13. Study design and choosing a statistical test
Sample size.

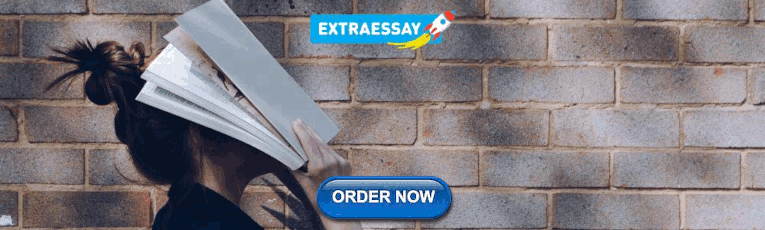
Choice of test
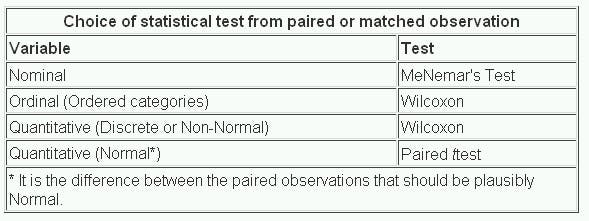
Modern Statistical Methods for Health Research
- © 2021
- Yichuan Zhao 0 ,
- (Din) Ding-Geng Chen 1
Department of Mathematics & Statistics, Georgia State University, Atlanta, USA
You can also search for this editor in PubMed Google Scholar
School of Mathematics, Statistics and Computer Science, University of KwaZulu-Natal, KwaZulu-Natal, South Africa
- Features the significant contributions of many leading statisticians and data scientists to contemporary health sciences
- Includes a comprehensive review of statistical methods and new approaches in health research to guide the reader in learning efficiently
- Covers new developments in EHR data analysis, clinical trials, FDR, big network analytics, applications in GWAS, graphical modeling in genomic studies, survival analysis, and functional data analysis
- Offers innovative statistical procedures, computer program, new data sets, and novel applications in modern health research
Part of the book series: Emerging Topics in Statistics and Biostatistics (ETSB)
38k Accesses
24 Citations
2 Altmetric
This is a preview of subscription content, log in via an institution to check access.
Access this book
Subscribe and save.
- Get 10 units per month
- Download Article/Chapter or eBook
- 1 Unit = 1 Article or 1 Chapter
- Cancel anytime
- Available as EPUB and PDF
- Read on any device
- Instant download
- Own it forever
- Compact, lightweight edition
- Dispatched in 3 to 5 business days
- Free shipping worldwide - see info
- Durable hardcover edition
Tax calculation will be finalised at checkout
Other ways to access
Licence this eBook for your library
Institutional subscriptions
About this book
This book brings together the voices of leading experts in the frontiers of biostatistics, biomedicine, and the health sciences to discuss the statistical procedures, useful methods, and novel applications in biostatistics research. It also includes discussions of potential future directions of biomedicine and new statistical developments for health research, with the intent of stimulating research and fostering the interactions of scholars across health research related disciplines. Topics covered include:
Health data analysis and applications to EHR data
Clinical trials, FDR, and applications in health science
Big network analytics and its applications in GWAS
Survival analysis and functional data analysis
Graphical modelling in genomic studies
The book will be valuable to data scientists and statisticians who are working in biomedicine and health, other practitioners in the health sciences, and graduate students and researchers in biostatistics and health.
Similar content being viewed by others
Data Analysis
Biostatistics in Clinical Trials
Causal Inference in Biostatistics
- fluorence image data
- clinical trial
- survival analysis
- gene expression analysis
- surveillance streams
- electronic health records
- clustering methods
- flase discovery rate
- Alzheimer's disease
- classification
- support vector machine
- functional data analysis
- genome-wide association studies
- biological network
- infectious disease epidemiology
Table of contents (21 chapters)
Front matter, health data analysis and applications to ehr data, the effective sample size of ehr-derived cohorts under biased sampling.
- Rebecca A. Hubbard, Carolyn Lou, Blanca E. Himes
Non-Gaussian Models for Object Motion Analysis with Time-Lapse Fluorescence Microscopy Images
- Hanyi Yu, Sung Bo Yoon, Robert Kauffman, Jens Wrammert, Adam Marcus, Jun Kong
Alternative Capture-Recapture Point and Interval Estimators Based on Two Surveillance Streams
- Robert H. Lyles, Amanda L. Wilkinson, John M. Williamson, Jiandong Chen, Allan W. Taylor, Amara Jambai et al.
A Uniform Shrinkage Prior in Spatiotemporal Poisson Models for Count Data
- Krisada Lekdee, Chao Yang, Lily Ingsrisawang, Yisheng Li
A Review of Multiply Robust Estimation with Missing Data
- Sixia Chen, David Haziza
Clinical Trials, FDR, and Applications in Health Science
Approaches to combining phase ii proof-of-concept and dose-finding trials.
- Yutao Liu, Ying Kuen Cheung, Naitee Ting, Qiqi Deng
Designs of Early Phase Cancer Trials with Drug Combinations
- José L. Jiménez, Márcio Augusto Diniz, André Rogatko, Mourad Tighiouart
Controlling the False Discovery Rate of Grouped Hypotheses
- Peter W. MacDonald, Nathan Wilson, Kun Liang, Yingli Qin
Classic Linear Mediation Analysis of Complex Survey Data Using Balanced Repeated Replication
- Yujiao Mai, Hui Zhang
A Review of Bayesian Optimal Experimental Design on Different Models
- Hongyan Jiang, Yichuan Zhao
Big Data Analytics and Its Applications
A selective review on statistical techniques for big data.
- Yaqiong Yao, HaiYing Wang
A Selective Overview of Recent Advances in Spectral Clustering and Their Applications
- Yang Xu, Arun Srinivasan, Lingzhou Xue
A Review on Modern Computational Optimal Transport Methods with Applications in Biomedical Research
- Jingyi Zhang, Wenxuan Zhong, Ping Ma
Variable Selection Approaches in High-Dimensional Space
- Bin Luo, Qian Yang, Susan Halabi
Estimation Methods for Item Factor Analysis: An Overview
- Yunxiao Chen, Siliang Zhang
Survival Analysis and Functional Data Analysis
Editors and affiliations, department of mathematics & statistics, georgia state university, atlanta, usa.
Yichuan Zhao
(Din) Ding-Geng Chen
About the editors
Professor Yichuan Zhao is a professor of statistics at Georgia State University in Atlanta. He has a joint appointment as associate member of the Neuroscience Institute, and he is also an affiliated faculty member of the School of Public Health at Georgia State University. His current research interest focuses on survival analysis, empirical likelihood methods, nonparametric statistics, analysis of ROC curves, bioinformatics, Monte Carlo methods, and statistical modelling of fuzzy systems. He has published 100 research articles in statistics and biostatistics, has co-edited four books on statistics, biostatistics and data science, and has been invited to deliver more than 200 research talks nationally and internationally. Dr. Zhao has organized the Workshop Series on Biostatistics and Bioinformatics since its initiation in 2012. He also organized the 25th ICSA Applied Statistics Symposium in Atlanta as a chair of the organizing committee to great success. He is currently serving as associate editor, or on the editorial board, for several statistical journals. Dr. Zhao is a Fellow of the American Statistical Association, an elected member of the International Statistical Institute, and serves on the Board of Directors, ICSA.
Professor (Din) Ding-Geng Chen is a fellow of the American Statistical Association. He is an honorary professor at the School of Mathematics, Statistics and Computer Science, University of KwaZulu-Natal, South Africa and an extraordinary professor at the Department of Statistics, University of Pretoria, South Africa. Professor Chen has written more than 200 refereed publications and co-authored/co-edited 33 books on clinical trial methodology, meta-analysis, causal inference and public health statistics. This work is partially supported by the National Research Foundation of South Africa (Grant Number 127727) and the South African National Research Foundation (NRF) and South African Medical Research Council(SAMRC) (South African DST-NRF-SAMRC SARChI Research Chair in Biostatistics, Grant Number 114613).
Bibliographic Information
Book Title : Modern Statistical Methods for Health Research
Editors : Yichuan Zhao, (Din) Ding-Geng Chen
Series Title : Emerging Topics in Statistics and Biostatistics
DOI : https://doi.org/10.1007/978-3-030-72437-5
Publisher : Springer Cham
eBook Packages : Mathematics and Statistics , Mathematics and Statistics (R0)
Copyright Information : The Editor(s) (if applicable) and The Author(s), under exclusive license to Springer Nature Switzerland AG 2021
Hardcover ISBN : 978-3-030-72436-8 Published: 15 October 2021
Softcover ISBN : 978-3-030-72439-9 Published: 15 October 2022
eBook ISBN : 978-3-030-72437-5 Published: 14 October 2021
Series ISSN : 2524-7735
Series E-ISSN : 2524-7743
Edition Number : 1
Number of Pages : XXV, 496
Number of Illustrations : 82 b/w illustrations
Topics : Statistics for Life Sciences, Medicine, Health Sciences , Biomedicine, general
- Publish with us
Policies and ethics
- Find a journal
- Track your research

An official website of the United States government
The .gov means it’s official. Federal government websites often end in .gov or .mil. Before sharing sensitive information, make sure you’re on a federal government site.
The site is secure. The https:// ensures that you are connecting to the official website and that any information you provide is encrypted and transmitted securely.
- Publications
- Account settings
- My Bibliography
- Collections
- Citation manager
Save citation to file
Email citation, add to collections.
- Create a new collection
- Add to an existing collection
Add to My Bibliography
Your saved search, create a file for external citation management software, your rss feed.
- Search in PubMed
- Search in NLM Catalog
- Add to Search
An overview of commonly used statistical methods in clinical research
Affiliations.
- 1 Center for Surgical Outcomes Research, The Research Institute at Nationwide Children's Hospital, Columbus, OH, USA.
- 2 Department of Surgery, Children's Mercy Hospital, 2401 Gillham Road, Kansas City, MO 64108, USA. Electronic address: [email protected].
- PMID: 30473041
- DOI: 10.1053/j.sempedsurg.2018.10.008
Statistics plays an essential role in clinical research by providing a framework for making inferences about a population of interest. In order to interpret research datasets, clinicians involved in clinical research should have an understanding of statistical methodology. This article provides a brief overview of statistical methods that are frequently used in clinical research studies. Descriptive and inferential methods, including regression modeling and propensity scores, are discussed, with focus on the rationale, assumptions, strengths, and limitations to their application.
Keywords: Descriptive statistics; Inferential statistics; Propensity scores; Regression analysis; Survival analysis.
Copyright © 2018 Elsevier Inc. All rights reserved.
PubMed Disclaimer
Similar articles
- Study design: the basics. Lim HJ, Hoffmann RG. Lim HJ, et al. Methods Mol Biol. 2007;404:1-17. doi: 10.1007/978-1-59745-530-5_1. Methods Mol Biol. 2007. PMID: 18450042 Review.
- Evaluating statistics in clinical trials: making the unintelligible intelligible. Gilmore SJ. Gilmore SJ. Australas J Dermatol. 2008 Nov;49(4):177-84; quiz 185-6. doi: 10.1111/j.1440-0960.2008.00465_1.x. Australas J Dermatol. 2008. PMID: 18855776 Review.
- Biostatistics primer: part 2. Overholser BR, Sowinski KM. Overholser BR, et al. Nutr Clin Pract. 2008 Feb;23(1):76-84. doi: 10.1177/011542650802300176. Nutr Clin Pract. 2008. PMID: 18203967
- Statistical problems in medical research. Okeh UM. Okeh UM. East Afr J Public Health. 2009 Apr;6 Suppl(1):1-7. doi: 10.4314/eajph.v6i3.45762. East Afr J Public Health. 2009. PMID: 20088069
- Biostatistics primer: part I. Overholser BR, Sowinski KM. Overholser BR, et al. Nutr Clin Pract. 2007 Dec;22(6):629-35. doi: 10.1177/0115426507022006629. Nutr Clin Pract. 2007. PMID: 18042950 Review.
- Type and approach of hysterectomy and oncological survival of women with stage II cancer of endometrium: a large retrospective cohort study. Shuai X, Xiao D, Han B, Du Y. Shuai X, et al. Front Oncol. 2024 May 13;14:1404831. doi: 10.3389/fonc.2024.1404831. eCollection 2024. Front Oncol. 2024. PMID: 38803540 Free PMC article.
- Say farewell to bland regression reporting: Three forest plot variations for visualizing linear models. Fries J, Oberleiter S, Pietschnig J. Fries J, et al. PLoS One. 2024 Feb 2;19(2):e0297033. doi: 10.1371/journal.pone.0297033. eCollection 2024. PLoS One. 2024. PMID: 38306346 Free PMC article.
- Correlation between hypertensive retinopathy and fetal outcomes in patients with preeclampsia in a tertiary care hospital: A prospective cohort study. Jacob N, Kasturi N, Deb AK, Maurya DK. Jacob N, et al. Indian J Ophthalmol. 2023 Mar;71(3):910-915. doi: 10.4103/IJO.IJO_2995_22. Indian J Ophthalmol. 2023. PMID: 36872708 Free PMC article.
- Genotyping Helicobacter pylori and fgf7 gene expression in gastric cancer. Hedayati MA, Khani D, Bashiri H. Hedayati MA, et al. Mol Biol Rep. 2022 Sep;49(9):8827-8834. doi: 10.1007/s11033-022-07732-3. Epub 2022 Jul 7. Mol Biol Rep. 2022. PMID: 35799083
- A systematic review and meta-analysis of the effect of transitional care interventions on the prognosis of patients with heart failure. Lin X, Ji R, Wang X, Xin R, Chen Q. Lin X, et al. J Thorac Dis. 2022 Apr;14(4):1164-1171. doi: 10.21037/jtd-22-102. J Thorac Dis. 2022. PMID: 35572902 Free PMC article.
Publication types
- Search in MeSH
LinkOut - more resources
Full text sources.
- Elsevier Science
- MedlinePlus Health Information
- Citation Manager
NCBI Literature Resources
MeSH PMC Bookshelf Disclaimer
The PubMed wordmark and PubMed logo are registered trademarks of the U.S. Department of Health and Human Services (HHS). Unauthorized use of these marks is strictly prohibited.
- For Individuals
- For Businesses
- For Universities
- For Governments
- Online Degrees
- Find your New Career
- Join for Free

