018 , 51 , 726–731. [ Google Scholar ] [ CrossRef ]
- Mukti, I.Z.; Biswas, D. Transfer learning based plant diseases detection using ResNet50. In Proceedings of the 2019 4th International Conference on Electrical Information and Communication Technology (EICT), Khulna, Bangladesh, 20–22 December 2019; IEEE: Piscataway, NJ, USA, 2019; pp. 1–6. [ Google Scholar ]
- Qassim, H.; Feinzimer, D.; Verma, A. Residual squeeze vgg16. arXiv 2017 , arXiv:1705.03004. [ Google Scholar ]
- Xia, X.; Xu, C.; Nan, B. Inception-v3 for flower classification. In Proceedings of the 2017 2nd International Conference on Image Vision and Computing (ICIVC), Chengdu, China, 2–4 June 2017; IEEE: Piscataway, NJ, USA, 2017; pp. 783–787. [ Google Scholar ]
- Shorten, C.; Khoshgoftaar, T.M. A survey on image data augmentation for deep learning. J. Big Data 2019 , 6 , 60. [ Google Scholar ] [ CrossRef ]
- Cang, H.; Yan, T.; Duan, L.; Yan, J.; Zhang, Y.; Tan, F.; Lv, X.; Gao, P. Jujube quality grading using a generative adversarial network with an imbalanced data set. Biosyst. Eng. 2023 , 236 , 224–237. [ Google Scholar ] [ CrossRef ]
- Li, Y.; Wang, H.; Zhang, Y.; Wang, J.; Xu, H. Inversion of the optical properties of apples based on the convolutional neural network and transfer learning methods. Appl. Eng. Agric. 2022 , 38 , 931–939. [ Google Scholar ] [ CrossRef ]
- Korzhebin, T.A.; Egorov, A.D. Comparison of combinations of data augmentation methods and transfer learning strategies in image classification used in convolution deep neural networks. In Proceedings of the 2021 IEEE Conference of Russian Young Researchers in Electrical and Electronic Engineering (ElConRus), St. Petersburg, Moscow, Russia, 26–29 January 2021; IEEE: Piscataway, NJ, USA, 2021; pp. 479–482. [ Google Scholar ]
- Guo, L.; Lei, Y.; Xing, S.; Yan, T.; Li, N. Deep convolutional transfer learning network: A new method for intelligent fault diagnosis of machines with unlabeled data. IEEE Trans. Ind. Electron. 2018 , 66 , 7316–7325. [ Google Scholar ] [ CrossRef ]
- Haoyun, W.; Yiba, L.; Yuzhuo, Z.; Xiaoli, Z.; Huanliang, X. Research on hyperspectral light and probe source location on apple for quality detection based on photon transmission simulation. Trans. Chin. Soc. Agric. Eng. 2019 , 35 , 281–289. [ Google Scholar ]
- Ribani, R.; Marengoni, M. A survey of transfer learning for convolutional neural networks. In Proceedings of the 2019 32nd SIBGRAPI Conference on Graphics, Patterns and Images Tutorials (SIBGRAPI-T), Rio de Janeiro, Brazil, 28–31 October 2019; IEEE: Piscataway, NJ, USA, 2019; pp. 47–57. [ Google Scholar ]
- Yang, M.; He, Y.; Zhang, H.; Li, D.; Bouras, A.; Yu, X.; Tang, Y. The research on detection of crop diseases ranking based on transfer learning. In Proceedings of the 2019 6th International Conference on Information Science and Control Engineering (ICISCE), Shanghai, China, 20–22 December 2019; IEEE: Piscataway, NJ, USA, 2019; pp. 620–624. [ Google Scholar ]
- Xu, C.; Wang, X.; Zhang, S. Dilated convolution capsule network for apple leaf disease identification. Front. Plant Sci. 2022 , 13 , 1002312. [ Google Scholar ] [ CrossRef ]