Statistical Analysis with R for Public Health Specialization
Master Statistics for Public Health and Learn R. Develop your statistical thinking skills and learn key data analysis methods through R
Taught in English
Some content may not be translated

Instructors: Victoria Cornelius +1 more
Instructors
Financial aid available
33,942 already enrolled

Specialization - 4 course series
(1,694 reviews)
Recommended experience
Beginner level
Familiarity with seeing graphs and tables. Basic numeracy (so NOT calculus, trigonometry etc). No medical, statistical or R knowledge is assumed.
What you'll learn
Recognise the key components of statistical thinking in order to defend the critical role of statistics in modern public health research and practice
Describe a given data set from scratch using descriptive statistics and graphical methods as a first step for more advanced analysis using R software
Apply appropriate methods in order to formulate and examine statistical associations between variables within a data set in R
Interpret the output from your analysis and appraise the role of chance and bias as explanations for your results
Skills you'll gain
- Logistic Regression
- Linear Regression
- Statistical Thinking
- Survival Analysis
- Data analysis with R
Details to know

Add to your LinkedIn profile
See how employees at top companies are mastering in-demand skills
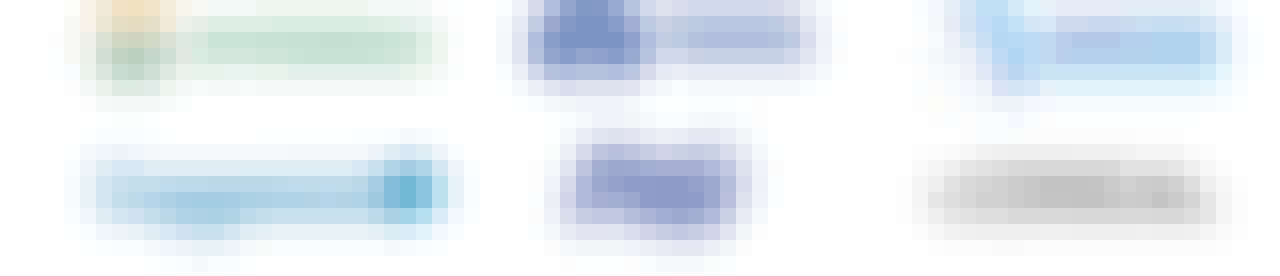
Advance your subject-matter expertise
- Learn in-demand skills from university and industry experts
- Master a subject or tool with hands-on projects
- Develop a deep understanding of key concepts
- Earn a career certificate from Imperial College London
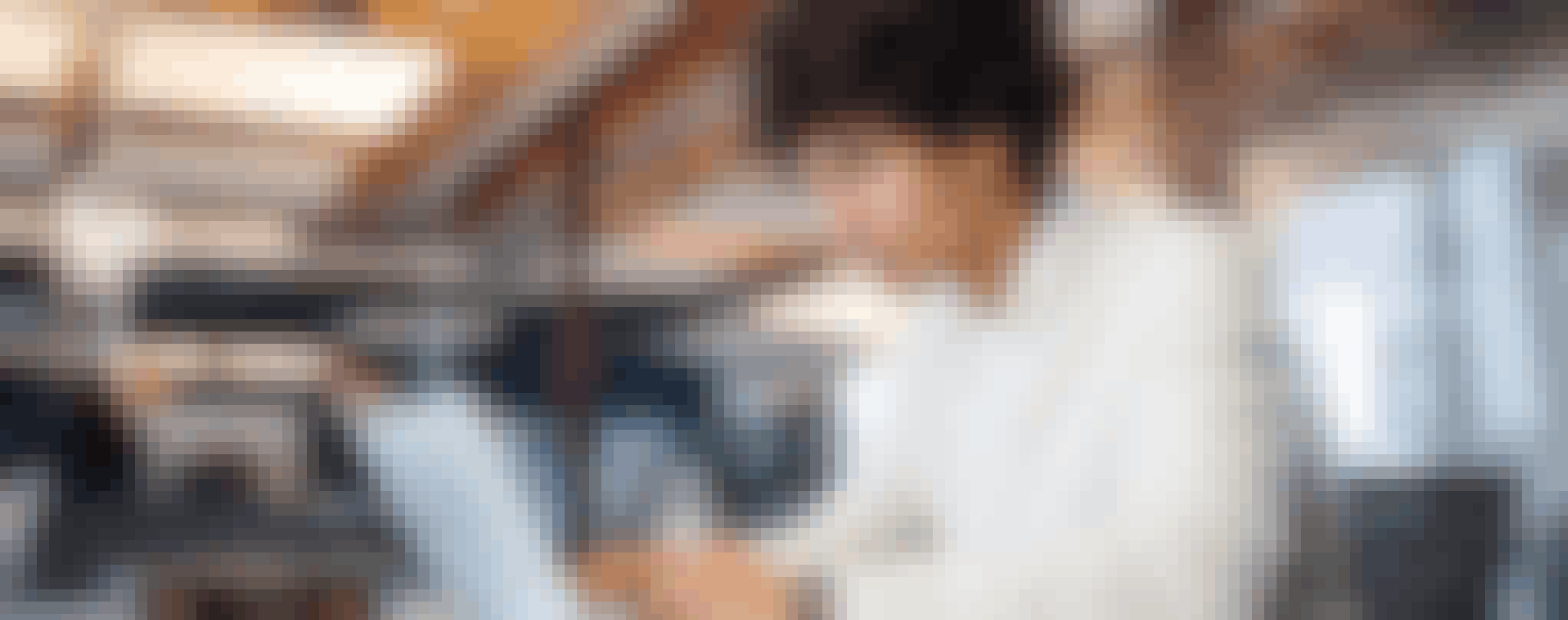
Earn a career certificate
Add this credential to your LinkedIn profile, resume, or CV
Share it on social media and in your performance review
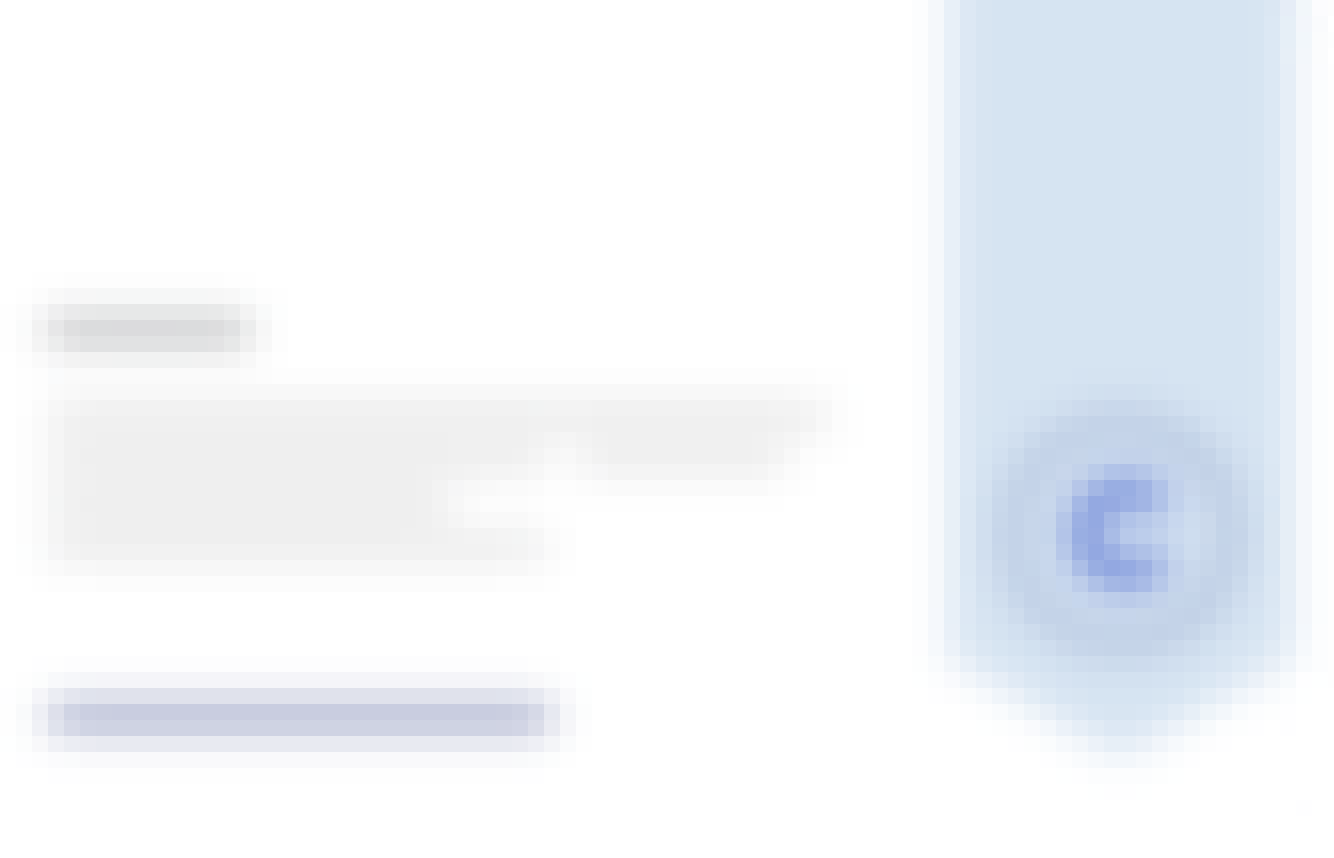
Statistics are everywhere. The probability it will rain today. Trends over time in unemployment rates. The odds that India will win the next cricket world cup. In sports like football, they started out as a bit of fun but have grown into big business. Statistical analysis also has a key role in medicine, not least in the broad and core discipline of public health.
In this specialisation, you’ll take a peek at what medical research is and how – and indeed why – you turn a vague notion into a scientifically testable hypothesis. You’ll learn about key statistical concepts like sampling, uncertainty, variation, missing values and distributions. Then you’ll get your hands dirty with analysing data sets covering some big public health challenges – fruit and vegetable consumption and cancer, risk factors for diabetes, and predictors of death following heart failure hospitalisation – using R, one of the most widely used and versatile free software packages around.
This specialisation consists of four courses – statistical thinking, linear regression, logistic regression and survival analysis – and is part of our upcoming Global Master in Public Health degree, which is due to start in September 2019.
The specialisation can be taken independently of the GMPH and will assume no knowledge of statistics or R software. You just need an interest in medical matters and quantitative data.
Applied Learning Project
In each course, you'll be introduced to key concepts and a data set to be used as a worked example throughout that course. Public health data are messy, with missing values and weird distributions all too common. The data you'll use are either real or simulated from real patient-level data sets (all anonymised and with usage permissions in place).
The emphasis will be on “learning through doing” and “learning through discovery” as you encounter typical data and analysis problems for you to solve and discuss among your fellow learners. You'll get the chance to work things out for yourself and with your peers before accessing the answers and explanation provided by the instructors.
Introduction to Statistics & Data Analysis in Public Health
Defend the critical role of statistics in modern public health research and practice
Describe a data set from scratch, including data item features and data quality issues, using descriptive statistics and graphical methods in R
Select and apply appropriate methods to formulate and examine statistical associations between variables within a data set in R
Interpret the output from your analysis and appraise the role of chance and bias
Linear Regression in R for Public Health
Describe when a linear regression model is appropriate to use
Read in and check a data set's variables using the software R prior to undertaking a model analysis
Fit a multiple linear regression model with interactions, check model assumptions and interpret the output
Logistic Regression in R for Public Health
Describe a data set from scratch using descriptive statistics and simple graphical methods as a first step for advanced analysis using R software
Interpret the output from your analysis and appraise the role of chance and bias as potential explanations
Run multiple logistic regression analysis in R and interpret the output
Evaluate the model assumptions for multiple logistic regression in R
Survival Analysis in R for Public Health
Run Kaplan-Meier plots and Cox regression in R and interpret the output
Describe a data set from scratch, using descriptive statistics and simple graphical methods as a necessary first step for more advanced analysis
Describe and compare some common ways to choose a multiple regression model

Imperial College London is a world top ten university with an international reputation for excellence in science, engineering, medicine and business. located in the heart of London. Imperial is a multidisciplinary space for education, research, translation and commercialisation, harnessing science and innovation to tackle global challenges. Imperial students benefit from a world-leading, inclusive educational experience, rooted in the College’s world-leading research. Our online courses are designed to promote interactivity, learning and the development of core skills, through the use of cutting-edge digital technology.
Prepare for a degree
Taking this Specialization by Imperial College London may provide you with a preview of the topics, materials and instructors in a related degree program which can help you decide if the topic or university is right for you.
Imperial College London
Global Master of Public Health
Degree · 2 or 3 years
Why people choose Coursera for their career

New to Public Health? Start here.

Open new doors with Coursera Plus
Unlimited access to 7,000+ world-class courses, hands-on projects, and job-ready certificate programs - all included in your subscription
Advance your career with an online degree
Earn a degree from world-class universities - 100% online
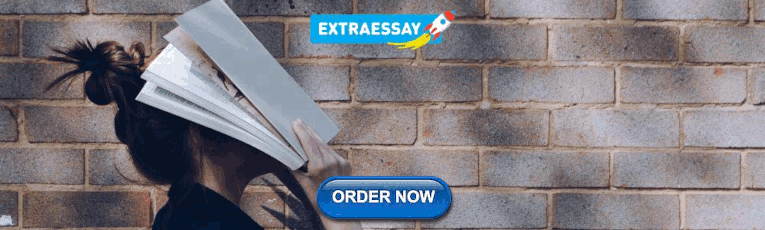
Join over 3,400 global companies that choose Coursera for Business
Upskill your employees to excel in the digital economy
Frequently asked questions
How long does it take to complete the specialization.
3/4 hours a week for 3 to 4 months
What background knowledge is necessary?
The specialisation will assume no knowledge of statistics or R software.
Do I need to take the courses in a specific order?
We recommend taking the courses in the order in which they are displayed on the main page of the Specialization
Is this course really 100% online? Do I need to attend any classes in person?
This course is completely online, so there’s no need to show up to a classroom in person. You can access your lectures, readings and assignments anytime and anywhere via the web or your mobile device.
What is the refund policy?
If you subscribed, you get a 7-day free trial during which you can cancel at no penalty. After that, we don’t give refunds, but you can cancel your subscription at any time. See our full refund policy Opens in a new tab .
Can I just enroll in a single course?
Yes! To get started, click the course card that interests you and enroll. You can enroll and complete the course to earn a shareable certificate, or you can audit it to view the course materials for free. When you subscribe to a course that is part of a Specialization, you’re automatically subscribed to the full Specialization. Visit your learner dashboard to track your progress.
Is financial aid available?
Yes. In select learning programs, you can apply for financial aid or a scholarship if you can’t afford the enrollment fee. If fin aid or scholarship is available for your learning program selection, you’ll find a link to apply on the description page.
Can I take the course for free?
When you enroll in the course, you get access to all of the courses in the Specialization, and you earn a certificate when you complete the work. If you only want to read and view the course content, you can audit the course for free. If you cannot afford the fee, you can apply for financial aid Opens in a new tab .
Will I earn university credit for completing the Specialization?
This Specialization doesn't carry university credit, but some universities may choose to accept Specialization Certificates for credit. Check with your institution to learn more.
More questions
The Use of Statistics in Health Sciences: Situation Analysis and Perspective
- October 2016
- Statistics in Biosciences 8(2):204–219
- 8(2):204–219

- The Andalusian School of Public Health
Abstract and Figures

Discover the world's research
- 25+ million members
- 160+ million publication pages
- 2.3+ billion citations

- Aurora García‐Camacha Gutiérrez

- Jaime Andrés Gaviria-Bedoya Gaviria-Bedoya

- Azizah Rahmat
- Zalizah Awang Long

- Kannan Thiruvengadam
- Basilea Watson

- Ezalina Ezalina
- Deswinda Deswinda
- Fitry Erlin

- Mark Monmonier
- P. Armitage
- J.N.S. Matthews
- Patricia Gilmartin

- John A. Lewis

- R.A. Fisher

- Recruit researchers
- Join for free
- Login Email Tip: Most researchers use their institutional email address as their ResearchGate login Password Forgot password? Keep me logged in Log in or Continue with Google Welcome back! Please log in. Email · Hint Tip: Most researchers use their institutional email address as their ResearchGate login Password Forgot password? Keep me logged in Log in or Continue with Google No account? Sign up
🚀 Think you’ve got what it takes for a career in Data? Find out in just one minute!