- Dou, S.; Wang, L.; Fan, D.; Miao, L.; Yan, J.; He, H. Classification of Citrus huanglongbing degree based on cbam-mobilenetv2 and transfer learning. Sensors 2023 , 23 , 5587. [ Google Scholar ] [ CrossRef ]
- Flach, P.A. ROC analysis. In Encyclopedia of Machine Learning and Data Mining ; Springer: Berlin/Heidelberg, Germany, 2016; pp. 1–8. [ Google Scholar ]
- Lobo, J.M.; Jiménez-Valverde, A.; Real, R. AUC: A misleading measure of the performance of predictive distribution models. Glob. Ecol. Biogeogr. 2008 , 17 , 145–151. [ Google Scholar ] [ CrossRef ]
Click here to enlarge figure
Classification | Classification Criteria |
---|---|
Two-class | Area = 0; Area! = 0 |
Three-class | Area = 0; Area ≤ 0.15; Area > 0.15 |
Four-class | Area = 0; Area ≤ 0.1; 0.1 < Area ≤ 0.2; Area > 0.2 |
Optical Parameter | Interval Range | Value (mm ) |
---|---|---|
[0.40, 1.60) | 1.00 | |
[1.60, 2.80) | 2.20 | |
[2.80, 4.00) | 3.40 | |
[4.00, 5.20) | 4.60 | |
[5.20, 6.40) | 5.80 | |
[0.03, 2.20) | 1.10 | |
[2.20, 4.40) | 3.30 | |
[4.40, 6.60) | 5.50 | |
[6.60, 8.70) | 7.70 | |
[0.01, 0.65) | 0.30 | |
[0.65, 1.30) | 0.90 | |
[1.30, 1.95) | 1.50 | |
[1.95, 2.70) | 2.10 | |
[1.69, 60.00) | 30.00 | |
[60.00, 75.00) | 67.50 | |
[75.00, 90.00) | 82.50 | |
[90.00, 110.00) | 100.00 | |
[110.00, 260.00) | 190.00 | |
[0.01, 15.00) | 7.50 | |
[15.00, 30.00) | 22.50 | |
[30.00, 45.00) | 37.50 | |
[45.00, 60.00) | 52.50 | |
[60.00, 75.00) | 67.50 | |
[0.01, 7.50) | 5.00 | |
[7.50, 15.00) | 12.50 | |
[15.00, 22.50) | 20.00 | |
[22.50, 30.00) | 27.50 |
Input | Operator | Exp Size | Output | SE | NL | Step Size |
---|---|---|---|---|---|---|
224 × 224 × 3 | conv2d,3 × 3 | - | 16 | - | HS | 2 |
112 × 112 × 16 | bneck,3 × 3 | 16 | 16 | √ | RE | 2 |
56 × 56 × 16 | bneck,3 × 3 | 72 | 24 | - | RE | 2 |
28 × 28 × 24 | bneck,3 × 3 | 88 | 24 | - | RE | 1 |
28 × 28 × 24 | bneck,5 × 5 | 96 | 40 | √ | HS | 2 |
14 × 14 × 40 | bneck,5 × 5 | 240 | 40 | √ | HS | 1 |
14 × 14 × 40 | bneck,5 × 5 | 240 | 40 | √ | HS | 1 |
14 × 14 × 40 | bneck,5 × 5 | 120 | 48 | √ | HS | 1 |
14 × 14 × 48 | bneck,5 × 5 | 144 | 48 | √ | HS | 1 |
14 × 14 × 48 | bneck,5 × 5 | 288 | 96 | √ | HS | 2 |
7 × 7 × 96 | bneck,5 × 5 | 576 | 96 | √ | HS | 1 |
7 × 7 × 96 | bneck,5 × 5 | 576 | 96 | √ | HS | 1 |
7 × 7 × 96 | conv2d,1 × 1 | - | 576 | √ | HS | 1 |
7 × 7 × 576 | pool,7 × 7 | - | - | - | - | 1 |
1 × 1 × 576 | conv2d,1 × 1, NBU | - | 1024 | - | HS | 1 |
1 × 1 × 1024 | conv2d,1 × 1, NBU | - | k | - | - | 1 |
Model | Classification | Loss | Accuracy (%) | Precision (%) | Recall (%) | F1-Score (%) | Model Size (M) |
---|---|---|---|---|---|---|---|
Our Method | Two-class | 0.0291 | 99.05 | 98.43 | 98.58 | 98.29 | 18.89 |
Three-class | 0.0327 | 96.77 | 96.30 | 96.45 | 96.12 | ||
Four-class | 0.1048 | 94.45 | 94.12 | 94.06 | 93.87 | ||
SVM | Two-class | 0.3941 | 80.16 | 79.76 | 79.21 | 79.45 | 114.6 |
Three-class | 0.4315 | 73.18 | 72.69 | 72.17 | 72.43 | ||
Four-class | 0.4819 | 71.99 | 71.74 | 71.53 | 71.68 | ||