Healthcare Data Analytics is the analysis of health data produced by the medical sector, driving substantial improvements in care and clinical outcomes. Learn everything you need to know about this groundbreaking field: types of data, analysis methods, concrete applications... here’s how Data Science is transforming the modern medical landscape!
Since the dawn of time, humanity has endeavored to enhance health and stretch the boundaries of medicine . From the herbalists of ancient civilizations to contemporary scientific discoveries, the objective has always been consistent.
The focus is on understanding diseases, discovering effective treatments, and delivering care that prolongs and enhances human life . Every advancement, whether stemming from empirical observation or experimental methods, has contributed to the development and expansion of a vast body of medical knowledge . Now, in the digital age, a new frontier is emerging: data. The plethora of data generated by modern health equipment, personal tracking technologies, and clinical trials provides an immensely valuable source of information.
When properly collected, analyzed, and interpreted, these data can revolutionize the way we diagnose diseases , administer treatments , and manage healthcare systems on a global scale. This approach represents a key to unlocking the hidden potential of medical data by merging the capabilities of computing, statistics, and artificial intelligence: this is Healthcare Data Analytics.
What is health data?
With the advent of computer and digital technologies, the healthcare sector has begun generating a wide array of data.
Firstly, clinical data includes Electronic Health Records (EHRs) , which encompass detailed information about patients’ medical history, diagnoses, treatments, and laboratory and imaging test results. These data are essential for monitoring patients’ health progress and evaluating the effectiveness of medical interventions, making them central to Healthcare Data Analytics .
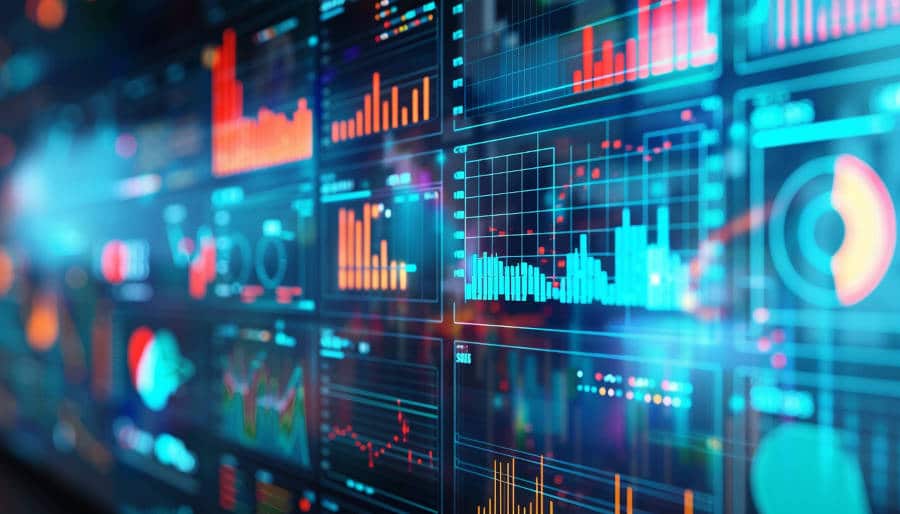
Often underappreciated, administrative data are also highly valuable for optimizing hospital operations. This data includes billing information, diagnostic and treatment codes, and patient admissions and discharge data. Analyzing these helps to identify trends and inefficiencies in administrative processes, facilitating better resource allocation and cost reduction.
With the rise of mobile technologies and health tracking devices such as smartwatches, patients themselves are increasingly generating data. Health applications on smartphones, smartwatches, and other wearable devices collect real-time data on parameters such as heart rate, physical activity levels, and sleep patterns.
All these data provide insights into daily health and enable continuous monitoring , allowing for timely interventions when necessary. Additionally, clinical trials generate a wealth of data that is extremely valuable for medical research . The results of trials, observed side effects, and other detailed information can be utilized.
By analyzing these data, researchers can ascertain the efficacy and safety of new treatments and medications , thereby expediting the development of novel therapies. Thus, Healthcare Data Analytics enables healthcare professionals to enhance care quality and make the healthcare system more efficient and patient-centered . But how do we accomplish this?
Different methods of analysis
The analysis of health data employs various methods and techniques to extract relevant information from collected data.
The starting point for exploring these data is often descriptive analysis. This involves using descriptive statistics like the mean, median, and standard deviation, alongside visualization techniques such as graphs and tables. These tools help to understand the basic characteristics of the data, identify trends and patterns, and summarize information for easy interpretation.
On the other hand, diagnostic analysis aims to uncover the underlying causes of health events or clinical outcomes. Advanced statistical techniques are employed to determine factors contributing to certain medical conditions or specific outcomes. For instance, the goal might be to study the risk factors associated with a particular disease or assess the impact of medical interventions on patient health .
To forecast future events or health outcomes, one can utilize predictive analysis. This method uses statistical models and Machine Learning algorithms to predict future events or health outcomes. The idea is to analyze historical data to identify trends and patterns that can be used to anticipate patient needs , foresee medical complications, or optimize treatments. These predictive models could be used, for example, to predict the risk of hospital readmission or recommend personalized adjustments in treatment plans .
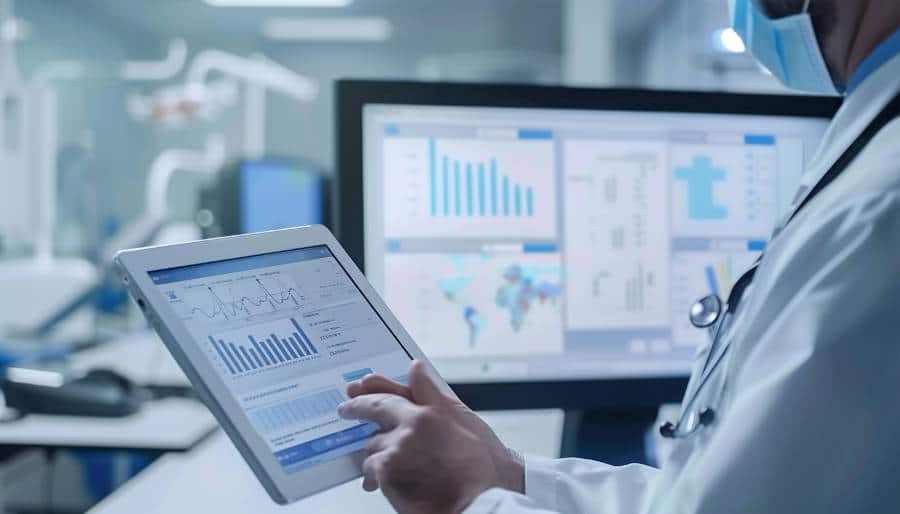
Beyond prediction, prescriptive analysis offers data-driven recommendations to enhance health outcomes.
It employs advanced methods such as mathematical optimization and simulations to determine the best actions to take based on specific patient conditions and clinical constraints. This might involve suggesting adjustments in medication dosages or chronic disease management strategies to optimize therapeutic outcomes.
What is it for?
Practical applications of Healthcare Data Analytics are myriad and diverse. Firstly, it enables the improvement of care quality . By identifying inefficient clinical practices and enhancing adherence to care protocols , medical errors can be significantly reduced.
Moreover, data analysis supports the personalization of treatments by tailoring care plans to individual patient needs. This enhances clinical outcomes. For patients with chronic diseases such as diabetes or hypertension, data analysis facilitates the continuous monitoring of health parameters.
This encourages the early detection of fluctuations, allowing for prompt interventions to prevent complications. Thus, it is an invaluable tool in battling such diseases. Furthermore, by analyzing administrative and operational data, hospitals can optimize resource management .
This optimization helps reduce patient waiting times and improve the efficiency of logistical processes such as medication stock management and bed rotation planning. Lastly, in medical research, data analysis assists in identifying emerging trends by providing a deeper understanding of clinical trials. This sets the stage for new discoveries and innovations in the development of new treatments and therapies through collaborative efforts!
The challenges that remain to be addressed
Despite the numerous benefits, Healthcare Data Analytics also presents significant challenges that must be addressed to ensure its effectiveness and future security.
Firstly, the protection of personal health information is a major concern. Health data are often sensitive, and compromising this information can have severe consequences for patients.
To prevent cyberattacks and unauthorized access, robust information security measures must be implemented. Compliance with regulations such as GDPR in the EU and HIPAA in the United States is essential. Additionally, the accuracy and reliability of health data are critical . Without these attributes, analyses cannot be accurate. The challenge lies in data normalization from diverse sources, managing errors and inconsistencies , and ensuring the completeness of records. Significant effort must be made throughout the analysis process to enhance data quality and maintain its integrity.
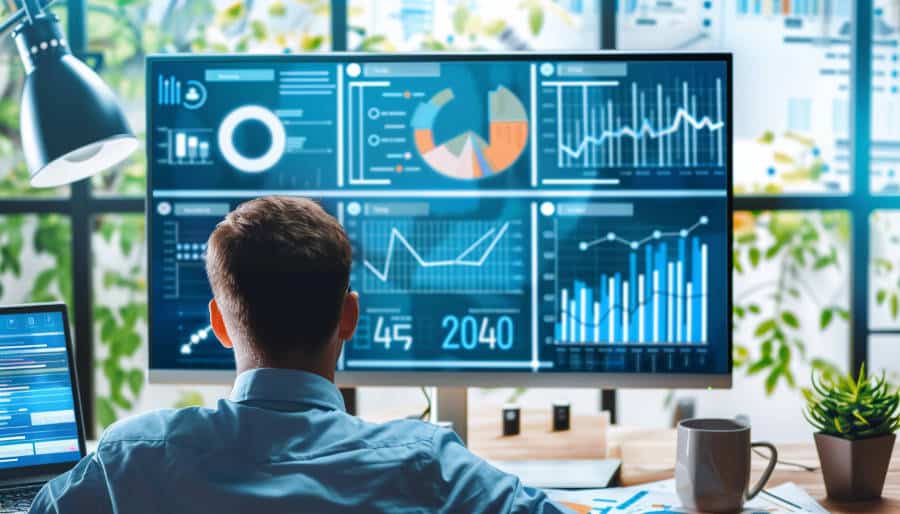
Furthermore, Healthcare Data Analytics necessitates advanced technologies and a robust infrastructure . This ensures the efficient and secure storage, management, and analysis of large volumes of data. Beyond technical considerations, the use of health data raises important ethical questions.
How can we ensure equity in access to care ? How can we utilize patient data ethically? These are pressing questions for professionals in the field. It is also crucial to minimize potential biases in analysis algorithms and ensure clinical decisions are not influenced by inappropriate factors.
Conclusion: Healthcare Data Analytics, the key to strengthening healthcare systems
y presenting significant opportunities to enhance patient care , optimize hospital operations , and promote medical innovation, Healthcare Data Analytics signifies a true revolution in the healthcare sector.
However, to fully harness its potential, it is critical to address challenges related to data security , technological infrastructure, and ethical considerations. To become an expert in health data analysis, consider choosing DataScientest. Our online training courses provide you with all the essential skills for careers in Data Science .
You will learn to collect , transform , analyze data , and present it through relevant visualizations. We offer training in handling all the tools and techniques used by Data Analysts, Data Scientists, and Data Engineers . Our programs are conducted in BootCamp, continuous training, or work-study formats, leading to a state-recognized diploma and a professional certification . Our organization is also CPF eligible for funding. Discover DataScientest!
You now know everything about Healthcare Data Analytics. For more information on the same topic, check out our comprehensive article on data analysis and our guide on the Data Analyst profession !
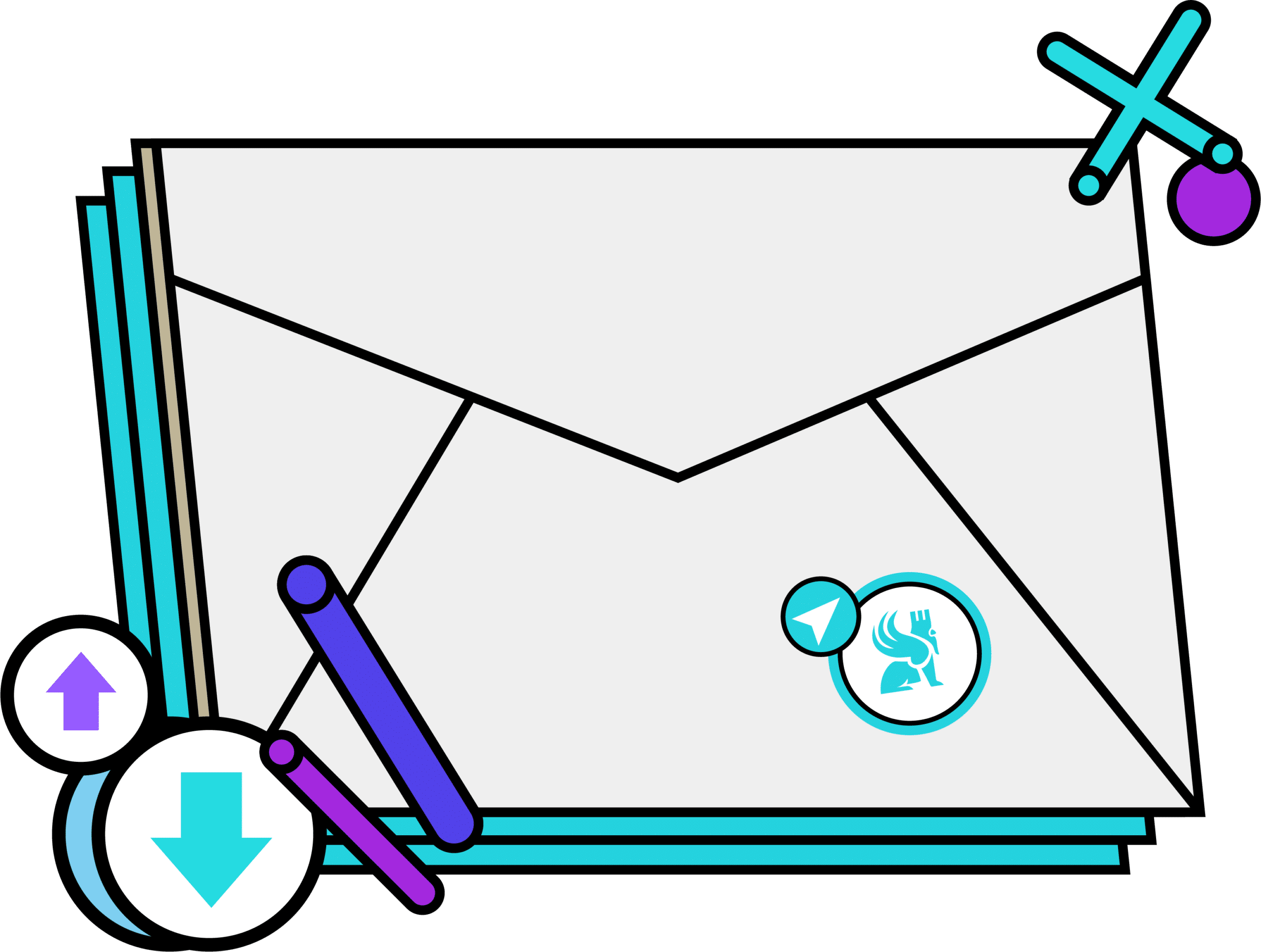
DataScientest News
Sign up for our Newsletter to receive our guides, tutorials, events, and the latest news directly in your inbox.
You are not available?
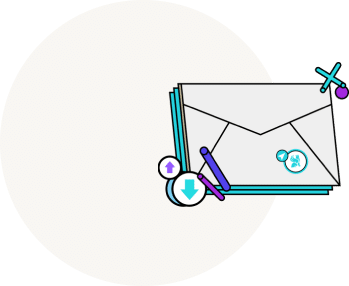
Related articles
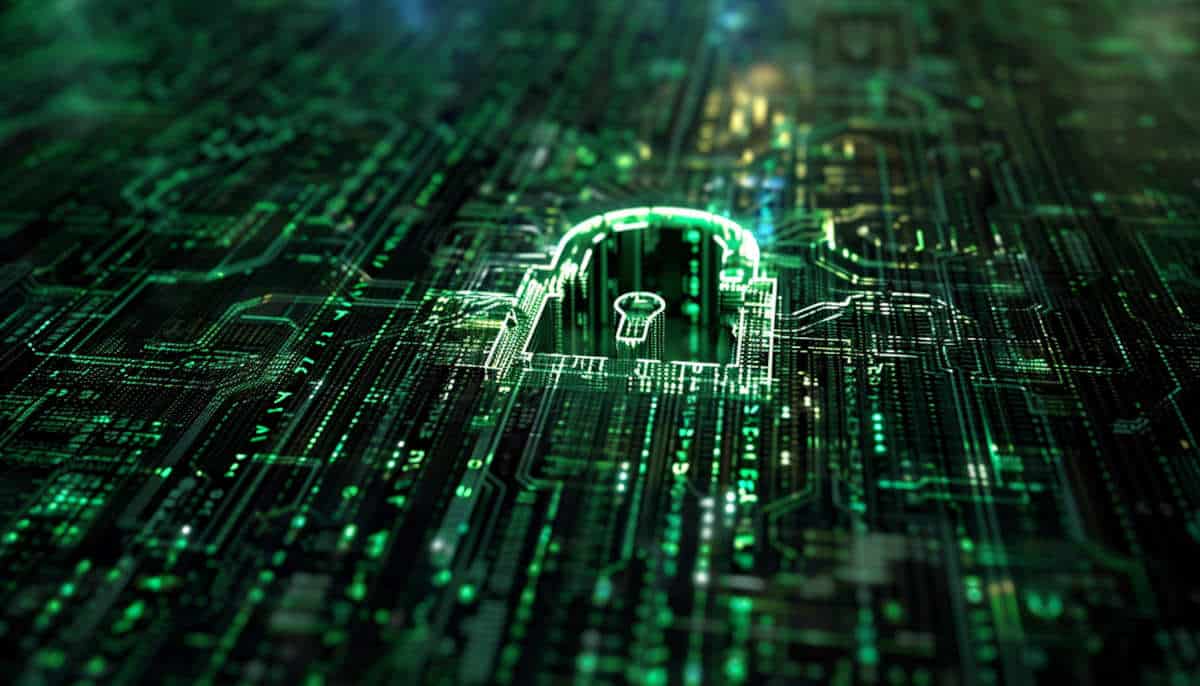
Cryptography: What is it? How does it work?
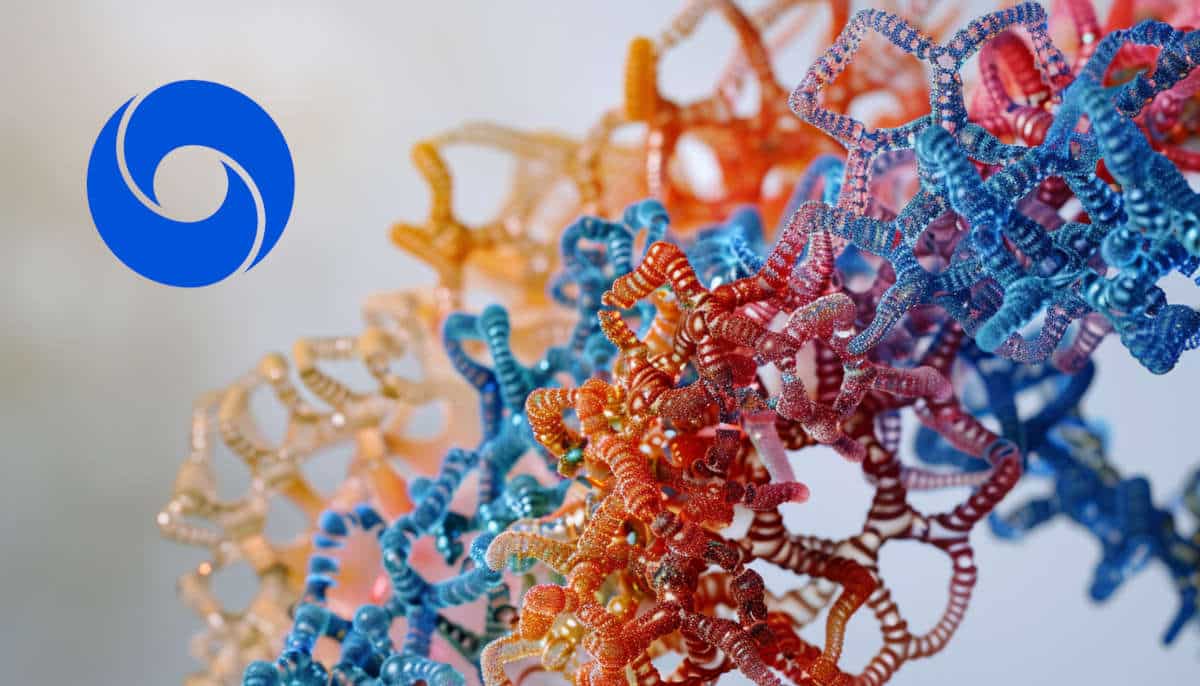
What is AlphaFold?
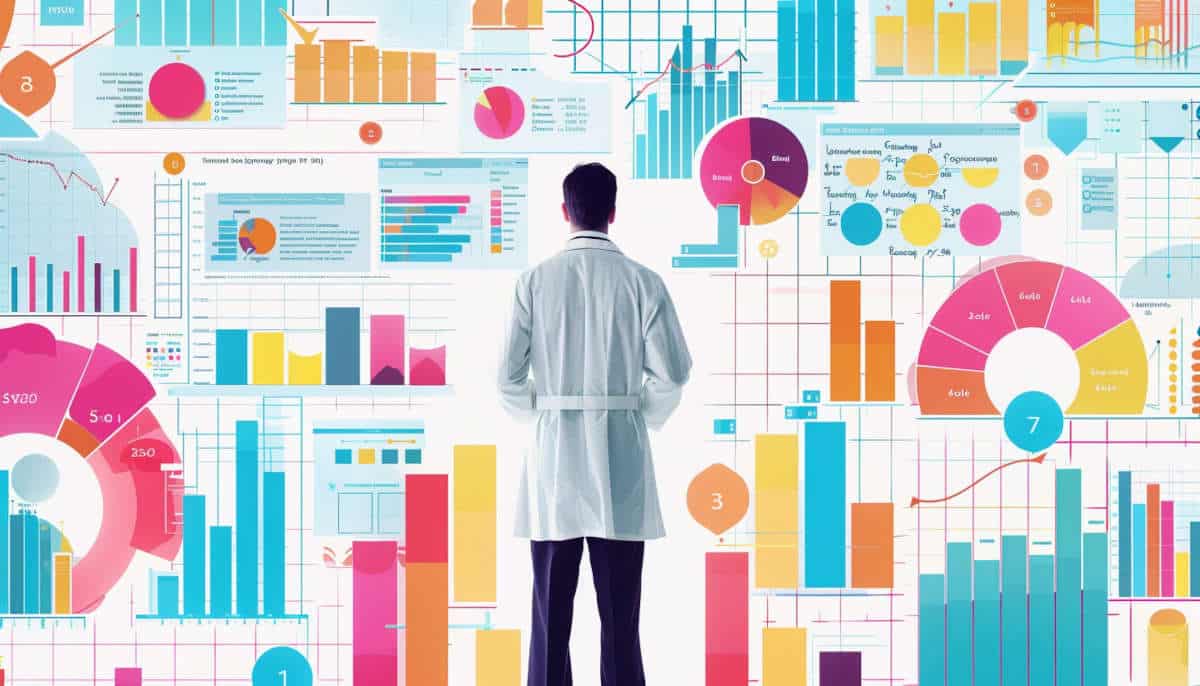
What is a Certified Ethical Hacker (CEH)?
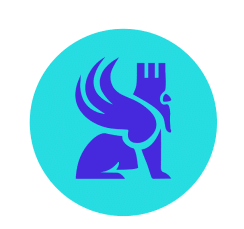
Get monthly insider insights from experts directly in your mailbox

Course details
- Mon 27 Jan 2025 to 21 Mar 2025
Introduction to Statistics for Health Care Research
Take your first steps to unlock the power of statistics
This eight week online statistics module is designed for health and social care professionals who want to understand the basics of analysis methods commonly used in medical research, in order to understand published research and to participate in more specialised courses. Students will learn to use and interpret basic statistical methods using SPSS, with reference to cohort studies, case control studies and randomized controlled trials. Online discussion forums enable communication between students and the tutor to provide support and to interpret and understand real-life scenarios.
The last date for receipt of complete applications is 5pm Friday 27th September 2024 . Regrettably, late applications cannot be accepted.
The overall aims of this module are to enable students to:
- Understand and manipulate basic mathematical and statistical techniques as preparation for more specialized courses in health care;
- Understand the statistical issues in the planning and analysis of research;
- Understand the basic statistical aspects of published research;
- Apply statistical analysis to cohort studies, case control studies and randomized controlled trials.
No previous knowledge of statistics is required to take this module. Students who are already familiar with the majority of the topics listed should consider taking the Essential Medical Statistics module.
For a detailed comparison of these two courses please click here.
This module will cover:
This course is divided into 8 units. Each unit covers a different aspect of statistics and statistical modelling.
Individual units are built around practical exercises and realistic case studies that will enable students to apply statistical knowledge in a health context.
Weekly study for each unit is self-paced using online resources. Movement through the units is structured week by week to enable students to complete sections at the same time as the rest of their course group.
Individual activities can involve reading of related text book chapters and articles, working through problems or analysing example datasets. The group activities will mainly involve online discussions of important issues in statistics so that students can put the application of statistics into an everyday context. Discussions occur weekly, but are "asynchronous" due to possible local time differences between the student's locations.
Programme details
Unit Content:
- The use and importance of statistics
- Handling and describing data, learning to use SPSS
- Analysis of numerical results
- Confidence intervals
- Comparison of two means
- Hypothesis testing
- Analysis of variance
- Linear regression and correlation
- Analysing binary outcomes
- Comparing two proportions
Recommended reading
- Kirkwood, B. R. and Sterne, J. A. C. Essential Medical Statistics (Oxford: Wiley-Blackwell, 3rd ed. 2024)
Description | Costs |
---|---|
Short Course in Health Sciences | £3175.00 |
Students enrolled on MSc in EBHC | £2570.00 |
Students enrolled on PG Cert in Health Research | £2570.00 |
Students enrolled on PG Dip in Health Research | £2570.00 |
Details of funding opportunities, including grants, bursaries, loans, scholarships and benefit information are available on our financial assistance page.
If you are an employee of the University of Oxford and have a valid University staff card you may be eligible to receive a 10% discount on the full stand-alone fee. To take advantage of this offer please submit a scan/photocopy of your staff card along with your application. Your card should be valid for a further six months after attending the course.
Dr Paul Bateman
Module co-ordinator.
Pau Bateman is a Statistical Programmer at the Nuffield Department of Primary Care Health Sciences, University of Oxford.
Dr Maria Vazquez Montes
Maria Vazquez Montes is a Senior Statistician at the Nuffield Department of Primary Care Health Sciences, University of Oxford. Her focus is on prognostic and risk prediction research.
Assessment methods
- Each unit includes a data analysis exercise to consolidate understanding
- The assessment consists of statistical problems in health research designed to give insight into real statistical problems in healthcare and to test ability to apply and understand correct statistical analysis.
Academic Credit
Applicants may take this course for academic credit. The University of Oxford Department for Continuing Education offers Credit Accumulation and Transfer Scheme (CATS) points for this course. Participants attending at least 80% of the taught course and successfully completing assessed assignments are eligible to earn credit equivalent to 20 CATS points which may be counted towards a postgraduate qualification.
Applicants can choose not to take the course for academic credit and will therefore not be eligible to undertake the academic assignment offered to students taking the course for credit. Applicants cannot receive CATS (Credit Accumulation and Transfer Scheme) points or equivalence. Credit cannot be attributed retrospectively. CATS accreditation is required if you wish for the course to count towards a further qualification in the future.
A Certificate of Completion is issued at the end of the course.
Applicants registered to attend ‘not for credit’ who subsequently wish to register for academic credit and complete the assignment are required to submit additional information, which must be received one calendar month in advance of the course start date. Please contact us for more details.
Please contact [email protected] if you have any questions.
Application
This course requires you to complete the application form and to attach a copy of your CV. If you are applying to take this course for academic credit you will also be required to provide a reference. Please note that if you are not applying to take the course for academic credit then you do not need to submit a reference.
Please ensure you read the guidance notes which appear when you click on the symbols as you progress through the application form, as any errors resulting from failure to do so may delay your application.
- Short Course Application Form
- Terms and Conditions
Selection criteria
Admissions Criteria: To apply for the course you should:
- Be a graduate or have successfully completed a professional training course
- Have professional work experience in the health service or a health-related field
- Have a good working knowledge of email, internet, word processing and Windows applications (for communications with course members, course team and administration)
- Show evidence of the ability to commit time to study and an employer's commitment to make time available to study, complete course work and attend course and university events and modules.
- Be able to demonstrate English Language proficiency at the University’s higher level .
IT requirements
Please ensure that you have access to a computer that meets the specifications detailed on our technical support page.
Terms & conditions for applicants and students
Information on financial support
Sign up for course news
- MSc in Evidence-Based Health Care
- MSc in EBHC (Teaching and Education)
- Postgraduate Diploma in Health Research
- Postgraduate Certificate in Health Research
and also available as an accredited short course in Health Sciences
Log in using your username and password
- Search More Search for this keyword Advanced search
- Latest content
- For authors
- Browse by collection
- BMJ Journals
You are here
- Volume 14, Issue 8
- Impact of aspirin dose according to race in secondary prevention of atherosclerotic cardiovascular disease: a secondary analysis of the ADAPTABLE randomised controlled trial
- Article Text
- Article info
- Citation Tools
- Rapid Responses
- Article metrics