ResNet50 | Two-class | 0.1032 | 96.14 | 95.53 | 95.74 | 96.03 | 97.7 |
Three-class | 0.1396 | 94.43 | 93.82 | 94.06 | 94.11 | ||
Four-class | 0.2311 | 92.43 | 92.16 | 92.63 | 91.95 | ||
VGG16 | Two-class | 0.1504 | 96.69 | 96.47 | 96.31 | 96.48 | 526.3 |
Three-class | 0.1685 | 94.81 | 94.59 | 94.43 | 94.61 | ||
Four-class | 0.2381 | 92.12 | 91.88 | 91.42 | 91.92 | ||
InceptionNetV3 | Two-class | 0.1283 | 94.28 | 94.10 | 93.85 | 93.71 | 91.16 |
Three-class | 0.1611 | 93.19 | 93.01 | 92.94 | 92.88 | ||
Four-class | 0.2524 | 91.98 | 91.70 | 91.59 | 91.29 | ||
ShuffleNetV2 | Two-class | 0.1471 | 93.83 | 83.31 | 93.22 | 93.48 | 28.8 |
Three-class | 0.1936 | 91.64 | 91.12 | 92.04 | 91.99 | ||
Four-class | 0.2736 | 87.76 | 88.14 | 87.31 | 87.14 | ||
MobileNetV3-large | Two-class | 0.0748 | 97.86 | 97.19 | 97.92 | 97.38 | 20.6 |
Three-class | 0.0912 | 95.45 | 95.17 | 95.96 | 95.16 | ||
Four-class | 0.1758 | 93.62 | 93.13 | 93.34 | 93.10 |
Number of Unfrozen Network Layers | Loss Value | Accuracy (%) | Precision (%) | Recall (%) | F1-Score (%) | Params (M) |
---|---|---|---|---|---|---|
1 | 0.0308 | 97.20 | 96.65 | 97.12 | 96.89 | 7.54 |
2 | 0.0304 | 97.60 | 97.24 | 97.33 | 96.92 | 7.54 |
3 | 0.0304 | 97.60 | 97.24 | 97.33 | 96.92 | 7.54 |
4 | 0.0304 | 97.60 | 97.24 | 97.33 | 96.92 | 7.54 |
5 | 0.0304 | 97.60 | 97.24 | 97.33 | 96.92 | 7.52 |
6 | 0.0322 | 96.70 | 96.18 | 96.27 | 96.23 | 7.50 |
7 | 0.0322 | 96.70 | 96.18 | 96.27 | 96.23 | 7.47 |
8 | 0.0349 | 95.90 | 95.47 | 95.25 | 95.28 | 7.49 |
9 | 0.0322 | 96.70 | 96.18 | 96.27 | 96.23 | 7.46 |
10 | 0.0349 | 95.90 | 95.47 | 95.25 | 95.28 | 7.43 |
Transfer Learning Methods | Classification | Loss Value | Accuracy (%) | Precision (%) | Recall (%) | F1-Score (%) | Params (M) |
---|---|---|---|---|---|---|---|
Freezing of all network layers | Two-class | 0.1768 | 92.25 | 91.53 | 91.74 | 91.48 | 4.24 |
Three-class | 0.2136 | 89.70 | 89.82 | 89.25 | 88.46 | 4.24 | |
Four-class | 0.2415 | 87.53 | 87.12 | 87.09 | 86.94 | 4.24 | |
Partial freezing of the network layers | Two-class | 0.0288 | 99.13 | 98.67 | 98.51 | 98.70 | 7.52 |
Three-class | 0.0304 | 97.60 | 97.19 | 97.23 | 97.41 | 7.52 | |
Four-class | 0.0982 | 95.32 | 94.91 | 94.95 | 95.13 | 7.52 | |
No freezing of network layers | Two-class | 0.0341 | 98.55 | 98.23 | 98.36 | 97.92 | 7.54 |
Three-class | 0.0376 | 96.20 | 95.97 | 96.68 | 96.45 | 7.54 | |
Four-class | 0.0419 | 93.97 | 93.60 | 93.34 | 93.65 | 7.54 |
Methods | Classification | Loss Value | Accuracy (%) | Precision (%) | Recall (%) | F1-Score (%) | Params (M) |
---|---|---|---|---|---|---|---|
MobileNetV3 | Two-class | 0.3251 | 81.23 | 81.68 | 81.80 | 81.42 | 2.54 |
Three-class | 0.4069 | 78.76 | 78.35 | 78.44 | 78.94 | 2.54 | |
Four-class | 0.4782 | 72.43 | 72.02 | 71.78 | 72.11 | 2.54 | |
MobileNetV3 + Dilated convolution | Two-class | 0.2471 | 90.58 | 90.26 | 89.97 | 90.12 | 24.10 |
Three-class | 0.2949 | 86.97 | 86.64 | 86.55 | 86.34 | 24.10 | |
Four-class | 0.3182 | 81.35 | 81.03 | 81.52 | 80.93 | 24.10 | |