- http://orcid.org/0000-0003-2965-1504 Guillaume Marquis-Gravel 1 ,
- Hillary Mulder 1 ,
- http://orcid.org/0000-0003-1732-9067 Lisa M Wruck 1 ,
- Catherine P Benziger 2 ,
- Mark B Effron 3 ,
- Peter M Farrehi 4 ,
- Saket Girotra 5 ,
- Kamal Gupta 6 ,
- Sunil Kripalani 7 ,
- Daniel Muñoz 7 ,
- Tamar S Polonsky 8 ,
- http://orcid.org/0000-0002-9544-838X Jeff Whittle 9 ,
- Robert Harrington 10 ,
- Russell Rothman 7 ,
- Adrian F Hernandez 11 ,
- WS Jones 12
- 1 Duke Clinical Research Institute , Durham , North Carolina , USA
- 2 Heart and Vascular Center , Essentia Health , Duluth , Minnesota , USA
- 3 John Ochsner Heart and Vascular Institute , New Orleans , Louisiana , USA
- 4 University of Michigan , Ann Arbor , Michigan , USA
- 5 University Texas Southwestern Medical Center , Dallas , Texas , USA
- 6 University of Kansas Medical Center , Kansas City , Kansas , USA
- 7 Vanderbilt University Medical Center , Nashville , Tennessee , USA
- 8 University of Chicago Medicine , Chicago , Illinois , USA
- 9 Medical College of Wisconsin , Milwaukee , Wisconsin , USA
- 10 Stanford University , Stanford , California , USA
- 11 Duke University , Durham , North Carolina , USA
- 12 Duke University Medical Center , Duke Clinical Research Institute , Durham , North Carolina , USA
- Correspondence to Dr Guillaume Marquis-Gravel; guillaume.marquis.gravel{at}umontreal.ca
Objectives To evaluate whether the effectiveness and safety of low (81 mg daily) versus high-dose (325 mg daily) aspirin is consistent across races among patients with established atherosclerotic cardiovascular disease (ASCVD).
Design A secondary analysis of the randomised controlled trial ADAPTABLE was performed.
Setting The study was conducted in 40 centres and one health plan participating in the National Patient-Centred Clinical Research Network (PCORnet) in the USA.
Participants Among 15 076 participants with established ASCVD, 14 096 had self-reported race available and were included in the analysis. Participants were divided according to self-reported race as Black (n=1311, 9.3%), White (n=11 990, 85.1%) or other race (n=795, 5.6%).
Interventions Participants were randomised to open-label daily aspirin doses of 81 mg versus 325 mg in a 1:1 ratio for a median of 26.2 months.
Primary and secondary outcomes measures The primary effectiveness endpoint was a composite of death from any cause, hospitalisation for myocardial infarction or hospitalisation for stroke. The primary safety endpoint was hospitalisation for bleeding requiring blood product transfusion.
Results Estimated cumulative incidence of the primary effectiveness endpoint at median follow-up with the 81 mg and the 325 mg daily doses were 6.70% and 7.12% in White participants (adjusted HR: 1.00 [95% CI: 0.88 to 1.15]); 12.27% and 10.69% in Black participants (adjusted HR: 1.40 [95% CI: 1.02 to 1.93]); and 6.88% and 7.69% in other participants (adjusted HR: 0.86 [95% CI: 0.54 to 1.39]) (p-interaction=0.12), respectively. There was no significant interaction between self-reported race and assigned aspirin dose regarding the secondary effectiveness and the primary safety endpoints.
Conclusion Race is not an effect modifier on the impact of aspirin dosing on effectiveness and safety in patients with established ASCVD. In clinical practice, treatment decisions regarding aspirin dose in secondary prevention of ASCVD should not be influenced by race.
Trial registration number NCT02697916 .
- Myocardial infarction
- CLINICAL PHARMACOLOGY
Data availability statement
No data are available.
This is an open access article distributed in accordance with the Creative Commons Attribution Non Commercial (CC BY-NC 4.0) license, which permits others to distribute, remix, adapt, build upon this work non-commercially, and license their derivative works on different terms, provided the original work is properly cited, appropriate credit is given, any changes made indicated, and the use is non-commercial. See: http://creativecommons.org/licenses/by-nc/4.0/ .
https://doi.org/10.1136/bmjopen-2023-078197
Statistics from Altmetric.com
Request permissions.
If you wish to reuse any or all of this article please use the link below which will take you to the Copyright Clearance Center’s RightsLink service. You will be able to get a quick price and instant permission to reuse the content in many different ways.
Strengths and limitations of this study
This study was embedded within in the daily routine of participants using a pragmatic, patient-oriented design.
The trial recruited 15 076 patients with the use of multimodal, low-touch recruitment strategies at the health-system level.
Race was self-reported, which may be associated with a reporting bias that is not possible to quantify.
Comparisons across races in this randomised trial should be viewed as hypothesis generating and may be subject to confounding by unmeasured variables.
Race was not available for 980 participants (6.5%) who could not be included in the analyses, which may potentially impact the external generalisability of the results.
Atherosclerotic cardiovascular disease (ASCVD) is associated with a high burden of mortality, morbidity and disability worldwide. 1 In the USA, disparities in outcomes between Black and non-Black patients with ASCVD have been well-documented. 2–4 These differences in clinical outcomes are affected by a complex combination of genetic, environmental, socio-economic, behavioural and healthcare access considerations. 5–7
The antiplatelet medication aspirin inhibits platelet cyclooxygenase-1, prevents clinical events in patients with established ASCVD 8 9 and is recommended in most patients for secondary prevention of cardiovascular events. 10–13 However, platelets of self-reported Black patients respond differently to aspirin compared with platelets of patients of other races in response to different types of agonists. 14 However, whether aspirin dose impacts clinical outcomes differently in Black patients has not been studied before. Given the high burden of ASCVD on the healthcare system, the racial disparities in ASCVD outcomes and the different pharmacodynamic response to aspirin documented in self-reported Black individuals, it is important to determine whether the treatment effect of different doses of aspirin on clinical outcomes also differs. The pragmatic ADAPTABLE randomised controlled trial compared the effect of two different doses of aspirin on clinical outcomes and is well-poised to address this question. 15 The main objective of this secondary analysis of the ADAPTABLE trial is to evaluate whether there is an interaction between race and aspirin dose (81 mg or 325 mg daily) in patients with established ASCVD.
This is a pre-specified analysis of the multi-centre, patient-centred, pragmatic ADAPTABLE randomised trial ( NCT02697916 ) conducted in the USA. The detailed methodology and the main results have been published previously. 15 16 A total of 15 076 patients with established ASCVD from 40 centres and one health plan from the National Patient-Centred Clinical Research Network (PCORnet) were randomised to open-label daily aspirin doses of 81 mg versus 325 mg in a 1:1 ratio. Detailed eligibility criteria are provided in online supplemental methods . Participants were divided according to self-reported race, as White, Black (self-reported as Black or African-American in the trial) or other (which includes Asians, American Indians or Alaska Natives, participants reporting multiple races and other races). Participants who did not report race at baseline or for whom race is missing were excluded from this analysis. Comorbidities and previous medical history of participants were captured from queries of electronic health records. The primary effectiveness endpoint was a composite of death from any cause, hospitalisation for myocardial infarction or hospitalisation for stroke. The individual components of the primary composite endpoint and coronary revascularisation (either percutaneous coronary intervention (PCI) or coronary artery bypass graft (CABG)) were key secondary endpoints. The primary safety endpoint was hospitalisation for bleeding requiring blood product transfusion. Adherence to treatment allocation was self-reported by participants through the study web portal or via telephone interviews with the study call centre. The intention-to-treat population of the ADAPTABLE trial was used for this secondary analysis, which includes all randomised participants. All participants provided written informed consent before entering in the trial. Patient partners (called Adaptors) were involved in the design, conduct and dissemination of the trial and its results. The Duke University institutional review board approved the overall programme (Pro00068525). The study is reported according to the Strengthening the reporting of observational studies in epidemiology (STROBE) statement (see appendix for STROBE checklist). 17
Supplemental material
Statistical analyses.
Baseline continuous variables are presented as median and 25 th /75 th percentiles. Categorical variables are presented as counts and proportions. P values are reported from the X 2 test for categorical measures and from the Kruskal–Wallis test for continuous variables. Missing values were excluded from descriptive statistical summaries.
To evaluate the association between race and clinical outcomes, unadjusted and adjusted Cox proportional hazards models were fit. The Fine–Grey method of accounting for the competing risk of death was used for non-lethal clinical outcomes (ie, hospitalisation for myocardial infarction, hospitalisation for stroke, coronary revascularisation and bleeding requiring blood product transfusion). Pre-specified adjustment factors of the multivariable model for the effectiveness endpoints were age, sex, body mass index (BMI), follow-up strata (3 month or 6 month intervals), non-internet use, current smoker, ethnicity, coronary artery disease (CAD), myocardial infarction, PCI, CABG, cerebrovascular disease, hypertension, hyperlipidemia, atrial fibrillation, congestive heart failure (CHF), peripheral artery disease (PAD), diabetes, prior aspirin dose and current use of P2Y12 inhibitors. Pre-specified adjustment factors of the multivariable model for the safety endpoint were age, sex, BMI, CAD, hypertension, diabetes, history of bleeds, use of non-steroidal anti-inflammatory drugs and current use of P2Y12 inhibitors. The comorbidities are self-reported by participants. The proportional hazards assumption was checked using weighted Schoenfeld residuals. Event rates for each clinical outcome were computed at the median follow-up time of 26.2 months using the Kalbfleisch and Prentice cumulative incidence function estimator for each racial group. Rates, counts, unadjusted and adjusted HRs (reference: White participants) or sub-distribution hazard ratios (SHR) and 95% cCIs were computed, and a forest plot was generated.
To evaluate the clinical outcomes by randomised aspirin dose and race, unadjusted and adjusted Cox proportional hazards models were fit with the covariates described above, race, randomised aspirin dose and an interaction term between randomised aspirin dose and race for the primary effectiveness endpoint, the primary safety endpoint and all-cause mortality. The Fine–Grey method was again used to account for the competing risk of death when evaluating non-lethal endpoints. The interaction term was included to assess if race modifies the effect of randomised aspirin dose on outcomes. A post hoc sensitivity analysis was performed to evaluate the interaction between aspirin dose and race, only including Black and White participants (excluding other races).
In a sensitivity analysis, the current self-reported aspirin dose was included in the model as a time-dependent exposure to account for non-adherence to randomised aspirin dose for the primary effectiveness endpoint, the primary safety endpoint and all-cause death. Models were fit with race, time-varying self-reported aspirin dose and an interaction term between self-reported aspirin dose and race. Adjustment measures were age, sex, ethnicity, prior aspirin dose, prior myocardial infarction, prior percutaneous intervention, history of atrial fibrillation, non-internet user at randomisation, baseline P2Y12 inhibitor use and history of bleeds. This methodology assumes that the decision to switch doses is not associated with factors related to the outcomes of interest beyond the baseline adjustment measures.
All hypothesis tests are two-sided, and p values of <0.05 are considered statistically significant. No penalty for multiple comparisons are applied in this exploratory analysis. Missing data were imputed using fully conditional specification methods across multiple datasets. Analysis was performed by the Duke Clinical Research Institute (Durham, NC) using SAS version 9.4 (SAS Institute, Inc. Cary, NC).
Patient and public involvement
Nine patient partners with lived experience of cardiovascular diseases (the Adaptors) meet twice a month during the design and conduct phases of the ADAPTABLE trial, and they were also invited in the executive and steering committees. They engaged in the development of the research question. They also provided input and developed initiatives to optimise recruitment and retention. The results of the ADAPTABLE trial were disseminated to study participants in a lay manner at the same time the study was published. Details of patient involvement in the trial have been described previously. 18 19
From 15 076 participants, 14 096 had self-reported race available and were included in the analysis, among which 1311 (9.3%) were Black, 11 990 (85.1%) were White and 795 (5.6%) had another self-reported race. The proportion of participants randomised to the 81 mg aspirin dose was similar across races (Black: 50.6%; White: 50.2%; other race: 53.7%; p=0.15). Black participants were more frequently female and more likely to have a number of comorbid conditions, including hypertension, diabetes, CHF and PAD ( table 1 ). In general, adherence was significantly higher in White participants compared with Black participants and participants with other races ( table 2 ). Baseline characteristics of the 980 participants who were excluded because race was not available are reported in online supplemental table 1 .
- View inline
Baseline characteristics by race
Adherence by race and treatment assignment
Over the median follow-up of 26.2 months, the cumulative incidence of the primary effectiveness endpoint was 6.9% in White participants, 11.5% in Black participants and 7.3% in other participants. The unadjusted HRs for Black participants and for other participants versus White participants were 1.68 (95% CI: 1.42 to 1.99) and 1.15 (95% CI: 0.90 to 1.48) (overall p value <0.001), respectively. The adjusted HRs for Black participants and other participants versus White participants were 1.11 (95% CI: 0.91 to 1.34) and 0.85 (95% CI: 0.66 to 1.10) (overall p value=0.20), respectively. There were no significant differences in any of the secondary effectiveness and safety endpoints between racial categories in adjusted analyses ( figure 1 ).
- Download figure
- Open in new tab
- Download powerpoint
Cumulative incidence at the median follow-up and adjusted HRs for the effectiveness and safety endpoints by race. Adjustment variables for the effectiveness endpoints include age, sex, BMI, follow-up strata, non-internet use, current smoker, CAD, MI, PCI, CABG, CVD, hypertension, hyperlipidaemia, atrial fibrillation, CHF, PAD, diabetes, prior aspirin dose and P2Y12 use. Adjustment variables for the safety endpoint include age, sex, BMI, CAD, hypertension, diabetes, history of bleeds, NSAIDs and P2Y12 use. Rates are calculated as the estimated cumulative incidence at the median follow-up (26.2 months). Abbreviations: BMI, body mass index; CABG: coronary artery bypass grafting; CAD, coronary artery disease; CHF, congestive heart failure; CIF, cumulative incidence function; CVD, cardiovascular disease; MI, myocardial infarction; NSAID, nonsteroidal anti-inflammatory drugs; PAD, pulmonary artery disease; PCI, percutaneous coronary intervention.
Among White participants, the estimated cumulative incidence of the primary effectiveness endpoint was 6.7% with the 81 mg aspirin dose and 7.1% with the 325 mg aspirin dose (unadjusted HR: 0.97 [95% CI: 0.85 to 1.11]; adjusted HR: 1.00 [95% CI: 0.88 to 1.15]). Among Black participants, the estimated cumulative incidence of the primary effectiveness endpoint was 12.3% with the 81 mg aspirin dose and 10.7% with the 325 mg aspirin dose (unadjusted HR: 1.36 [95% CI: 0.99 to 1.86]; adjusted HR: 1.40 [95% CI: 1.02 to 1.93]). The estimated cumulative incidence of the primary effectiveness endpoint in participants with other self-reported races was 6.9% with the 81 mg aspirin dose and 7.7% with the 325 mg aspirin dose (unadjusted HR: 0.86 [95% CI: 0.53 to 1.39]; adjusted HR: 0.86 [95% CI: 0.54 to 1.39]). There was no significant interaction between randomised dose and race for the primary effectiveness endpoint (p unadjusted=0.13; p adjusted=0.12), for the primary safety endpoint (p unadjusted=0.36; p adjusted=0.46) and for the secondary effectiveness endpoints ( figure 2 ). A post hoc sensitivity analysis evaluating the interaction between aspirin dose and race, only including a Black and White participants (excluding other races), is presented in online supplemental table 2 ). The cumulative incidence function of the primary effectiveness endpoint by race and aspirin dose is presented in figure 3 . The results of the analysis of interaction between race and assigned aspirin dose were consistent in a sensitivity analysis considering adherence to aspirin dose, in which there was no significant interaction between time-dependent self-reported aspirin dose and race ( table 3 ).
Cumulative incidence at the median follow-up and adjusted HRs for the effectiveness and safety endpoints by randomised aspirin dose and by race. Adjustment variables for the effectiveness endpoints include age, sex, BMI, follow-up strata, non-internet use, current smoker, CAD, MI, PCI, CABG, CVD, hypertension, hyperlipidaemia, atrial fibrillation, CHF, PAD, diabetes, prior aspirin dose and P2Y12 use. Adjustment variables for the safety endpoint include age, sex, BMI, CAD, hypertension, diabetes, history of bleeds, NSAIDs and P2Y12 use. Reference group for the HR is 325 mg. Rates are calculated as the estimated cumulative incidence at median follow-up (26.2 months). Abbreviations: BMI, body mass index; CABG: coronary artery bypass grafting; CAD, coronary artery disease; CHF, congestive heart failure; CIF, cumulative incidence function; CVD, cardiovascular disease; MI, myocardial infarction; NSAID, nonsteroidal anti-inflammatory drugs; PAD, pulmonary artery disease; PCI, percutaneous coronary intervention.
Cumulative incidence function of the primary effectiveness endpoint by race and aspirin dose.
Effect of time-varying self-reported aspirin dose by race
In this secondary analysis of the ADAPTABLE randomised trial, the main finding is that race does not modify the effect of aspirin dosing (81 mg or 325 mg daily) on clinical endpoints in secondary prevention of ASCVD. In Black participants only, the observed event rates of the primary effectiveness endpoint were higher with the 81 mg daily dose (12.3%) versus the 325 mg daily dose (10.7%) (adjusted HR: 1.40 [95% CI: 1.02 to 1.93]), but the overall interaction between race and aspirin dose was not significant (adjusted p value=0.12), indicating that the treatment effect of aspirin dose is consistent across races. Black and non-Black participants in ADAPTABLE had significant differences in demographic characteristics and comorbid conditions, which mirror observational and registry studies of patients with ASCVD. In a study from the National Cardiovascular Data Registry (NCDR) Chest Pain—MI Registry including 155 397 acute MI patients (7.3% black) from 753 US sites from 2008 to 2016, Black patients also had a higher burden of cardiovascular risk factors despite being younger on average, and they were also more likely to be women. 3
Previous real-world studies have shown racial discrepancies in ASCVD management and outcomes. In carotid stenosis treated with endarterectomy, Black patients are less likely prescribed evidence-based medications like aspirin and are more likely to experience a major cardiovascular and cerebrovascular events. 4 In the above-mentioned NCDR Chest Pain MI Registry study, the 30-day readmission rate after MI was significantly higher in Black patients compared with non-Black patients. 3 However, readmission rate was similar across races following multivariable adjustment, suggesting that socioeconomic factors, clinical presentations and comorbidities are responsible for the observed racial disparities in outcomes. In our study, the event rates were also significantly higher in Black versus White participants (unadjusted HR: 1.68; 95% CI: 1.42 to 1.99; overall p value across races <0.001), but the association was no longer significant after covariate adjustment (adjusted HR: 1.11; 95% CI: 0.91 to 1.34; overall p value across races=0.20), thus confirming the registry data, and suggesting that race by itself is not intrinsically related to an adverse prognosis, but that other factors are responsible for this disparity in outcomes. Our results, along with the real-world observational data, call for future studies evaluating the determinants of these differential racial outcomes and implementing strategies to mitigate these disparities.
In the ADAPTABLE trial, there was no significant difference in effectiveness and safety between the two aspirin doses (81 mg and 325 mg once daily) in secondary prevention of ASCVD. 15 The rationale for studying this question is that platelet COX-1 selectivity of aspirin decreases as the dose increases, with a more pronounced COX-2 affinity at higher doses. 20 There is biological plausibility to expect that self-reported race could modify the association between aspirin dose and clinical outcomes. In a study by Infeld and colleagues comparing platelet response to aspirin in 209 self-reported Black versus 282 self-reported European-American patients with or without ASCVD, suppression of platelet activity with aspirin from baseline, as assessed with light transmission aggregometry in response to 0.5 mmol/L of arachidonic acid, was significantly less pronounced in Black individuals (95% CI: − 53.5,–12.1; p<0.01). 14 However, Black patients had lower platelet reactivity at baseline, and racial response to aspirin differed across the spectrum of the agonists used for testing platelet reactivity (collagen, ADP or epinephrine). This raises the hypothesis that Black patients may require different aspirin doses to benefit from a similar treatment effect than non-Black individuals. Our study is the first to have specifically assessed the differential outcomes of aspirin dosing in ASCVD according to race and did not identify race as an effect modifier. However, significantly more primary effectiveness events were observed with the 81 mg dose compared with the 325 mg dose in Black participants only (after multivariable adjustment), raising the hypothesis that platelet reactivity inhibition with higher aspirin doses may be more pronounced in Black patients. While no strong conclusion can be drawn from a non-significant subgroup interaction analysis in a neutral randomised trial, the enrollment of a smaller cohort of Black participants may have limited our power to identify a significant interaction. The results were consistent in a sensitivity analysis when only Black and White participants were considered (excluding other races), except for a significant interaction between aspirin dose and race for stroke. In Black participants, the 325 mg aspirin dose was associated with a significant reduction in the risk of stroke compared with the 81 mg aspirin dose but not in White participants (adjusted p value for interaction: 0.028). However, given that the absolute stroke event rate is very low, that this is a post hoc analysis, that the main comparison between aspirin doses was neutral for strokes and that no penalty for multiple comparisons were made, this finding should be viewed as exploratory and hypothesis-generating only.
Our study has limitations worth mentioning. First, race was self-reported, which may be associated with a reporting bias that is not possible to quantify. Random misclassification of race would, however, tend to bias our estimate towards the null. Second, although substantial efforts were made to enrol a diverse and inclusive patient population in ADAPTABLE with the help of a patient–partner advisory board, a large majority of participants were White (85.1%), and as noted above, the small number of Black participants may have limited our power to detect a difference. Third, comparisons across races in this randomised trial should be viewed as hypothesis generating and may be subject to confounding by unmeasured variables. Fourth, our study did not collect blood samples to determine platelet reactivity while on aspirin. Fifth, race was not available for 980 participants (6.5%) who could not be included in the analyses. This may potentially impact the external generalisability of the results if the distribution of baseline characteristics and of outcome rates across race groups differs in these excluded patients compared with patients included in the analysis. Sixth, the potential modifying effect of race on aspirin dose could not be assessed for clinically relevant bleeding that did not require transfusions because the latter were not captured, which may underestimate the treatment effect. Finally, the differences in adherence rates across races add additional complexity to the interpretation of the associations between aspirin dose and race observed in this analysis.
In conclusion, in this secondary analysis of the ADAPTABLE randomised trial, race was not an effect modifier on the impact of aspirin dosing on effectiveness and safety in patients with established ASCVD. In clinical practice, treatment decisions regarding aspirin dose in secondary prevention of ASCVD should not be influenced by race.
Ethics statements
Patient consent for publication.
Not applicable.
Ethics approval
This study involves human participants and was approved by The Duke University IRB approved the overall program. The IRB # is Pro00068525. Participants gave informed consent to participate in the study before taking part.
Acknowledgments
We thank the Adaptors (patient partner investigators) involved in the conduct of the trial: Desiree Davidson, Kevin Edgley, Greg Merritt, PhD, Linda S. Brown, Henry Cruz, Nadina Zemon, Tom E. McCormick III, MS, Jacqueline Alikhaani, BA, Ken C. Gregoire, and Bill Larsen (in memoriam).
- Abate KH , et al
- Douglas PS ,
- Peterson ED , et al
- Keshvani N ,
- Khera R , et al
- Wayangankar SA ,
- Kennedy KF ,
- Aronow HD , et al
- Edmund Anstey D ,
- Thomas L , et al
- Pun PH , et al
- Al-Khatib S ,
- Lopes RD , et al
- Baigent C ,
- Blackwell L ,
- Collins R , et al
- Théroux P ,
- McCans J , et al
- Arnett DK ,
- Blumenthal RS ,
- Albert MA , et al
- Bainey KR ,
- Cantor WJ , et al
- Saraste A , et al
- Visseren FLJ ,
- Smulders YM , et al
- Friede KA ,
- San TR , et al
- Wruck LM , et al
- Marquis-Gravel G ,
- Robertson HR , et al
- Elm E von ,
- Altman DG ,
- Egger M , et al
- Faulkner M ,
- Merritt G , et al
- Alikhaani J ,
- Brown L , et al
- Patrono C ,
- García Rodríguez LA ,
- Landolfi R , et al
Supplementary materials
Supplementary data.
This web only file has been produced by the BMJ Publishing Group from an electronic file supplied by the author(s) and has not been edited for content.
- Data supplement 1
- Data supplement 2
Contributors GMG is responsible for the overall content [as guarantor]. GMG: conception, writing and editing. HM: conception, statistical analyses and editing. LMW: conception, statistical analyses and editing. CPD: critical review and editing. MBEF: critical review and editing. PM: critical review and editing. SG: critical review and editing. KG: critical review and editing. SK: critical review and editing. DM: critical review and editing. TSP: critical review and editing. JW: critical review and editing. RAH: critical review and editing. RLR: critical review and editing. AFH: conception, critical review and editing. WSJ: conception, writing and editing.
Funding This secondary analysis of ADAPTABLE has been funded by Bayer (investigator initiated research grant). The ADAPTABLE trial was upported through a Patient-Centered Outcomes Research Institute (PCORI) Award (ASP-1502-27029).
Disclaimer GMG: honoraria from Pharmascience, JAMP Pharma, Bayer, KYE, Novartis, Population Health Research Institute, and Canadian Heart Research Center; Research funding from Canadian Institutes of Health Research, Fonds de Recherche du Québec – Santé, Duke Clinical Research Institute, Bayer, Université de Montréal, Montreal Heart Institute; MBE: equity in and receives a pension from Eli Lilly and Company; SG is funded by the National Heart, Lung and Blood Institute (NHLBI, R56HL158803), and receives funding from the American Heart Association for editorial work. SKG: research PI for Medtronic, Boston Scientific and Abbott; RLR declares family-owned stocks from Moderna (not related to this study). WSJ: grants from Patient-Centered Outcomes Research Institute during the conduct of the study; grants from Boehringer Ingelheim, grants and personal fees from Bayer, grants and personal fees from Janssen Pharmaceuticals, personal fees from Bristol-Myers Squibb, grants from Merck, personal fees from Medscape, outside the submitted work. The other authors have no conflict to declare.
Competing interests None declared.
Patient and public involvement Patients and/or the public were involved in the design, conduct, reporting or dissemination plans of this research. Refer to the Methods section for further details.
Provenance and peer review Not commissioned; externally peer reviewed.
Supplemental material This content has been supplied by the author(s). It has not been vetted by BMJ Publishing Group Limited (BMJ) and may not have been peer-reviewed. Any opinions or recommendations discussed are solely those of the author(s) and are not endorsed by BMJ. BMJ disclaims all liability and responsibility arising from any reliance placed on the content. Where the content includes any translated material, BMJ does not warrant the accuracy and reliability of the translations (including but not limited to local regulations, clinical guidelines, terminology, drug names and drug dosages), and is not responsible for any error and/or omissions arising from translation and adaptation or otherwise.
Read the full text or download the PDF:

An official website of the United States government
The .gov means it’s official. Federal government websites often end in .gov or .mil. Before sharing sensitive information, make sure you’re on a federal government site.
The site is secure. The https:// ensures that you are connecting to the official website and that any information you provide is encrypted and transmitted securely.
- Publications
- Account settings
Preview improvements coming to the PMC website in October 2024. Learn More or Try it out now .
- Advanced Search
- Journal List
- Int J Environ Res Public Health

Statistical Advances in Epidemiology and Public Health
Domenica matranga.
1 Department of Health Promotion, Mother and Child Care, Internal Medicine and Medical Specialties, University of Palermo, 90127 Palermo, Italy
Filippa Bono
2 Department of Economics, Business and Statistics, University of Palermo, 90128 Palermo, Italy; [email protected]
Laura Maniscalco
3 Department of Biomedicine, Neuroscience and Advanced Diagnostics, University of Palermo, 90127 Palermo, Italy; [email protected]
Associated Data
No new data were created or analyzed in this study. Data sharing is not applicable to this article.
The key role of statistical modeling in epidemiology and public health is unquestionable. The methods and tools of biostatistics are extensively used to understand disease development, uncover the etiology, and evaluate the development of new strategies of prevention and control of the disease. Through data analysis, epidemiology can steer decision-making processes, guide health and healthcare policy, and plan and assist in the management and care of health and disease in individuals. The growing availability of large healthcare databases allows drawing new evidence in the use of healthcare interventions, drugs, and devices, and in the knowledge of population health and health inequality. Clinical decisions grounded on evidence of efficacy and safety of medical interventions can contribute to prolonging people’s lives, improving their quality, and promoting appropriateness [ 1 ].
The present Special Issue focuses on statistical methods applied to epidemiology and public health. It is advisable to enlarge the evidence-based approach to a value-based approach by including the quality and the costs dimensions from both the demand and the supply of healthcare services [ 2 ]. Thus, particular attention has also been given to the evaluation and the cost-effectiveness of procedures and services. This special issue includes twelve articles from research teams worldwide, specifically Belgium, Spain, China, Canada, Austria, and Romania, apart from Italy. These studies focus on epidemiology, public health, and health promotion [ 3 , 4 , 5 , 6 ], suggest appropriate statistical methodologies for specific research questions [ 7 , 8 , 9 , 10 , 11 ], and analyze the quality of services provided to patients [ 12 , 13 , 14 ].
Of the four articles in the field of public health, two studies focus on disease prevention and health promotion strategies. In detail, Matranga et al. aim to assess whether the adoption of healthy behaviors could be significantly associated with psychological well-being in a cohort of students in the healthcare area [ 3 ]. This study shows that students inclined to well-being consider healthcare professionals as models for their patients and all people in general. Furthermore, the positive relationship between a virtuous lifestyle and psychological well-being suggests the construction, development, and growth of individual skills to counteract unhealthy behaviors. The study of Fujihara et al. addresses the issue of health promotion turning to a different target [ 4 ]. Through a prospective cohort design on older people in Japan, it focuses on examining the possible association between community-level social capital and the incidence of Instrumental activities of daily living (IADL) disability. The outcomes obtained from the present investigation show that community-based interventions that are finalized to promote community-level social capital could help preventing IADL disability or reducing its incidence.
In the field of occupational epidemiology, the Special Issue includes two studies regarding job exposures, and one of these uses large databases [ 5 ]. Specifically, Maniscalco et al. investigate to what extent a change in employment contributes to cardiovascular, musculoskeletal, and neuropsychological health [ 5 ]. Using a sample of 10,530 Belgian workers in a seven-year follow-up study period, the authors find an increased risk of cardiovascular disease and a psychosocial load in association with a job change experience. The issue of students’ professional exposures has been considered by Verso et al. [ 6 ]. The authors scrutinize the prevalence of latent tuberculosis infection (LTBI) among undergraduate and postgraduate students in the healthcare area at the University of Palermo, Italy, and investigate about the occupational risk of infection among students. This study shows very few cases of LTBI, confirming that the incidence of LTBI is low among Italian students. Not only healthcare workers, but also healthcare students involved in traineeships, are daily exposed to the occupational risk of contracting tuberculosis, due to close and prolonged contact with patients. Effective prevention strategies are mandatory for University hospitals.
Regarding the articles of this Special Issue that are focused on statistical methodology, we advocate reading the study of Trivelli et al. [ 7 ] describing the spatio-temporal distribution of cardiovascular mortality, and the study of Maniscalco et al. [ 8 ] suggesting a data-driven approach to investigate the interrelationship among health indicators. These two papers give important contributions in the context of environmental epidemiology and health promotion. Specifically, the study of Trivelli et al. aims to determine the spatio-temporal association between environmental exposure to particulate matter, PM2.5 µg/m 3 , and the risk of cardiovascular mortality in the 2010–2015 period in an Italian region with a high level of air pollution and human activities, using a Bayesian smoothed approach [ 7 ]. Such an approach consists of a hierarchical mixed log-linear model with a Besag, York, and Mollié (BYM) random component in a fully Bayesian framework. As described by the authors in detail, this model produces a smoothed map of relative risks (RR) and allows extra-Poisson variability induced by the spatio-temporal data structure. The proposed model includes two Gaussian random effects, one spatially unstructured (exchangeable or white noise in the lattice) and one spatially structured (conditional autoregressive based on a Gaussian–Markov random field in the lattice). Through the smoothed maps of RRs, the authors show that the distribution of estimated risk of death for cardiovascular diseases did not change across the years between 2010 and 2015, and show evidence of three clusters of high-risk for cardiovascular diseases in Lomellina area in all the studied years.
The study of Maniscalco et al. in the field of health promotion attempts to investigate the interrelationship among statistical indicators, which are typically used to capture health multidimensionality of elderly people [ 8 ]. They are self-perceived health (SPH), quality of life (QoL) in older ages, chronic or non-communicable diseases (NCDs), global activity limitation, lifestyle, and cognitive functioning. The authors analyze data of people aged 50 or above, living in twenty-seven European countries and Israel, extracted from the Survey on Health, Ageing, and Retirement in Europe (SHARE). Through additive Bayesian network modeling, the authors consider all the indicators jointly and identify all direct and indirect relationships between them. Three directed acyclic graphs for each one of Spain, Italy, and Greece show SPH significantly associated with cognitive functioning and QoL of people aged 50 and above, and confirm the well-known association with chronic diseases. Two of the studies composing the Special Issue contribute in the field of generating real-world evidence, through a census of the available healthcare utilization databases [ 9 ] and through a study that accounts for immortal time bias in observational clinical studies [ 10 ]. These two papers supply an example of the importance of the information provided by healthcare administrative databases, which can be used in many fields of pharmacoepidemiology, such as safety, efficacy, and cost–efficacy of therapeutic strategies.
Specifically, Skrami et al. perform a census of the available Healthcare Utilization databases (HUD) across 19 Italian regions and two autonomous Trento and Bolzano provinces [ 9 ]. This paper is worthy of note, as many studies use data from HUDs to produce scientific evidence about the safety and efficacy profile of drugs. However, in order to combine the HUDs and compare diagnostic and therapeutic care pathways (PDTA) and protocols among different Italian regions, it is essential that the HUDs are harmonized and their information comparable. The work of Skrami et al. counts 352 HUDs between January 2014 and October 2016 that cover the whole population of a single region and recorded local-level data referred to the healthcare service; these databases are classified as healthcare services, conditions/diseases, and others, on the basis of the recorded observational unit. The authors find that the HUDs are homogeneous with respect to the unique personal identification code, the anonymization technique, and the DMS adopted, so that the record linkage across them is always possible. Additionally, the classification systems for diseases and drugs are found to be homogeneous across regions, while the anonymization procedures are not. This work can be considered as a model for other countries that wish to inventory their available HUDs to ease multicentric epidemiologic studies.
The study of Corrao et al. is a valuable example of real-world evidence that can be extracted from the merging of HUDs [ 10 ]. The authors aim to investigate the association between third-trimester exposure to macrolide antibiotics and the risk of preterm delivery as primary outcome and low birth weight (less than 2500 g), with smallness for gestational age and five-minutes Apgar score <7 as secondary outcomes. The merged HUDs are (1) the electronic database of the NHS beneficiaries, (2) the outpatient drugs dispensed in community pharmacies, and (3) the specialist visits and diagnostic examinations reimbursable by the NHS and the database of the Certificates of Delivery Assistance of all of Lombardy in the period between 2007 and 2017. The merging procedure gives arise to a database of 549,082 mothers with their newborns. From the methodological point of view, this paper contributes for considering the so-called immortal bias, the time window between the index date (27th gestational week) and the start of exposure (first antibiotics prescription) in which the event of interest (preterm birth) is not possible by design.
Finally, the last methodological paper of this special issue is concerned with managing data separation by logistic regression [ 11 ], which is a frequent problem in the analysis of small or sparse clinical datasets. Data can be defined as separated if there exists one covariate or a linear combination of covariates that allows a perfect prediction of some or all observations of the dataset. The authors describe several situations a medical data-analyst is faced with, as the occurrence of unbalanced outcomes [ 15 ], rare exposures, as in the case of case-control studies with controls free of any local and systemic variables [ 16 ], correlated covariates, and sparse data. The authors aim to compare Firth’s penalization, which is widely used to deal with data-separation, with the maximum likelihood method applied to an augmented dataset. Through a well-done data simulation study, they show that bringing more sampling data is not a cost-adjusted relative efficient strategy compared to logistic regression with Firth penalization.
In our Special Issue, quality evaluation of healthcare is addressed from both the patient’s point of view, in terms of quality perception of healthcare services, and from the healthcare point of view, in terms of efficiency and efficacy of care. From the patients’ viewpoint, Druică et al. investigate patients’ health services satisfaction with health services [ 12 ]. On a cross-sectional sample of 1500 Romanian patients, the authors use a partial least square–path modeling approach (PLS–PM) to determine their health services satisfaction. The authors develop a variance-based structural model, emphasizing the mediating role of trust and satisfaction with various health services categories. Results show the mediating role of trust in shaping the relationship between the procedural accuracy of health professionals, the perceived intensity of their interaction with patients, and patients’ experienced quality of the health services. As the most relevant variable for intervention, the authors detect the degree of attention patients perceive to have received. The paper suggests three methods to turn waiting time into attention deserved to patients.
Gálvez et al. evaluate hospitals’ efficiency by comparing a new model of organizational innovation based on Advanced Practice Nurse in the care of people with Ostomies (APN-O) versus usual care [ 13 ]. The study involves twelve Andalusian hospitals that implement this model. On a total of 75 patients followed-up for six months, the authors analyze clinical outcomes, healthcare resources, health-related quality of life, and willingness to pay. The economic evaluation takes into consideration the healthcare direct and indirect cost and finds evidence of an increased value-based healthcare in ostomies. This study suggests that APN-O is an effective and highly efficient patient management model for improving patients’ health status.
Li et al. deal with different objectives in the efficiency evaluation of Chinese hospitals [ 14 ]. In a first step, efficiency and change in efficiency are evaluated using Data Envelopment Analyses and the Malmquist index. The study considers 29 provinces, where data from 1336 hospitals are observed in a time-span from 2003 to 2016; in a second step of the study, starting from the efficiency differences calculated in the first step of the analysis, the authors use the Theil index to obtain the efficiency decomposition. Then, they find the inefficiency determinants through the Grey correlation analysis. The study results show that the township hospitals achieve efficiency gains in most provinces and that the intra-regional difference is the major cause of the overall efficiency scores’ difference. This paper further examines the Grey correlations between overall provincial efficiency difference of Chinese township hospitals and the determinants of such a difference, within each of the eastern, central, and western regions of China.
In conclusion, the authors suggest that local governments should take measures to improve the level of education, increase public financial support for township hospitals, and guide household expenditure to invest more on health care and medical services through public education, so as to shrink the differences among provinces. Furthermore, township hospitals in relatively backward provinces should not ignore the effects of increasing the proportion of licensed doctors and assistant doctors, and the proportion of managerial personnel in the total number of medical personnel.
The Special Issue encompasses different and valuable works related to what extent statistical disciplines are important in the fields of epidemiology, public health, and health promotion. The selected studies for this Special Issue contribute relevant information that may help suggesting appropriate statistical methodologies for specific biomedical research questions and analyzing the quality of services provided in public health. Furthermore, it highlights several statistical methods currently used in public health and epidemiological studies and some of the frequent problems encountered in the analysis of clinical datasets.
Acknowledgments
As Guest Editors of this Special Issue, we would like to acknowledge the contribution of all the authors that participated in this Special Issue, for sharing their expertise and research within this topic. Finally, we are grateful to the editorial staff for the support in the management of this Special Issue.
Author Contributions
D.M., F.B. and L.M. were all responsible for the conceptualization of the paper and for the original draft preparation, as well as for reviewing and editing the final version of the manuscript. All authors have read and agreed to the published version of the manuscript.
This research received no external funding.
Institutional Review Board Statement
Not applicable.
Informed Consent Statement
Data availability statement, conflicts of interest.
The authors declare no conflict of interest.
Publisher’s Note: MDPI stays neutral with regard to jurisdictional claims in published maps and institutional affiliations.
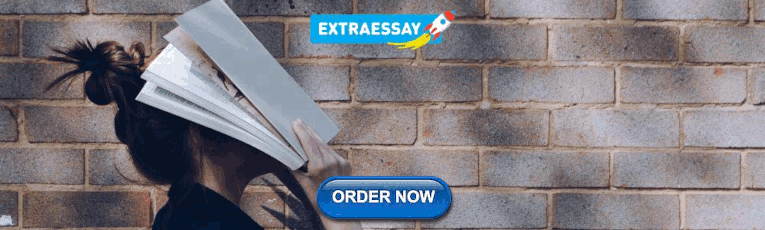
IMAGES
COMMENTS
Introduction. Statistical analysis is necessary for any research project seeking to make quantitative conclusions. The following is a primer for research-based statistical analysis. It is intended to be a high-level overview of appropriate statistical testing, while not diving too deep into any specific methodology.
Correlation analysis has three general outcomes: (1) the two variables rise and fall together; (2) as values in one variable rise, the other falls; and (3) the two variables do not appear to be systematically related. To make those determinations, we use the correlation coefficient (r) and related p value or CI.
Introduction. This two-part series will present basic statistical principles for the practicing physician to use in his or her review of the literature and to the physician engaged in clinical research. The purpose of this series is threefold: (1) to provide an overview of common epidemiological and statistical terms and concepts that can be ...
Results. Although biostatistical inputs are critical for the entire research study (online supplemental table 2), biostatistical consultations were mostly used for statistical analyses only 15.Even though the conduct of statistical analysis mismatched with the study objective and DGP was identified as the major problem in articles submitted to high-impact medical journals. 16 In addition ...
Design. In many ways the design of a study is more important than the analysis. A badly designed study can never be retrieved, whereas a poorly analysed one can usually be reanalysed. (1) Consideration of design is also important because the design of a study will govern how the data are to be analysed. Most medical studies consider an input ...
This module will introduce you to some of the key building blocks of knowledge in statistical analysis: types of variables, common distributions and sampling. You'll see the difference between "well-behaved" data distributions, such as the normal and the Poisson, and real-world ones that are common in public health data sets. What's ...
Statistical Methods in Medical Research is a highly ranked, peer reviewed scholarly journal and is the leading vehicle for articles in all the main areas of medical statistics and therefore an essential reference for all medical statisticians. It is particularly useful for medical researchers dealing with data and provides a key resource for medical and statistical libraries, as well as ...
March 22, 2022. This JAMA Guide to Statistics and Methods discusses instrumental variable analysis, a method designed to reduce or eliminate unobserved confounding in observational studies, with the goal of achieving unbiased estimation of treatment effects. Research, Methods, Statistics Guide to Statistics and Methods.
Ruth Etzioni, PhD has been on the faculty at the Fred Hutchinson Cancer Research Center since 1991 and is an affiliate professor of biostatistics and health services at the University of Washington. She develops statistical models and methods for health policy and is a member of national cancer policy panels including the American Cancer Society and the National Comprehensive Cancer Network.
Includes a comprehensive review of statistical methods and new approaches in health research to guide the reader in learning efficiently. Covers new developments in EHR data analysis, clinical trials, FDR, big network analytics, applications in GWAS, graphical modeling in genomic studies, survival analysis, and functional data analysis.
A hands-on guide to using statistics in health research, from planning, through analysis, and on to reporting. A Practical Approach to Using Statistics in Health Research offers an easy to use, step-by-step guide for using statistics in health research.The authors use their experience of statistics and health research to explain how statistics fit in to all stages of the research process.
All-Access Plan. One Year Subscription. $499. Enroll in all three courses in the Medical Statistics program. View and complete course materials, video lectures, assignments and exams, at your own pace. Revisit course materials or jump ahead - all content remains at your fingertips year-round.
In order to interpret research datasets, clinicians involved in clinical research should have an understanding of statistical methodology. This article provides a brief overview of statistical methods that are frequently used in clinical research studies. Descriptive and inferential methods, including regression modeling and propensity scores ...
However, the complexity of statistics and statistical analysis presents a hurdle in adopting research findings into everyday clinical practice. 1,2 Accurately interpreting statistical data is not just a technical skill but a cornerstone for quality patient care. 3 This becomes increasingly important as healthcare organizations rely more on ...
Introduction. Statistical analysis is necessary for any re-search project seeking to make quantitative conclusions. The following is a primer for research-based statistical analysis. It is intend-ed to be a high-level overview of appropriate statistical testing, while not diving too deep into any specific methodology.
Recently, a systematic review study identified nonadherence to standards of methodological approaches required by the Agency for Healthcare Research and Quality for research based on the National Inpatient Sample database even in high‐quality publications. 1 It is found that the appropriate use of methods and their standardized reporting ...
Modern Statistical Methods. for Health Research. Series: Emerging Topics in Statistics and Biostatistics. Features the significant contributions of many leading statisticians and data. scientists ...
This article provides a brief overview of statistical methods that are frequently used in clinical research studies. Descriptive and inferential methods, including regression modeling and propensity scores, are discussed, with focus on the rationale, assumptions, strengths, and limitations to their application.
This specialisation consists of four courses - statistical thinking, linear regression, logistic regression and survival analysis - and is part of our upcoming Global Master in Public Health degree, which is due to start in September 2019. The specialisation can be taken independently of the GMPH and will assume no knowledge of statistics ...
1. Introduction. Statistical modeling methods [Citation 1-17] are widely used in clinical science, epidemiology, and health services research to analyze and interpret data obtained from clinical trials as well as observational studies of existing data sources, such as claims files and electronic health records.Diagnostic and prognostic inferences from statistical models are critical if ...
SAS. 8. Minitab. 9. Statistica. 10. Excel. Kolabtree freelance statistician Kingsley Ukwuoma writes about the top statistical tools used in medical research and clinical data analysis . There was a time when validating experiments through data was done fully using manual computation.
Abstract. Statistics plays a crucial role in research, planning and decision-making in the health. sciences. Progress in technologies and continued research in computational statistics has ...
The analysis of health data employs various methods and techniques to extract relevant information from collected data. The starting point for exploring these data is often descriptive analysis. This involves using descriptive statistics like the mean, median, and standard deviation, alongside visualization techniques such as graphs and tables ...
Each unit includes a data analysis exercise to consolidate understanding; The assessment consists of statistical problems in health research designed to give insight into real statistical problems in healthcare and to test ability to apply and understand correct statistical analysis. Academic Credit. Applicants may take this course for academic ...
Objectives To evaluate whether the effectiveness and safety of low (81 mg daily) versus high-dose (325 mg daily) aspirin is consistent across races among patients with established atherosclerotic cardiovascular disease (ASCVD). Design A secondary analysis of the randomised controlled trial ADAPTABLE was performed. Setting The study was conducted in 40 centres and one health plan participating ...
The key role of statistical modeling in epidemiology and public health is unquestionable. The methods and tools of biostatistics are extensively used to understand disease development, uncover the etiology, and evaluate the development of new strategies of prevention and control of the disease. Through data analysis, epidemiology can steer ...
All analyses were conducted in R statistical software version 3.6.3 (R Project for Statistical Computing); the multiple location and baseline ITS GAMs were fitted using the R package mgcv while the simple ITS models were pooled using metafor. 32,33 P values were 2-sided, and statistical significance was set at P < .05. Data were analyzed ...
Background CT performed for various clinical indications has the potential to predict cardiometabolic diseases. However, the predictive ability of individual CT parameters remains underexplored. Purpose To evaluate the ability of automated CT-derived markers to predict diabetes and associated cardiometabolic comorbidities. Materials and Methods This retrospective study included Korean adults ...