MobileNetV3 + Dilatedconvolution + transfer learning | Two-class | 0.0288 | 99.13 | 98.67 | 98.51 | 98.70 | 7.52 |
Three-class | 0.0304 | 97.60 | 97.19 | 97.23 | 97.41 | 7.52 | |
Four-class | 0.0982 | 95.32 | 94.91 | 94.95 | 95.13 | 7.52 |
The statements, opinions and data contained in all publications are solely those of the individual author(s) and contributor(s) and not of MDPI and/or the editor(s). MDPI and/or the editor(s) disclaim responsibility for any injury to people or property resulting from any ideas, methods, instructions or products referred to in the content. |
Share and Cite
Chen, Z.; Wang, H.; Wang, J.; Xu, H.; Mei, N.; Zhang, S. Non-Destructive Detection Method of Apple Watercore: Optimization Using Optical Property Parameter Inversion and MobileNetV3. Agriculture 2024 , 14 , 1450. https://doi.org/10.3390/agriculture14091450
Chen Z, Wang H, Wang J, Xu H, Mei N, Zhang S. Non-Destructive Detection Method of Apple Watercore: Optimization Using Optical Property Parameter Inversion and MobileNetV3. Agriculture . 2024; 14(9):1450. https://doi.org/10.3390/agriculture14091450
Chen, Zihan, Haoyun Wang, Jufei Wang, Huanliang Xu, Ni Mei, and Sixu Zhang. 2024. "Non-Destructive Detection Method of Apple Watercore: Optimization Using Optical Property Parameter Inversion and MobileNetV3" Agriculture 14, no. 9: 1450. https://doi.org/10.3390/agriculture14091450
Article Metrics
Article access statistics, further information, mdpi initiatives, follow mdpi.
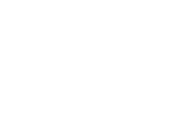
Subscribe to receive issue release notifications and newsletters from MDPI journals
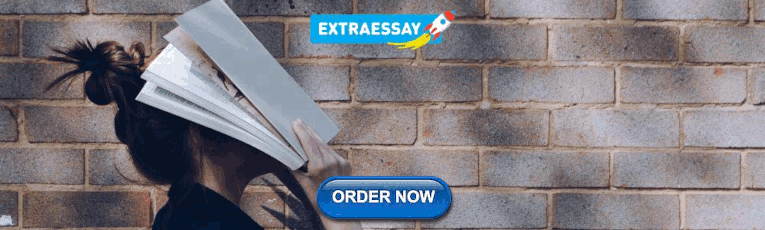
COMMENTS
The Magnetic Hysteresis loop above, shows the behaviour of a ferromagnetic core graphically as the relationship between B and H is non-linear. Starting with an unmagnetised core both B and H will be at zero, point 0 on the magnetisation curve.. If the magnetisation current, i is increased in a positive direction to some value the magnetic field strength H increases linearly with i and the flux ...
A curve, or loop, plotted on B-H coordinates showing how the magnetization of a ferromagnetic material varies when subjected to a periodically reversing magnetic field, is known as Hysteresis Loop or Magnetization Curve. The term 'hysteresis' means to lag behind. In electrical terminology it is used to describe the lag between a change in ...
Demonstration of B-H Curve experiment with data and calculations
About Press Copyright Contact us Creators Advertise Developers Terms Privacy Policy & Safety How YouTube works Test new features NFL Sunday Ticket Press Copyright ...
This video covers the most important viva questions of BH curve experiment.===== Thanks for WatchingPlease leave a LIKE to Support me...
The B-H curve is usually used to describe the magnetization properties of such materials by characterizing the permeability , which is defined as: where and represent the magnetic flux density in tesla (T) and the magnetic field intensity in ampère per meter (A/m), respectively. COMSOL Multiphysics has more than 200 built-in materials with B-H ...
• calculate the magnetic flux density B(t) from the flux-linkage using (4); • plot B(t) versus H(t) to obtain the BH loop (see Fig. 5), determine the peak values of B and H. • calculate the average power loss Ploss using (7), the loss per kg can be calculated by dividing this by the weight of the test core laminations.
A huge increase in B for the same H! The hysteresis comes into play when the material has been magnetized. The B within the material does not go back to what it was before, but is dependent on the history of its magnetization. c. The slope of the curve - Permeability. The slope of the B-H curve at some location on its curve is its incremental ...
Hysteresis curves, also called B-H curves, describe the Intrinsic and Normal magnetic properties of a material. The Hysteresis curve is commonly seen in supplier catalogs as a second quadrant curve showing Br, Hc, Hci and BH(max). The test is normally performed by the magnet manufacturer during the initial stage of processing.
The width of the hysteresis loop tells us a lot about the losses. The narrower the curve, the lower the losses are. Hard magnetic materials have a very wide hysteresis curve, which makes them practical in applications where they exert their magnetic field on soft magnetic materials. As seen in the figure below, hard magnetic materials have high ...
Hysteresis loop provides information about the magnetic properties of a material. It is important that the B-H hysteresis loop is as small as possible so loss will be less because shape of B-H curve decides the loss. Bigger the area then more is the loss and vice-versa. The shape of hysteresis loop depends upon the nature of the material used i ...
2 Jul 2021 by Electrical Workbook. In this topic, you study B-H Curve or Magnetization Curve - Definition, Diagram & Theory. Consider the test piece of the magnetic material made up in the form of a ring (C) with across-section 'a' square metres and a mean length of magnetic path ' l ' metres. Let the ring be wound uniformly with N ...
The B-H curve (or a magnetization curve, excitation curve, magnetic hysteresis or saturation function) is the way to describe relation between a strength of a magnetic field H and a corresponding magnetic flux density B for a certain material. To have the understanding and ability to interpret the B-H curve, understanding both B and H is required. . Therefore, the short introduction of both is fol
This link provides the lab manual used for B-H curve loop tracing using CRO. The required setup and the necessary calculations are in this file.
This experiment aims to study the B-H (magnetic flux density-magnetic field strength) curve for ferromagnetic materials to determine their coercivity and retentivity. An apparatus is used that includes a B-H curve tracer, specimen solenoid, thin metal wires, and CRO. The experiment involves applying a voltage to the solenoid to generate a magnetic field and plot the resulting B-H hysteresis ...
The B-H curve or magnetisation curve is the graph plotted between magnetic flux density (B) and magnetising force (H). The B-H curve indicates the manner in which the magnetic flux density varies with the change in magnetising force. The following figure shows the general shape of B-H curve of a magnetic material.
B.E/B.Tech/B.Sc students
Price : $ 1,917.00. Holmarc's B-H curve experiment model no: HO-ED-EM-09 is used for the analysis of the magnetic hysteresis loop / B-H curve for soft iron & laminated iron cores used in two coils bound electromagnet with DC current supply. Magnetic field intensity H & flux density B is measured & hysteresis loop recorded using the dedicate ...
magnetic field H. In this experiment magnetic field H is produced by passing ... Circuit Diagram for obtaining B-H curve on CRO screen Hence calibration constant for H axis is: H 4 II n 2 I rms max = 10 C = x 2H max L ... CALCULATIONS: From the trace of BH loop on tracing paper measure different lengths i.e. Ob, Oa', OC etc. and calculate the ...
The document describes an experiment to obtain the B-H loop and magnetization curve for a magnetic core and determine the core losses. Key steps include: 1. Connecting equipment like an auto transformer, oscilloscope, and integrator in a circuit to generate the B-H loop. 2. Measuring and plotting the magnetic flux density (B) and magnetic field strength (H) to obtain the hysteresis loop on an ...
The Monte Carlo method , also known as the statistical simulation method, is a probabilistic calculation method based on statistical theory. It uses random (or pseudo-random) numbers to address various computational problems. ... This was used as the measured data for the experiment. ... (Figure 8b). The curve stabilised after 600 rounds.
About Press Copyright Contact us Creators Advertise Developers Terms Privacy Policy & Safety How YouTube works Test new features NFL Sunday Ticket Press Copyright ...